1 Introduction
Preferences for clinical relative to statistical judgments have been an important focus of research in judgment and decision making, from Paul Meehl’s seminal contribution to the present day (Reference MeehlMeehl, 1954). This research shows that people generally prefer to rely on human rather than statistical judgment (e.g., actuarial rules; Reference Dawes, Faust and MeehlDawes et al., 1989). Algorithm aversion is a timely and consequential example of this preference, whether characterized as a preference for human judgment relative to similar algorithmic judgment, or as a more general distrust of algorithmic judgment (Reference Dietvorst, Simmons and MasseyDietvorst et al., 2015; cf., Reference Castelo, Bos and LehmannCastelo et al., 2019; Reference Logg, Minson and MooreLogg et al., 2019). In this vein, we recently published an article examining how algorithm aversion influences utilization of healthcare delivered by human and artificial intelligence providers (Reference Longoni, Bonezzi and MorewedgeLongoni et al., 2019).
Reference Pezzo and BecksteadPezzo and Beckstead’s (2020) commentary on our article raises the question of whether, in the medical context, algorithm aversion takes the form of a noncompensatory decision strategy determined by a single attribute (i.e., provider type), or if algorithm aversion is one of several attributes considered in a compensatory decision of which healthcare provider to utilize (Reference Elrod, Johnson and WhiteElrod et al., 2004). In other words, do people always prefer human providers to AI providers, no matter the circumstances? Or do they exhibit a relative preference for healthcare delivered by human providers than by AI providers, which can be outweighed by other attributes, such as the price and performance of each provider? Fortunately, our article provides a clear answer (Reference Longoni, Bonezzi and MorewedgeLongoni et al., 2019).
We hypothesized that, all else equal, people prefer to receive healthcare from a human provider than from an AI provider. In other words, whether a provider is an algorithm or human is one of several attributes that influence compensatory healthcare utilization decisions – whether to utilize healthcare delivered by a particular provider, or which healthcare provider to utilize. The studies we report tested our theory across a variety of forms of healthcare (prevention, diagnoses, treatments), medical conditions (e.g., stress, skin cancer, emergency triage, coronary by-pass surgery), and preference elicitation measures (utilization decisions, pairwise choice, relative preferences, adherence, and willingness to pay). We found that participants were on the average less likely to utilize healthcare delivered by an AI provider than a comparable human provider (Study 1). Participants were willing to pay more to switch from an AI provider to a comparable human provider than vice versa (Study 2). Participants were less sensitive to provider performance information when indicating their relative preference between an AI and human provider, than when indicating their preference between two human providers (Studies 3A–3C). In a conjoint analysis, where respondents faced a trade-off among healthcare provider type (human versus AI), performance, and price, we found negative perceived utility associated with an AI provider relative to a human provider (Study 4). These findings clearly show that, all else equal, participants were less likely to utilize the same healthcare delivered by an AI provider than by a human provider. These results suggest that, in the medical context, algorithm aversion is one of many important attributes that influence healthcare utilization and provider choice.
It is important to note that, based on a reading of our discussion of studies 3A and 3C, Reference Pezzo and BecksteadPezzo and Beckstead (2020) mischaracterized our predictions and conclusions. We did not claim that, “people always prefer a human to an artificially intelligent (AI) medical provider.” While we see how it is possible to have extracted that interpretation from the verbatim text of a few sentences of our paper, their interpretation is inaccurate and obviously wrong.
Their commentary further remarks that our results show that “People actually did prefer the AI provider so long as it outperformed the human provider.” This remark made us realize that what seemed obvious to us, might not have been obvious to readers. Reference Pezzo and BecksteadPezzo and Beckstead (2020) implicitly assume that resistance to medical AI implies a noncompensatory decision rule that ought to be assessed with respect to an absolute reference point (e.g., the point of indifference on the scale we used in studies 3A-3C). We made a conscious effort to communicate our stance on the compensatory nature of this decision process as clearly as possible, and often, in our manuscript. In the abstract, we state that “(consumers) are less sensitive to differences in provider (AI as compared to human) performance.” In the General Discussion, we remark that “in studies 3A–3C, participants exhibited weaker preference for a provider that offered clearly superior performance when such provider was automated rather than human” (p. 645). In the General Discussion, we further remark that, “The choice-based conjoint in study 4 showed a negative partworth utility for an automated provider, suggesting that, when we control for accuracy and cost, participants preferred a human to an automated provider” (p. 645; all italics added). Overall, these studies illustrate that all else equal, consumers are reluctant to utilize healthcare provided by AI rather than human providers, but that other factors, such as price and performance also significantly contribute to provider choice.
We did not emphasize these obvious points, but a casual reader should note that the results of several of our “effect” studies (1–4) explicitly demonstrate that other factors also contribute to choice of provider – that provider choice was compensatory. In study 2, the focal dependent variable is the amount of money at which participants were indifferent between a human and an AI provider. Thus, price and provider choice were compensatory. In study 4, compensatory decisions were also evident, as the partworth utilities of price, performance, and provider (human or AI) were all significant. Moreover, tests of our process account demonstrated several moderators, cases in which participants exhibited no reluctance to utilize healthcare delivered by an AI provider relative to healthcare delivered by a comparable human provider. Participants were happy to utilize AI providers that were personalized to their conditions in study 7, were happy to utilize AI providers if there was human oversight of their decisions in study 9, and we illustrated how individual differences moderated preferences for healthcare providers in study 5.
An important question for future work to investigate is whether, and under what circumstances AI healthcare providers are preferred to human healthcare providers. As we explicitly discuss in our paper (Reference Longoni, Bonezzi and MorewedgeLongoni et al., 2019, p. 647), we believe that many people may prefer algorithmic to human judgment if they are being tested or treated for stigmatized health conditions (e.g., sexually transmitted diseases). Another circumstance where algorithms might be preferred is when interacting with the provider might endanger his or her life (e.g., triage services for COVID-19).
We wish to correct a second inaccurate claim made by Reference Pezzo and BecksteadPezzo and Beckstead (2020), that “the effects of accuracy could not be tested in seven of their ten studies, either because participants received incomplete accuracy information that did not allow for a direct comparison between human and computer (studies 1 and 4).” Yet, performance statistics for human and AI providers were presented in exactly the same, complete format to all participant across all studies, whether within or between subjects. Study 4 employed a conjoint-based exercise, in which participants made several choices among three providers that explicitly varied in price, performance, and type (i.e., AI versus human; see Figure 1). The output of the choice-based conjoint provides a partworth utility measure for each of those attributes, which describes the relative impact of each attribute when they are considered together (Table 1). These values suggest that provider type was the most important attribute, but that accuracy was a close second, and price was a relatively distant third. These relative ratings demonstrate unambiguously that if performance gaps are sufficiently great, then people may prefer an AI to a human provider. Studies 3A–3C, which Pezzo and Beckstead highlight in their commentary, employed a joint evaluation paradigm, specifically to observe comparative preferences when accuracy was easily comparable and easily evaluable. Manipulation checks confirmed that participants encoded and recalled this information correctly.
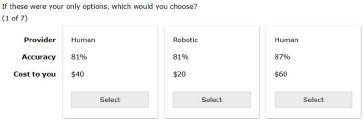
Figure 1: Sample conjoint choice task in study 4 (reproduced from Reference Longoni, Bonezzi and MorewedgeLongoni et al., 2019).
Table 1: Utilities from choice-based conjoint task in study 4 (reproduced from Reference Longoni, Bonezzi and MorewedgeLongoni et al., 2019).
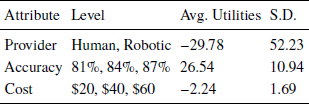
Our findings provide a clear answer to the question Reference Pezzo and BecksteadPezzo and Beckstead (2020) allude to in their commentary. Algorithm aversion is one of several attributes that can be included in a compensatory decision process that determines healthcare utilization and choice of healthcare providers. Put simply, resistance to medical AI is an important factor in a compensatory decision strategy, not a noncompensatory decision rule. Clearly, people do not always reject healthcare provided by AI, and our article never claimed that they do.