INTRODUCTION
Researchers have long recognized that knowledge sharing (KS) among employees allows organizations to exploit and capitalize on knowledge-based resources, which contribute to knowledge application, innovation, and ultimately the competitive advantage of the organization (Jackson, Chuang, Harden, Jiang, & Joseph, Reference Jackson, Chuang, Harden, Jiang, Joseph and Joseph2006). To pursue the alleged benefits of KS, many organizations have deployed virtual communities to foster online KS among employees (Lee & Choi, Reference Lee and Choi2003; Wellman, Salaff, Dimitrova, Garton, Gulia, & Haythornthwaite, Reference Wellman, Salaff, Dimitrova, Garton, Gulia and Haythornthwaite1996). The literature on online communities suggests a rational choice perspective (Grandori, Reference Grandori1997), which puts a premium on understanding the attributes of users (Jeppesen & Fredriksen, Reference Jeppesen and Frederiksen2006), aligning the motivations of participation and contribution (Shah, Reference Shah2006), and deploying formal and informal governance mechanisms within online communities (Li-Ying & Salomo, Reference Li-Ying and Salomo2013). The underlying logic embedded in such a stream of literature implies that as long as users with appropriate attributes in online communities are motivated according to their attributes and corresponding governance is in place, KS will somehow occur (Cook, Reference Cook2008).
However, several studies find that some factors may hinder KS behaviors in virtual communities (Chen & Hung, Reference Chen and Hung2010; Hsu, Ju, Yen, & Chang, Reference Hsu, Ju, Yen and Chang2007); thus online virtual communities need facilitators as a supporting tool to reduce KS barriers and enhance the effectiveness of KS enablers, such as corporate culture and trust, which eventually cause KS behaviors to actually take place (Ardichvili, Reference Ardichvili2008). The role of facilitation in online KS communities needs more research attention (Cacciamani, Cesareni, Martini, Ferrini, & Fujita, Reference Cacciamani, Cesareni, Martini, Ferrini and Fujita2012) because the literature to date has not clearly addressed the effectiveness of specific supporting tools in online KS communities and the corresponding outcomes for organizations with respect to the KS behaviors of online users (employees) (Chen & Hung, Reference Chen and Hung2010; Wang & Noe, Reference Wang and Noe2010). Even worse, the literature on KS is unclear about whether KS behavior creates positive outcomes that benefit organizations (Haas & Hansen, Reference Haas and Hansen2007; Wang & Noe, Reference Wang and Noe2010).
To contribute to this underdeveloped research area, in this study we focus on the effectiveness of a specific (and a rather unconventional) type of supporting mechanism of online KS virtual communities within an organization: hidden knowledge facilitators. Recently, we have observed some firms starting to use a small number of employees to facilitate KS in intrafirm online R&D communities without revealing their identities as facilitators. These hidden knowledge facilitators (HKFs) are responsible to the firm, which ‘hires’ them to influence the interactions on the intrafirm online R&D community in hopes of enhancing KS and innovations. These HKFs actively participate in the online KS communities and may create a bandwagon effect for other regular community members to follow (Leibenstein, Reference Leibenstein1950) – a phenomenon that is similar to that in consumer psychology where increasing demand creates more demand (van Herpen, Pieters, & Zeelenberg, Reference van Herpen, Pieters and Zeelenberg2009). The intentions of these HKFs are to serve the company as a whole, and employees who seek new knowledge in particular, by facilitating online KS within the firm. The firm's management imposes a job mandate on these HKFs to facilitate online R&D communities by boosting online KS behaviors, while HKFs’ job mandate and performance objectives are hidden from regular employees, who might not be willing to share their specific knowledge with colleagues, should they know the online traffic of discussion is somehow ‘manipulated’ by HKFs. The special status and functions of HKFs in firms’ online R&D communities provide us with a perfect research context for investigating how effective HKFs are at making their online contributions to yield positive KS outcomes for their organization.
In this study, we ask a research question: to what extent does the quantity and quality of HKFs’ online interventions effectively lead to positive knowledge sharing outcomes? The answer to this question is important for managers to understand how to use HKFs as a supporting mechanism to enhance intraorganizational KS. To understand the effectiveness of HKFs, several theories, including user communities in innovation management, the bandwagon effect in sociology, and consumer psychology, are relevant. However, none of them alone sufficiently explains the effect of HKFs. In our research design, we focus on the KS literature and take the phenomenon of HKFs as a specific case to highlight the effectiveness of a supporting mechanism for online KS, while drawing on insights from several relevant theories to build argumentation for hypothesis development. Using log data from an intrafirm online R&D KS community in a large Chinese company, we use a netnographic method based on extensive content analysis to test several hypotheses about the relationships between the quantity and quality of HKFs’ online interventions and KS outcomes among employees. In Table 1, we summarize the research design and conceptual model, on which several hypotheses will be developed, and the related methodology will be detailed in the next sections.
Table 1. Research design and conceptual model

We pay special attention to three concrete KS outcomes: enhanced online discussion (reflected in the length of discussion), problem resolution, and convergent and divergent thinking. This is because these measurable KS outcomes, respectively, correspond closely to the three major KS facilitation functions suggested theoretically by prior studies (Cabrera & Cabrera, Reference Cabrera and Cabrera2002), namely, encouraging communication, decreasing KS cost and increasing KS benefit, and increasing perceived efficacy of contributors.
This study makes a direct contribution to the literature on KS on virtual platforms in general and the design of intrafirm online knowledge community in particular. First, in this study, we clearly show to what extent HKFs’ interventions affect organizational KS outcomes. Thus, a missing link in the literature on KS regarding whether KS behavior creates positive outcomes that benefit organizations (Haas & Hansen, Reference Haas and Hansen2007; Wang & Noe, Reference Wang and Noe2010) is established with empirical evidence. Second, in a more nuanced sense, this study pinpoints the importance of considering both the quantity and the quality of HKFs’ interventions when an organization designs its online KS communities. We suggest managers at any organization deploying or considering deploying HKFs in their KS intranet to pay attention to the joint properties of quantity and quality in the work of HKFs.
THEORETICAL BACKGROUND
Knowledge Sharing
An organization's capacity to share knowledge among its employees and apply that shared knowledge to perform important activities is increasingly seen as a vital source of competitive advantage (Damodaran & Olphert, Reference Damodaran and Olphert2000; Jackson et al., Reference Jackson, Chuang, Harden, Jiang, Joseph and Joseph2006). Knowledge sharing refers to the provision of task information and individual expertise to help and collaborate with others to solve problems, develop new ideas, or implement policies or procedures. This process takes place via different means of communications, including the traditional paper format or various electronic formats (Cummings, Reference Cummings2004; Haas & Hansen, Reference Haas and Hansen2007). Recently, KS via digital means such as online discussion forums and communities has become extremely popular among various organizations (Cacciamani et al., Reference Cacciamani, Cesareni, Martini, Ferrini and Fujita2012; Ma & Yuen, Reference Ma and Yuen2011).
KS is believed to benefit organizations. In a recent review of KS, Wang and Noe (Reference Wang and Noe2010) found research has shown that KS makes a potentially positive impact on firms’ performance in terms of reductions in production costs, rapid completion of new product development projects, effective team performance, innovation capabilities, and consequent increases in sales and revenue from new products and services. However, as KS also bears costs and barriers both within and across organizations, obtaining and using knowledge from other parts of a firm does not necessarily guarantee improved firm performance (Haas & Hansen, Reference Haas and Hansen2005). Therefore, recent studies have emphasized that scholars need to move beyond studying the facilitating conditions for KS and pay more attention to examining whether and how facilitated KS eventually leads to positive organizational outcomes (Haas & Hansen, Reference Haas and Hansen2007).
The literature also recognizes that sharing codified and tacit knowledge within and across organizations by either electronic or interpersonal means (Bordia, Irmer, & Abusah, Reference Bordia, Irmer and Abusah2006) does not take place by itself, because KS intention and behavior are influenced by organizational culture and climate (Taylor & Wright, Reference Taylor and Wright2004), personal attributes (Cabrera, Collins, & Salgado, Reference Cabrera, Collins and Salgado2006), and incentives (Shah, Reference Shah2006; Wasko & Faraj, Reference Wasko and Faraj2005) – a complex system that needs management support and facilitation (Kulkarni, Ravindran, & Freeze, Reference Kulkarni, Ravindran and Freeze2006). Therefore, intervention mechanisms and management support are needed to facilitate KS (Cabrera et al., Reference Cabrera, Collins and Salgado2006).
Motivating and Bandwagon Effect: The Need for Knowledge Sharing Facilitation
The need for KS facilitation within organizations and on virtual KS communities in particular can be understood based on at least two streams of literature: one is on how to motivate online KS participation and contribution, and the other is about creating a bandwagon effect.
With respect to the first, when an organization establishes and launches an online KS platform, there is no guarantee that employees will find it interesting to participate and share knowledge on it; in fact, there is a potential lack of motivation to do so. Many case studies have demonstrated that people are motivated to join online KS communities for very different reasons (Dahlander & Magnusson, Reference Dahlander and Magnusson2005; Dutton, Reference Dutton2008; Nambisan, Reference Nambisan2002; Nambisan & Baron, Reference Nambisan and Baron2007; Shah, Reference Shah2006; von Hippel & von Krogh, Reference von Hippel and von Krogh2003; West & O'Mahony, Reference West and O'Mahony2008). While some find contributing to a particular community intrinsically interesting, others are extrinsically motivated to seek solutions to their specific needs (Jeppesen & Frederiksen, Reference Jeppesen and Frederiksen2006; Shah, Reference Shah2006). A firm normally needs to foster community interactions among differently motivated users, create a sense of belonging, and show strong commitment from top management (Ma & Yuen, Reference Ma and Yuen2011). Providing appropriate incentives for altering or manipulating one's motivations is a key function of KS facilitation (Sundaramurthy & Lewis, Reference Sundaramurthy and Lewis2003; Walsh & Seward, Reference Walsh and Seward1990).
With respect to the second stream of literature, when a small number of employees can be motivated to start sharing knowledge, ideally many others will follow, creating a so-called bandwagon effect. As a non-functional demand in economics, the bandwagon effect refers to the extent to which the demand for a commodity increases because other people are buying the same commodity (Leibenstein, Reference Leibenstein1950). Early studies in economics on demand theory and theories in consumer psychology have noticed non-additivity in consumer demand, which has links to herd behavior (Banerjee, Reference Banerjee1992; Bikhchandani, Hirshleifer, & Welch, Reference Bikhchandani, Hirshleifer and Welch1998; Corneo & Jeanne, Reference Corneo and Jeanne1997) and the psychological theories of conformity and social influence (Asch, Reference Asch1955; Baron, Vandello, & Brunsman, Reference Baron, Vandello and Brunsman1996). Bandwagon effects occur when consumers follow the behavior of others, either because they want to get ‘into the swim of things’, or conform with the people they wish to associate with (Berger & Heath, Reference Berger and Heath2007; Escalas & Bettman, Reference Escalas and Bettman2005), or because they believe that the choice behavior of others reveals a product's uniqueness (van Herpen et al., Reference van Herpen, Pieters and Zeelenberg2009) or superior quality (Huang & Chen, Reference Huang and Chen2006; Kardes, Posavac, & Cronley, Reference Kardes, Posavac and Cronley2004), which they cannot afford to go without. The behavior of others thus provides additional clues as the consumer creates a mental shortcut used as a judgment rule for making quick evaluations. This is the so-called bandwagon heuristic (Sundar, Reference Sundar, Metzger and Flanagin2007; Sundar, Oeldorf-Hirsch, & Xu, Reference Sundar, Oeldorf-Hirsch and Xu2008). In other cases, the presence and observable act of an expert might trigger the expert heuristic, which leads directly to positive evaluations of an expert's statement without scrutiny of its content. Often, the joint forces of the bandwagon heuristic and the expert heuristic create a bandwagon effect on actors’ social behavior.
In the context of KS within organizations, as organizational, cultural, and structural hurdles prevail and prevent employees from actively sharing knowledge, various kinds of business practices are deployed to create a bandwagon effect that can benefit a firm. For instance, knowledge facilitators in an online education and training environment have received some research attention, particularly with respect to the role of online tutor support around facilitator styles and metacognition (Cacciamani et al., Reference Cacciamani, Cesareni, Martini, Ferrini and Fujita2012). Among other things, online facilitator support contributes to the educational success of online education activities, as the facilitators play their roles as instructors, facilitators, and moderators (Cesareni et al., Reference Cesareni, Albanese, Cacciamani, Castelli, De Marco, Fiorilli, Luciani, Mancini, Martini, Vanin and Varisco2008). The basic premise in this stream of research is that a moderated online community is preferable to a non-moderated one, because the bandwagon effect can be created and utilized by online community facilitators (Wise, Hamman, & Thorson, Reference Wise, Hamman and Thorson2006).
Knowledge Sharing Facilitations and KS Outcomes
A vast majority of the research on KS has focused on what factors motivate or hinder KS behavior without addressing whether KS behaviors actually lead to positive outcomes for organizations (e.g., Gagné, Reference Gagné2009; Pee & Min, Reference Pee, Pee, Min and Min2017; Reinholt, Pedersen, & Foss, Reference Reinholt, Pedersen and Foss2011). In other words, the literature has provided us with rich insights on why people share (or do not share) knowledge within and across organizations, but whether KS behaviors actually bring any advantage to organizations is still to some extent unclear (Haas & Hansen, Reference Haas and Hansen2007; Naim & Lenkar, Reference Naim and Lenka2017; Wang & Noe, Reference Wang and Noe2010). To address this research gap, we adopt the KS intervention mechanisms suggested by Cabrera and Cabrera (Reference Cabrera and Cabrera2002) and focus on how HKFs’ intervention functions attain positive outcomes (if any) for the KS community, which in turn benefit the organization.
Cabrera and Cabrera (Reference Cabrera and Cabrera2002) lay a profound theoretical foundation for KS facilitation functions: (1) to promote group identity and personal responsibility by encouraging communication; (2) to decrease KS cost and increase sharing benefit; and (3) to increase perceived efficacy of contributors. It can be argued that the deployment of an online R&D community platform itself and the corresponding HR policy oriented toward rewarding KS on the intranet are themselves unique KS management practices (interventions) that help decrease KS cost and increase KS benefit. Nevertheless, firms need to design and implement other forms of interventions to facilitate KS, such as awards (Dahlander & Magnusson, Reference Dahlander and Magnusson2005), regular support from community managers and senior management (Nambisan, Reference Nambisan2002; Nambisan & Baron, Reference Nambisan and Baron2007), and protocols and guidelines (Gilbert & Dabbagh, Reference Gilbert and Dabbagh2005). To our knowledge, while the KS facilitation mechanisms suggested by Cabrera and Cabrera (Reference Cabrera and Cabrera2002) have received much research attention, they have rarely been used to test the relationships between interventions and KS outcomes directly.
Despite all the useful means of facilitating KS that are addressed in prior studies, the phenomenon of HKFs is new to researchers and practitioners. Compared to conventional online KS facilitators, HKFs are believed to play their facilitating roles differently for at least two reasons. First, whether and to what extent expert heuristics can be created and effective is questionable, as HKFs’ identities are unknown to online community members. Second, HKFs are appointed by the firm and their job responsibilities of boosting KS in the intrafirm online community are unknown to regular community members (other employees). Their acts do cause other regular online community members to make decisions (for example, uploading documents, sharing experiences, posting messages, and participating in collaborative innovation) that they would not otherwise make. This creates a natural experiment with a condition, under which KS facilitation is deemed as non-existing from a regular online community member's view, removing any potential priming effect on KS behaviors of community members due to the knowledge about being ‘facilitated’ by non-genuine members. This, in turn, makes the HKFs a perfect case to investigate the effect of KS interventions on KS outcomes. Having noticed the importance and special features of HKFs, we are motivated to investigate the objective impacts of HKFs on KS outcomes in relation to Cabrera and Cabrera's typology of intervention mechanisms so that the established theory in KS facilitation can be consolidated.
HYPOTHESES DEVELOPMENT
In this study, we chose three indicators of community-level KS outcomes, which are associated with the generic online intervention mechanisms proposed by Cabrera and Cabrera (Reference Cabrera and Cabrera2002). First, the length of discussion in each thread directly indicates the extent to which information and knowledge are exchanged among online community members (Masters & Oberprieler, Reference Masters and Oberprieler2004; Nisbet, Reference Nisbet2004). This KS outcome indicator corresponds to the first intervention mechanisms proposed by Cabrera and Cabrera (Reference Cabrera and Cabrera2002), namely, encouraging communication. The second online intervention mechanism is to decrease KS cost and increase KS benefit. This mechanism is fundamentally important because a discussion thread of any length bears communication costs to participating members. If it does not reach a solution to the question or problem, participants in the discussion will deem this form of KS useless and members who did not directly participate in the discussion will get the impression that the KS community is ineffective. In relation to this mechanism, a straightforward indicator of KS outcome is to evaluate whether a solution is found, or a problem resolved in each discussion thread. A positive result justifies the KS cost and creates a positive sum of KS benefit within the online community. Whether a solution is found or not reflects community-outcome expectations (Hsu et al., Reference Hsu, Ju, Yen and Chang2007). The third intervention mechanism proposed by Cabrera and Cabrera (Reference Cabrera and Cabrera2002) is to increase the perceived efficacy of contributors. Ideally, HKFs should aim at inducing online community members to make useful, reliable, and creative contributions. The meaningful consequence of doing so at the community level is the observable formation of divergent and convergent discussion (Runco & Acar, Reference Runco and Acar2012) within each discussion thread.[Footnote 1] This is because convergent thinking among contributors indicates that someone's contribution is useful and collectively considered to be reliable; divergent thinking among contributors indicates that they think from different perspectives to solve the problem. Collective convergent and divergent thinking both have creative potential and are good indicators of effective learning as a result of KS (Dijksterhuis & Meurs, Reference Dijksterhuis and Meurs2006; Runco, Reference Runco2007). Table 2 summarizes the generic intervention mechanisms of KS facilitators and the corresponding choices of KS outcomes.
Table 2. Online intervention mechanisms (Cabrera & Cabrera, Reference Cabrera and Cabrera2002) and corresponding community level KS outcomes

As far as HKFs’ observable interventions are concerned, we notice that HKFs need to constantly monitor the development of opinions and information dissemination on the online community to become effective advocates for KS. Actual online intervention behaviors are concrete actions that are performed by actively posting original discussion topics, responding to other community members’ posts, posting knowledge sources, and uploading useful documents.
Online interventions can take various forms, and typically can be observed in one of two ways: by the classification of communication patterns (Adendorff, Reference Adendorff2005; Westerski, Dalamagas, & Iglesias, Reference Westerski, Dalamagas and Iglesias2013) or by generically observing the quantity and quality of observations based on content analysis (Bliss & Lawrence, Reference Bliss and Lawrence2009; Gilbert & Dabbagh, Reference Gilbert and Dabbagh2005; Nisbet, Reference Nisbet2004). HKFs are a special type of KS facilitators and we have little knowledge on how to classify their intervention patterns. In contrast, observing the quantity and quality of HKFs’ interventions can be achieved relatively objectively, and the results may provide a foundation of understanding about their communication patterns for future research. Therefore, we focus on observing the quantity and quality of HKFs’ intervention in our research design. First, quantity matters because a silent HKF will never effectively facilitate KS in the online R&D community. A straightforward measure of online interaction quantity is, for instance, a count of the number of posts made by community members (Masters & Oberprieler, Reference Masters and Oberprieler2004). Second, an intervention with low quality might be ineffective because it might be unnoticed or regarded as useless and unreliable. Prior studies have found that the quality of interaction in asynchronous discussion forums has a positive impact on individuals’ learning outcomes (Nandi, Hamilton, & Harland, Reference Nandi, Hamilton and Harland2012). In a sense, high quality online intervention reflects the extent to which the information provided in an online post meets or exceeds the expectations of those who receive or use it because it is sufficiently informative and reliable and relatively easy for other community members to refer to and further act upon (Hackman, Reference Hackman and Lorsch1987; Nisbet, Reference Nisbet2004). Therefore, both quantity and quality of online interventions are important factors in the managerial objective of making an impact on KS within communities.
Moreover, we argue that when many HKFs coordinate their interventions collectively, it is possible to create a bandwagon heuristic; when a small number of HKFs repeatedly contribute to the online community, they might be seen as experts, creating an expert heuristic. Both heuristics are supposed to facilitate KS. However, the quantity and quality of online interventions need to be considered in combination because their joint effects can be more relevant than their separate effects: a large quantity of low quality interventions may create an impression of useless online discussion; a large quantity of high quality intervention may create information overload and confusion (Edmunds & Morris, Reference Edmunds and Morris2000); a small quantity of low quality interventions will become completely unnoticed; and a small quantity of high quality interventions will have limited reach within an online community. Therefore, we will develop hypotheses for both separate and joint effects of quantity and quality of HKFs interventions.
Hypotheses
First, HKFs cannot afford to stand by when there is an inactive discussion forum. To encourage communication in online KS communities, and in turn promote group identity and personal responsibility among community members, HKFs need to actively post online by commenting, suggesting clues to solving problems, introducing new ideas, encouraging feedback, or directly providing sources of information and documents (Nisbet, Reference Nisbet2004; Westerski et al., Reference Westerski, Dalamagas and Iglesias2013). Like regular online community managers, HKFs need to ensure frequent and timely feedback in the online community to create the feeling of belonging that some employees appreciate (Dahlander & Magnusson, Reference Dahlander and Magnusson2008; Nambisan, Reference Nambisan2002; Nambisan & Baron, Reference Nambisan and Baron2007). The more interventions as such they make, the longer a discussion will last, allowing more employees to participate and exchange information. In some cases, even if other community members are not initially activated by HKFs’ online intervention, the posts made by the HKFs themselves may lengthen the discussion, making it appear more interesting so that the thread may catch other members’ attention later on. In this respect, the quantity of HKFs’ online interventions are clearly related to the length of discussion as a quantitative indicator of community KS outcome. We thus hypothesize,
Hypothesis 1: The quantity of hidden knowledge facilitators’ interventions will be positively associated with the length of discussion.
When the quality of the interventions is high, online community members are able to learn from HKFs’ posts, access the right sources of information and knowledge, become inspired, and be more likely to engage in further discussion. High quality HKF interventions can also reduce the time needed by other employees to search for information and learn so that the learning cost of the entire community in general can be reduced (Haas & Hansen, Reference Haas and Hansen2007). This potentially results in timesaving for community members participating in online discussions and sharing what they have learned. In contrast, low quality HKF interventions offer little value for KS and learning, discouraging further participation in discussions by other community members (Correia & Baran, Reference Correia and Baran2010). Therefore, we argue that high quality HKF interventions will foster more online KS. We thus hypothesize,
Hypothesis 2: The quality of hidden knowledge facilitators’ interventions will be positively associated with the length of discussion.
Furthermore, it is possible that the effects of quantity and quality of HKF interventions may interact. When several HKFs make high quality online interventions on average in a discussion thread, the solution to a problem is found more quickly and there are fewer opportunities for other community members to participate. The consequence is that a KS circle is completed, and the related knowledge documented, formulated, and institutionalized. Thus, it tends to end a discussion thread sooner. Notably, this interplay between quantity and quality of HKFs’ intervention suggests a rapid learning process, indicated by the length of discussion for each thread, but does not suggest inferior quality KS. In fact, HKFs can deliberately shorten an online discussion thread by contributing a number of high quality posts that provide concrete clues to solving the problem. In other words, when HKFs make a number of high quality posts in a thread, the aim of the HKFs is likely to end the discussion by providing a solution as quickly as possible. Our hypothesis is thus:
Hypothesis 3: The positive relationship between the quantity of hidden knowledge facilitators’ intervention and the length of discussion will be less evident when the quality of the interventions is high.
In an online R&D KS forum, engineers and managers typically ask various kinds of R&D-related professional questions, as they contend with different work problems and challenges on a daily basis. The intrafirm online R&D forum becomes a natural choice where the entire community can render some help, as long as someone in the community has the knowledge to provide the right solution or guidance to finding the solution. Therefore, it is very important for the knowledge seeker and the community as a whole to recognize that solutions to posted questions are usually found, a positive indicator of a KS outcome at the community level. In a sense, the online R&D community provides a shortcut for knowledge seekers to find the knowledge within an organization, particularly when high quality online interventions are performed. With respect to any particular question posted online, the more clues and information provided by HKFs, the more likely a solution will be found, because a thread with (seemingly) heated discussions catches people's attention and it is more likely that online community members will collectively solve the problem (Jeppesen & Frederiksen, Reference Jeppesen and Frederiksen2006). Therefore, we hypothesize,
Hypothesis 4: The quantity of hidden knowledge facilitators’ interventions will be positively associated with the likelihood of solutions being found.
In our observation, HFKs can use different approaches when intervening in a discussion thread in order to facilitate employees finding a solution to a posted problem. For instance, they can simply acknowledge the relevance and importance of the problem, help clarify the question, provide some sources of relevant information for people to discuss, verify the usefulness of tips, refer to a specific person who is knowledgeable about the problem, or suggest a solution to the problem directly. These approaches present different levels of quality of interventions, which online community members find useful to varying degrees. When the quality of an HKF's intervention is high, it is sufficiently informative and constructive that the knowledge seeker and other discussion participants can rely on the high-quality posts to either find a feasible path toward a solution based on HKF comments or directly accept a suggested solution. In other cases, HKFs can ‘promote’ challenging development tasks by making high quality interventions to challenge seekers, making them intrinsically interested and satisfied (Dahlander & Magnusson, Reference Dahlander and Magnusson2005). Thus, HKFs’ high quality interventions tend to help employees find solutions. We hypothesize accordingly,
Hypothesis 5: The quality of hidden knowledge facilitators’ interventions will be positively associated with the likelihood of solutions being found.
However, when we take both quantity and quality of HKFs’ interventions into account, we might have a quite different observation. When an intervention is of high quality with little need for rework, it usually means some concrete information or a possible solution to the problem is suggested for the knowledge seekers to consider and verify. In these cases, a small quantity of high quality interventions will suffice, because if a high-quality approach is used too often, it will present too many concrete information clues for the initial knowledge seekers and follower participants to verify and absorb. A large number of high quality HFKs interventions in turn might make people confused about what the actual and effective solutions are. Here, our expectation is that at high levels of quality, the positive effect of quantity is reduced. Therefore, we have the next hypothesis:
Hypothesis 6: The positive relationship between the quantity of hidden knowledge facilitators’ intervention and the likelihood of solutions being found will be less evident when the quality of these interventions is high.
Next, we consider divergent and convergent thinking. These two processes require imaginary and rational cognitive information processing, respectively, based on a certain level of useful clues or information (Dijksterhuis & Meurs, Reference Dijksterhuis and Meurs2006). While divergent thinking is responsible for creating novelty, convergent thinking, which evaluates novel ideas based on knowledge, is an important component of individual creativity (Cropley, Reference Cropley2006). Though conceptually distinguishable, divergent thinking and convergent thinking are two highly integrated parts of creative thinking and they reinforce each other to perform the function of creativity (Runco & Acar, Reference Runco and Acar2012). On the one hand, without convergent thinking, divergent thinking is useless and meaningless; on the other hand, without divergent thinking, convergent thinking has no ground to build on. These two cognitive processes take place recurrently within the mind. As divergent thinking and convergent thinking are not mutually exclusive, it is possible that an external factor may have an effect on them in the same direction, depending on the context. In our specific case, it depends on the intention/purpose of the HKFs. Note that the HKFs are employed by the firm with a special job mandate and they know about each other. Therefore, their actions of interventions in online threads are highly coordinated. For some issues, HKFs want to inspire regular employees and get as many diversified discussions as possible; for other issues, HKFs might simply want to prevent people from spending too much energy in exploring options by quickly focusing on the right choices of solutions. Bearing this in mind, we develop some hypotheses for the effects of HKFs’ online interventions on convergent thinking and divergent thinking separately.
A convergent discussion pattern in online KS communities serves as a safeguard and an enabler for creative solution development in groups. Thus, convergent discussion can be viewed as an aspect of organizational creativity, a KS outcome that reflects the increased efficacy of online contributors as a whole. For convergent thinking, the quantity of interventions matters mainly due to herd behaviors as a result of bandwagon effects created by HKFs (Leibenstein, Reference Leibenstein1950). That is, the more HKFs make interventions, the more likely it is that a bandwagon effect will be created, where people seem to agree with each other. This function is similar to ghost customers (ghost bidders) in online stores, where positive feedback from a large number of ghost customers suggests a quality and price advantage of a product, so that actual online customers are influenced by these opinions and make irrational purchases (van Herpen et al., Reference van Herpen, Pieters and Zeelenberg2009). Following this line of reasoning, we hypothesize,
Hypothesis 7: The quantity of hidden knowledge facilitators’ interventions will be positively associated with convergent discussion.
Moreover, when an HKF's post is of low quality, providing little useful information or knowledge that others can use directly or build upon, it will be hard to trigger any further convergent thinking among other community members, because little knowledge is provided to help community members evaluate ideas and potential solutions. In practice, HFKs can foster convergent discussions by posting high quality interventions with concrete sources of critical information and knowledge, pathways to solutions, and criteria for judging effectiveness and novelty (Cropley, Reference Cropley2006). HKFs’ high quality interventions may also create potential expert heuristics, which also contributes to a bandwagon effect. Therefore, we predict,
Hypothesis 8: The quality of hidden knowledge facilitators’ interventions will be positively associated with convergent discussion.
While a bandwagon effect can be achieved through a large quantity of online interventions or some high-quality interventions, a strategy of combining both could potentially backfire. If many HFKs make high quality posts in small numbers (aiming at creating a bandwagon heuristic) or a small number of HFKs repeatedly make high quality posts (aiming at creating an expert heuristic), the result is that too much seemingly useful information and too many feasible pathways to solve problems are presented to the online community. A large quantity of high quality HKF interventions could create information overload and confusion (Edmunds & Morris, Reference Edmunds and Morris2000; Eppler & Mengis, Reference Eppler and Mengis2004), rather than convergent thinking. Note that this does not necessarily mean that it is impossible for any individual to learn, but for the community at large it will create stress, rather than a foundation for organizational creativity. Therefore, we predict that,
Hypothesis 9: The positive relationship between the quantity of hidden knowledge facilitators’ intervention and convergent discussion will be less evident when the quality of these interventions is high.
Divergent thinking is another critical aspect of creativity. Although divergent thinking alone does not guarantee creativity, it is by nature variety-seeking, and acts as the source of novelty, which is then subject to convergent thinking for evaluation (Runco & Acar, Reference Runco and Acar2012). A divergent discussion in an online community features many ideas, information clues, and alternative pathways to solving problems. Quantity is a double-edged sword – it can be used to create a bandwagon effect to confirm on the right solution so that people's ideas converge, but it can also be used to inspire diversified discussion by ‘stirring the pot’. Thus, we expect a large quantity of HKFs’ interventions will steer divergent discussions and help diversify the direction of discussions. The more HKFs intervene, the more diversified the discussions are. Therefore, we predict that,
Hypothesis 10: The quantity of hidden knowledge facilitators’ interventions will be positively associated with divergent discussion.
As far as the quality of HKFs’ intervention is concerned, low quality interventions lack concrete information clues, making them of little use for idea diversification within online discussions. However, if an HKF's post appears to be a clear solution to a problem with little need for rework, indicating a high level of intervention quality, then it will be difficult to ignite further divergent thinking in other community members, because a KS and learning circle has been completed within the online discussion. Ideally, KFs’ interventions at a medium level of quality will require additional conceptual and practical work from others, either by providing a new viewpoint or referral to a (media) file, document, standard, or knowledgeable person. This inevitably forces people to think and act according to a guided cognitive path through divergent thinking (Basadur, Runco, & Vega, Reference Basadur, Runco and Vega2000). Based on these arguments, we suggest the following hypothesis:
Hypothesis 11: The quality of hidden knowledge facilitators’ interventions will have an inverted-U shaped relationship with divergent discussion.
Finally, if HKFs’ medium quality interventions are the best means of igniting divergent discussion in an online community, then we expect that a large number of HKF interventions will intensify such an effect and make the optimal level of medium level quality more evident. This is because, on an online discussion thread with a small number of HKF posts, a few medium quality interventions might get full attention once or twice, so that other community members will directly follow the suggestion to seek additional information in order to solve the problem. The effect on diversified opinions will not be that evident. However, if the HKFs make a large number of interventions of varying quality, the inspiring and igniting effect of those with medium quality on divergent thinking will be evident, because the large number of interventions makes it possible for the medium quality ones to stand out. Therefore, we hypothesize,
Hypothesis 12: The inverted U-shaped relationship between the quality of hidden knowledge facilitators’ interventions and divergent discussions will be more evident when the quantity of interventions is large.
EMPIRICAL BACKGROUND
The empirical context for this research is within a Chinese multinational heavy machinery manufacturing company (for confidentiality reasons, it will be referred hereinafter as ‘the company’), headquartered in Hunan Province. This company is one of the largest heavy equipment manufacturers in the world, listed on the FT Global 500 and the Forbes Global 2000 indexes. The company is organized into a number of major divisions and subsidiaries, including a concrete pump division, a road construction division, a port machinery division, a mobile crane division, an electric utility company, two heavy machinery subsidiaries, a heavy equipment subsidiary, and a science and technology subsidiary. The company has four international R&D and manufacturing facilities in India, the USA, Germany, and Brazil. The company puts a premium on R&D excellence and pursues global leadership in product innovation in its industry. On average, 5–7 percent of the group's annual revenue is used for R&D investment. By the end of 2014, the company had made 8,282 Chinese patent applications and 405 international patent applications.
The company has approximately 90,000 employees worldwide, of which 4,000 R&D personnel are located in China within about 70 in-house R&D institutes. The intrafirm online R&D platform was established in June 2012 and has been operating ever since. It was designed and introduced with the purpose of enhancing KS and innovation among R&D personnel. In principle, all the company's R&D personnel in China have access to the online platform as regular users, and several online forums have been formed around topic areas such as hydraulic engineering, mechanical engineering, electrical engineering, material engineering, specific crafts, technology benchmarking, and simulation. These forums form virtual knowledge communities that can be roughly divided into six categories: technological exchanges, R&D management, application of tools, product development, knowledge management, and administrative topics.
Since its establishment, the intrafirm online R&D platform has received increasing attention from top management and R&D personnel within the company. This has been demonstrated by the fact that the management of all the research institutes has recognized its relevance to the company's strategy and development of employee competence and has participated in discussions in the online forums; and that the average number of monthly visits and viewing time has been consistently increasing. Still, according to the manager of the intrafirm online R&D platform, it had not yet reached its full effectiveness when the research was conducted: extensive discussions and exchange of knowledge had been relatively concentrated within a dozen R&D institutes and only a few hundred active R&D personnel comprised the key online community members. For this reason, the question of how to systematically use HKFs among these forums (communities) to boost KS caught management's attention. Thanks to the support of the senior manager of the online communities, we were given access to multiple data sources related to the intrafirm R&D online communities and HKFs.
This case company was chosen for a number of specific reasons: first, it has been using HKFs to facilitate online R&D communities for a relatively long period, allowing longitudinal observations; second, we are able to get full access to the entire population of HKFs within the company and observe their online log data for a sufficiently long period; and third, the company is representative of large corporations using intranet platforms to facilitate internal KS in multiple areas of technological R&D.
Prior to collecting online log data of the community communication, we conducted several semi-structured interviews with key staff members, who are highly representative of different perspectives in the company. The purpose of conducting these pre-study interviews was to have a sound understanding of who the HKFs are and how they function. The interviewees comprised the chief manager of the intrafirm online communities, two official HKFs, and a regular community member. All interviewees were employees of the company and registered users of the online R&D platform. We did not associate the questions with any established theoretical perspectives on knowledge facilitators, for instance, the mechanisms suggested by Cabrera and Cabrera (Reference Cabrera and Cabrera2002). Instead, we used several open-ended questions to allow the interviewees to freely express their views. Each interview took at least two hours. An overview of the interviewees and their representativeness is provided in Table 3 below. The insights gained from these interviews also helped the authors to develop the study's hypotheses.
Table 3. An overview of interviewees and their background

Among the interviewees, the online R&D platform manager was responsible for knowledge management of the entire company and has been an advocate for the use of the online community to foster KS among R&D staff. He was also one of the founders of the online R&D platform and is knowledgeable about various aspects of online community design. He recalled that using HKFs was one of the original plans implemented to ensure a sufficient level of online interactions and KS in discussion forums. The two HKFs interviewed both felt that they had been making positive contributions to the online community by creating a more friendly and active atmosphere for regular members participating and sharing knowledge. Thus, it was a common understanding among HKFs that they were doing the company and online community members a favor without behaving illegally or unethically. We also interviewed a regular member of the online R&D platform. He had been an active member of the online community since the platform's establishment. He fully understood the logic of HKFs and believed strongly that if HKFs were used appropriately, they might well serve a good purpose for the company and employees alike.
When asked how HKFs functioned or should function on the online R&D platform, the interviewees provided interesting insights about the roles of HKFs. The main insights from the pre-study interviews are summarized below.
(1) HKFs’ identities: There is no hierarchy among HKFs on the online communities, because they use an alias for their usernames and their real identities are not always known. An HKF is usually aware of the existence of other HKFs. An HKF may be active in multiple discussion forums under different topic categories and may also have multiple usernames, appearing with multiple identities without being identified as the same person.
(2) HKFs’ motivations: Because the key performance indicators of KS for each of the company's R&D institutes are summarized, evaluated, and reported by the manager of the online R&D platform to top management, the HKFs at each institute have some incentive to keep intervening in online R&D communities. There is also a monthly monetary award, granted at the corporate level, for the best contributing community members. Therefore, expectations of personal advancement in terms of status, competence, promotion, or an increase in salary may directly motivate them to actively play their role as an HKF. HKFs constantly monitor and participate in the discussions on the online forums that match their interests. However, some HKFs also have intrinsic motivations: they find it psychologically rewarding to see other online community members learning from them and gain some benefit themselves from the experience.
(3) HKFs’ interventions: HKFs recognize that finding a balance between overdoing it – resulting in overly heated online interactions and too powerful individual status – and underdoing it – resulting in ineffective user interactions in online communities – is an art unto itself. In other words, both the quantity and the quality of their online interventions matter, and a good balance between quantity and quality takes judgment and sensitivity. An HKF needs to be fairly knowledgeable in the topics where he/she intervenes. When an HKF continues contributing, he/she is more likely to be perceived as an expert who gradually becomes more and more influential in the online community.
DATA AND METHODS
Data
In designing the research study, we sought to both understand the effect of HKFs on KS outcomes within organizations and overcome the shortcomings of self-reporting common to the questionnaire method, the approach used in the majority of prior studies on KS effectiveness (e.g., Chen & Hung, Reference Chen and Hung2010; Haas & Hansen, Reference Haas and Hansen2007; Lee, Reference Lee2001; Ma & Yuen, Reference Ma and Yuen2011). Thus, we chose to deploy a research design that fully explored the log data of an intrafirm online R&D community for KS, based on extensive content analysis of HKFs’ objective online intervention behaviors, in order to reveal their actual effectiveness. We treated each online discussion thread as the unit of observation and conducted statistical analysis using different modeling techniques to examine the impact of quantity and quality of HKFs’ online interventions on the KS outcomes in online R&D communities. We observed the textual output of the company's online R&D communities, giving a special focus on the quantity and quality of the HKFs’ online interventions, during the two-month period of March–April, 2014[Footnote 2], during which more than 50 percent of the R&D personnel on average visited the online platform on a monthly basis. Discussion topics in different forums received different levels of attention, ranging from fewer than five visits to more than 300 visits and taking from less than one minute to more than three hours of viewing time.
Senior management of the company provided us with the names of all 23 HKFs (with their names, employee IDs, online user names, and email addresses) who were operating on the intrafirm R&D communities during the study period. The online R&D community manager granted us direct access to the online log data. Of the various sections[Footnote 3] of the online platforms, we focused on online Q&A forums for two reasons: (1) they are the most active areas of the online R&D platform; and (2) the discussions mostly form ‘vertical questions’, which assume that a correct answer exists and can be found, and ‘horizontal questions’, which invite negotiation around a plausible answer (Fahy, 2003). These types of questions and communication patterns match our research purpose.
The web log data of all the online threads in the Q&A forums were used to calculate the quantity of HKF interventions and the length of each thread. To assess the content of online communications, we followed a ‘netnographic’ approach, as suggested by prior studies (Jeppesen & Fredriksen, Reference Jeppesen and Frederiksen2006). Netnography is an interpretive methodology, focusing on the textual output of Internet-related fieldwork (Kozinets, Reference Kozinets1998). The ‘netnographic’ approach enables us to fully understand the context of a particular act of an HKF in a given situation without any potential misinterpretation. This serves as a solid foundation on which content analysis can subsequently be conducted to measure the variables that need qualitative judgment and evaluation, such as quality of intervention, solution found, and convergent and divergent discussion.
Variables and Measures
First, quantitative approaches to measuring the amount of online interaction are well known, and it is relatively straightforward to measure the number of posts in each thread (Masters & Oberprieler, Reference Masters and Oberprieler2004; Nisbet Reference Nisbet2004). Therefore, one independent variable, quantity of HKF intervention, is measured by counting the number of HKFs’ posts (this is possible because we know the user IDs of all HKFs). However, we are also interested in a related but different measure of HKF intervention, measured by the number of unique HKFs involved in each thread. These two measures for quantity of HKFs’ interventions are highly correlated (and thus not to be included in the same regression models as independent variables) but represent different aspects of the quantity variable. In the analysis, we run regressions for these two measures of quantity of HKFs’ intervention separately.
Second, regarding the electronic format of KS with regard to content and process, there are several ways of measuring quality of online intervention discussed in the literature, which primarily recommends that researchers ask other community members or managers to rate the online contributions of a particular type of user (Haas & Hansen, Reference Haas and Hansen2007). This approach requires raters to have a good understanding of the context of the contributions to be rated in order to ensure that the rating is impartial and reliable. However, we could not use such an approach because the practice of using HKFs amounted to a corporate secret – only a handful of managers knew about it. If we had followed the rating approach by involving other managers and employees, it would have inevitably revealed the identities of the HKFs. Therefore, we had to use a different approach.
In this study, we follow Haas and Hansen (Reference Haas and Hansen2007) and focus on the concept of level of rework. Level of rework measures the degree to which a user's online contribution requires additional work by other online users before it becomes sufficiently informative and useful. Level of rework in this sense is conceptually representative of the concept of intervention quality, especially as our observation focuses on the online Q&A forums, where helpful information and useful solutions are expected among users. An online intervention of high quality should have a low level of rework. Thus, quality of an HKF's online intervention is a reversed measure of level of rework. We use content analysis (Wickersham & Dooley, Reference Wickersham and Dooley2006) to measure quality of intervention, using the following coding convention: 0 = no useful information; 1 = suggesting a perspective, viewpoint, or potential way of seeking solutions; 2 = referring to a concrete document, link, media file, or authoritative viewpoint with evidence, but requires further learning by those who raised the question; 3 = providing direct answers to the question with certainty. If there is more than one intervention by an HKF, we take the average value of the quality measure.
Three dependent variables were identified. The first, length of discussion, is measured by counting the number of total posts in each thread and subtracting the number of posts made by HKFs.
Next, whether a solution has been found in each discussion thread is measured by a binary variable, which draws on straightforward information from each discussion thread. In some typical cases, solutions feature a clear statement by the question raiser, saying, for instance, ‘Aha, problem solved, thank you guys so much!’, ‘This works! Awesome!’, or ‘I have just tested (it), XXX was so helpful!’ Many other cases became an open discussion without any concluding remarks or testimony of effectiveness from anyone in the discussion thread.
Third, there has been a long tradition of measuring divergent and convergent thinking at an individual level, using the so-called ‘alternative uses task’ (AUT) test and ‘remote associates task’ (RAT) test, respectively (Hommel, Colzato, Fischer, & Christoffels, Reference Hommel, Colzato, Fischer and Christoffels2011; Jauk, Benedek, & Neubauer, Reference Jauk, Benedek and Neubauer2012). These tests have been criticized for their potential bias, derived from subjective evaluation of quality scoring (Runco & Acar, Reference Runco and Acar2012). These traditional tests do not suit our research purpose, because the focus of our unit of observation is convergent and divergent patterns of discussion occurring collectively in online discussion threads. Therefore, relying on content analysis of original text and context, we used the following coding convention to judge the occurrence of divergent thinking: (1) different opinions, which are complementary to each other, appear; (2) different opinions, which disagree with one another, appear; (3) different opinions, which seem completely unrelated, appear. When any of these three scenarios takes place, we code the variable as ‘1’, otherwise ‘0’. Based on the same approach, we use the following coding convention to judge the occurrence of convergent thinking: (1) all following posts converge eventually, agreeing on a plausible or convincing solution to the original question; (2) the question raiser and the following posts that attempt to answer the question converge eventually, but the question does not necessarily find an answer. It could be the case that the question raiser confirmed that the suggestion or further inquiries from the posts are relevant and important to the original question. This could also happen when a question was not clearly defined or vague, or lacked visual information, so that a few more rounds of communication were needed to clarify the question and related issues. This process itself is a good learning outcome; (3) the question raiser and the following posts converge by recognizing the relevance and importance of the same question, and other members in the community share an interest in getting the answer to the same question as well. When any of these scenarios takes place, we code the variable as ‘1’, otherwise ‘0’. Among the 379 observations, 14.8% of the cases are convergent ONLY, 30.9% are divergent ONLY, and only 1.6% are BOTH convergent and divergent.
When using content analysis, a coding team of two of the co-authors and two research assistants with both engineering and management backgrounds independently coded the relevant variables. Then we compared our coding results to identify and discuss any inconsistencies and agree on final scores. A list of coding schemes of the variables based on content analysis of online communications are provided in Table 4, with a number of real examples from online discussion threads.
Table 4. Discussion thread examples, variables, and coding schemes

Finally, we also include two dummies as control variables: (1) whether a discussion thread was initiated by an HKF; and (2) whether the discussion thread took place in March versus April, 2014, to control for any unobservable variance pertaining to time.
Statistical Models
In this study, the unit of observation for data analysis is each discussion thread, instead of each individual or the entire online community. As the natures of the dependent variables for our hypotheses are quite different, we use different statistical modeling techniques for each. The dependent variable for H1, H2, and H3 is the length of discussion, a count variable. Thus, we use a Poisson regression model to appropriately count so that discrete events can be modeled (Cameron & Trivedi, Reference Cameron and Trivedi2005). To test H4–H12, we use binary logistic regressions because the dependent variables for these hypotheses are binary variables.
RESULTS
During March and April of 2014 there were 379 online discussion threads and 1,717 posts in total in the R&D Q&A forums; thus the average length of a thread is 4.53 posts. On average, HKFs made 1.32 posts per thread. Interestingly, most of the threads were not initiated by an HKF (mean value = 0.17). In Table 5, the mean and standard deviation of all variables are presented with their correlations. Among all the independent variables and control variables, we found that several correlations are moderately high, for instance, between HKFs initiation and the number of unique HKFs (0.508), between HFKs initiation and the number of HKFs’ posts (0.533), and between quality of intervention and the number of HKFs’ posts (0.554). We further checked the variance inflation factors (VIF) for all the independent and control variables against the corresponding dependent variables: we found that no variable has a VIF value higher than 2, much lower than the critical value, 10. Thus, multicollinearity is not a concern. Furthermore, as the two measures of quantity of HKFs’ interventions (the number of unique HKFs in each thread and the number of HKFs’ posts) are not included in the same estimation models in any case, the high correlation between these two measures (0.737) is not of concern.
Table 5. Descriptive statistics and correlations (N = 379)

Note: Significance levels: * p < 0.05; ** p < 0.01
Table 6 presents the results of the Poisson regression to test H1, H2, and H3, which predict the impact of quantity and quality of HKFs’ online intervention on the length of discussion as the dependent variable. We first introduce a base model (model 0), using only two main control variables: HKF's initiation and month. Then, we introduce the two main effect variables, quantity and quality of online intervention (models 1 and 3). Next, we introduce the interaction terms of quantity and quality of HKFs intervention into the model (models 2 and 4). The Wald Chi2 statistics for all the models are significant compared to an intercept-only model. In models 1 and 2, the quantity of intervention is measured by the number of unique HKFs, while in models 3 and 4 it is measured by the total number of HKFs’ posts.
Table 6. Results of Poisson Regression Models for H1, H2, and H3

Notes: Significance levels: * p < 0.10; ** p < 0.05; *** p < 0.01; Standard errors in brackets; N = 379
We find that the quantity of intervention has a positive and significant effect on the dependent variable in both models 1 and 2 (β = 0.354, p < 0.01; β = 0.514, p < 0.01, respectively). When it is measured differently, the quantity of intervention has a positive and significant effect on the dependent variable in both models 3 and 4 as well (β = 0.138, p < 0.01; β = 0.271, p < 0.01, respectively). Therefore, H1 is supported. However, the effect of HKFs’ intervention quality has a marginal effect on the dependent variable in models 1 and 2 (β = −0.063, p < 0.10; β= 0.094, p < 0.10, respectively). Only when the interaction term of quantity and quality of HKFs’ intervention is introduced in model 4, does the quality of intervention show a positive and significant effect (β = 0.123, p < 0.01). Therefore, H2 is only partially supported. H3 predicts a negative moderating effect of quality on the positive effect of quantity. We find that the coefficients of quantity times quality are both negative and significant in models 2 and 4 (β = −0.170, p < 0.01; β= −0.098, p < 0.01, respectively). Thus, H3 seems supported. The interaction effect is plotted and shown in Figure 1A and 1B. However, by calculating the standardized mean difference (SMD) effect size for the moderating effect, we find that SMD is -0.25 (with an estimation of [-0.364, -0.129] at the 95% confidence interval) when quantity of intervention is measured by number of unique HKFs. The SMD effect size for the moderating effect is -0.156 (with an estimation of [-0.209, -0.10] at the 95% confidence interval) when quantity of intervention is measured by number of HKFs’ post. These tests about effect size indicate no effect.

Figure 1A-1B. Interaction effect of quantity, moderated by quality, of HKFs intervention on length of discussion (H3)*
The actual effect of quantity of intervention, given the levels of quality of intervention, also can be intuitively interpreted by visualizing the actual data. Following the recent call for paying more attention to making sense of actual data in management research beyond showing coefficient estimates, standard errors, and significance levels (Greve, Reference Greve2018; Levine, Reference Levine2018), we make scatterplots to further inspect the hypothesized moderating effect. Figure 2A shows all cases with value of length of discussion vis-à-vis quantity of intervention, which is measured by the number of unique HKFs. Figure 2B shows cases with low or high levels of quality of intervention separately. Similarly, Figure 3A shows the relationship between length of discussion and quantity of intervention, which is measured by the number of HKFs’ posts. Figure 3B separates cases with low or high levels of quality of intervention. We find that regardless in which way the quantity of intervention is measured, it is not easy to visually judge that a seemingly positive relationship between invention quantity and length of discussion is less evident for cases with high levels of quality of intervention than those with low levels of quality of intervention. Thus, it makes sense to conclude that H3 is not supported.

Figure 2A-2B. Scatterplot for effect of quantity, moderated by quality, of HKFs intervention on length of discussion (H3) (Quantity of intervention measured by number of unique HKFs)

Figure 3A-3B. Scatterplot for effect of quantity, moderated by quality, of HKFs intervention on length of discussion (H3) (Quantity of intervention measured by number of HKFs’ posts)
To test H4, H5, and H6, which assess the impact of HKFs’ intervention quantity and quality on the solution found, we ran a binary logistic regression because the dependent variables with a binary value; the results are shown in Table 7. Both models meet the Hosmer-Lemeshow test (non-significant chi-square), indicating that the data fit the models well. In the first model, the quantity of intervention is measured by the number of unique HKFs, while in the second model it is measured by the total number of HKFs’ posts. H4 predicts that the quantity of HKFs’ online interventions is positively associated with the likelihood that solutions will be found. The results show that the quantity of intervention has a positive and significant effect in both models (β = 0.619, p < 0.01, odds ratio = 1.858; β = 0.470, p < 0.01, odds ratio = 1.600, respectively), supporting H4. Next, H5 predicts that the quality of HKFs’ intervention is positively associated with the likelihood that solutions will be found. We find that the quality of intervention has a positive and significant effect in both models (β = 0.616, p < 0.01, odds ratio = 1.852; β = 1.007, p < 0.01, odds ratio = 2.737, respectively), supporting H5. When the interaction term of quantity and quality is introduced into the models, we find that it shows a negative and significant effect only in the second model, where the quantity is measured by the number of HKFs’ posts (β = −0.286, p < 0.05, odds ratio = 0.752), but not in the first model. Thus, H6 is only supported only when intervention quantity is specifically measured by the number of HKF's posts with a relatively small effect size of the moderating effect of 0.752 (odds ratio). The interaction effect is plotted and shown in Figure 4. We also make scatterplots to further inspect the hypothesized moderating effect. Figure 5A shows all cases regarding the relationship between predicted probability of solution found and quantity of intervention, which is measured by the number of HKFs’ posts. Figure 5B shows cases with low and high levels of quality of intervention separately. It is visually not difficult to figure out that, the cases with a low level of quality of intervention align with a positive effect line in a better shape than those with a high level of quality of intervention.

Figure 4. Interaction effect of quantity, moderated by quality, of HKFs intervention on solution found (H6)* (quantity of intervention measured by number of HKFs’ posts)

Figure 5A-5B. Scatterplot for effect of quantity, moderated by quality, of HKFs intervention on solution found (H6) (Quantity of intervention measured by number of HKFs’ posts)
Table 7. Results of Binary Logistic Regression Models for H4, H5, and H6

Notes: Significance levels: * p < 0.10; ** p < 0.05; *** p < 0.01; Standard errors in brackets; N = 379
To test H7, H8, and H9, which assess the impact of HKFs’ intervention quantity and quality on convergent discussion, binary logistic regression is used again; the results are shown in Table 8. In the first model, the quantity of intervention is measured by the number of unique HKFs, while in the second model it is measured by the total number of HKFs’ posts. The first model does not meet the Hosmer-Lemeshow test, indicating that the model is not a good fit. Therefore, we only count the results shown in the second model, where quantity of intervention is measured by the number of HKFs’ posts. H7 predicts that the quantity of HKFs’ online interventions is positively associated with convergent thinking. The results show that the quantity of intervention has a positive and significant effect (β=0.336, p < 0.05, odds ratio = 1.400), supporting H7. Next, H8 predicts that the quality of HKFs’ interventions is positively associated with convergent thinking. We find that the coefficient of quality of intervention is positive but not significant. Thus, H8 is not supported. This is probably because convergent thinking in a group level actually takes place offline after high quality posts are viewed by employees, and we have no means to observe offline convergent thinking. When the interaction term of quantity and quality is introduced into the model, we find that it shows a negative and significant effect with a marginal level of significance (β = −0.170, p < 0.10, odds ratio = 0.8442). Thus, H9 is only marginally supported when intervention quantity is measured by the number of HKFs’ posts with a small effect size of the moderating effect of 0.8842 (odds ratio). The interaction effect is plotted and shown in Figure 6. We also make scatterplots to visually inspect the hypothesized moderating effect. Figure 7A shows all cases regarding the relationship between predicted probability of convergent discussion and quantity of intervention, which is measured by the number of HKFs’ posts. Figure 7B shows cases with low and high levels of quality of intervention separately. It is visually not difficult to figure out that the cases with a low level of quality of intervention align with a positive effect line in a better shape than those with a high level of quality of intervention.

Figure 6. Interaction effect of quantity, moderated by quality, of HKFs intervention on convergent discussion (H9)* (quantity of intervention measured by number of HKFs’ posts)

Figure 7A-7B. Scatterplot for effect of quantity, moderated by quality, of HKFs intervention on Convergent discussion (H9) (Quantity of intervention measured by number of HKFs’ posts)
Table 8. Results of Binary Logistic Regression Models for H7, H8, and H9

Notes: Significance levels: * p < 0.10; ** p < 0.05; *** p < 0.01; Standard errors in brackets; N = 379
To test H10, H11, and H12 regarding the impact of HKFs’ intervention quantity and quality on divergent discussion, we use binary logistic regression as well; the results are shown in Table 9. In the first two models, the quantity of intervention is measured by the number of unique HKFs, while in the last two models it is measured by the total number of HKFs’ posts. All models meet the Hosmer-Lemeshow test, indicating that the data fit the models well. In the first model, we include quantity and quality of intervention and the squared term of quality of intervention. In the second model, we add the interaction term between the quantity of intervention and the squared term of quality of intervention. We use the same steps for the last two models. H10 predicts that the quantity of HKFs’ online interventions is positively associated with divergent thinking. The results show that the quantity of intervention has a positive and significant effect in all models for both measures of quantity of intervention (β=0.532, p < 0.01, odds ratio = 1.702; β=0.642, p < 0.01, odds ratio = 1.899; β=0.306, p < 0.01, odds ratio = 1.358; β=0.335, p < 0.01, odds ratio = 1.427, respectively). Thus, H10 is supported. Next, H11 predicts that the quality of HKFs’ interventions has an inverted U-shaped relationship with divergent thinking. We find that the coefficient of the square term of quality of intervention is negative and marginally significant only in the third model, where quantity of intervention is measured by the number of HKFs’ posts (β= -0.326, p < 0.10). Thus, H11 only finds weak support. Finally, H12 predicts that the inverted U-shaped relationship between the quality of HKFs’ interventions and divergent discussion is more evident when the quantity of interventions is large. However, the results in Table 9 for both types of measures for quantity of intervention show no significant effect. Thus, H12 is not supported. This weak result for H11 and non-support for H12 can be interpreted that quality of intervention alone does not matter much for stimulating divergent discussion, but another reason could be that the measure for quality of intervention should be more finely-grinded to observe any effects on online divergent thinking.
Table 9. Results of Binary Logistic Regression Models for H10, H11, and H12

Notes: Significance levels: * p < 0.10; ** p < 0.05; *** p < 0.01; Standard errors in brackets; N = 379
Finally, although we did not formally hypothesize the effect of HKFs’ initiation (whether a discussion is initiated by an HKF), we find its effects on different KS outcomes as dependent variables interesting. It is consistently negatively related to the length of discussion (see results in Table 6) and to divergent thinking (see results in Table 9). A list of dependent and independent variables for the corresponding hypotheses with a summary of results are shown in Table 10.
Table 10. A summary of hypotheses and results
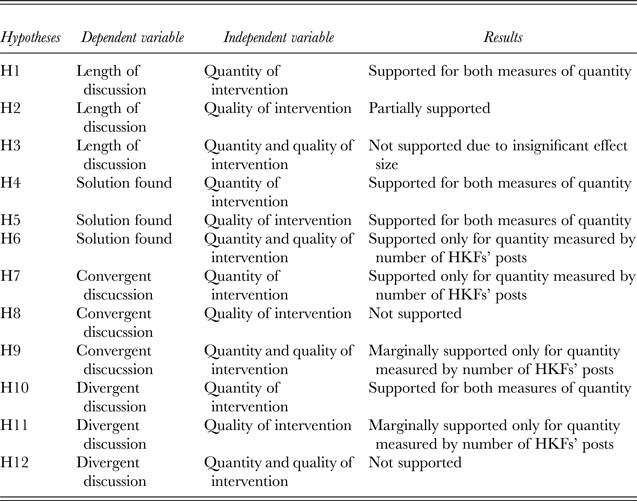
DISCUSSION
Theoretical Contribution and Practical Implications
HKFs’ online contributions, expressed through original posts or comments on others’ posts, will be seen by all other community members. As a consequence, knowledge exchange in online communities (Li-Ying & Salomo, Reference Li-Ying and Salomo2013) is more likely to happen with the function of HKFs. The behavior of HKFs provides an additional clue for other community members, who can create a mental shortcut used as a judgment rule for making quick evaluations. As long as a number of other online community members (including other HKFs) react to an HKF's posts, a bandwagon effect can potentially emerge (Sundar, Reference Sundar, Metzger and Flanagin2007; Sundar et al., Reference Sundar, Metzger and Flanagin2008). As firms deploy HKFs as hidden moderators in their online KS communities, it is relevant and interesting to understand whether and how it works to foster greater KS. Informed and inspired by the insights gained from a number of in-depth interviews with relevant stakeholders, we developed a number of hypotheses with regard to the relationships between the quantity and quality of HKFs’ online contributions and community-level KS outcomes, and tested these hypotheses based on online log data using content analysis. The results reveal interesting and surprising findings.
First, companies need to understand that the role of HKFs and their effect on KS rely on both the quantity and quality of their contributions in the communities. To stimulate online interaction by extending the length of discussion, HKFs can either add more responses to a thread regardless of their quality, or to contribute to a thread with posts of high quality. However, it is not conclusive whether the approach of combining a large number of interventions with high quality posts will do good or harm to the intended outcome. Therefore, we suggest that the online community manager should guide HKFs to try out a mix of a relatively large number of posts with low quality and a relatively small number of posts with high quality.
Second, when a question is raised in an online discussion thread, it is important to pay attention to whether a solution is found in the discussion. This is an indicator of effective KS among employees, because a discussion with a found solution will result in formalized and institutionalized knowledge that will be documented by the community manager on the knowledge bank portal. The results of our research in this regard show strong positive effects of quantity and quality of HKFs’ intervention on the likelihood of solutions found. Moreover, a negative interactive effect of quantity and quality has a relatively small but sensible effect size. Therefore, we suggest that to ensure that a solution will be found in a discussion, HKFs may consider two possible strategies: (1) to get many HKFs to react to the question or to make many posts reacting to the question; and (2) to make high quality interventions. Using a large number of high quality interventions may not create higher likelihood of solutions found.
Third, convergent discussion can be fostered by a large number of posts made by HKFs, or by involving many HKFs in a discussion thread. However, quality of HKFs’ intervention does not necessarily help to form a convergent discussion online. The results of our research also show a negative interactive effect of quantity and quality with a marginal significance level and a small but sensible effect size. Therefore, we are confident to suggest that increasing the use of large number of posts with high quality may lower the chance of having convergent discussion in the online community.
Finally, if many HKFs make posts or if a large number of posts are made by a small number of HKFs, divergent discussion will probably emerge. In addition, a medium level of HKF intervention quality could be optimal to foster divergent discussion to some extent. In sum, the quantity and quality of HKFs’ interventions present complex patterns of impact on KS outcome in online R&D communities. Therefore, managers need to pay close attention to a balanced approach to using HKFs in terms of quantity and quality to foster KS, depending on the organizational objectives around KS.
Within the specific context of our research, these findings make clear contributions to the literature on KS with regard to how interventions made by knowledge facilitators can actually lead to desired KS outcomes for an organization. We suggest that future research make good use of KS practices in the digitalization process of organizations using content analysis for future empirical studies. In this way, our knowledge on the linkage among KS motivations, KS behavior, and KS outcomes can be enriched. These findings also make specific contributions to the understanding of the roles of knowledge facilitators in digitized organizations so that the typology proposed by Cabrera and Cabrera (Reference Cabrera and Cabrera2002) can be further developed.
Although using HKFs may be seen as an acceptable practice in China, other cultures may view it differently. This study is limited to the context of the business culture in China, where perceptions of what type of actions of an employer are acceptable by employees might be different from those in the Western cultures. As HKFs are hidden from regular employees, there is a question about whether regular employees will accept the practice of HKFs as a deliberate management decision. Prior research has suggested that perceived organizational justice and citizenship behavior differ between the US and China (Schilpzand, Martins, Kirkman, Lowe, & Chen, Reference Schilpzand, Martins, Kirkman, Lowe and Chen2013). Also, group harmony plays a significant role to mediate the effect of justice climate on group effectiveness (Ünal, Chen, & Xin, Reference Ünal, Chen and Xin2017). Thus, the reason why HKFs can effectively facilitate online KS within an organization in China is deeply rooted in the Chinese culture. However, this does not mean that HKFs cannot be deployed in firms with Western culture at all if the practices of HKFs are well managed, as long as their organizational culture values group harmony and the good will of management more important than individual interests and justice. It can be interesting to see how firms in other national cultures perceive the usefulness of HKFs in online communities (Jackson, Reference Jackson2011). Here experimental design methods can be highly promising, for instance, to have two controlled business units of the same company (one in China and the other in the US) and test the potentially different effects of HKFs’ KS intervention.
Limitations and Future Research Directions
This study has a number of limitations. First, it is based on a single case study using content analysis. We are aware that there are clearly limitations pertaining to a study based on the observation of a single organization (Yin, Reference Yin1993). However, as the phenomenon of using HKFs within intrafirm online communities is likely a common practice among firms and their roles and effectiveness are theoretically unnoticed and practically unclear, an in-depth study of such a kind is extremely helpful to explore the nature of HKFs, their interventions, and organizational implications. The combined use of pre-study interviews and online log data provide deep insight into a yet unexplored phenomenon. Should we have access to several similar case firms and replicate the research approach, the validity and generalizability of the findings will be significantly improved. Future study will benefit from a multi-case study design that takes cross-organizational differences into account. Second, in this study we only looked into the use of HKFs when they have already participated in the online R&D communities and resumed their ‘duties’. We were not able to investigate the behaviors of HKFs before they accepted and after they fulfilled their responsibilities. As participation and contribution to an online community is in a broad sense a special exchange relationship, future research in this direction may differentiate the roles of HKFs at different stages of involvement by observing HKFs’ behaviors pre-exchange, during exchange, and after exchange (Li-Ying & Salomo, Reference Li-Ying and Salomo2013).
There is a limitation regarding the measures of divergent and convergent thinking. The binary measures that we use have an advantage of simplicity, but it might also miss out richer variation among situations in the real world. Alternative measures for divergent and convergent thinking are encouraged to be used in future research. Another limitation concerns the temporal effect of HKFs’ online intervention on the occurrence of divergent and convergent thinking in online discussions. As the job mandate of HKFs in R&D communities is to promote knowledge sharing and innovation, it is reasonable to expect that HKFs can use quantity as a means to inspire divergent discussion first, and then use quality as a means to help employees to converge the ideas to something constructive, tangible, and useful. Therefore, it could be highly interesting to develop hypotheses accordingly regarding the sequential effect of quantity and quality of HKFs’ online intervention on divergent and convergent thinking, respectively. However, when measurements are only taken at the level of a discussion thread, involving multiple employees, it is practically very hard to examine such a potential sequential effect because, in fact, not all divergent and convergent thinking as cognitive processes in online community members’ minds are manifested in the online discussion, and those offline actions of learning are not observable in this research. For instance, by observing the online discussion, we might only identify the occurance of divergent thinking among those who participated in the online discussion without identifying convergent discussions. However, this does not necessarily mean that some employees have not experienced some sort of intermediate process of convergent thinking and took some offline learning actions, which we could not observe. Conversely, if we could only observe convergent thinking manifested by online communication, this does not mean divergent discussions have not taken place offline. Therefore, it is very hard (and perhaps methodologically risky) to argue for the sequential effect and test it, if means of observing KS outcome is limited to only one form of communication. Future research may fill in this gap by using multiple mechanisms of observation.
Other Managerial Issues and Future Research
First, firms using HKFs to intentionally influence employees in intrafirm online KS communities have a clear purpose: to transform a firm's organizational learning culture from a hierarchical to a distributed model. This is where theories of organizational culture become relevant to HKFs as well. Future research may draw on the literature on organizational culture – for instance, the three perspectives of organizational culture (Martin, Reference Martin2002) and the group and grid culture theory (Jackson, Reference Jackson2011; Thompson, Ellis, & Wildavosky, Reference Thompson, Ellis and Wildavosky1990) – to understand the rationale of using HKFs and the potential conflicts they might create. Future research may observe how a firm uses various means to achieve a transition of organizational culture without creating ‘differentiation’ and ‘fragmentation’ (Martin, Reference Martin2002).
Second, the broad stream of the literature on management transparency has developed a dynamic perspective, which views transparency as an IT-driven communication process, in which firms and stakeholders interact to share information and cooperate (Santana & Wood, Reference Santana and Wood2009; Turilli & Floridi, Reference Turilli and Floridi2009). It is with this dynamic perspective that many large firms introduce intrafirm online KS platforms, because they want knowledge distributed among employees within the organization to be shared transparently without being constrained by the hierarchical organizational structure, creating an effective and efficient transactive memory system (Brandon & Hollingshead, Reference Brandon and Hollingshead2004). Role transparency ensures that the right persons with the right function and competence can enter the right kind of exchange relationship in a community (Nambisan, Reference Nambisan2002), and process transparency makes information exchange relationships clear and explicit (Nambisan, Reference Nambisan2002; West & O'Mahony, Reference West and O'Mahony2008). The problem of HKFs’ actions in intrafirm online KS communities is that role transparency and process transparency might be compromised. Future research is encouraged to investigate the impact of predefined role and process transparency on the perceived roles and actual actions of HKFs in an online KS community, which may in turn reshape the transparency of the online community as a whole.
Last but not least, the fact that HKFs’ identities and roles are non-transparent to regular community members may raise the issue of management ethics (Sandin, Reference Sandin2009). Some empirical studies have suggested that an ethical culture is needed for successful adoption of information systems (Ruppel & Harrington, Reference Ruppel and Harrington2001) and ethical leadership is important as well because it influences followers’ decision-making (Steinbauer, Renn, Taylor, & Njoroge, Reference Steinbauer, Renn, Taylor and Njoroge2014). At first glance, the deployment of HKFs is far from the virtue of truthfulness, a higher level of management transparency (das Neves & Vaccaro, Reference das Neves and Vaccaro2013). However, the literature has also recognized that there are not only ethics of justice but also ethics of care (Sandin, Reference Sandin2009; Simola, Reference Simola2003, Reference Simola2005): HKFs might not be regarded as ethical in justice, but they can be used to strengthen ethics of care for the sake of fostering better organizational culture and enhancing KS by judiciously responding to other people's posts about new ideas and threads, maintaining the active atmosphere within a community, and continuously motivating participants’ contribution. Therefore, the behaviors of HKFs serve the organization, an important aspect in management ethics (Jackson, Reference Jackson2000; Reference Jackson2001). On the other hand, once the systematic use of HKFs is compromised (i.e., HKFs are detected by regular online community members), it may completely backfire, and senior management may be criticized for taking advantage of employees’ trust and manipulating their knowledge contribution. Future research should observe management and HKFs in these circumstances in a well-defined context.
CONCLUSION
Although we believe that HKFs are often used in the KS online platforms of large companies, the unique phenomenon of HKFs is still far from being well understood. Primarily based on the literature of KS and KS facilitation, we explore how HKFs make their online interventions in terms of quantity and quality to achieve desired KS outcomes in online R&D communities. Both quantity and quality of HKFs’ online contribution have impacts on the KS outcomes of online communities in a complex and interactive fashion, depending on the objectives of KS outcomes. Senior managers interested in using HKFs should consider the findings of this study carefully, to ensure effective enhancement of KS and innovation within their organizations.