INTRODUCTION
Cognitive deficits are a defining feature of preclinical dementia, observable years or even decades before a clinical diagnosis (Boraxbekk et al., Reference Boraxbekk, Lundquist, Nordin, Nyberg, Nilsson and Adolfsson2015; Elias et al., Reference Elias, Beiser, Wolf, Au, White and D’Agostino2000; Rajan, Wilson, Weuve, Barnes, & Evans, Reference Rajan, Wilson, Weuve, Barnes and Evans2015). While research has often focused on episodic memory, a broad range of cognitive domains show deficits during the preclinical phase (Bäckman, Jones, Berger, Laukka, & Small, Reference Bäckman, Jones, Berger, Laukka and Small2005).
Tests from the episodic memory (Devanand et al., Reference Devanand, Liu, Tabert, Pradhaban, Cuasay, Bell and Pelton2008; Eckerström et al., Reference Eckerström, Olsson, Bjerke, Malmgren, Edman, Wallin and Nordlund2013; Gomar, Bobes-Bascaran, Conejero-Goldberg, Davies, & Goldberg, Reference Gomar, Bobes-Bascaran, Conejero-Goldberg, Davies and Goldberg2011) or executive function (Eckerström et al., Reference Eckerström, Olsson, Klasson, Berge, Nordlund, Bjerke and Wallin2015; Ewers et al., Reference Ewers, Walsh, Trojanowski, Shaw, Petersen, Jack and Hampel2012) domains are often identified as the best individual predictors of future dementia and Alzheimer’s disease (AD). It has been suggested that the best results can be obtained through the combination of multiple tests, spanning several cognitive domains (Belleville et al., Reference Belleville, Fouquet, Hudon, Zomahoun, Croteau and Consortium Early2017; Belleville, Gauthier, Lepage, Kergoat, & Gilbert, Reference Belleville, Gauthier, Lepage, Kergoat and Gilbert2014; Palmer, Backman, Winblad, & Fratiglioni, Reference Palmer, Backman, Winblad and Fratiglioni2003; Tian, Bucks, Haworth, & Wilcock, Reference Tian, Bucks, Haworth and Wilcock2003), with combinations among domains of episodic memory and executive function (Albert, Moss, Tanzi, & Jones, Reference Albert, Moss, Tanzi and Jones2001; Tierney et al., Reference Tierney, Szalai, Snow, Fisher, Nores, Nadon and St George-Hyslop1996), or episodic memory and verbal fluency (Gallagher et al., Reference Gallagher, Mhaolain, Coen, Walsh, Kilroy, Belinski and Lawlor2010; Palmer et al., Reference Palmer, Backman, Winblad and Fratiglioni2003), shown to be particularly good at predicting future dementia. Models combining across cognitive measures have been found to be highly predictive, comparable to models including preclinical biomarkers from multiple modalities (Gomar et al., Reference Gomar, Bobes-Bascaran, Conejero-Goldberg, Davies and Goldberg2011; Payton et al., Reference Payton, Kalpouzos, Rizzuto, Fratiglioni, Kivipelto, Bäckman and Laukka2018).
Although the predictivity of cognitive measures may be influenced by various factors, one factor that may modify the patterns of cognitive deficits in the preclinical phase is age. Previous research has shown that during early old age, those who develop dementia tend to show a pattern of cognitive deficits more closely associated with AD (episodic memory deficits), whereas during later old age a broader pattern of deficits, spanning multiple cognitive domains, is seen (Bondi et al., Reference Bondi, Houston, Salmon, Corey-Bloom, Katzman, Thal and Delis2003; Stricker et al., Reference Stricker, Chang, Fennema-Notestine, Delano-Wood, Salmon and Bondi2011). As the older old are more often affected by mixed pathologies (Esiri et al., Reference Esiri, Matthews, Brayne, Ince, Matthews, Xuereb and Morris2001), these differences may be driven by differences in dementia subtype. If the patterns of cognitive deficits differ between young-old and old-old persons, tailoring the cognitive tests used within dementia prediction models to suit the age group being targeted may be beneficial.
Evidence supports a link between low education and increased dementia risk (Beydoun et al., Reference Beydoun, Beydoun, Gamaldo, Teel, Zonderman and Wang2014; Sharp & Gatz, Reference Sharp and Gatz2011). Furthermore, educational attainment is associated with level of cognitive performance, but not with rate of age-related cognitive decline (Berggren, Nilsson, & Lövdén, Reference Berggren, Nilsson and Lövdén2018). A recent meta-analysis by Opdebeeck, Martyr, and Clare (Reference Opdebeeck, Martyr and Clare2016) showed a small-to-moderate effect of education on cognitive performance in later life, with this effect varying little over cognitive domains. How, or if, the level of education would affect the predictive value of tests from different cognitive domains is therefore worth investigating.
Deficits across multiple cognitive domains have been shown in both preclinical AD (Bäckman et al., Reference Bäckman, Jones, Berger, Laukka and Small2005; Saxton et al., Reference Saxton, Lopez, Ratcliff, Dulberg, Fried, Carlson and Kuller2004; Twamley, Ropacki, & Bondi, Reference Twamley, Ropacki and Bondi2006) and preclinical vascular dementia (VaD) (O’Brien et al., Reference O’Brien, Erkinjuntti, Reisberg, Roman, Sawada, Pantoni and DeKosky2003; Roh & Lee, Reference Roh and Lee2014). Although separation between AD and VaD based on cognitive performance is difficult (Bäckman & Small, Reference Bäckman and Small2007; Graham, Emery, & Hodges, Reference Graham, Emery and Hodges2004; Laukka, Jones, Small, Fratiglioni, & Bäckman, Reference Laukka, Jones, Small, Fratiglioni and Bäckman2004; Mathias & Burke, Reference Mathias and Burke2009), there are some distinguishing factors. For example, cognitive decline has been shown to start earlier in AD (Laukka, MacDonald, Fratiglioni, & Backman, Reference Laukka, MacDonald, Fratiglioni and Backman2012) and episodic memory has been found to be relatively more impaired in AD compared to VaD (Andriuta et al., Reference Andriuta, Roussel, Barbay, Despretz-Wannepain, Godefroy and Grp2018; Graham et al., Reference Graham, Emery and Hodges2004), suggesting that dementia type may play a role in the pattern of preclinical cognitive deficits.
Which cognitive tests work best in prediction models may also differ between the sexes. For example, women are more at risk of AD-type dementia (Podcasy & Epperson, Reference Podcasy and Epperson2016; Seshadri et al., Reference Seshadri, Wolf, Beiser, Au, McNulty, White and D’Agostino1997) and, as mentioned above, different dementias have different cognitive profiles (Andriuta et al., Reference Andriuta, Roussel, Barbay, Despretz-Wannepain, Godefroy and Grp2018; Graham et al., Reference Graham, Emery and Hodges2004). There is also evidence suggesting that risk profiles for dementia are different for men and women (Artero et al., Reference Artero, Ancelin, Portet, Dupuy, Berr, Dartigues and Ritchie2008). Within cognitive profiles, it is well documented that women perform better on verbal tasks and men on visuospatial and motor tasks (Li & Singh, Reference Li and Singh2014), with the female advantage on verbal memory tasks seemingly retained during early stages of dementia (Ferretti et al., Reference Ferretti, Iulita, Cavedo, Chiesa, Schumacher Dimech, Santuccione Chadha and Hampel2018; Sundermann et al., Reference Sundermann, Maki, Rubin, Lipton, Landau and Biegon2016). These differences between sexes may potentially result in different cognitive profiles in the preclinical dementia phase for male and females.
Carrying the ϵ4 allele of the apolipoprotein E (APOE) gene is a strong risk factor for AD (Corder et al., Reference Corder, Saunders, Strittmatter, Schmechel, Gaskell, Small and Pericakvance1993; Raber, Huang, & Ashford, Reference Raber, Huang and Ashford2004; Roses & Allen, Reference Roses and Allen1996). It has been linked to impairment in global cognition, episodic memory, and executive functioning (Wisdom, Callahan, & Hawkins, Reference Wisdom, Callahan and Hawkins2011) as well as steeper rates of decline in episodic memory and perceptual speed (Knopman, Mosley, Catellier, & Coker, Reference Knopman, Mosley, Catellier and Coker2009; Salmon et al., Reference Salmon, Ferris, Thomas, Sano, Cummings, Sperling and Aisen2013). However, detangling the effects of being an ϵ4 carrier from that of being in a prodromal phase of AD has been difficult. The influence of APOE on specific cognitive domains and on dementia risk indicates that the most predictive domains may differ depending on APOE status, with episodic memory most likely to be a stronger predictor for those with an ϵ4 allele compared to those without.
Longitudinal studies mapping rates of decline suggest that some cognitive domains start to decline earlier and some later in relation to the dementia diagnosis, and they may follow different trajectories (Cloutier, Chertkow, Kergoat, Gauthier, & Belleville, Reference Cloutier, Chertkow, Kergoat, Gauthier and Belleville2015). Thus, different cognitive domains may be more or less useful in predicting future dementia depending on time to diagnosis. The effect of time to diagnosis on dementia prediction models has not been comprehensively explored. Few studies have observed cognitive deficits more than 10 years before diagnosis, and results are mixed regarding which cognitive domain is most affected early in the preclinical phase (Amieva et al., Reference Amieva, Le Goff, Millet, Orgogozo, Peres, Barberger-Gateau and Dartigues2008; Elias et al., Reference Elias, Beiser, Wolf, Au, White and D’Agostino2000; Rajan et al., Reference Rajan, Wilson, Weuve, Barnes and Evans2015). Amieva et al. (Reference Amieva, Le Goff, Millet, Orgogozo, Peres, Barberger-Gateau and Dartigues2008) observed significant differences in a test of category fluency between groups of preclinical dementia and cognitively normal participants 12 years before diagnosis. In terms of predictive ability, Elias et al. (Reference Elias, Beiser, Wolf, Au, White and D’Agostino2000) found that tests of episodic memory and abstract reasoning were significantly predictive of dementia at least 10 years before diagnosis. Similarly, Rajan et al. (Reference Rajan, Wilson, Weuve, Barnes and Evans2015) found significant predictive ability of tests of episodic memory and executive function between 10 and 18 years before diagnosis.
In this study, we have access to dementia diagnoses up to 12 years after baseline assessment. We aim to test which cognitive markers are significantly more predictive of future dementia over a model of demographic factors, providing a more stringent test of the models’ clinical usefulness. Moreover, we will test the predictive ability of different markers and combinations of markers at different distances to diagnosis within the same individuals, enabling a proper test of the role of time to diagnosis in dementia prediction.
METHODS
Participants
Data were collected from participants involved in a longitudinal population-based study, the Swedish National Study on Aging and Care in Kungsholmen (SNAC-K). Baseline assessment was conducted on 3363 individuals, belonging to specific age cohorts. Older age groups (≥78 years) were re-examined every 3 years and younger age groups (60–72 years) every 6 years. The assessment at each wave consisted of a nurse interview, a medical examination, and neuropsychological testing.
Of the original sample, 2848 underwent cognitive testing. Due to exclusion and dropout, follow-up data were available for 2357 participants. Of those, 1733 remained dementia-free, 246 developed dementia (of whom 36 were diagnosed from death certificates and medical records), and 378 died during the 6-year follow-up (Figure 1). The main sample analyses focused on predicting dementia up to 6 years later, as results from the 12-year analysis provided very few significant predictors and could not be used for model building. The 6-year follow-up also contained a larger number of participants and therefore allowed for subsample analyses.

Fig. 1. Flow-chart of study participants – main sample.
Time-to-diagnosis sample
A select sample of the SNAC-K participants had cognitive data available at baseline, 6-year, and 9-year follow-ups, with dementia diagnosis performed at 12 years. Only those with data at all time points were included. Everyone who developed dementia or died at or before 9 years were excluded and only those who developed dementia or died between the 9- and 12-year follow-ups were included in the dementia and dead outcome categories, respectively. This subsample included 407 participants, of whom 284 remained dementia-free, 48 were diagnosed with dementia at 12 years (of whom 4 were diagnosed from death certificates and medical records), and 75 died during the same period (Figure 2).

Fig. 2. Flow-chart of study participants – time to diagnosis sample.
Ethical considerations
All stages of SNAC-K have been approved by the Karolinska Institutet’s ethical committee or the regional ethical review board, and written informed consent was collected from all participants. In cases where participants had severe cognitive impairment, a proxy was asked for consent.
Dementia diagnosis
Dementia diagnoses were made according to the Diagnostic and Statistical Manual of Mental Disorders, 4th edition (DSM-IV, 1994). The procedure consisted of three steps. A preliminary diagnosis was made by the examining physician, followed by a secondary diagnosis based on computerized data from the medical examination. In cases of disagreement, a final decision was made by a senior physician. A differential diagnosis of AD was made according to the NINCDS-ARDRA criteria (McKhann et al., Reference McKhann, Drachman, Folstein, Katzman, Price and Stadlan1984). The clinical cognitive assessment used for diagnosis included the Mini Mental State Examination (MMSE; Folstein, Folstein, & McHugh, Reference Folstein, Folstein and McHugh1975), the Clock test (Manos & Wu, Reference Manos and Wu1994), and items regarding memory, executive functioning, problem-solving, orientation, and interpretation of proverbs. The cognitive test battery investigated in this study was not used for diagnostic purposes. For those who died before receiving a dementia diagnosis in SNAC-K, death certificates and medical records were reviewed to identify additional dementia cases.
Cognitive Assessment
Episodic memory was assessed by presenting a word list of 16 unrelated nouns with a new word appearing every 5 s (Laukka et al., Reference Laukka, Lovden, Herlitz, Karlsson, Ferencz, Pantzar and Backman2013). This was immediately followed by a 2-min free-recall task and number of words correctly remembered was recorded. Word recognition was assessed with an untimed list of 32 nouns, including the original words and an equal number of distractors, where recognition reflected number of hits minus number of false alarms.
Two semantic memory tasks were administered. The general knowledge task consisted of 10 moderately difficult questions, and participants were asked to pick the correct answer from two alternatives (Dahl, Allwood, & Hagberg, Reference Dahl, Allwood and Hagberg2009). The vocabulary task involved matching a target word to the correct synonym among five alternatives (Dureman, Reference Dureman1960; Nilsson et al., Reference Nilsson, Bäckman, Erngrund, Nyberg, Adolfsson, Bucht and Winblad1997). In both tasks, semantic memory was measured as number of correct answers.
Verbal fluency was assessed with letter and category fluency. These tasks involved generating as many words as possible within 60 s, either starting with the letters “F” and “A” (letter fluency) or belonging to the categories “animals” and “professions” (category fluency). The fluency measures were derived by averaging the total number of words produced within each task.
Three tasks were used to assess perceptual speed. Digit cancellation (Zazzo, Reference Zazzo1974) comprised 11 rows of random digits and participants were required to mark the target number (4) whenever they encountered it during 30 s. Pattern comparison (Salthouse & Babcock, Reference Salthouse and Babcock1991) consisted of pairs of basic line constructs; 30 s were given to mark the pairs as “same” or “different”. The average number of correct answers was calculated from two trials. Trail Making Test (TMT) part A (Lezak, Reference Lezak2004) involved connecting 13 encircled digits in numeric order as fast and accurately as possible. Time to complete the task constituted the TMT-A score, although time was only taken for those who completed the task correctly, with maximum one careless connection.
Executive function was measured using TMT-B (Lezak, Reference Lezak2004). In this task, circles with numbers and letters were connected based on numeric and alphabetical order, alternating between the two categories (1-A, 2-B, etc.). Similar to TMT-A, time was only taken for those who completed the task correctly, or had maximum one careless connection.
Genotyping
DNA genotyping was performed using Matrix-Assisted Laser Desorption/Ionization - Time Of Flight (MALDI-TOF) analysis on the Sequenom MassARRAY platform (Oeth et al., Reference Oeth, Park, Kosman, del Mistro, van den Boom and Jurinke2005). The APOE (rs429358) polymorphism was analyzed as a binary variable, that is, “ϵ4 versus no ϵ4 carriers”.
Statistical Analyses
All statistical analyses were conducted in IBM SPSS 23. Baseline differences between incident dementia and no-dementia groups were determined using χ 2 tests for dichotomous variables and ANOVAs for continuous variables.
Multinomial logistic regressions were employed to investigate how well various markers, or combination of markers, predicted future dementia, with three outcomes possible: no dementia (reference group), incident dementia, and death. The third outcome was included to take into account mortality as a competing event. However, as the outcome of interest was dementia, only data from the reference and incident dementia groups are reported in this study. Age, sex, and education were included as covariates in all models, except during subgroup analyses where the focus was sex, age, or education, and all variables were entered simultaneously. To determine which marker or combination of markers best predicted future dementia, the receiver operating characteristics (ROCs) were calculated using the estimated probabilities from the multinomial logistic regressions. The area under the curve (AUC) values thus represent the predictivity of each model, consisting of the covariates and one or several cognitive predictors.
The predictive value of individual variables was determined first. Significant individual measures with the highest AUC value within their cognitive domain were entered into subsequent models. This reduced the number of variables and addressed issues of collinearity. The threshold for statistical significance was set to p < .05.
Models were created by starting with the best cognitive predictor (based on AUC value) and adding a second variable, systematically testing all available combinations. The two-variable model with the highest AUC was then used as the base for testing a possible three-variable model using the same method. When no predictor added further unique variance, this was considered the final model. The statistical significance of AUC changes between models was assessed using DeLong’s tests (DeLong, DeLong, & Clarke-Pearson, Reference DeLong, DeLong and Clarke-Pearson1988). The DeLong’s tests allow for increases in predictivity to be evaluated in a statistical manner, where a nonsignificant result would indicate that the addition of further tests is not improving predictivity in a significant way. The Bayesian information criterion (BIC) was used as a measure of model fit, an increase in BIC denotes a worsening of model fit and potential overfitting of the data leading to artificial increases in predictivity.
All continuous variables were standardized and all scores where a higher value was related to a decreased risk were reversed so that odds ratios (ORs) represent increased risk per SD unit change in the predictor.
Subsample analysis
In the subsample analyses, prediction for dementia was again set to up to 6 years before diagnosis, with further categorization made for age, sex, educational level, APOE ϵ4 status, and AD-type dementia. The “old-old” group was ≥78 years and the “young-old” was <78 years old at baseline. High education was defined as those who had attended high school (“gymnasium”) or above, whereas low education included those with maximum 9 years of education. APOE ϵ4 status was a binary subgrouping of carrying at least one ϵ4 allele or no ϵ4 allele. The number of subjects across subgroups is provided in Table 1.
Table 1. Descriptive characteristics across dementia status at follow-up (main sample) and n for subgroupings

a Follow-up refers to the 6-year follow-up for the no dementia and time of diagnosis for the incident dementia group.
RESULTS
Descriptive statistics across the main sample groups are shown in Table 1. For descriptive statistics of the time-to-diagnosis sample, see Table 2. Persons who developed dementia were older, had fewer years of education, and had lower MMSE scores at baseline compared to the no-dementia group in all samples.
Table 2. Descriptive characteristics across dementia status at follow-up (time-to-diagnosis sample)

Main sample
Results from multinomial logistic regressions and ROC analyses showed that the category fluency (verbal fluency) task was the strongest individual predictor of future dementia up to 6 years later (Table 3). This was followed by word recall (episodic memory), pattern comparison (perceptual speed), TMT-B (executive function), and vocabulary (semantic memory), respectively, as the best predictors in their specific domains. These tests were then entered into combined models.
Table 3. Multinomial logistic regressions for individual variables (main sample)

Note. OR, odds ratio; CI, confidence intervals; ROC, receiver operating characteristic curve; AUC, area under the curve.
a Incident dementia versus no dementia.
Adding further variables from other cognitive domains generally increased predictivity (Table 4). The model starting with the strongest individual predictor (category fluency, AUC = .903) was most improved by adding word recall or pattern comparison (AUC = .907), although the increase in predictivity compared to the one-variable model was not significant (DeLong’s test, p = .57). The final model included three predictors, that is, category fluency, word recall, and pattern comparison, and represented a significant increase in predictivity from the one- and two-variable models (AUC = .913, p = .002). The final model achieved a sensitivity of 48.6%, specificity of 98.4%, and an accuracy value of 92.9% in predicting future dementia. All models performed significantly better than a model including only the covariates (p < .001).
Table 4. Multinomial logistic regressions for combined models (main sample)
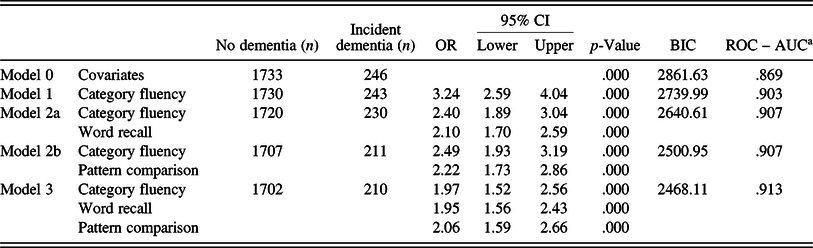
OR, odds ratio; CI, confidence intervals; BIC, Bayesian information criterion; ROC, receiver operating characteristic curve; AUC, area under the curve; Model 0 includes, sex, age, and education.
DeLong’s (statistically comparing AUC values): Model 0 to 1: p < .000; Model 1 to 2: p = .057; Model 2 to 3: p = .015; Model 1 to 3: p = .002.
a Incident dementia versus no dementia.
Repeating the analyses in different subgroups, similar patterns emerged. The strongest predictor among the old-old group was category fluency, with a final model of category fluency, word recall, and TMT-B. While for the young-old group, category fluency and number cancellation were equally predictive, the final models included both of these variables and word recall (supplementary Table S1). The same strongest predictor (category fluency) and pattern of domains (verbal fluency, episodic memory, and perceptual speed) were apparent in a female only sample, while for men, a test of perceptual speed was the strongest individual predictor (supplementary Table S2). Category fluency was the strongest individual predictor for both high- and low-educated subgroups, with tests of verbal fluency, episodic memory, and perceptual speed present in the final models (supplementary Table S3). For those carrying at least one ϵ4 allele, word recall was the strongest individual predictor, although the most predictive model once again included tests of episodic memory, verbal fluency, and perceptual speed. For APOE ϵ4 noncarriers, episodic memory was less important as an individual predictor, but revealed the same pattern of cognitive tests for the final model as all previous analyses (supplementary Table S4). For those who would develop AD-type dementia, category fluency and word recall performed equally well. A final model included tests of category fluency, episodic memory, and perceptual speed (supplementary Table S5).
Sensitivity analyses were performed removing individuals diagnosed with depression, using the International Statistical Classification of Diseases and Related Health Problems - Tenth Revision (ICD-10; n=95), or using anticholinergic drugs (n = 114) at baseline. This did not change the patterns of results (data not shown).
Time-to-diagnosis sample
In the sample with 12 years of follow-up, word recall, vocabulary, general knowledge, category fluency, pattern comparison, and TMT-B were all significant predictors of future dementia 12 years later, with pattern comparison being the most predictive test (AUC = .686). As in the main sample analyses, category fluency was the most predictive individual test (AUC = .733) 6 years before diagnosis. Three years before diagnosis, category fluency was again the strongest predictor (AUC = .781) (supplementary Table S6).
Twelve years before diagnosis, no additional predictors could be added to the model including pattern comparison (AUC = .686). Models 6 years before diagnosis built upon category fluency and TMT-B (AUC = .748) to arrive at a final two-variable model, while 3 years before diagnosis yielded category fluency and TMT-A as a two-variable model (AUC = .794), with the addition of word recall for a final three-variable model (AUC = .814; see Table 5). Twelve years before diagnosis, none of the individual variables were significantly more predictive than a model containing covariates only. However, 6 years before diagnosis, category fluency alone (p = .01) and the two-variable model with category fluency and TMT-B (p < .05) performed better than the covariate model. Three years before diagnosis, all models were significantly more predictive than a model of covariates (p < .001). Comparing the final models, there was no significant difference in predictivity from 12 to 6 years before diagnosis. However, from 12 to 3 years (p = .001), and from 6 to 3 years (p = .021), there was a significant increase in predictivity for the final models.
Table 5. Multinomial logistic regressions for combined models (time-to-diagnosis sample)

OR, odds ratio; CI, confidence intervals; BIC, Bayesian information criterion; ROC, receiver operating characteristic curve; AUC, area under the curve; TMT-B, Trail Making Test-B; TMT-A, Trail Making Test-A; Model 0 includes, sex, age, and education.
DeLong’s (statistically comparing AUC values): 12 years before diagnosis: Model 0–1: p = .188; 6 years before diagnosis: Model 0–1: p = .010; Model 1 to 2: p = .487; Model 0–2: p = .026; 3 years before diagnosis: Model 0–1: p < .001; Model 1–2: p = .102; Model 2–3: p = .155; Model 1–3: p < .001; Model 0–3: p < .001.
a Incident dementia versus no dementia.
Further analyses removing those with depression (ICD-10 diagnosis, n = 13) and those using anticholinergic drugs (n = 23) at baseline did not change the patterns of results.
DISCUSSION
The present study demonstrates that the addition of tests from multiple cognitive domains increases predictivity of future dementia within 6 years. The cognitive domains that found most useful in predicting dementia were verbal fluency, episodic memory, and perceptual speed. Models containing any combination of these domains consistently performed well in predicting future dementia both in general and over a range of modifying factors, such as age, educational level, sex, ϵ4 status, and dementia type. Furthermore, predictivity of the cognitive markers increased closer to diagnosis.
Individual Predictors
While episodic memory is often purported as being an especially good predictor of dementia, this study adds to the literature supporting relative homogeneity among cognitive predictors (Chen et al., Reference Chen, Ratcliff, Belle, Cauley, DeKosky and Ganguli2000). None of the tests included in the modeling differed significantly from one another in this regard. This homogeneity in predictivity reflects deficits over a wide range of cognitive domains, which can be observed in preclinical dementia and AD (Bäckman et al., Reference Bäckman, Jones, Berger, Laukka and Small2005; Economou, Papageorgiou, Karageorgiou, & Vassilopoulos, Reference Economou, Papageorgiou, Karageorgiou and Vassilopoulos2007). This may stem from wide-ranging brain changes in both the hippocampus and beyond, during the preclinical phase (Twamley et al., Reference Twamley, Ropacki and Bondi2006). That said, these changes may affect different cognitive domains differently, with episodic memory being more linked to the hippocampus (Burgess, Maguire, & O’Keefe, Reference Burgess, Maguire and O’Keefe2002) and therefore affected to a greater degree by AD pathology, whereas perceptual speed has been more linked to white matter damage and vascular pathology (Penke et al., Reference Penke, Maniega, Murray, Gow, Hernandez, Clayden and Deary2010; Prins & Scheltens, Reference Prins and Scheltens2015).
A possible reason why tests from a range of domains were predictive of future dementia is that the majority of the persons with dementia were likely to be mixed cases (Esiri et al., Reference Esiri, Matthews, Brayne, Ince, Matthews, Xuereb and Morris2001). Addressing this issue by redoing the analyses in different subsamples rendered some support to this hypothesis. Analyzing the subsamples where the etiology suggested AD or where the persons carried at least one ϵ4 allele indicated greater importance of word recall as a predictor of dementia. This is consistent with episodic memory being an early marker of AD (Backman, Small, & Fratiglioni, Reference Backman, Small and Fratiglioni2001; Elias et al., Reference Elias, Beiser, Wolf, Au, White and D’Agostino2000) and with APOE being a risk factor especially for AD-type dementia (Roses & Allen, Reference Roses and Allen1996). Carrying an ϵ4 allele has been associated with poorer episodic memory performance (Small et al., Reference Small, Rosnick, Fratiglioni and Bäckman2004) and faster episodic memory decline (Knopman et al., Reference Knopman, Mosley, Catellier and Coker2009; Salmon et al., Reference Salmon, Ferris, Thomas, Sano, Cummings, Sperling and Aisen2013). The presence of the same three cognitive tests across the final models suggests robustness of the domains of verbal fluency, episodic memory, and perceptual speed, in predicting future dementia.
Despite the relative homogeneity among the cognitive predictors, category fluency was consistently a good predictor. This may be due to the fact that this task has a broad neural base, covering frontal, parietal, and temporal regions (Baldo, Schwartz, Wilkins, & Dronkers, Reference Baldo, Schwartz, Wilkins and Dronkers2006; Gourovitch et al., Reference Gourovitch, Kirkby, Goldberg, Weinberger, Gold, Esposito and Berman2000), and is thus likely to be affected by several aspects of dementia pathology, making it a good predictor of both AD and VaD. As with previous studies (Clark et al., Reference Clark, Gatz, Zheng, Chen, McCleary and Mack2009), this study found better predictivity of category fluency over letter fluency. It should be noted that category fluency was identified as the strongest predictor both in the main analyses and in the time-to-diagnosis analyses (6 years before diagnosis). Due to the difference in follow-up length, the time-to diagnosis sample represents a different but overlapping group of individuals, which may be considered a replication in a semi-independent sample as different individuals are included in the incident dementia group.
Although not statistically significant, the patterns of cognitive impairment across domains suggest a prediction gap between the more predictive domains of verbal fluency, episodic memory, and perceptual speed, and the less predictive domains of semantic memory and executive function. Similar patterns have been observed previously (Belleville et al., Reference Belleville, Fouquet, Hudon, Zomahoun, Croteau and Consortium Early2017). Semantic memory has been shown to be relatively well preserved in the early dementia stages, possibly as impairment runs along a fluid/crystallized spectrum, with fluid abilities being impaired earlier in disease progression and crystallized abilities later (McDonough et al., Reference McDonough, Bischof, Kennedy, Rodrigue, Farrell and Park2016; Thorvaldsson et al., Reference Thorvaldsson, MacDonald, Fratiglioni, Winblad, Kivipelto, Laukka and Backman2011). As semantic memory showed poor predictivity of future dementia, the results from this study support this idea.
Executive functioning also performed relatively poorly as a predictor in this study, despite belonging to the fluid cognitive domain. The benefit of executive function as a predictive domain has been suggested to be lower than other cognitive domains, such as episodic memory and verbal fluency (Belleville et al., Reference Belleville, Fouquet, Hudon, Zomahoun, Croteau and Consortium Early2017). However, executive function spans a wide range of cognitive abilities and some aspects, for example, updating of representations in working memory, not addressed by the TMT-B test, may be more predictive than others. Due to missing data, TMT-B included fewer participants and, as the AUC is sensitive to sample size, the results are not directly comparable. A subanalysis, including only those who completed all tests, showed that predictivity for executive function was comparable to the other more predictive domains once sample size-related AUC issues were removed (supplementary Table S7). This, alongside its presence in the 6-year final model of the 12-year analysis, suggests that executive function may still be a useful predictor of future dementia.
The similarities in predictivity among the cognitive domains could be extended to subsamples of different age, sex, and educational level. As mixed pathology is most common in the oldest old (Esiri et al., Reference Esiri, Matthews, Brayne, Ince, Matthews, Xuereb and Morris2001), cognitive domains with a broader neural basis, such as category fluency, might be expected to be affected to a greater degree in the old-old, whereas in the young-old the presence of mixed pathology would be less likely to have an influence. Moreover, female gender and higher educational level have been associated with better cognitive performance in non-demented aging.
In regard to education, cognitive performance may reflect educational level as well as disease-specific pathology (Kawano et al., Reference Kawano, Umegaki, Suzuki, Yamamoto, Mogi and Iguchi2010). However, our results suggest that patterns of predictors did not change due to high or low educational level, and that the same tests were useful as predictors of future dementia across different levels of education.
It has been shown that women are at greater risk of AD-type dementia (Podcasy & Epperson, Reference Podcasy and Epperson2016; Seshadri et al., Reference Seshadri, Wolf, Beiser, Au, McNulty, White and D’Agostino1997). However, in the current study, the pattern of cognitive predictors did not vary greatly by type of dementia. This difference may be larger in a sample consisting or more pure dementia types. There was some influence of sex on which cognitive test was found to be the most predictive. This may reflect long-standing differences in cognitive ability between men and women on tasks, such as verbal or spatial abilities (Li & Singh, Reference Li and Singh2014). However, the final models for both sexes included tasks of verbal fluency, episodic memory, and perceptual speed, suggesting that these domains are good predictors of future dementia regardless of sex.
Due to the sensitivity of the AUC measure to sample size, the results from the subsamples cannot be directly compared. However, the overall pattern suggests that the same cognitive markers that showed the highest predictivity in the full sample worked equally well regardless of these sample characteristics, with domains of verbal fluency, episodic memory, and perceptual speed showing consistently good predictivity.
Combined models
Previous research has shown that models of combined neuropsychological tests can improve predictivity (Belleville, Fouquet et al., Reference Belleville, Fouquet, Duchesne, Collins, Hudon and Grp2014; Palmer et al., Reference Palmer, Backman, Winblad and Fratiglioni2003) and may perform equally as well as multimodal models including also biological markers (Gomar et al., Reference Gomar, Bobes-Bascaran, Conejero-Goldberg, Davies and Goldberg2011; Payton et al., Reference Payton, Kalpouzos, Rizzuto, Fratiglioni, Kivipelto, Bäckman and Laukka2018). However, the frequent lack of statistical testing when comparing models in previous studies makes it hard to determine the added value of including additional cognitive markers.
In the present study, we were able to show that combining cognitive tests between multiple domains significantly increased predictivity of future dementia, above models of covariates and individual predictors. One reason why combining across domains may be useful is because cognitive tasks are differentially sensitive to different types of dementia pathology. In line with the results of this study, a task of episodic memory specifically combined with tasks of either verbal fluency (Artero, Tierney, Touchon, & Ritchie, Reference Artero, Tierney, Touchon and Ritchie2003; Small, Herlitz, Fratiglioni, Almkvist, & Backman, Reference Small, Herlitz, Fratiglioni, Almkvist and Backman1997) or perceptual speed (Chapman et al., Reference Chapman, Mapstone, McCrary, Gardner, Porsteinsson, Sandoval and Reilly2011; Jungwirth et al., Reference Jungwirth, Zehetmayer, Bauer, Weissgram, Tragl and Fischer2009) has been shown to increase predictivity to the largest degree. As noted, executive function was not a high-performing predictor in this study; however, there is a strong research basis to suggest that it is a domain which also performs well together with tasks of episodic memory (Albert et al., Reference Albert, Moss, Tanzi and Jones2001; Chen et al., Reference Chen, Ratcliff, Belle, Cauley, DeKosky and Ganguli2000).
While the current data allow for some conclusions regarding which domains and tests may be the best predictors in the early detection of dementia, note that not all cognitive tests within a certain domain are equally useful in predicting dementia. It cannot be discounted that variation among tests can be partially responsible for variations among results between studies. For example, within the episodic memory domain, tasks of word recall are typically better predictors than word recognition (Belleville et al., Reference Belleville, Fouquet, Hudon, Zomahoun, Croteau and Consortium Early2017). Tests which are categorized as the same cognitive domain may also be assessing different aspects of that domain, for example, verbal fluency. Category fluency and letter fluency have been shown to have a different neural basis drawing on semantic and phonologic aspects, respectively (Gourovitch et al., Reference Gourovitch, Kirkby, Goldberg, Weinberger, Gold, Esposito and Berman2000). The exact tests chosen will therefore have an effect on predictivity of the models.
Despite good prediction values, it should be noted that sensitivity of cognitive tests for predicting future dementia was lower than ideal in this study. In line with previous studies, specificity and accuracy of cognitive tests tend to be higher than specificity (Belleville et al., Reference Belleville, Fouquet, Hudon, Zomahoun, Croteau and Consortium Early2017). It has been shown that the addition of biological markers to cognitive tests can increase sensitivity, as is the case with cerebral spinal fluid (CSF) (Mazzeo et al., Reference Mazzeo, Santangelo, Bernasconi, Cecchetti, Fiorino, Pinto and Magnani2016) or MRI (Peters, Villeneuve, & Belleville, Reference Peters, Villeneuve and Belleville2014) markers. Nevertheless, cognitive testing constitutes a quick and inexpensive way of first identifying at-risk individuals on a large scale before further, more expensive or invasive testing, such as CSF or MRI markers are used.
Time-to-diagnosis
In the time-to-diagnosis sample, most cognitive tests and combined models were significantly more predictive closer to a diagnosis of dementia. This is consistent with earlier findings which show an increase in predictive value or an increase in magnitude of the effect as the dementia diagnosis approaches (Boraxbekk et al., Reference Boraxbekk, Lundquist, Nordin, Nyberg, Nilsson and Adolfsson2015; Elias et al., Reference Elias, Beiser, Wolf, Au, White and D’Agostino2000; Gomar, Conejero-Goldberg, Davies, & Goldberg, Reference Gomar, Conejero-Goldberg, Davies and Goldberg2014; Rajan et al., Reference Rajan, Wilson, Weuve, Barnes and Evans2015). Conceivably, cognitive deficits become more pronounced closer to diagnosis due to a continuing worsening of the underlying disease pathology as the disease progresses. While the results are in keeping with previous studies, these studies have typically used different groups of dementia cases in their time to dementia classification. In contrast, this study followed the same individuals over an extended period with a narrow time window for testing, allowing for a direct comparison within individuals at specific times before diagnosis.
Twelve years before diagnosis, perceptual speed was the most predictive individual test of dementia. While few studies have been conducted covering such an extended time period, there are studies showing the possibility to predict dementia far in advance (Boraxbekk et al., Reference Boraxbekk, Lundquist, Nordin, Nyberg, Nilsson and Adolfsson2015; Elias et al., Reference Elias, Beiser, Wolf, Au, White and D’Agostino2000). Tests of verbal fluency, specifically category fluency, (Amieva et al., Reference Amieva, Le Goff, Millet, Orgogozo, Peres, Barberger-Gateau and Dartigues2008), episodic memory (Elias et al., Reference Elias, Beiser, Wolf, Au, White and D’Agostino2000; Rajan et al., Reference Rajan, Wilson, Weuve, Barnes and Evans2015), and executive function (Rajan et al., Reference Rajan, Wilson, Weuve, Barnes and Evans2015) have all been found to predict dementia over a decade before diagnosis. In line with these findings, tests representing these domains were found to predict future dementia 12 years before diagnosis (supplementary Table S6), although the strongest predictor observed in this study was pattern comparison (representing perceptual speed). However, applying more stringent testing using the DeLong’s test, which most previous studies have not applied, none of the individual tests performed significantly better than a model of covariates in predicting future dementia, suggesting that 12 years before diagnosis may be too far to accurately predict dementia in the population. That significantly predictive models can be made 6 years, but not 12 years, before diagnosis suggests that neuropsychological tests can be used to accurately predict dementia during the final 6 years before dementia but further away from diagnosis predictions become more uncertain.
Although it was clear that the cognitive markers were better predictors of dementia closer to diagnosis, the relative importance among the predictors did not appear to substantially change over time. It has been shown that the rate of cognitive decline in the preclinical phase differ across cognitive domains (Cloutier et al., Reference Cloutier, Chertkow, Kergoat, Gauthier and Belleville2015; Grober et al., Reference Grober, Hall, Lipton, Zonderman, Resnick and Kawas2008; Thorvaldsson et al., Reference Thorvaldsson, MacDonald, Fratiglioni, Winblad, Kivipelto, Laukka and Backman2011), suggesting that different domains may be more or less useful for predicting future dementia depending on time until diagnosis. However, using the scores from single points in time (as opposed to investigating rate of decline), this study rather points to the same domains being the most predictive of future dementia. While the best cognitive domain predictors altered depending on time to diagnosis, one or more tests of verbal fluency, episodic memory, and perceptual speed were once again present in each final model, suggesting a robustness of these domains for predicting future dementia regardless of time until diagnosis.
Strengths and limitations
A major strength of the current study is the population-based sample, making the results generalizable outside a clinical setting. Also, the use of tests to diagnose dementia which were not included as predictors reduces circularity in diagnosis. Alongside this, there was a strict test of time to diagnosis within the same individuals. A potential limitation is that the results from the main and subsamples were not directly comparable, and the time-to-diagnosis sample was smaller and more selective compared to the main sample.
The focus on preclinical cognitive deficits regardless of mild cognitive impairment (MCI) status renders the study more inclusive. However, it also means some of those classified as dementia-free at follow-up may have had MCI, resulting in lower prediction accuracy than may have been achieved if comparing cognitively normal and dementia groups.
Implications
Our results show that combining cognitive tests across multiple domains significantly increases the ability to predict future dementia, and that the same cognitive tests and combinations of test are predictive of future dementia across a number of subgroups, such as age, educational level, sex, APOE status, or dementia type. Alongside the fact that patterns of the most predictive tests remained stable both further and closer to diagnosis of dementia, this suggests that tests of verbal fluency, episodic memory, and perceptual speed can be widely used as screening tools to detect individuals with increased dementia risk in the general population.
ACKNOWLEDGMENTS
We thank the participants as well as all staff involved in the data collection and management of the SNAC-K study. SNAC-K is financially supported by the Swedish Ministry of Health and Social Affairs, the participating County Councils and Municipalities, and the Swedish Research Council. This work was further funded by grants from the Swedish Council for Working Life and Social Research (EL, LF, LB), the Swedish Research Council (EL, LF), Karolinska Institutet doctoral grant (NP), Swedish Alzheimer Foundation (EL), Osterman Foundation (EL), Gamla Tjänarinnor Foundation (EL), Knut and Alice Wallenberg Foundation Sweden (MK), Center for Innovative Medicine at Karolinska Institutet Sweden (MK), Stiftelsen Stockholms sjukhem Sweden (MK), Konung Gustaf V:s och Drottning Victorias Frimurarstiftelse Sweden (MK), and a donation from the af Jochnick Foundation (LB). This study was accomplished while NP was affiliated with the Swedish National Graduate School for Competitive Science on Aging and Health (SWEAH), which is funded by the Swedish Research Council.
CONFLICTS OF INTEREST
The authors declare no conflicts of interest.
SUPPLEMENTARY MATERIAL
To view supplementary material for this article, please visit https://doi.org/10.1017/S1355617720000272