Introduction
Pre-pregnancy overweight and obesity are associated with increased risk of pregnancy complications, such as gestational diabetes mellitus, Reference Athukorala, Rumbold, Willson and Crowther1–Reference Chu, Callaghan and Kim7 preeclampsia, Reference Athukorala, Rumbold, Willson and Crowther1,Reference Baeten, Bukusi and Lambe2,Reference Cedergren8,Reference Fraser, Tilling and Macdonald-Wallis9 macrosomia Reference Baeten, Bukusi and Lambe2 and stillbirth. Reference Cedergren8 Intrauterine exposure to high maternal adiposity or high gestational weight gain (GWG) is associated with adverse fetal development and may influence the offspring’s health later in life, according to the Developmental Origins of Health and Disease (DOHaD) hypothesis. Reference Barker, Gluckman and Godfrey10–Reference Pringle, Lee and Weatherall13 However, whether the observed effects are due to intrauterine effects directly following mother’s overweight or explained by shared environmental or genetic factors is under debate. Reference Hannon, Knox and Sugden14
Environmental factors may translate into epigenetic modifications that can alter gene expression without changing the DNA-sequence, such as DNA methylation, histone modification or micro-RNAs (miRNAs). Reference Fleming, Watkins and Velazquez15,Reference Felix and Cecil16 DNA methylation results from the addition of a methyl group to the 5′-C, modifying the interactions between DNA and proteins, for example, transcriptional machinery, and could change gene expression. Reference Feinberg17 DNA methylation usually occurs at CpG-dinucleotides, creating methylcytosine (5-mCG). The CpG-sites studied most in mammals are so-called CpG clusters or CpG islands, which are often found in association with genes. Reference Jones and Takai18 miRNAs can influence gene expression as they are small RNA molecules that are complementary to specific transcribed mRNA sequences, can bind to these and thus lower their expression levels. Reference Mohr and Mott19 Histone modifications are post-translational modifications at histone tails that alters chromatin structure resulting in either increased or decreased transcriptional activity. Reference Jorde, Carey and Bamshad20
The relationship between obesity and CpG-site methylation has been described extensively in non-pregnant populations, Reference van Dijk, Tellam, Morrison, Muhlhausler and Molloy21–Reference Drummond and Gibney25 but to our knowledge, there are no systematic reviews exploring how maternal body mass index (BMI) and GWG are associated with DNA methylation, miRNA or histone modification in maternal, placental or offspring tissues. This systematic review aimed to summarize the current literature on associations between maternal BMI and GWG and epigenetic marks.
Materials and Methods
This systematic review was performed according to the PRISMA statement for reporting systematic reviews. Reference Liberati, Altman and Tetzlaff26 The search method and inclusion criteria were defined in advance and the protocol was registered in PROSPERO (registration number: CRD42018094349). We assessed for eligibility all articles that reported weight status prior to and/or during pregnancy associated with epigenetic modifications in either maternal, placental, or offspring tissues, in otherwise healthy pregnant women. Only papers published in the English language were assessed. We chose a descriptive design for this review since all types of epigenetic marks in both maternal and offspring tissues were eligible for inclusion. The descriptive design was further supported by variations in methods, tissues, the timing of weight status variables, and results. The primary outcomes reported are the associations between weight status and DNA methylation, histone modification or miRNA.
Comprehensive searches in the PubMed (which includes the MEDLINE database) and EMBASE databases were completed on October 10th, 2019. We used a combination of the terms and synonyms: pregnancy, gestation, maternal, weight gain, adiposity, BMI, obesity, epigenomics, epigenetic, CpG, methylation, miRNA and histone modifications (for full search, see Supplementary Table S1). A total of 1252 records were screened, and 66 full-text articles were assessed for eligibility (Fig. 1). We also searched the US National Library of Medicine database clinicaltrials.org and reference list of each included paper manually to identify other suitable studies.

Fig. 1. Flow chart describing the paper selection process.
Two independent authors reviewed the searches, read and extracted relevant abstracts. Two independent authors read the included papers. The full author team discussed potential inconsistencies.
Articles studying the effect of maternal weight loss after bariatric surgery Reference Guenard, Deshaies and Cianflone27,Reference Berglind, Muller and Willmer28 were excluded due to the risk these patients have of nutritional deficiencies. Reference Bal, Finelli, Shope and Koch29 Studies with famine as the exposure were also excluded due to difficulties untangling whether the effects on DNA methylation worked via nutritional deficiencies, underweight, insufficient weight gain or stress.
We systematically reviewed information on sample size, study design, a number of CpG-sites/miRNAs studied, phenotype studied, handling of potential confounders like ethnicity and cell composition, and correction for multiple testing if applicable. To identify potential consistencies in findings, we systematically sorted results by the corresponding gene to the CpG-sites discovered, or the specific miRNA studied. We reported the tissues studied, the direction of the association with BMI or GWG, and a description of the corresponding gene function (Supplementary Tables S2 and S3, respectively).
We used the packages “pwr” Reference Champely30 and “ggplot2” 31 in R v. 3.6.2 (https://cran.r-project.org/) to calculate the sample sizes necessary for epigenetic studies to achieve a statistical power of 80 %, using both approaches for a linear model, and comparison of means in a case–control design. We compared the needed sample size for different effect sizes for candidate studies (α = 0.05), Bonferroni correction for 450 k tests (α = 450,000/0.05 = 1.11 × 10−7) and Bonferroni correction for 850 k tests (α = 850,000/0.05 = 5.88 × 10−8).
Results
We identified 49 studies that met the inclusion criteria. Amongst these, 29 studies examined the association of epigenetic marks to maternal BMI, two related to GWG, 15 to both maternal BMI and GWG, and one to maternal BMI and fat mass. Pre-pregnancy BMI (ppBMI) was used in 37 of the included studies, while 12 used maternal BMI measured in pregnancy. Of the 49 included studies, 9 were Epigenome-Wide Association Studies (EWASs), 3 examined total miRNA, and the rest were targeted studies on candidate CpG-sites or miRNAs (Tables 1–3). We did not find any studies examining histone modifications. With the exception of the Pregnancy and Childhood Epigenetics (PACE) consortium meta-analysis which included 19 cohorts (9340 mother–newborn pairs), Reference Sharp, Salas and Monnereau32 the sample sizes were generally small; 18 studies with n < 50, 10 studies with n = 50–100, 11 studies with n = 100–500 and 12 studies with n > 500 (Tables 1–3). Twenty-four of the studies were performed in a case–control design. Seven studies included only one ethnic group, 16 studies did not specify the ethnic groups of their participants and 24 studies included mixed ethnic groups.
Table 1. Systematically reviewed papers studying epigenetics in maternal tissues

ppBMI, pre-pregnant BMI; GWG, gestational weight gain; WBC, white blood cells; RT-PCR, real-time polymerase chain reaction/quantitative PCR; NS, not significant or could not be validated.
a Term as used in paper.
Table 2. Systematically reviewed papers studying epigenetics in placental tissue

ppBMI, pre-pregnant BMI; GWG, gestational weight gain; WBC, white blood cells; RT-PCR, Real time polymerase chain reaction/quantitative PCR; NS, not significant or could not be validated.
a Term as used in paper.
Table 3. Systematically reviewed papers studying epigenetics in offspring tissues
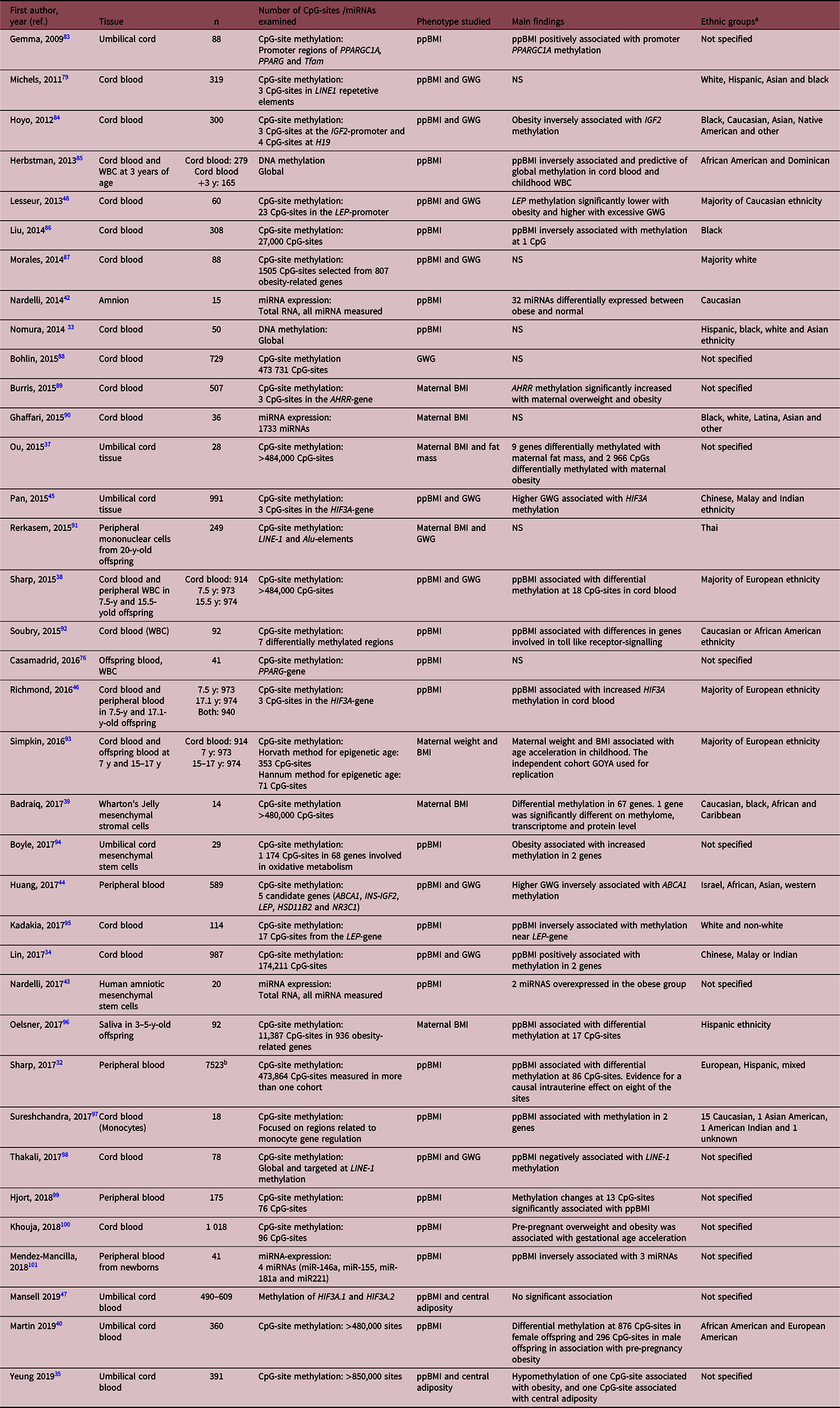
ppBMI, pre-pregnant BMI; GWG, gestational weight gain; WBC, white blood cells; Y, years; NS, not significant or could not be validated.
a Term as used in paper.
b Meta-analysis from the pregnancy and childhood epigenetics consortium.
Supplementary Table S2 provides a summary of the corresponding genes of the CpGs studied, the tissues they have been studied in, their association with BMI or GWG, and their suggested function. Supplementary Table S3 provides the same data for miRNAs.
Genome-wide analyses
EWASs were reported in nine studies, one in the placenta and eight in offspring tissues. To correct for multiple testing, four used Bonferroni Reference Sharp, Salas and Monnereau32–Reference Yeung, Guan and Mumford35 and five used false discovery rate (FDR). Reference Kawai, Yamada and Abe36–Reference Martin, Jima and Sharp40 The largest study was a meta-analysis of newborn peripheral blood from 19 cohorts (n = 7523 included in this specific analysis) by Sharp et al. Reference Sharp, Salas and Monnereau32 The authors observed an association between ppBMI and newborn peripheral blood DNA methylation at eight CpG-sites (after Bonferroni correction). Reference Sharp, Salas and Monnereau32 The second-largest EWAS (n = 914), reported on DNA methylation in cord blood, finding 18 CpG-sites associated with ppBMI (FDR correction). Reference Sharp, Lawlor and Richmond38 These data may suggest a transplacental effect of mother’s BMI on the offspring’s epigenome.
Untargeted studies of miRNA were reported in one study of maternal blood and two in offspring tissues. In the study of maternal white blood cells (n = 40), Enquobahrie et al. Reference Enquobahrie, Wander and Tadesse41 found 27 miRNAs differentially expressed in association with ppBMI. One of the reported miRNAs that showed higher expression with ppBMI, Let-7d, was also reported in a study of amnionic cells (n = 15) by Nardelli et al. Reference Nardelli, Iaffaldano and Ferrigno42 Nardelli et al. also performed an independent study (n = 20) in mesenchymal stem cells from amnion and found higher expression of two miRNAs in samples from the obese participants. Reference Nardelli, Granata and Iaffaldano43 None of these three studies reported adjustment for multiple testing, and the small sample sizes result in low statistical power for untargeted studies of miRNA.
Targeted studies
The search retrieved 31 studies of candidate CpGs. These were mainly CpGs in genes previously associated with BMI or weight gain in non-pregnant populations. Three studies had large sample sizes (n > 500), and are therefore described in more detail. Huang et al. Reference Huang, Siscovick, Hochner, Friedlander and Enquobahrie44 examined peripheral blood of adult offspring (n = 589) and found mother’s GWG to be associated with higher methylation of ABCA1, a gene involved in lipid transportation. Two studies reported differential methylation in HIF3A, a hypoxia-gene; Pan et al. Reference Pan, Lin and Wu45 reported an increase in cord tissue methylation in association with GWG (n = 991), and Richmond et al. Reference Richmond, Sharp and Ward46 reported higher methylation in cord blood in association with ppBMI (n = 973). A recent study Reference Mansell, Ponsonby and Januar47 was not able to find a significant association between ppBMI or central obesity with cord blood methylation in HIF3A (n = 490–609). Lesseur et al. Reference Lesseur, Armstrong and Paquette48 reported differential methylation of CpG-sites in the promoter region of the gene for leptin (LEP) in cord blood (n = 60), which was higher in offspring of obese mothers, and lower in association with excess GWG. In maternal blood, they found lower methylation of the LEP-gene in the obese participants. Reference Lesseur, Armstrong and Paquette48 Nogues et al. Reference Nogues, Dos Santos and Jammes49 showed that DNA-methylation of leptin and adiponectin-systems in placental tissue differed in the obese group (n = 12) compared to non-obese controls (n = 18).
The search retrieved nine studies of targeted miRNAs previously associated with genes regulating inflammatory and metabolic processes related to obesity in non-pregnant populations. Few of the studies explored the same miRNAs (Supplementary Table S3). However, three groups examined the expression of miR-210, a hypoxia-related miRNA. Reference Nakada, Tsukamoto and Matsuura50 Murlaminanoharan et al. (n = 36) Reference Muralimanoharan, Guo, Myatt and Maloyan51 and Prince et al. (n = 52) Reference Prince, Maloyan and Myatt52 found miR-210 to be increased with high ppBMI, but after adjustment for multiple testing, the findings were only significant in pregnancies with female fetuses. In contrast, Tsamou et al. (n = 215) Reference Tsamou, Martens and Winckelmans53 found an inverse association between miR-210 and ppBMI in pregnancies with female fetuses. The findings of the miR-210 direction of expression associated with obesity are inconclusive.
Estimation of Statistical Power
Most of the studies performed linear regression or t-test with a case–control design (Supplementary Table S4). Figure 2a illustrates the sample size needed for a power of 80 % across different effect sizes with linear regression, for candidate studies and untargeted approaches with 450 and 850 k sites. Effect sizes ranging from 0.5% to 5% are shown as examples as most studies reported findings in this magnitude. Figure 2b illustrates the sample size of each group needed for t-test in a case–control design with a power of 80 % across Cohen’s d effect sizes for candidate studies and untargeted approaches with 450 and 850 k sites. Cohen’s d = (mean for Group 1 – mean for Group 2)/pooled SD, where 0.2 is considered a small effect, 0.5 a medium effect and 0.8 a large effect. According to Fig. 2a, b, most of the included studies did not have adequate statistical power to detect small or moderate effect sizes, and some of the studies were also underpowered for large effect sizes.

Fig. 2. Sample size needed for linear regression (A) or t-test in a case–control design (B) with a power of 80% across different effect sizes and significance levels. Significance levels correspond to targeted candidate approach (α = 0.05), and Bonferroni correction of untargeted approaches with the 450 k and 850 k assays (α = 1.11e−07 or α = 5.88e−08, respectively).
Discussion
This systematic review included 49 studies that examined the association of DNA methylation or miRNA to maternal BMI and/or GWG. We found no studies that reported histone modification in relation to ppBMI or GWG. With a few exceptions, most of the studies we reviewed were small, statistically underpowered, with varying methods. We found some consistent results across epigenetic marks and tissue. Taken together, our review suggests that we at present have insufficient evidence to conclude about the relationships between epigenetic marks and ppBMI/GWG.
Two independent studies, one in maternal blood and one in the amnion, found higher Let-7d expression with increasing ppBMI in a genome-wide setting. Reference Enquobahrie, Wander and Tadesse41,Reference Nardelli, Iaffaldano and Ferrigno42 Although it is unclear whether the authors corrected for multiple testing, similar findings in two different tissues may suggest that this miRNA could be of importance. Reference Enquobahrie, Wander and Tadesse41,Reference Nardelli, Iaffaldano and Ferrigno42 The Let-7 miRNA family has target genes linked to type 2 diabetes mellitus, impaired glucose tolerance and insulin resistance. Reference Zhu, Shyh-Chang and Segre54,Reference Frost and Olson55 Both impaired glucose tolerance and insulin resistance are highly correlated with obesity. Reference Shalitin, Abrahami, Lilos and Phillip56
Targeted studies of candidate CpG-sites and miRNAs did in general report on different targets and showed varying results. In 2015, Sharp et al. discovered higher methylation of BDNF in cord blood in offspring of obese mothers in an EWAS, FDR adjusted for multiple testing (n = 914). Reference Sharp, Lawlor and Richmond38 BDNF is a validated miR-210 target, Reference Fasanaro, Greco and Lorenzi57 involved in neuronal development and maintenance in the brain, Reference Schleger, Linder and Walter58 as well as being involved in placental development. Reference Kawamura, Kawamura and Sato59 Prince et al. also found a negative correlation between mature Brain derived neurotrophic factor (BDNF) protein and miR-210 expression (n = 52). Reference Prince, Maloyan and Myatt52 Two independent research groups examined placentas and found increased expression of miR-210 in placentas from pregnancies association with high ppBMI, yet only in pregnancies with female fetuses in female fetuses was found by two independent research groups. Reference Muralimanoharan, Guo, Myatt and Maloyan51,Reference Prince, Maloyan and Myatt52 In contrast, Tsamou et al. (n = 215) Reference Tsamou, Martens and Winckelmans53 reported an inverse relationship between miR-210 and ppBMI, and their sample size was larger (n = 215). Reference Tsamou, Martens and Winckelmans53 Hence, the association between ppBMI and epigenetic marks related to the BDNF gene seems somewhat consistent across tissues and in both DNA methylation and miRNA, and the direction of effect seems to point to repression.
Further, miR-210 is involved in the response to hypoxia in several tissues, and under the direct control of hypoxia-inducible factor (HIF). Reference Kulshreshtha, Ferracin and Wojcik60 HIF3A is a gene linked to BMI in non-pregnant populations. Reference Dick, Nelson and Tsaprouni61 Pan et al. Reference Pan, Lin and Wu45 examined umbilical cord tissue (n = 991) and found HIF3A-methylation to be associated with GWG. Richmond et al. Reference Richmond, Sharp and Ward46 studied umbilical cord blood (n = 973) and found higher methylation of the HIF3A-gene in association with ppBMI, Bonferroni adjusted for multiple testing. Another study did not find significant associations between methylation of the gene in cord blood with neither ppBMI nor central obesity (n = 609). Reference Mansell, Ponsonby and Januar47 A recent study in a large non-pregnant population showed that most obesity-related DNA methylation is a consequence of the obesity, and not the cause – with one exception: methylation of NFATC2IP, which seemed to be predictive of BMI. Reference Wahl, Drong and Lehne62
The variety of examined tissues in the reviewed studies could be considered a strength, such as when the findings seem consistent across tissues and different epigenetic modifications. However, the large variation in tissues, assays, phenotypes (e.g. BMI before, and at different times during pregnancy), as well as an epigenetic mark in the mother or the offspring, may also to a large extent explain the inconsistency in findings. Also, comparing epigenetic signatures across different tissues may prove difficult, as the desired biological response to chosen environmental stimuli may differ across tissues. Further, miRNA findings will vary across input material and type of assay. Reference El-Khoury, Pierson, Kaoma, Bernardin and Berchem63,Reference Brunet-Vega, Pericay and Quílez64 A study compared the performance of absolute (DNA methylation assays for methylation levels of single CpG-sites), relative (comparing samples to references) and global (total methylation content) assays for examining of DNA methylation of specific regions, and found good agreement among all tested methods and between different laboratories. 65 However, it is important to note that the epigenome-wide assays are improving, analyzing new CpG-sites for each generation and that the overlap from the previous chip is not absolute. Reference Pidsley, Zotenko and Peters66 Moreover, different experimental approaches along with diverse bioinformatics pipelines may contribute to potential inconsistencies in findings. As whole-genome bisulfite sequencing (WGBS), the current gold standard to profile CpGs genome-wide, may identify CpGs that are not well covered by other platforms such as reduced bisulfite sequencing (RRBS) or array-based solutions (such as Infinium Human Methylation 450K Beadchip or EPIC array), that are designed to cover preferentially CpG-sites in CpG rich areas. Reference Allum and Grundberg67 Further, as reportedly only a small part of CpG-sites throughout the genome seems to be dynamically regulated and mostly overlaps with regulatory regions that are less well covered on platforms other than WGBS, this may impact on current findings and conclusion drawn from the current literature. Reference Ziller, Gu and Müller68
The methods used to control for multiple testing vary across studies; some use the strict Bonferroni correction, while most use the more relaxed FDR although some fail to report the actual rate used. Consequences of using too strict significance levels in observational studies of epigenetic markers may lead to false negatives, which could leave out possible hits with moderate effect size and thereby blur the understanding of a bigger biological picture. On the other hand, more relaxed significance levels will produce false-positive results, which, if too many, will be difficult to follow-up and substantiate. As shown in Fig. 2b, even large effect sizes require large sample sizes, although a small difference in epigenetic marks may have a significant impact on the function of a cell. Reference Breton, Marsit and Faustman69 Hence, most of the studies reported here have limited statistical power to detect small or moderate effect sizes, and some have limited statistical power to detect even large effects.
Our review unraveled several important challenges when interpreting results of epigenetic studies of maternal BMI and GWG: (1) Sample size – due to the high cost of quantifying epigenetics and the explorative nature of this emerging field, sample sizes are often too small and the studies are often statistically underpowered. Further network collaborations, such as the PACE consortium effort, Reference Sharp, Salas and Monnereau32 will help increase statistical power. (2) Correction for multiple testing – beneficially, researchers should agree on the preferred correction method and significance level, as has been done for GWAS. Reference Panagiotou and Ioannidis70 (3) Lack of reporting essential information – although most of the untargeted studies distinctly report which methods they have used to correct for multiple testing, some fail to do so. Likewise, several of the EWASs did not report how they accounted for cell composition, which is important considering different methylation patterns across cell types. Reference Jaffe and Irizarry71 Several studies failed to report the ethnic origin of the participants, or whether this was accounted for in the statistical analysis. Ethnicity is closely linked to differences in minor allele frequencies of gene variants, Reference Huang, Shu and Cai72 which may impact on DNA methylation or miRNA. In addition, lifestyle and cultural differences across ethnic groups may introduce further bias. Therefore, all studies should distinctly report a method for correction of multiple testing, cell composition, ethnic origin and other important potential confounders. (4) Study design – the majority of the studies presented had a case–control design. This design has clear advantages with regard to the need for smaller sample sizes resulting in lower analysis costs since the maximization of differences leads to larger effect sizes. However, such studies are prone to biases due to unrecognized differences between cases and controls and arbitrary cut-offs to define the groups (e.g. BMI or GWG). Hence, the use of continuous variables in full cohorts may give more robust, comprehensive and reliable results, although they require larger analysis cost and sample size. Further, they allow for the study of several phenotypes and outcomes, so that the cost–benefit may not deviate substantially over time.
From a methodological and conceptual point of view, the main weaknesses of the studies included in this review lie in the multitude of different target tissues analyzed, and in the different nature of the assays applied (amongst them CpG methylation measured by pyrosequencing, array-based or sequencing-based methods (RRBS seq); global DNA methylation or LINE1 assays, miRNA expression etc.). The high variability of applied methods together with, in general, small effect sizes and small sample sets (although there are noteworthy exceptions), hamper us from drawing any causative conclusion so far. Efforts to perform larger and statistically well-powered studies, such as the meta-analysis by Sharp et al., Reference Sharp, Salas and Monnereau32 are warranted.
This systematic review could be affected by language bias, as the inclusion criteria only allowed for studies published in English. However, a retrospective study of meta-analyses found that including or excluding studies published in other languages than English had little impact on effect estimates. Reference Jüni, Holenstein, Sterne, Bartlett and Egger73 This review may also be subject to publication bias, since protocol registration is not required in observational studies and negative results are less likely to be published, especially in statistically underpowered studies.
To conclude, this systematic review of published literature shows that at the present, there is insufficient evidence to conclude about the relationships between mother’s BMI and GWG and their associations to epigenetic modifications in mother and child. However, maternal BMI was associated with both DNA methylation and miRNA related to the expression of the BDNF gene, as well as the HIF3A, across different tissues. We propose a need for larger, well-powered and methodologically coordinated studies, and meta-analyses of independent cohorts, to elucidate potentially important relationships between mothers’ weight and epigenetic differences in the mother and her offspring.
Supplementary materials
For supplementary material for this article, please visit https://doi.org/10.1017/S2040174420000811.
Acknowledgements
None.
Financial support
J.O.O. was supported by the Medical Student Research Program, which is funded by Norwegian Research Council and University of Oslo. G.H.M. and C.S. were funded by the South Eastern Norway Regional Health Authority, grant numbers 2015008 and 2016076.
Conflict of Interest
None declared.