In this review, the term individual dairy cow management (IDCM) will be used rather than precision dairy farming (PDF). The term ‘precision’ seems to be limiting, (like precision spraying or precision irrigation) while ‘individual management’ is more associated with ‘paying attention’, something that will appeal to informed and increasingly welfare-conscious consumers. Arguably, therefore, IDCM is technically and commercially preferable to PDF.
Tracing, storing and recording information on each individual cow in herds that are growing all the time is no longer manageable by many dairy farmers. Sensing technologies were developed to provide animal-centric information to serve as the farmer's ‘eyes and ears’, driven by advanced technologies and powerful computers that provide massive data storage, modeling and computing capabilities. The sensors and technological advances evolved into indispensable individual management aids, which in turn led to IDCM. IDCM can be defined as: enabling the individual cow to reach its full genetic potential in accordance with the farm's economic goals, while maintaining or improving the cow's health and wellbeing. During the last 50 years, sensing has evolved from manually recording individual cow milk production using the ‘jar’ milk meter (scaled glass jar in which the milk was collected and released to the milk pipe after volume recording) to electronic individual identification (ID) and electronic milk meters with automatic computer recording. Numerous off-the-shelf sensors are now available for recording performance, health, reproduction and wellbeing under practical commercial conditions. Even more systems are available for basic research and in various stages of R&D (see below, and Neethirajan, Reference Neethirajan2017). Included in this are sensors that are affixed to the cow in some manner, are introduced into her body internally, or are present in the cow's environment. These sensors provide information on individual cows, ranging from milk yield (MY) and composition and body weight (BW), through estrus detection to rumination time, rumen pH, body temperature, heart rate, behavior, concentrates rationing and more. This multitude of sensors does not include robotics, individual milking frequency nor single milking cup attachment and detachment. The high demand for such off-the-shelf sensors is reflected in the fact that they are available from a variety of manufacturers.
We cannot ignore the gap between the available technologies on the one hand and the disappointing application of IDCM on the other. One of the pioneers of precision livestock farming (PLF), the late Christopher M. Wathes, published an article in 2005 (Wathes et al., Reference Wathes, Kristensen, Aerts, Berkmans and Cox2005) in which he concluded that PLF (including dairy IDCM) ‘…is an embryonic technology with great potential…’, and that in order to materialize it ‘…we foresee four significant hurdles to be overcome….’: These were (and are) technological (precision, cost and ability to handle physiological data and models), specific livestock applications (clear, and based upon sound biological principles), marketing on a commercial scale (to win the confidence of farmers) and bioethics (public concern about using instruments on animals). In addition, following a survey of farmers, Bewley and Russell (Reference Bewley and Russell2010) characterized several obstacles stymieing the adoption and use of IDCM indicating that though a great deal had been done to overcome the hurdles described by Wathes et al. (Reference Wathes, Kristensen, Aerts, Berkmans and Cox2005) there is still a long way to go. From farmers that were either aware or familiar with technologies that are available, the most common problems cited were: ‘immature introduction’ and user ‘unfriendliness’, even when the cost to expected benefit was considered reasonable. Now, 13 years after Wathes original publication, and 10 years after the survey, what does the dairy farmer do with all this technology? Unfortunately, the gap between the potential benefits and the actual exploitation of these technologies is still rather large.
IDCM technology can be divided into two categories: time sensitive and time tolerant. Time sensitive refers to a sensor that requires an immediate response, e.g. the sensor flags estrus in a particular cow. A time tolerant sensor is one that produces data for which the response can wait, without affecting the result. The same sensors can often be used for both categories, although a different approach by the manufacturer and the farmer is necessary. Time sensitive technology is used primarily to provide information about health/illness and reproduction. In the time tolerant category are included, among others, technologies for individual feeding and animal wellbeing. The initial approach in R&D of sensors in the dairy industry was aiming to a single target, i.e. a sensor was developed to address a specific need. For example, a sensor to detect electrical conductivity in milk was developed, for early detection of udder illnesses, particularly mastitis. Similarly, activity sensors were developed for estrus detection. However, it was recognized quite soon that applying several sensors to a single target is far more effective. This requires an adjustable approach to the IDCM process, which encompasses the following elements:
• Sensors that can provide detailed on-line data, that are automatically recorded and stored, about the physiological and behavioral status of an individual cow in a herd, regardless of the herd's size.
• Labeling and interpretation of these data that can be translated into meaningful information about physiological processes.
• Physiological modeling that supports management decision-making at the level of the individual cow, with the option of providing automatic decisions according to pre-defined management strategy.
• Automatic implementation of decisions made, with the option of manual implementation.
When multiple sensors are used for a certain purpose, the data generated by each sensor has to go through the abovementioned process for the purpose for which it is gathering data. For example, milk yield (MY), which is used in both detection of mastitis and estrus, requires different labeling and physiological modeling for each of the goals. Each of these steps contains potential hurdles that challenge promising technologies. IDCM can and is applied to all aspects of dairy management: nutrition, reproduction, health and animal wellbeing. For each of these this review will examine the challenges, the advances and the bottlenecks, and then go on to identify future needs and possibilities. Finally, new R&D directions will be suggested and highlighted for each of these management aspects.
The review will deliberately not consider all, or even most, of the sensors and technologies available and under R&D for IDCM. Rather, it will focus on specific, characteristic examples. A significant proportion of the studies, practical results and examples as well as sensors, hardware and software in this review are from Israeli research at the Volcani Center and in commercial dairy farms or with Israeli manufacturers. Nevertheless, the analysis and conclusions are of a general nature and characterize the achievements, obstacles and prospects of IDCM all over the world. The first part of the review will deal with two examples of sensors, one successfully applied (activity for estrus detection) and one with difficulties in application (body weight (BW) for health, reproduction and nutrition management).The second part will include detailed analysis and discussion of achievements and set backs of IDCM technologies regarding health, reproduction, nutrition and cow wellbeing, in order to identify knowledge and practical gaps that hold back the exploitation of IDCM. This will be done using results obtained and published in these fields in literature as well as our own unpublished data. Some commercially sensors will be identified by name and manufacturer for clarity, but this is in no way made as a recommendation or endorsement of that product.
Successes and difficulties: estrus detection and body weight measurement
In practice, the goal of IDCM is to execute in real time a rational management decision for each individual cow that will have a predictable outcome. For example, when an estrus detection system indicates estrus, the rational decision is to inseminate the cow; the predicted outcome is that the cow will conceive. This example represents the successful application of a time-dependent technology, based on continuous monitoring of cow activity utilizing hardware and software.
The hardware is in the form of a sensor tag affixed to each cow. The sensors monitor the number of steps taken by the cow (SAE Afikim, Israel) or the cow's head movements (SCR Netanya, Israel). The data generated is analyzed using software with an algorithm that compares current to historical activity. In Israel it was adopted by most dairy farms, and has since spread to dairy farms throughout the world including sensors such as those manufactured by IceRobotics (UK) DeLaval (Sweden), BouMatic (USA) and GEA (Germany). A number of factors contributed to the rapid success of these IDCM technologies. First and foremost, they are very user-friendly. The system generates a list of cows whose activity during a specific time period was significantly higher than their average values during the same period in several previous days. This is the basic information that the farmer would need to make the decision to have the cow inseminated. Other parameters, like the amount of time that elapsed after the cow calves, and MY for that time period, could be taken into account as well. The farmer then enters each ‘flagged’ cow's number into the ‘separate cow’ management program, and arranges for insemination. After the next milking, the cows selected will be separated and wait in the separation yard for insemination. In some cases, the entire decision-making process can be done automatically, by setting the thresholds in advance in the management software. In other words, the system doesn't require the farmer to do any data analysis in making the decision to inseminate the cow. In most of the cases, the action will yield the predicted result: satisfactory conception. The method frees him from conducting visual inspection and manually recording standing heat cows.
An additional factor that contributed to the widespread adoption of this technology is the fact that it is maintenance free, and doesn't require any calibration. The manufacturers achieved this objective by thoroughly testing and re-testing the product, first in laboratories and then in the field, before marketing it.
By contrast, the following example demonstrates the hurdles and barriers that Wathes et al. (Reference Wathes, Kristensen, Aerts, Berkmans and Cox2005) and Bewley and Russell (Reference Bewley and Russell2010) spoke about. In the early 1980s, we developed a sensor, a walk through scale, that weighs every cow in the herd after milking. The method was developed for research purposes, to reduce the time and labor entailed in weighing cows manually. Once we succeeded (Peiper et al., Reference Peiper, Edan, Devir, Barak and Maltz1993), the potential of this method as a management tool was recognized and adopted by scientists and farmers. Indeed, several papers were published indicating the significance and usefulness of individual body weight (BW) regarding production (Maltz et al., Reference Maltz, Devir, Kroll, Zur, Spahr and Shanks1992a, Reference Maltz, Grinspan, Edan, Antler, Kroll, Spahr, Ipema, Lipus, Metz and Rossing1992b), nutrition (Maltz et al., Reference Maltz, Devir, Kroll, Zur, Spahr and Shanks1992a, Reference Maltz, Barbosa, Bueno, Scagion, Kaniyamattam, Greco, De Vries and Santos2013), reproduction (Maltz et al., Reference Maltz, Devir, Metz and Hogeveen1997; Van Straten et al., Reference Van Straten, Shpigel and Friger2009a, Reference Van Straten, Friger and Shpigel2009b), health (Maltz, 1997; Maltz et al., Reference Maltz, Devir, Metz and Hogeveen1997; Østergaard and Grohn, Reference Østergaard and Grohn1999) and individual food intake modeling (Halachmi et al., Reference Halachmi, Maltz, Metz and Devir1997, Reference Halachmi, Edan, Moallem and Maltz2004; NRC, 2001; De Vries et al., Reference De Vries, Gay, Barbosa, Du, Kaniyamattam and Maltz2013). Nonetheless, the method is not widely used in managerial IDCM. The system includes hardware and software, generating a daily or weekly record of the BW of every cow in the herd. Each component of the system contains pitfalls that prevents its exploitation despite its potential benefits in IDCM.
The hardware consists of a weighing plate connected to a load cell or several load cells and an ID system, which is placed on the path the cows take out of the milking parlor. To obtain sufficient data from the load cells, a single cow must linger on the weighing plate without hampering the regular flow of cows leaving the milking parlor. Any obstruction in cow traffic that impedes cow movement out of the milking parlor makes the weighing system unusable in commercial dairy farms. Furthermore, the weighing plate is exposed to manure accumulation, which causes erosion, and affects the accuracy and thus the reliability of the data generated by the load cell. As a result, continuous maintenance and calibration are required, which might ruin the potential advantages of using this sensor.
The software has several phases. The first is to obtain a weight from the load cells, after identifying that a single cow is on the weighing plate. Data logic editing is performed to filter out erroneous data generated by the load cells. A reliable weight is then calculated after that particular milking, which is usually the average of all load cell samples that qualify for ‘cow lingering alone with all four legs on the weighing plate’ (Peiper et al., Reference Peiper, Edan, Devir, Barak and Maltz1993). Finally, the daily or weekly weight to be used for individual management is produced. The last phase requires special attention, because of the possibility of missing data. Naturally, not all the cows can be weighed after every milking. In addition, weight fluctuations during the day may reach up to 10% of the cow's weight (Peiper et al., Reference Peiper, Edan, Devir, Barak and Maltz1993). Therefore, a proper ‘missing data’ strategy should be included in the software for the last stage, providing an indication of the reliability of the BW presented. Finally, the way in which the information is presented to the farmer or used for other calculations can be problematic, since the results are purpose dependent. In the literature, BW changes occur not only throughout the day (Peiper et al., Reference Peiper, Edan, Devir, Barak and Maltz1993), but also diurnally (Maltz et al., Reference Maltz, Devir, Kroll, Zur, Spahr and Shanks1992a, Reference Maltz, Devir, Metz and Hogeveen1997). Therefore, special attention is required when associating BW changes with health problems, and in making feeding and reproduction decisions. For time dependent decision making, health for example, it may be enough to present a list of cows whose BW is exceptionally lower in comparison to their normal weight, with a proper indication as for the time window and the reliability of the BW presented. For time tolerate decisions some sort of data smoothing has to be used, even for a cow that is weighed after every milking. In addition, BW is really only useful in management decision-making when it is one of an array of indices provided by a variety of sensors like milk yield, milk composition, activity, etc. (Maltz et al., Reference Maltz, Devir, Metz and Hogeveen1997, Reference Maltz, Barbosa, Bueno, Scagion, Kaniyamattam, Greco, De Vries and Santos2013).
None of the sensor manufacturers currently provide any management decision-making, automatic or manual, or any other information that includes BW data, nor do they provide possible integration of BW data into the data from other sensors that they market. The most that is achieved is presenting a visual indication of the cow's BW changes during the lactation cycle, which may assist the farmer in long term nutritional decisions. As will be described below, BW changes, together with other individual variables, can indicate health problems, concentrates supplementation, suitable insemination timing, dry matter intake (DMI), and body condition (BC) modeling.
In order to expand use of this sensor in the dairy farm and contribute to IDCM in conventional dairy farms, the following points have to be addressed:
Walk through scale: location, cow flow, and infrastructure
In order to allow a fluent cow traffic from the milking parlor with minimized cow standing time and avoiding pushing through the weighing system, there are several steps that should be taken into consideration when installing a walk through or stationary weighing system. The weighing plate should be located as far as possible from the outlet of the milking parlor, especially if the parlor is equipped with a quick release system. Slowing down steps (Peiper et al., Reference Peiper, Edan, Devir, Barak and Maltz1993) or a movable separation device situated before the cow steps onto the weighing plate will help to prevent cows crowding onto the scale. The slowing down step should be of the same height as the weighing plate. In the case of parlors from which cows are released in a row, separation gates over the weighing plate can be useful. If installed, they should be positioned on both ends of the plate, closing and opening at the same time. The weighing plate should be installed on the path on a concrete surface, with a gutter around it, to enable flushing the manure that can accumulate on or underneath the plate.
Walk through scale: hardware improvements and calibration
A visual display over the weighing system, while the cow's ID and weight is recorded, is very important for continuous tracking of the reliability of the weighing process. The display should be big enough to allow data visibility from a distance, so that the cows' motion is not obstructed by the presence of the observer. Manufacturers should provide an easy to use calibration process, either as a built-in feature or as a service. In addition, an alarm should be installed to indicate malfunctioning. For example, gradual but continuous BW decrease of most of the cows using the device usually indicates a load cell malfunction, or manure accumulation under the weighing plate, whilst exceptional numbers of missing data might indicate either a load cell or ID system malfunction.
Walk through scale: software and operation routine
The value of BW that is used to detect health problems may be a single daily weight that drops significantly below a certain threshold of historical weight (Maltz et al., Reference Maltz, Devir, Metz and Hogeveen1997). Other benefits can be derived from several days of a running average that indicates the cow's current energy balance. Changes in the BW slope can be used to time insemination (Maltz, Reference Maltz2010). Several weeks of slope change can indicate that the cow is reaching a satisfactory body condition toward dry off in comparison to its post-calving BW and body condition score.
Sensors for health
As a rule, sensors for detecting health problems are time sensitive. The linkage between measurable physical and behavioral variables accompanying dairy cow ailments, and advanced technology and computing power raised hopes for early detection of health problems. This is based on the conviction (usually well justified) that ‘machines’ are sensitive and accurate enough to identify the physical and/or behavioral variables early enough to treat the cow before the severe outbreak of disease and the economic and welfare damage that it causes. Several steps are necessary to do so:
• identify and delineate a physiological or behavioral characteristic related to a specific disease
• find or develop a technology that measures and quantifies it
• connect this to an ID system
• connect both to a computer
• position both in a location that all cows pass, e.g. a milking parlor, feeding device, etc.
The milk conductivity meter was developed based on the physiological phenomenon that udder health problems are usually accompanied by the penetration of extracellular electrolytes into the milk, changing its electrical conductivity. Electrical conductivity can be measured in the milk outflow when milking the cow. Another hypothetical example: ketosis is accompanied with presence of acetone exhaled through the lungs. An electronic ‘sniffer’ (Neethirajan, Reference Neethirajan2017) sensitive to acetone, in the milking parlor or located in a computer-controlled concentrates self-feeder (see below) may indicate ketosis in cows that exhale acetone. Health problems for which individual early detection sensors were developed, some of which are common practice, will be analyzed to evaluate their practical performance and possible improvements.
Udder health
The best known sensor that was developed to identify a specific health problem is probably the milk conductivity sensor, which is incorporated into the milking machine of many manufacturers, and which was specifically developed for early detection of mastitis. Despite the high hopes, the outcome did not stand up to expectations. The main problem of this sensor is that it measures conductivity of all of the milk that a cow produces, rather than the milk produced by a single quarter, which reduces its effectiveness. This is solved in robotic milking systems, with single cup attachment and detachment, which can measure changes in milk conductivity in each quarter. In any case, the hope that this sensor would enable the detection of subclinical mastitis, even when milk decline was added as an indication, did not fulfill expectations (Shoshani and Berman, Reference Shoshani, Berman, Ipema, Lipus, Metz and Rossing1992), despite the fact that it is a built-in feature in the milking cluster, and therefore widely used. Furthermore, the farmer is often reluctant to apply treatment, which is usually antibiotics, merely on the basis of suspicion, because of the substantial loss of milk that has to be thrown away following treatment.
A significant improvement in udder health is achieved by robot milking, which puts an end to empty milking by using single cluster attachment and detachment thus reducing machine milking pressure time on fore quarters that produce less milk than hind quarters. However, there is even a greater contribution to udder health that is offered by this technology that is not yet pursued. This is milking by milking cups customized to teat size and shape. Glands with acute teat-end lesions have higher rates of infection (Sieber and Farnsworth, Reference Sieber and Farnsworth1981), and such lesions are usually the result of milking. Milking cannot be avoided but a better way to imitate the calf's suckling can be offered by using a milking cup that fits each teat. Today, all teats are milked by the same equipment and routine, despite the fact that the teats differ from one another even on the same udder. In a preliminary study, it was demonstrated that teats of different lengths and hyperkeratoses respond differently to the same milking conditions (Davis et al., Reference Davis, Reinemann and Maltz2002). If the machine picks up a single cup to be attached to a single teat like in the VMS system (DeLaval, Sweden), then potentially it is capable of selecting the one predesigned cup from an array of milking cups that suits this particular teat. The cup would be identified when the cow enters the milking stall by pre-programing. Such an innovation requires research regarding milking cup design, but is certainly within the realm of possibility.
Lameness detection
Dedicated sensors were also developed for lameness detection. One that is based on physical variables and gait movements detected lameness and even the limb that causes it (Rajkondawar et al., Reference Rajkondawar, Liu, Dyer, Neerchal, Tasch, Lefcourt, Erez and Varner2006; Liu et al., Reference Liu, Neerchal, Tasch, Dyer and Ructajkondawar2009). Another lameness detection sensor based on the posture and back curvature of walking cows (Van Hertem et al., Reference Van Hertem, Bahr, Schlageter Tello, Viazzi, Steensels, Romanini, Lokhorst, Maltz, Halachmi and Berckmans2016, Reference Van Hertem, Schlageter Tello, Viazzi, Viazzi, Bahr, Viazzi, Steensels, Romanini, Lokhorst, Maltz, Halachmi and Berckmans2018) indicated lameness before its visual appearance. Both products were put on the market, but did not meet expectations and are not yet widely used. Studies were also performed on early detection of lameness by measuring walking limb pressure on a pressure mattress (Van De Gucht et al., Reference Van De Gucht, Saeys, Van Weyenberg, Lauwers, Mertens, Vandaele, Vangeyte and Van Nuffel2017), but that concept did not reach the stage of practical application. The reason for suboptimal use of the early detection sensors for lameness is the fact that there are many hoof and leg health reasons for lameness. So, when the sensor indicates lameness before visual occurrence, and even the limb that causes it (Liu et al., Reference Liu, Neerchal, Tasch, Dyer and Ructajkondawar2009) it does not indicate the cause. At that stage, the vet, even when lifting the suspected limb and observing it, can't find the reason for the lameness and apply treatment. Moreover, sometimes an indication of early lameness never develops to the pathological stage because of spontaneous recovery. Nonetheless, when several sensors are used for the same target, indications of the real hoof and leg problem are improved (Liu et al., Reference Liu, Neerchal, Tasch, Dyer and Ructajkondawar2009).
Post-calving diseases
One case in which the absence of specific diagnosis does not ruin the prospects for an early detection system is by applying it during the period that the dairy cow is most susceptible to health problems. Such a period is immediately after calving. Usually, when a cow is presented to the vet after calving, even without a specific diagnosis, the vet can identify which of the post-calving health problems is to be treated. Recent studies (Steensels et al., Reference Steensels, Maltz, Bahr, Berckmans, Antler and Halachmi2017a, Reference Steensels, Maltz, Bahr, Berckmans, Antler and Halachmi2017b) indicated that by analyzing continuously generated data from several performance and behavioral sensors after calving, post-calving health problems may be detected before severe damage is caused. This is the case especially when the farmer and the vet know the normal post-calving changes in performance and behavior (Steensels et al., Reference Steensels, Bahr, Berckmans, Halachmi, Antler and Maltz2012). Novel sensors like Afilab (Arazi et al., Reference Arazi, Pinski, Schcolnik, Aizinbud, Katz and Maltz2008), a milk composition analyzer developed jointly by The Volcani Center (ARO, Israel) and SAE Afikim (Kibbutz Afikim, Israel), provide a significant contribution to post-calving diagnosis, particularly in detecting ketosis (Arazi et al., Reference Arazi, Pinski, Schcolnik, Aizinbud, Katz and Maltz2008; Schcolnik and Maltz, Reference Schcolnik and Maltz2013). This capability is in addition to its outstanding contribution to precision feeding (see below).
Possible directions for improvement
The main reason that milk conductivity and lameness detection systems that accurately provide the parameter for which they were developed, i.e. an increase in milk conductivity and early detection of lameness, but still fail to play the prevention role for which they were developed, is that they are not specific. The specific diagnosis is usually done by the vet when the symptoms are clear: a sharp drop in milk production or visible lameness. Unfortunately, this happens after the damage, both financial and to the cow's wellbeing, was already done. Does this mean that we have to give up the hope of early detection? Fortunately, no. Two developments have the potential to indicate specific health problems early. The first one is to incorporate as many sensors as possible into one indication system, that minimizes the false positive indications of symptoms. Thus, only those cows that are really on the verge of health problems are presented to the vet. The attitude of a vet to a case of a cow presented to him because ‘something might be wrong with her’ will be different when he knows that several different sources indicate that something is wrong with her, and not just the data from one sensor that might be a false positive. For example, a gradual but continuous decline in MY and BW accompanied by behavioral changes like the number or duration of visits to feeding trough or self-feeders; an increase in body temperature, even without an increase in milk conductivity, are strong enough indications of a developing health problem to be taken seriously by the vet, even without a specific diagnosis. This approach was tested for lameness detection (Van Hertem et al., Reference Van Hertem, Bahr, Schlageter Tello, Viazzi, Steensels, Romanini, Lokhorst, Maltz, Halachmi and Berckmans2016) and post-calving health problems (Steensels et al., Reference Steensels, Maltz, Bahr, Berckmans, Antler and Halachmi2017a, Reference Steensels, Maltz, Bahr, Berckmans, Antler and Halachmi2017b see below). It was found that it minimized false positives and could be effective for health management. Unfortunately, integrating the data for useful information is still in the hands of the farmer. Manufacturers should do this with their own sensors until the day when, hopefully, they will start cooperating with one another to connect sensors for an IDCM purpose.
The second possibility is the development of specific biosensors. As described by Neethirajan et al. (Reference Neethirajan, Tuteja, Huang and Kelton2017) and Meshram et al. (Reference Meshram, Agrawal, Adil, Ranvir and Sande2018), ‘The potential for biosensor technology is enormous and is likely to revolutionize analysis and control of biological systems.’ It is only a question of time before biosensors for specific diagnoses will be incorporated into milk meters adjusted to take minute samples from the milk flow to detect specific health problems.
Reproduction
A delay in getting a cow pregnant is very costly. As a result, this issue attracted scientists, extension experts and farmers interested in improving reproduction management. The reproductive process goes through several physiological and management stages: estrus detection, insemination and conception and finally pregnancy and calving. Each of these stages carries potential risks that might disrupt the whole process.
Estrus detection
As described above, estrus detection is a good example of the successful application of an IDCM technology, and one that is constantly improving. The history of estrus detection by means other than visual observation of ‘standing heat’ goes from paint marks on the back of the cow ready for insemination (Foote, Reference Foote1975), through intravaginal and milk temperature sensors (Fordham et al., Reference Fordham, Rowlinson and McCarthy1988) to implanted tissue conductivity electrodes (Lehrer et al., Reference Lehrer, Lewis and Aizinbud1992). The system that has prevailed is the one that takes advantage of behavioral changes of the cow during the reproduction cycle. Restlessness of a cow during the estrus part of the cycle is expressed by change in activity, that can be measured by a variety of commercial off-the-shelf sensors (Mayo et al., Reference Mayo, Silvia, Ray, Jones, Stone, Tsai, Clark, Bewley and Heersche2019). Reduced food consumption followed by reduced measurable rumination (see below) may also contribute to detection, especially in cases of ‘silent estrus’ (Bar, Reference Bar2010; Bar and Solomon, Reference Bar and Solomon2010; Reith and Hoy, Reference Reith and Hoy2012). These estrus detection systems are so successful, that they are now used in farms all over the world. However, the aim of estrus detection is to get a cow pregnant, but the most accurate estrus detection system does not secure conception, even when the insemination time was optimal.
Insemination and conception
The cow has to be inseminated when she is still receptive, but the farmer gets the information from the sensor post factum, when it is downloaded from the activity tag, usually at milking time. Fortunately, changes in behavior occur ahead of the optimal time for the cow to conceive (Maatje et al., Reference Maatje, Loeffler and Engel1997), which allows the farmer to adopt an optimal insemination routine related to estrus detection time in accordance with the sensor's indication and the service availability. However, even when inseminated at the optimum time, not all cows get pregnant. The primary reason for this is that the cow's metabolic status when she starts the cycle after calving is a negative energy balance, which prevents conception (Butler and Smith, Reference Butler and Smith1989). Assessing exactly when the cow emerges from a negative energy balance is difficult. Farmers forestall a poor conception rate by delaying insemination time, sometimes as much as 100 d after calving, despite the fact that they would like the cows to get pregnant earlier. Sensors are available that enable quite accurate pinpointing of every cow's emergence from a negative post calving energy balance. A study in Israel was performed using MY and BW changes at estrus to indicate emergence from negative energy balance, to improve insemination time and obtain earlier pregnancies (Maltz, Reference Maltz2010; Mahmoud et al., Reference Mahmoud, Bogdahn, Alsaaod, Hüsler, Starke, Steiner and Hirsbrunner2017). The criteria that were selected were:
• MY decreasing or increasing by 0.5 kg/d or less, an indication of being just past or reaching peak production
• BW increasing by 0.1% or more over its postcalving weight, calculated for 3 d running average for 7 d prior to estrus detection
This is illustrated in Fig. 1. In the dairy that this study was performed the routine was to inseminate only after estrus detection which occurred 85 and 65 d after calving for heifers and cows respectively, and the conception rate was 35% (out of 84 inseminations) and 20% (out of 137 inseminations) for heifers and cows respectively. By contrast, when BW and MY data were used to assess the point at which the cows emerged from post-calving negative energy balance, 42% of the heifers that showed estrus between 57–85 d after calving and 37% of the cows that showed estrus between 47–65 d after calving (out of 55 and 54 inseminations for heifers and cows respectively) conceived.
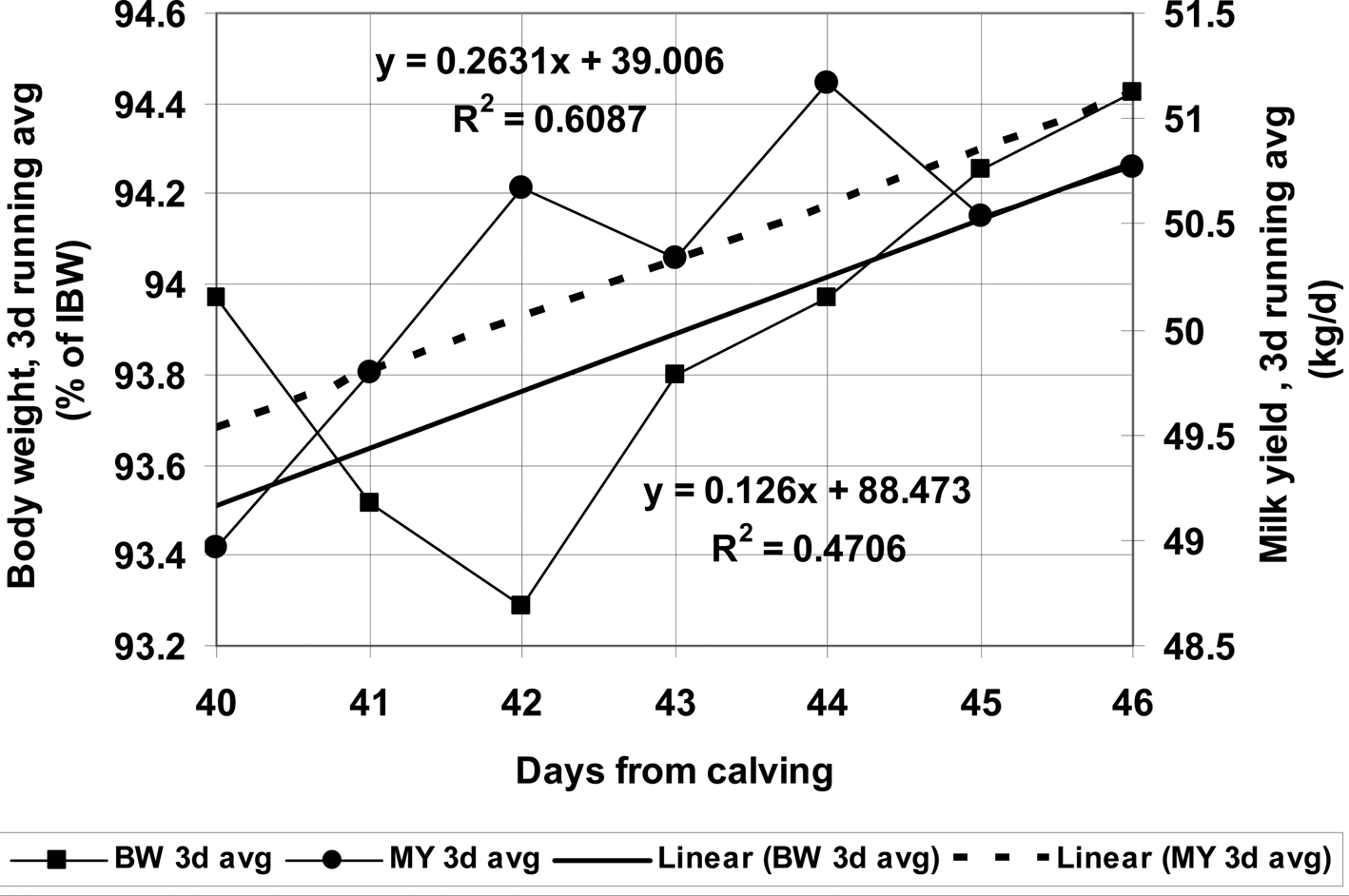
Fig. 1. Successful conception following insemination decision for one cow showed estrus 46 d after calving. Daily averages of MY were calculated for 3 d running, as was daily body weight as a percentage of post-calving initial body weight (IBW). In the last 7 d prior to estrus detection, the MY of this cow increased by only 0.2631 and BW increased by 0.126% of IBW. From (Maltz, 2010).
Conception probability index
In farms that possess estrus detection, MY and BW sensors, it is not too difficult to incorporate a ‘conception probability index’ into the existing herd management software. Together with the estrus detection system, based on MY and BW, the index would indicate the probability of the cow's ability to conceive following detection of this particular estrus. Intensive study regarding the correlation between the conception rate and the BW nadir after calving, the MY and BW curves, and behavior following the BW nadir, may contribute to improvement in in the first insemination conception rate (Kaim et al., Reference Kaim, Nativ, Zuckerman, Antler and Maltz2010; Maltz, Reference Maltz2010). In addition, post calving health problems like retained placenta or metritis, that have a strong effect on conception rate can be incorporated into the index. This approach can improve the conception rate of any reproduction strategy including hormonal synchronization. Only the cows that have a proper ‘conception probability index’ would be synchronized. It will be beneficial to the industry if manufacturers that market these sensors and herd management programs will join with scientist and extension service to produce this index.
Pregnancy and calving
We do not have direct measurements to indicate embryo absorption (other than the cow returns to cyclicity), natural abortion or still birth, but there are several sensors that indicate approaching calving. Close observation of cattle in the late gestation period is essential to detect the onset of calving and, thereby, reduce neonatal losses (Jensen, Reference Jensen2012). The process of calving entails two stages and each has practical and managerial significance. The first is when the cow starts to change its behavior; and the second, when the physiological process of calving actually starts and the cow is in labor. Naturally, the second stage is more attractive for researchers to develop sensors to alert the farmer that the cow is in labor (Mahmoud et al., Reference Mahmoud, Bogdahn, Alsaaod, Hüsler, Starke, Steiner and Hirsbrunner2017), so little attention has been given to the first stage. It was only recently that it became clear that a cow changes her behavior well ahead of actual calving; these changes are gradual but can accurately indicate that the cow is about to calve (Maltz et al., Reference Maltz, Medini, Bercovitch, Parmet, Halachmi, Antler, Edan, Lokhorst and Berckmans2011; Jensen, Reference Jensen2012). Lying time and frequency of lying bouts, number of steps and neck movements are relevant sensor data, but the fact that the feeding behavior changes too, resulting in reduced rumination time (Bar, Reference Bar2010; Fadula et al., Reference Fadula, Bogdahna, Alsaaoda, Huslerb, Starkec, Steinera and Hirsbrunner2017), reinforces the predictive capability for approaching calving. In a study conducted at the Volcani Center, it was found that when using computer-controlled self-feeders (CCSF) for allocating concentrates in the period of preparation, i.e. about a month before calving, the change in cow behavior can also indicate impending calving. The dry cow receives a low density ration and is very eager to consume concentrates that are provided by the CCSF, therefore, they visit the feeder during every feeding window. If they miss a visit, it is usually when calving is about to start. An alert sent to the farmer when a cow misses a feeding window could potentially be a good indicator of approaching calving.
Nutrition
In the 1990s, an attempt was made by a commercial manufacturer (Lely, The Netherlands) to develop an individual feeding technology for forage. Nonetheless, up until now, individual feeding includes a basic mixture (that may or may not contain concentrates) and supplemental concentrates using CCSF. Here, individual feeding will be described and discussed primarily from the point of view of individual concentrates supplementation (ICS) by CCSF, since this technology is commercially implemented in both conventional and robot milking dairy farms, and its characteristics indicate that it will become the dominant individual feeding technology in the dairy industry. The largest expense in a dairy farm is food and the most expensive ingredient in the food is grain, the price of which keeps increasing. Accordingly, the first individual feeding technology was developed to feed concentrates individually according to the farmers decision. The technology (produced by De Laval, Sweden) included a neck transmitter that was manually charged in the milking parlor, to set the concentrates ration for each cow. When a cow presented herself to a concentrates self-feeder, the transmitter operated an electrical motor, and concentrates were released to the cow based on the pre-set charge. The technology was designed to correlate the amount of the expensive ingredient, the concentrates, fed to the cow according to her production. When individual ID was developed and computer use was adopted by the industry, this approach was accelerated, and most of the process was controlled by a computer, with the exception of several components, enumerated below. The technology enabled the farmer to control the individual concentrates rationing from his office. This technology was very promising. Studies were conducted in the early 1980s to find the best way to adapt this strategy of correlating concentrates supplementation to performance and the economic goals of the particular dairy farm (Cassel et al., Reference Cassel, Merrill, Bul, Milligan and Guest1982; Maltz et al., Reference Maltz, Kroll, Sagi, Devir, Spahr and Genizi1991, Reference Maltz, Devir, Kroll, Zur, Spahr and Shanks1992a, Reference Maltz, Grinspan, Edan, Antler, Kroll, Spahr, Ipema, Lipus, Metz and Rossing1992b). Despite this, almost 40 years later, the most popular feeding strategy applied in medium and large dairy farms ignores this technology, and uses group feeding of a total mixed ration (TMR), and mostly a flat rate TMR feeding, throughout the lactation cycle. To some extent, this strategy adopted aspects of individual feeding management in dairy farms and research settings, where the cows could be grouped according to time post calving (Maltz et al., Reference Maltz, Devir, Kroll, Zur, Spahr and Shanks1992a; Grinspan et al., Reference Grinspan, Kahn, Maltz and Edan1994) and/or production potential, or body weight changes (Spahr et al., Reference Spahr, Shanks, McCoy, Maltz and Kroll1993; Gaillard et al., Reference Gaillard, Friggens, Taghipoor, Weisbjerg, Lehmann and Sehested2016), and cows could be transferred between feeding groups according to changes in performance (Spahr et al., Reference Spahr, Shanks, McCoy, Maltz and Kroll1993; Gaillard et al., Reference Gaillard, Friggens, Taghipoor, Weisbjerg, Lehmann and Sehested2016) or time after calving (Kroll et al., Reference Kroll, Owen and Whitaker1987). Then, each group could be fed a TMR that was adjusted to the group's average production characteristics.
To evaluate the reasons why ICS did not achieve significant market penetration, or at least become a significant applicable strategy in the dairy industry, we should assess the advantages and disadvantages of the TMR group feeding, including its individual feeding aspects, in comparison to an individual feeding strategy via CCSF. The biggest advantage of the group feeding is the convenience and reliability of the on-site ration preparation; the hardware consists of a weighing mixer wagon and portable computers; the software entails a ration preparation program. Even easier, the ration can be received from a food center that can serve one or several dairy farms based on the average group variables: the number of cows, performance, characteristics of the feeding group, etc. Once this is done, the feeding is a worry free process. In addition, numerous research papers based on this strategy studied various components that could be incorporated into the ration, in order to improve performance or make it more efficient (Miron et al., Reference Miron, Yosef, Maltz and Halachmi2003, Reference Miron, Yosef, Nikbachat, Zenou, Maltz, Halachmi and Ben-Ghedalia2004, and see references in Van Empel et al., Reference van Empel, Makkar, Dijkstra and Lund2016). The principal disadvantage is that all ration preparation calculations for a group of cows or for the entire herd are made for the ‘average cow’. The performance parameters used for the calculation are: average food consumption, average milk production, average milk composition, average BW, average body condition. One can argue that there is no such thing as an ‘average cow’ because of individual variations in production efficiency (Ben Meir et al., Reference Ben Meir, Nikbachat, Fortnik, Jacoby, Levit, Adin, Cohen Zinder, Shabtay, Gershon, Zachut, Mabjeesh and Halachmi2018, Reference Ben Meir, Nikbachat, Jacoby, Portnik, Levit, Kleinjan Elazary, Gershon, Adin, Cohen Zinder, Shabtay, Zachut, Mabjeesh, Halachmi and Miron2019). In terms of ration density, under this feeding regime, around half of the cows are overfed and half of them are underfed. Nonetheless, it can be said that a TMR feeding strategy covering all nutritional demand is convenient, reliable and gives the farmer peace of mind. Even small dairy farms that do not have the convenience of an on-site food center that were considered to be excellent candidates for introducing ICS by CCSF do not use this technology because of all the problems entailed in operating them. The fact that even when applying ICS by CCSF a basic mixture is still fed to the cows via a common feeding trough, with operations similar to TMR feeding also does not encourage the use of CCSF. However, several new factors emerged that led to a re-examination of individual feeding. Firstly, the price of concentrates keep rising, making efficient use more desirable. Secondly, robotic milking typically requires a feeding inducement to entice the cow to visit the robot, and that is usually concentrate. Thirdly, the value of individual nutrition is now recognized to include decreased environmental impact as well as increased production efficiency. Individual feeding is built on the fundamentals of IDCM described above: sensors, computer, physiological model, decision-making based on the model and decisions execution by the CCSF. Unfortunately, problems and obstacles exist in each of these components, and despite substantial efforts, individual feeding did not take its expected role in the dairy industry.
Individual feeding has two goals. The first is to provide the dairy cow the precise ration that she requires according to her physiological condition and performance. The second derives from the first, which is to prevent the waste of concentrates on cows that do not qualify for a ration of the same energy density or protein concentration that is required by high-yielding cows or by the ‘average cow’. It was clearly demonstrated that when individual feeding is established and feeding is done according to energy balance, these two goals are achieved and production efficiency increases substantially (Maltz et al., Reference Maltz, Barbosa, Bueno, Scagion, Kaniyamattam, Greco, De Vries and Santos2013).
Sensors
Individual feeding is sensor dependent. As noted above, the first attempts to use sensors were in correlating supplementation concentrates to milk production, since this was the only performance measurement available from a sensor (Cassel et al., Reference Cassel, Merrill, Bul, Milligan and Guest1982; Maltz et al., Reference Maltz, Kroll, Sagi, Devir, Spahr and Genizi1991). Quite soon, it was recognized that more performance variables are required to build a satisfactory physiological model on which decisions concerning supplementation entailing the energy provided by rations and the density of concentrates and protein could be established. When individual on-line BW measurement became possible it was incorporated into the system, bringing some improvement (Maltz et al., Reference Maltz, Devir, Kroll, Zur, Spahr and Shanks1992a, Reference Maltz, Grinspan, Edan, Antler, Kroll, Spahr, Ipema, Lipus, Metz and Rossing1992b; Bossen and Weisbjerg, Reference Bossen and Weisbjerg2009; Bossen et al., Reference Bossen, Weisbjerg, Munksgaard and Højsgaard2009; Gaillard et al., Reference Gaillard, Friggens, Taghipoor, Weisbjerg, Lehmann and Sehested2016). The breakthrough came with the individual milk composition analyzer (Afilab, Israel), that enabled milk fat and protein composition to be incorporated into the physiological model for accurate rationing of concentrates, especially during periods of transition (Maltz et al., Reference Maltz, Livshin, Antler, Brukental and Arieli2004, Reference Maltz, Antler, Halachmi, Schmilovitch, Lokhorst and Groot Koerkamp2009). When individual food intake was measured manually, decisions could be made regarding the ration density based on a substantial energy balance maintaining protein on a constant level. The results were outstanding. Physiological status improved and production increased with no increase in concentrates rationing compared to a flat rate TMR feeding group (Maltz et al., Reference Maltz, Barbosa, Bueno, Scagion, Kaniyamattam, Greco, De Vries and Santos2013). We can conclude that the sensors necessary for optimal concentrates rationing are: MY, milk composition, BW, body condition (Bercovich et al., Reference Bercovich, Edan, Alchanatis, Moallem, Parmet, Honig, Maltz, Antler and Halachmi2013) and food intake. Then, the model formulated by Maltz et al. (Reference Maltz, Barbosa, Bueno, Scagion, Kaniyamattam, Greco, De Vries and Santos2013) can be used for rationing concentrates using the body condition sensor (De Laval, Sweden) to provide additional information, especially during the late lactation period (Bercovich et al., Reference Bercovich, Edan, Alchanatis, Moallem, Parmet, Honig, Maltz, Antler and Halachmi2013). In addition, the rumination sensor (SCR, Israel) and rumen pH sensor (SmaXtec®, Austria) can provide supporting information to establish sound physiological models (Duffield et al., Reference Duffield, Plaizier, Fairfield, Bagg, Vessie, Dick, Wilson, Aramini and McBride2004; Phillips et al., Reference Phillips, Mottram, Poppi, Mayer and McGowan2009; Mottram et al., Reference Mottram, Lowe, McGowan and Phillips2008). All the sensors except that for measuring food intake are commercially available. Food intake is crucial to calculating individual energy balance, and we will consider this next.
Individual food intake
Attempts are being made to develop a sensor to measure individual daily food intake (Bloch et al., Reference Bloch, Levit and Halachmi2019), but so far it is limited to costly research facilities all over the world. For example, such research is being conducted in the Volcani Center in Israel (Halachmi et al., Reference Halachmi, Edan, Maltz, Peiper, Moallem and Brukental1998) and in research dairy farms in universities, like the University of Guelph in Canada and University of Florida. In such facilities, individual food intake can be measured for cows kept in a group in conditions that are close to commercial (i.e. not restricted to tied stalls). Though not practical for commercial dairy farms, the results of research in such facilities together with available sensors that measure individual performance, can provide all that is needed to design physiological models to evaluate individual daily food intake based on performance and other individual information, like time after calving and reproduction status. The first attempt to model individual daily DMI with only performance variables was published in 1997 (Halachmi et al., Reference Halachmi, Maltz, Metz and Devir1997) using individual daily BW and MY. The model was improved following a model published by the NRC in 2001 (NRC, 2001), which added a measurement of milk fat (Halachmi et al., Reference Halachmi, Maltz, Metz and Devir1997). The availability of cow location sensors (CowView® GEA, Germany) led to an attempt to incorporate time spent in the feeding trough into the daily DMI model. Improvement to the model was achieved, but to a lesser extent than expected (Halachmi et al., 2016). Quite possibly, further improvements in modeling individual daily DMI using performance and behavioral variables can be achieved, if the data goes through a smoothing process first. It is common knowledge that MY and BW fluctuate daily. Now it is also recognized that food intake fluctuates as well (Figs. 2a and b).
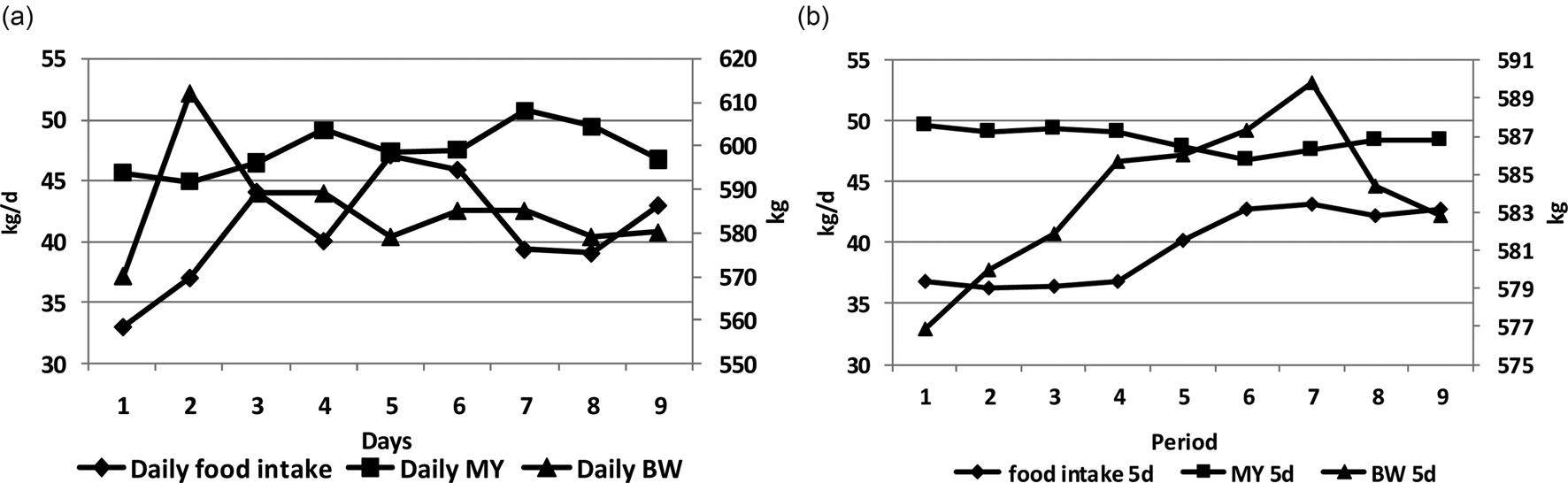
Fig. 2. Daily food intake, MY and BW of a cow kept in a group of 42 cows. Individual measurements were made in the barn at the Volcani Center (Halachmi et al., Reference Halachmi, Edan, Maltz, Peiper, Moallem and Brukental1998) during 14 successive days at mid lactation. (a) Describes the daily measured values and (b) describes the running averages of these same data over periods of 5 d (days 1–5, 2–6 and so on).
The daily fluctuations in different performance variables do not necessarily correlate well, as can be seen in Fig. 2a, however, better correlations emerge when the data are smoothed by the average of five running days (Fig. 2b). The number of days required for a usable running average will be different during the transition period when daily performance variables change more markedly (Maltz et al., Reference Maltz, Devir, Kroll, Zur, Spahr and Shanks1992a) than from peak production onward, when changes are more moderate (Fig. 1). A further improvement of the DMI model may come from replacing milk fat by daily milk energy, calculated from MY and milk composition. As shown in Fig. 3, daily running averages over 5 d of milk energy correlates significantly with the running daily average of food intake over 5 d. Hence, milk energy measured on line, which makes rationing concentrates according to energy balance possible (Maltz et al., Reference Maltz, Barbosa, Bueno, Scagion, Kaniyamattam, Greco, De Vries and Santos2013), can also improve modeling individual food intake. However, even the best modeling results cannot predict food intake of the marginal cows on both sides of production efficiency scale (Ben Meir et al., Reference Ben Meir, Nikbachat, Fortnik, Jacoby, Levit, Adin, Cohen Zinder, Shabtay, Gershon, Zachut, Mabjeesh and Halachmi2018, Reference Ben Meir, Nikbachat, Jacoby, Portnik, Levit, Kleinjan Elazary, Gershon, Adin, Cohen Zinder, Shabtay, Zachut, Mabjeesh, Halachmi and Miron2019). Therefore, a feedback system has to be incorporated into the concentrates rationing process, which would evaluate the actual results of the rationing decisions compared to those expected. For example, a decision to increase ration density by increasing daily concentrate supplements, because of a measurement of negative energy balance, (see below) should be followed by close observation to determine if the outcome is not an undesired increase in BW or BC, while MY is not affected or vice versa.
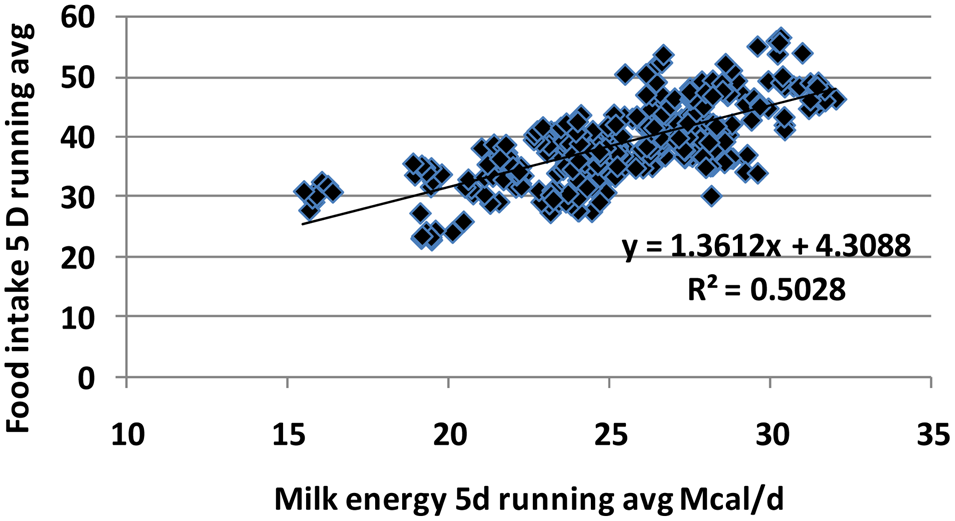
Fig. 3. Correlation between daily milk energy and food intake (as is) of a TMR, according to NRC (2001) based on a running average of 5 d. Individual measurements done on 42 cows mid lactation, over a period of 14 d in the individual measurements barn at the Volcani Center (Halachmi et al., Reference Halachmi, Edan, Maltz, Peiper, Moallem and Brukental1998). Each point represents 1 d of one cow.
ICS can be applied in many ways, and a variety of interpretations can be made regarding the variable or variables to which ICS is related. In some studies it is applied according to MY, BW and estimated food intake (Cassel et al., Reference Cassel, Merrill, Bul, Milligan and Guest1982; Maltz et al., Reference Maltz, Kroll, Sagi, Devir, Spahr and Genizi1991, Reference Maltz, Devir, Kroll, Zur, Spahr and Shanks1992a, Reference Maltz, Grinspan, Edan, Antler, Kroll, Spahr, Ipema, Lipus, Metz and Rossing1992b; Spahr et al., Reference Spahr, Shanks, McCoy, Maltz and Kroll1993; Bossen and Weisbjerg, Reference Bossen and Weisbjerg2009; Bossen et al., Reference Bossen, Weisbjerg, Munksgaard and Højsgaard2009) or as a percentage of the basic measured mixture consumed (Henriksen et al., Reference Henriksen, Weisbjerg, Løvendahl, Kristensen and Munksgaard2018). Neither stage of lactation nor reproduction status have been included in these ICS decision-making processes. These are physiological conditions that significantly affect energy balance especially in transition time and, in the case of reproductive status, late lactation. Incorporating the physiological status into the ICS decision making process as was done in TMR precision feeding (Maltz et al., Reference Maltz, Barbosa, Bueno, Scagion, Kaniyamattam, Greco, De Vries and Santos2013) may improve its performance. Any ICS strategy is affected by the management conditions under which the dairy is operating. For example, the ICS strategy might be different under grazing (especially range grazing as in Australia and New Zealand) vs. intensive or semi intensive conditions (see review by John et al., Reference John, Clark, Freeman, Kerrisk, Garcia and Halachmi2016 and Halachmi et al., Reference Halachmi, Ben Meir, Miron and Maltz2016). Interpretation of the data also differs according to system. In either conventional or robot milking conditions, ICS strategy needs to answer the question, ‘are all the ration concentrates supplemented individually through CCSF?’ This, in turn, raises the question, ‘how much concentrate remains in the basic mixture?’ The composition and quality of forages as well as of concentrates is also a factor in ICS strategy. Availability of byproducts that maintain the quality of concentrates, such as cotton seeds, citrus pulp or vegetable waste (Maltz et al., Reference Maltz, Kroll, Sagi, Devir, Spahr and Genizi1991, Reference Maltz, Barbosa, Bueno, Scagion, Kaniyamattam, Greco, De Vries and Santos2013; Miron et al., Reference Miron, Yosef, Nikbachat, Zenou, Maltz, Halachmi and Ben-Ghedalia2004) are all parameters that have to be considered when applying an ICS strategy by CCSF. Typically this is done without including environmental conditions that may affect the cow's use of CCSF, but it is important to recognize that each of the conditions listed above may affect the outcome of one ICS strategy or another. It is quite possible that every dairy will have to fine tune the ICS strategy selected to its own specific conditions. To summarize, all the variables that are affected by ration energy and concentrates density have to be incorporated into the physiological model that dictates concentrates supplementation. These include:
• Milk yield
• Body weight
• Milk composition (fat, protein, lactose)
• Magnitude of MY increase post calving, and MY and MY changes after peak production.
• Magnitude of BW decrease post calving, and BW and BW changes after peak production.
• BC and BC changes during the lactation cycle.
• Food intake
• Energy balance
For all of these variables (except food intake) sensors exist, or the variable can be calculated from the variables measured, e.g. energy balance. Individual food intake has to be evaluated out of the measured variables as described above. The strategy that has the greatest potential to become the dominant strategy after all the practical problems are solved (see below), is the one that will use measured energy balance (Maltz et al., Reference Maltz, Barbosa, Bueno, Scagion, Kaniyamattam, Greco, De Vries and Santos2013), using calculated individual food intake until a proper sensor is developed. Regardless of which ICS strategy is employed under any management systems, some basic practical conditions must be fulfilled. These are mainly related to the CCSF.
Computer controlled self-feeders
The device on which the whole of ICS is based is the CCSF. Therefore, second only to the ICS strategy adopted, the proper operation and cow use of the CCSF is crucial in exploiting the benefits of individual feeding. Several conditions must be met for successful operation of the CCSF, as follows.
Firstly, every cow in the group must have enough feeding-stall time to consume the amount of concentrates rationed to her. Therefore, the ICS strategy has to correlate with the maximum amount of time that a cow is expected to spend in the CCSF. The maximum rate that can be consumed by a cow at the rate it is allowed by the machine, without leaving left overs behind when the cow, for any reason, leaves the stall, is around 300 g/min (Kroll et al., Reference Kroll, Maltz and Spahr1988) of pelleted grain. Crushed grain is not recommended because of its powdery consistency. A cow that qualifies for 10 kg/d needs 33.3 min in the stall, plus about 30 s to enter and back out every time she feeds. If the maximum allowance of one feed is, say, 3 kg, then this cow will need approximately 35 min in the stall diurnally. In calculating the number of CCSFs needed to feed a group of cows comfortably, one has to take into consideration the fact that during the night, the CCSF are visited less frequently than they are during the day. Under robot milking conditions with no external (out of the milking stall) CCSF, the concentrates consumption time has to correlate with milking time, which itself must correlate with MY and milking frequency. In any case, if ICS is expected to work, the ratio of concentrates allowance in a group of cows has to correlate to the number of CCSF available. These calculations are particularly significant, because cows that do not qualify for high energy density rations thrive on the leftovers from cows that are butted out of the feeding stall or forced out of the robot milking stall before consuming their allowance. This is a bit more complicated when dual-channel CCSF that can ration two components of concentrates are employed to individually feed energy and protein to the cows (Maltz et al., Reference Maltz, Livshin, Antler, Brukental and Arieli2004, Reference Maltz, Antler, Halachmi, Schmilovitch, Lokhorst and Groot Koerkamp2009). The rate of feeding the cow with the two components together should not exceed 300 g/min.
Next, all cows in the group need to be rationed a significant amount of concentrates. Cow behavior has to be considered in any ICS strategy, especially in conventional dairy farms. The physiological model of ICS strategy when applied to a group of cows should be designed in such a way that it allows all cows to use the CCSF and consume a substantial quantity of concentrates. Cows that are not given supplements (especially those that are familiar with self-feeders) tend to roam around the self-feeders, disturbing other cows and preventing them from entering the feeding stalls, as well as butting cows that are using them.
Furthermore, the ICS needs to be allocated so that it is consumed evenly throughout the day, and the number of ‘feeding windows’ as well as the amount fed in each of them should be planned accordingly. Rationing must be planned so that every cow receives at least 1 kg of concentrates at every feeding window.
In addition, attention must be paid to calibration. Most CCSF systemsdisperse the concentrates by volume, hence, calibration is required to translate the volume to weight. This has to be done frequently, especially when the storage containers are refilled or the type of concentrates is changed. Unfortunately, CCSF calibration is complicated, difficult and time consuming, but it must be done in order to make individual feeding work. In time, it is hoped that solutions will be developed so that CCSF will disperse the feed and concentrates by weight or a more friendly calibration routine will be provided.
Another factor is that food that is palatable and attractive to the cows must always be available in the common feeding trough, since an empty trough or unpalatable food will always cause unnecessary pressure on the self-feeders, disturbing the feeding behavior routine.
In terms of layout, in a no-stall compost bedded barn, the self-feeders surrounding should be concrete and not far from the common feeding trough.
Finally, placement of the CCSF should take into consideration environmental conditions, to avoid direct sunlight and heat in the summer. Fulfilling all of these conditions will ensure that the fundamentals for successful ICS implementation have been met, and each cow consumes her ICS ration.
Individual electrolytes feeding
Several studies have indicated that providing sodium, potassium and chloride as a fixed proportion of the ration may not be adequate at transition times. This happens especially with sodium and potassium (Shalit et al., Reference Shalit, Maltz, Silanikove and Berman1991; Silanikove et al., Reference Silanikove, Maltz, Halevi and Shinder1996; Silanikove et al., Reference Silanikove, Maltz, Shinder, Bogin, Bastholm, Christensen and Norggarrd1998). This might happen because of incompatibility between the relative increases in MY and food intake: MY running ahead of intake might drain electrolytes from the cow, which is not compensated by the more gradual increase in food intake containing a fixed proportion of these electrolytes. A study in which the cows received these electrolytes in proportion to the increased food intake was found to be superior to providing the electrolytes as a fixed proportion of the cows' food (Silanikove et al., Reference Silanikove, Maltz, Shinder, Bogin, Bastholm, Christensen and Norggarrd1998). CCSF are capable of providing several different components, and further technological refinements to provide minute components like electrolytes, minerals and vitamins may advance individual feeding.
Welfare
The fact that ‘an animal manifests by its behaviour her wellbeing and physiological status’ (Hurnik, Reference Hurnik, Ipema, Lipus, Metz and Rossing1992) requires the farmers eye and interpretation ability to maximize wellbeing. Today, this is supported by a battery of sensors that can virtually surround the herd and individuals within it. We are able to monitor activity in the barn, characterize temperament and restlessness as well as behavioral and physiological responses to milking and milkers. Unfortunately, most of this information is not yet properly utilized to improve dairy cow wellbeing.
For our discussion, aspects of the cow's wellbeing can be divided into two categories: those related to the animal's environment, i.e. the barn and its facilities, and those related to the milking process, including both conventional or robotic milking. In the conventional milking process, the cow's wellbeing depends very much on her interaction with humans, and it is this interaction that provides the prime example of unused but potentially useful data. The major amount of data that can be obtained at milking includes milking cohort variables as well as individual cow data. For milking cohort data:
• The arrangement time in the milking parlor – first and last ID time in the milking cohort
• Time between successive milking cohorts – last cluster detachment time, first attachment time
• Time group spends in the parlor – first ID time, group release time
For individual data
• Waiting time in the milking line before milking – time between ID and attachment.
• Milking time – time between attachment and detachment
• Milk let down readiness (oxytocin release) – time between cluster attachment and continuous milk flow
• Amount milked at any time interval
• Milk flow rate at any time interval during milking
These factors relate to physiological variables that are of great significance to the cow's wellbeing. As a simple example, milking time and milk flow will vary depending on whether the cows are milked by a familiar milker or by someone they do not know (Table 1). It is to be hoped that more use will be made of such data in the future.
Table 1. Milking parameters of two successive morning milkings

The first day, morning milking was performed by the dairy's regular experienced staff, and the second by substitute milkers.
All differences between experienced and inexperienced workers are significant: P < 0.001.
There is considerable debate regarding the wellbeing consequences of the cow's interactions with her environment. Many take the view that allowing maximum freedom of movement (‘free range’) and choice is paramount but, on the other hand, an optimal housed environment removes the vagaries and stresses of harsh environmental conditions. It is quite possible to argue that the well-known Five Freedoms of good welfare (Webster, Reference Webster2001) can best be achieved in a fully roofed, dry manure, bedded, no-stall barn, with milking done by robots. These conditions provide two crucial elements that contribute to dairy cows' life quality. First, there are no stalls to hamper lying behavior and second, there is relatively little human interaction. Space does not allow for a full discussion of the many ways in which individual cow management might contribute to improved wellbeing in the future, so instead a specific example of what is possible will now be given.
Free stalls
Under fully roofed, no-stall conditions, dairy cows enjoy significant longer lying times than do cows in a free-stall barn under the same environmental and climatic conditions (Livshin et al., Reference Livshin, Antler, Zion, Stojanovski, Bunevski, Maltz and Cox2005). This occurs when the free stalls are properly maintained. Nevertheless, most of the dairy barns in the U.S. and Europe are designed as free stall system. The advantages of this system are:
• Lying space is provided for every cow in the barn with a minimal roof area, enabling better control of environmental conditions
• Every cow in the group can rest in such a way that the stalls remain relatively free of feces and urine, which can easily be collected and removed from the barn
Unfortunately, stalls are often designed for moderately sized and small cows, to minimize the labor necessary for stall cleaning, and to economize on stall bedding material. This, by definition, means that the stall will be uncomfortable for larger cows. The conflicting needs of having sufficient lying space for all cows to be comfortable, on the one hand, and prevent the accumulation of excreta in the stalls, on the other, can be achieved by installing adjustable stalls, the length of which automatically adjusts when a larger-framed cow approaches it. The stall then automatically returns to its original size when the cow leaves it. The length will be adjusted by means of a movable bar or fence at the end of the stall with a hydraulic, pneumatic or similar mechanism to adjust it. The opposite stall will be insignificantly shorter, and still suitable for medium sized and short cows. About 20% more adjustable stalls are required than the number of cows in the group that require stalls longer than the standard size. The required facilities are:
• An ID system connected to a camera on the milking parlor outlet path, with suitable software to ‘measure’ the cow size
• An ID system connected to the adjustable stalls so that every cow that presents herself is identified, and the system decides whether the stall's length should be changed
• Software in the herd management system that combines all three elements: length measurement connected to ID, length adjustable free stalls connected to the ID system and decision-making software
Conclusions
Achievements and obstacles
The principal achievements of IDCM include additional individual sensors and intensified research in the field. The principal disappointment is the painfully slow dissemination of IDCM. Among the achievements are:
• Automatic estrus detection.
• Availability of a variety of sensors that allow the generation of more data and details about function and behavior of each individual cow, which is particularly applicable when there is concern about a specific cow.
The reasons for the low dissemination of IDCM were summarized by Bewley and Russell (Reference Bewley and Russell2010) and van Empel et al. (Reference van Empel, Makkar, Dijkstra and Lund2016) and are outlined here with practical examples:
• Technologies promoted as user-friendly are actually not easy to use and costly. In this respect we can include regular maintenance and calibration needs, as described for CCSF and walk through scales
• Technologies and concepts that are not fully developed and go to market too quickly. The term ‘too quickly’ might be misleading. The sensor performs satisfactorily, but there are no instructions regarding how to translate the data into useable information, what to do with this information, or how to incorporate it with the data obtained from other sensors. Moreover, it is marketed without guidance on the variety of management aspects that can be improved.
• Technologies are developed without considering integration with other technologies or the farmer's routine work patterns. This problem is one of the most disturbing. Perhaps manufacturers will reach a point where they work together to integrate technologies that each produce, although currently this seems a long way off.
• Scarcity of large-scale field trials and demonstrations for commercial purposes. This is a crucial step toward dissemination. However, such field trials are costly, and the outcome is not guaranteed. Therefore, such trials should be a joint effort of the manufacturer and research institutes. Scientists should study the implementation of new technologies, and extension services should teach farmers about the advantages and disadvantages of IDCM technologies and how to use them.
• Technology is marketed without adequate interpretation of the biological significance of the data. This, too, is currently the task of researchers.
• Information is provided with no clear plan of action. Of all the problems listed, this one is probably the most significant. A dairy farmer's most valuable resource is time. A farmer may get and use aggregated or averaged data on groups of cows, but is usually unable to analyze data on each individual cow, even if he only has 50 cows, and certainly can't if his herd is larger than that.
If IDCM is to become a widespread practice, it must have clear instructions for use and application or, even better, it should be capable of automatic decision-making and execution. Until we reach this stage, the solution may be outsourcing. Farms could be connected via the internet to a center, where extension experts can analyze the data, run them through physiological models, make decisions and even execute them for ICS, or send short and conclusive reports regarding each cow's health, reproduction and welfare. The manufacturers would have to give this center access to their sensors, so that sensors from different manufacturers can be used on the same farm.
Future research and applications
There are numerous possibilities for IDCM research, be it sensors, biomarkers and application of existing and newly developed technologies and management routines. This review indicated several future research directions for R&D in IDCM which are a short distance from practical and beneficial application:
• Health: integrating several sensors to indicate a health problem, and developing teat accustomed milking cups in milking robots
• Reproduction: conception index based on emergence point from negative energy balance
• Nutrition: individual concentrates supplementation (ICS) using measured energy balance with model calculated food intake that uses actual feeding time and milk energy, and accurate supplementation of Na, K and Cl during transition time
• Wellbeing: development of cow-size adjustable free stalls and usage of milking parlor variables to improve milker/cow interactions