Introduction
Tossa (Corchorus olitorius L.) is known as ‘sonali ansh’ (the golden fibre) of Bangladesh which is the finest-quality natural jute fibre (Mir et al., Reference Mir, Rustgi, Sharma, Singh, Goyal, Kumar, Gaur, Tyagi, Khan, Sinha, Balyan and Gupta2008). Tossa is broadly cultivated in Bangladesh, India, Nepal, China, Indonesia, Thailand, Myanmar and South American countries (Mukul et al., Reference Mukul, Akter, Islam, Bhuiyan, Mostofa, Ghosh, Saha and Ali2021). Tossa was cultivated commercially as a traditional cash crop and used as a commercial source of fibre in Bangladesh (Rahman et al., Reference Rahman, Kaza, Begum and Alam2017). It belongs to the genus Corchorus of the family Malvaceae, which consists of 50–60 species distributed all over the tropical and sub-tropical region (Bayer and Kubitzki, Reference Bayer, Kubitzki, Kubitzki and Bayer2003). Fibres stripped from the Tossa stem are used in packaging (bags, ropes, carpet, sacks), for coarse cloth and paper, handicrafts for home decoration, shoes and jewellery, etc. Tossa is also used to produce insulation, engineered wood, animal food, seed oil and potential bio-fuel (Das et al., Reference Das, Banerjee, Topdar, Kundu, Mir, Sarkar, Sinha, Balyan and Gupta2012; Chakraborty et al., Reference Chakraborty, Sarkar, Satya, Karmakar and Singh2015). Tossa is cultivated in the lower flood plain of Bangladesh from mid-April to September (YAS, 2015). It has tolerance to drought but is prone to waterlogged conditions (Prodhan et al., Reference Prodhan, Rahman and Haque2001). It has higher productivity in loamy and clay soil (Banerjee, Reference Banerjee1955) where, weather condition also influences to grow world's best quality of jute in Bangladesh. Even though Bangladesh is the largest exporting country contributes approximately 39% of the overall raw jute supply cultivated on 39% of the total worldwide jute area (Rahman et al., Reference Rahman, Kaza, Begum and Alam2017). Its production is estimated lower despite extending planting due to crop damage with excessive rainfall, premature harvesting, lack of labour and farmers' choice of paddy production.
Climate change encompasses both global warmings caused by greenhouse gas emissions and the ensuing large-scale weather pattern adjustments. Climate feedbacks such as the loss of sunlight-reflecting snow and ice cover, increasing water vapour (a greenhouse gas) and changes to land and ocean carbon sinks speed up or slow down temperature rise. Warmer temperatures increase evaporation rates, resulting in more severe storms and weather extremes (IPCC, Reference Stocker, Qin, Plattner, Tignor, Allen, Boschung, Nauels, Xia, Bex and Midgley2013). Many of the expected effects of climate change will exacerbate Bangladesh's existing environmental problems, i.e. increased flooding, increased drought and temperature extremes (Huq and Ayers, Reference Huq and Ayers2008). The production of Tossa is detrimental due to global warming, e.g. drought by increased temperature and excessive rainfall or flood, while it can thrive at a minimum temperature of 15°C to maximum temperature of 38°C and may increase up to 46°C (Islam et al., Reference Islam, Begum, Alam, Ahmed and Alamgir2009). The seed sowing time has been changed over time due to changing response of increased temperature and rainfall. The increased temperature due to climate change is substantial for ecosystem and biodiversity loss, which affects species' natural distribution (Glick et al., Reference Glick, Stein and Edelson2011; Anup, Reference Anup2021). Conservation is important to mitigate the impacts of climate change on the ecosystem (Balmford and Bond, Reference Balmford and Bond2005). Therefore, two Representative Concentration Pathway scenarios (RCP4.5 and RCP8.5) of global warming were selected to identify the climatic influence on the distribution of Tossa in Bangladesh for the 21st century (Solomon et al., Reference Solomon, Qin, Manning, Chen, Marquis, Averyt, Tignor and Miller2007).
Maximum entropy (Maxent) modelling was used to detect the occurrences of Tossa in Bangladesh under biophysical and environmental conditions. Maxent is the most widely used method for the prediction and mapping of potentially suitable habitat for threatened species with presence-only data which is critical for monitoring and restoration, and selecting conservation sites for the management of the native habitat (Gaston, Reference Gaston and Gaston1996; Elith et al., Reference Elith, Graham, Anderson, Dudik, Ferrier, Guisan, Hijmans, Huettmann, Leathwick, Lehmann, Li, Lohmann, Loiselle, Manion, Moritz, Nakamura, Nakazawa, Overton, Peterson, Phillips, Richardson, Scachetti-Pereira, Schapire, Soberon, Williams, Wisz and Zimmermann2006). It could be performed using a small number of occurrence records successfully and statistically significant (Kumar and Stohlgren, Reference Kumar and Stohlgren2009). There was a recent study in the Journal of Agricultural Science that used the state-of-the-art techniques of Maxent in geo-spatial sciences and computer soft supremacy which have aided numerous studies of climate change impact and spatial modelling (Chhogyel et al., Reference Chhogyel, Kumar, Bajgai and Jayasinghe2020). In the current study, the significance of Maxent modelling is to predict the potentially suitable habitat for Tossa in Bangladesh using nine environmental variables consequently prioritizing conservation needs.
EdGCM has been used to study the climatic variation of Bangladesh at a regional scale to the upcoming decades of 2100 (Ishaque et al., Reference Ishaque, Ripa, Hossain, Sarker, Uddin, Rahman and Baidya2021). It has the benefit to the climatic model setup, model operation, post-processing, interpretation and scientific visualization developed by NASA and Columbia University (Saadat et al., Reference Saadat, Islam and Rahman2012). It deals with the assembly of climate impacts that will occur over the coming decades (Chandler et al., Reference Chandler, Sohl, Zhou and Sieber2011).
Recently, global warming is one of the threatening problems due to emissions of greenhouse gases. This leads to the amplified sea level, monsoon shifting, melted glaciers and natural hazards, i.e. floods, cyclones and droughts. Tossa Jute production is probable to fall due to deficient monsoon, excessive floods and droughts (Islam et al., Reference Islam, Begum, Alam, Ahmed and Alamgir2009). The major objectives of the current research were: (1) predicting suitable habitat distribution for threatened Tossa to inform conservation planning in Bangladesh; and (2) identifying the environmental factors associated with Tossa habitat distribution.
Materials and methods
Research site
Bangladesh (20°34′ to 26°38′ North latitude and 88°01′ to 92°41′ East longitude) was primarily a low-lying plain land of about 1 44 000 km2, situated on deltas of large rivers flowing from the Himalayas (Shahid, Reference Shahid2010a, Reference Shahid2010b). Bangladesh was bordered by India to the west, north and east, by Myanmar to the south-east and by the Bay of Bengal to the south (Fig. 1). Most parts of the country are low-lying land comprising mainly the delta of the Ganges and Brahmaputra rivers (Rashid, Reference Rashid1991). Eighty per cent of the land surface in Bangladesh was a floodplain, 8% terraced and 12% hilly areas (Brammer, Reference Brammer1996; Rahman et al., Reference Rahman, Moslehuddin, Saha, Ahmed and Egashira2005). Only in the extreme northwest are elevations greater than 30 metres above sea level (m a.s.l.). The northeast and southeast portions of the country are hilly, with some tertiary hills over 1000 m a.s.l. (Huq and Asaduzzaman, Reference Huq, Asaduzzaman, Huq, Karim, Asaduzzaman and Mahtab1999). Bangladesh has a subtropical humid climate with wide seasonal variations in rainfall and moderately warm temperatures (Shahid, Reference Shahid2010a, Reference Shahid2010b). The annual average temperature of the country ranges from 17°C to 31°C and annual precipitation varies from 1400 mm in the west to more than 4300 mm in the east of the country (Rahman et al., Reference Rahman, Shahjahan and Ahmed2021). In Bangladesh rainfall mostly happens in monsoon, instigated by tropical depressions transported from the Bay of Bengal into Bangladesh by the wet monsoon winds (Islam and Uyeda, Reference Islam and Uyeda2008). The planting time of Tossa is during March–April and grows in low-lying land (Gupta et al., Reference Gupta, Sahu and Banerjee2009).
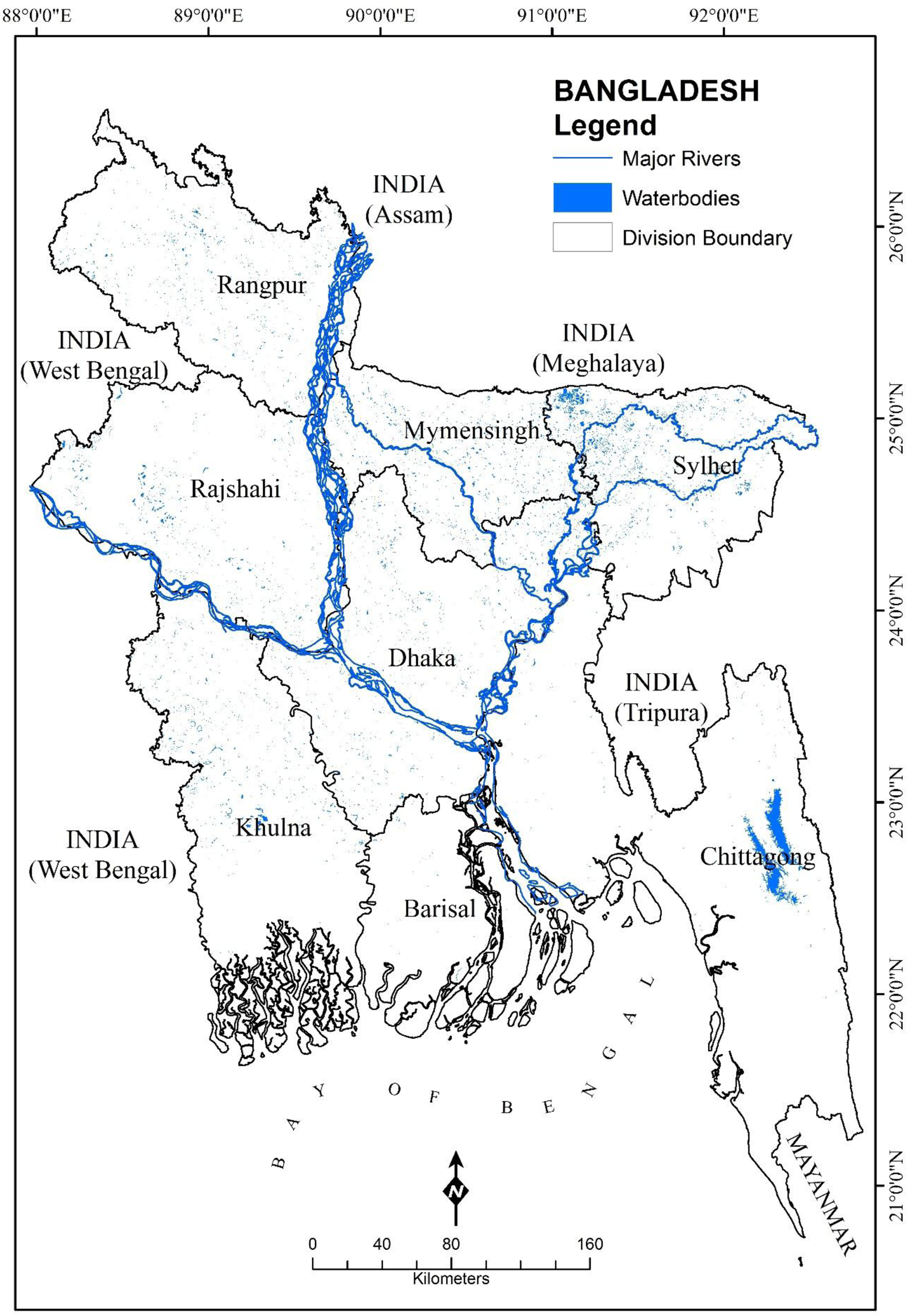
Fig. 1. Colour online. The location of Bangladesh including the surrounding border countries.
Species occurrence data
Species occurrence records, Geographical Information System (GIS) environmental layers (bioclimatic and topographic) and the Maxent and educational global climatic modelling (EdGCM) modelling approach were used to predict the potentially suitable habitat for Tossa under climate change for the 21st century. The 239 sample points were collected as the zones of Tossa in Bangladesh via Global Positioning System (GPS) in 2017 using a stratified random sampling technique. Nine significant environmental variables were subsequently selected as the predictors and used to predict the suitable locations of Tossa in Bangladesh. The data of nitrogen (N), phosphorus (P), potassium (K) and sulphur (S) were obtained from Soil Resources Development Institute (SRDI, 2015). Precipitation, temperature, geomorphology and soil pH data were collected from Bangladesh Country Almanac (BCA, 2016). The elevation data were derived from the United States Geological Survey (USGS) (2017). All environmental variables were projected to World Geodetic System (WGS) 1984 Geographic Projection System. All the variables were converted to American Standard Code for Information Interexchange (ASCII) grid format for the analysis at a cell size of (~90 × 90 m) in ArcGIS 10.8.
Climatic scenarios
The climatic scenarios of RCP4.5 and RCP8.5 were used to project the possible suitable localities of Tossa in the context of climatic change (mean temperature rise of 1.8°C and 3.7°C respectively) to the year of 2100 in Bangladesh. While RCP4.5 means radiative forcing increase of 4.5 W/m2, which assumes that greenhouse gas emissions slow down through mid-century and decrease abruptly afterwards (Thomson et al., Reference Thomson, Calvin, Smith, Kyle, Volke, Patel, Arias, Sabrina, Bond-Lamberty, Wise, Clarke and Edmonds2011). According to the Intergovernmental Panel on Climate Change (IPCC), RCP8.5 corresponds to persistent increases in greenhouse gas emissions up to the end of the 21st century (IPCC, Reference Stocker, Qin, Plattner, Tignor, Allen, Boschung, Nauels, Xia, Bex and Midgley2013). 19 bioclimatic variables were used from the Australian Community Climate and Earth-System Simulator (ACCESS1-0) for the year 2070 from the Coupled Model Intercomparison Project (CMIP5) Phase 5 by the IPCC at 30 arc seconds and ~1 km2 resolution (http://worldclim.org/) (Yang et al., Reference Yang, Hu, Xie, Lai, Yang, He and Zhou2017). Amongst the Global Climate Model (GCM), ACCESS1-0 was used because of the reliable projection of temperature and precipitation which is a historic experiment, projecting the global climate flanked by 1850–2006, by historical forcings (Collier and Uhe, Reference Collier and Uhe2012; Dix et al., Reference Dix, Vohralik, Bi, Rashid, Marsland, O'Farrell, Uotila, Hirst, Kowalczyk, Sullivan, Yan, Franklin, Sun, Watterson, Collier, Noonan, Rotstayn, Stevens, Uhe and Puri2013). The bioclimatic variables represent mean annual temperature, annual precipitation; seasonality (e.g. annual range in temperature and precipitation); and temperature of the coldest and warmest month and precipitation of the wet and dry quarters.
Maxent modelling algorithm
Maxent (version 3.3.3, http://www.Cs.princeton.edu/-schapire/maxent/) modelling was used to predict the potential distribution of Tossa species from presence-only data and environmental variables (Phillips et al., Reference Phillips, Dudík and Schapire2004; Zhang et al., Reference Zhang, Tang, Ren, Zhao and Wang2021). Currently, this method is widely used and has the efficiency to handle complex interactions between response and predictor variables (Elith et al., Reference Elith, Graham, Anderson, Dudik, Ferrier, Guisan, Hijmans, Huettmann, Leathwick, Lehmann, Li, Lohmann, Loiselle, Manion, Moritz, Nakamura, Nakazawa, Overton, Peterson, Phillips, Richardson, Scachetti-Pereira, Schapire, Soberon, Williams, Wisz and Zimmermann2006). The Maxent modelling was conducted with the default variable responses settings and a logistic output format which resulted in a map of habitat suitability of the species ranging from 0 to 1 per grid cell, wherein the average observation should be close to 0.5 (Elith et al., Reference Elith, Phillips, Hastie, Dudík, Chee and Colin2011). The models were evaluated by the Area Under the Curve (AUC). The area under the Receiver Operating Curve (ROC), known as AUC which is one of the most common statistics to assess the model performance. AUC can be interpreted as the probability that a presence cell has a higher predicted value than an absence cell, both of them being chosen randomly. 25% data of the samples were used for the testing and 75% for the training in the Maxent modelling algorithm.
Educational global climatic modelling (EdGCM)
EdGCM (version 3.2, build 926) was used to compare the climate modelling results from the scenarios of the IPCC RCP4.5 and RCP8.5 (GISS, 2016). EdGCM is a climate modelling software suite and computationally efficient enough for use on personal computers, which was developed based on the Goddard Institute for Space Studies (GISS) General Circulation Model II, NASA for global climate modelling (Chandler et al., Reference Chandler, Shopsin and Richards2005). This model has 7776 grid cells in the atmosphere, with each horizontal column corresponding to the spatial resolution of the climate model is 8° × 10° layers (8° latitude by 10° longitude), containing nine vertical layers, two ground layers (one to model surface absorption and one to model deep ground properties) and two ocean layers (Saadat et al., Reference Saadat, Islam and Rahman2012). It allowed running simulations, post-processing and analysing data using scientific visualization for data display. Scheming for each grid cell was iterated in fifteen-minute (simulation time) during the simulation.
Results
The extinction of Tossa in Bangladesh from 2005 to 2018
The southwestern part of Bangladesh is the most cultivated region of Tossa due to favourable weather conditions. Mainly the low-lying flood plain including the riverside area is preferable for the Tossa cultivation across the country (Fig. 2). The total area under Tossa has been estimated at 677 678 ha in 2018 which is 0.75% higher than that of last year (BBS, 2018). The geographical regions of Brahmaputra Alluvium, Ganges Alluvium and Teesta Silt is known as the Ganges delta in Bangladesh contribute >80% of the jute production in the world (Islam et al., Reference Islam, Xiaoying, Uddin and Bhuiyan2015). Brahmaputra Alluvium comprises part of the districts of Dhaka, Mymensingh, Tangail and Comilla of Bangladesh annually receives a fresh deposit of silts carried down by the floodwater including acidic soils with the varied texture from sandy loam to clay loam. The Ganges Alluvium comprises part of the districts of Kushtia, Jessore, Khulna, Rajshahi, Pabna and Dhaka of Bangladesh. The soil of this area is slightly alkaline clay loam to light loam in grey to dark grey colour (NIIR Board of Consultants and Engineers, 2014; Nitu et al., Reference Nitu, Islam and Tarique2019). The northern area of Teesta Silt comprises part of Dinajpur, Rangpur, East Bogra and Sirajganj districts of Bangladesh, which has sandy soil with low moisture retention capacity including slightly acidic soil retention (Mukund, Reference Mukund2018).

Fig. 2. Colour online. The locations of Tossa species in 2005 and 2018 through Bangladesh.
Evaluation of the Maxent model output
All Maxent model outputs had a high discriminative ability with an average AUC of 0.82 ± 0.02 for the testing data and 0.86 ± 0.02 for the training data while the algorithm terminated after 500 iterations. The threshold-independent Maxent modelling approach performed better with the higher AUC values of 0.86 for ROC (receiver-operation characteristic), because the higher the AUC (ranging from 0.5 to 1.0) the better is the predictive performance and discriminative ability of the model. Based on AUC, the application of the Maxent model has predicted Tossa occurrence with high accuracy.
Contribution of environmental variables
To identify the relative importance of various environmental characteristics for Tossa habitat suitability, nine environmental variables were used for the Maxent model. However, the per cent contribution heavily relied on the chosen algorithm and did not reflect correlation among characteristics. Table 1 shows that elevation ranked first (contribution >29%) among all of the nine environmental variables suitable to Tossa establishment. N, temperature and precipitation have a cumulative contribution ⩾16% for the suitability prediction of Tossa. Soil S and K were also important factors determining the Tossa habitat suitability with a contribution of >3%. Geomorphology, soil pH and P gained the least contribution <1% among all the variables.
Table 1. The contribution of nine environmental variables to the prediction of possible habitats for Tossa in Bangladesh

The most preferred land elevation for Tossa was 0 to 3 m since Tossa usually grows well in low elevated land and plain alluvial soil (Fig. 3). The preferable N content in the soil was 20–50 kg/ha for Tossa. The suitable climate for growing Tossa was offered by the monsoon climate (hot and humid). Temperatures ranged from 20°C to 30°C and relative humidity of 70–80% was favourable for successful Tossa cultivation. Tossa required 1000–2000 mm annual rainfall for the well growing. For healthy Tossa cultivation S content in the soil ranged 15–30 kg/ha, K 10–20 kg/ha and P 12–20 kg/ha were suitable. The suitable geomorphology was 0–90 feet and soil pH 4.5–6 for the regeneration Tossa.
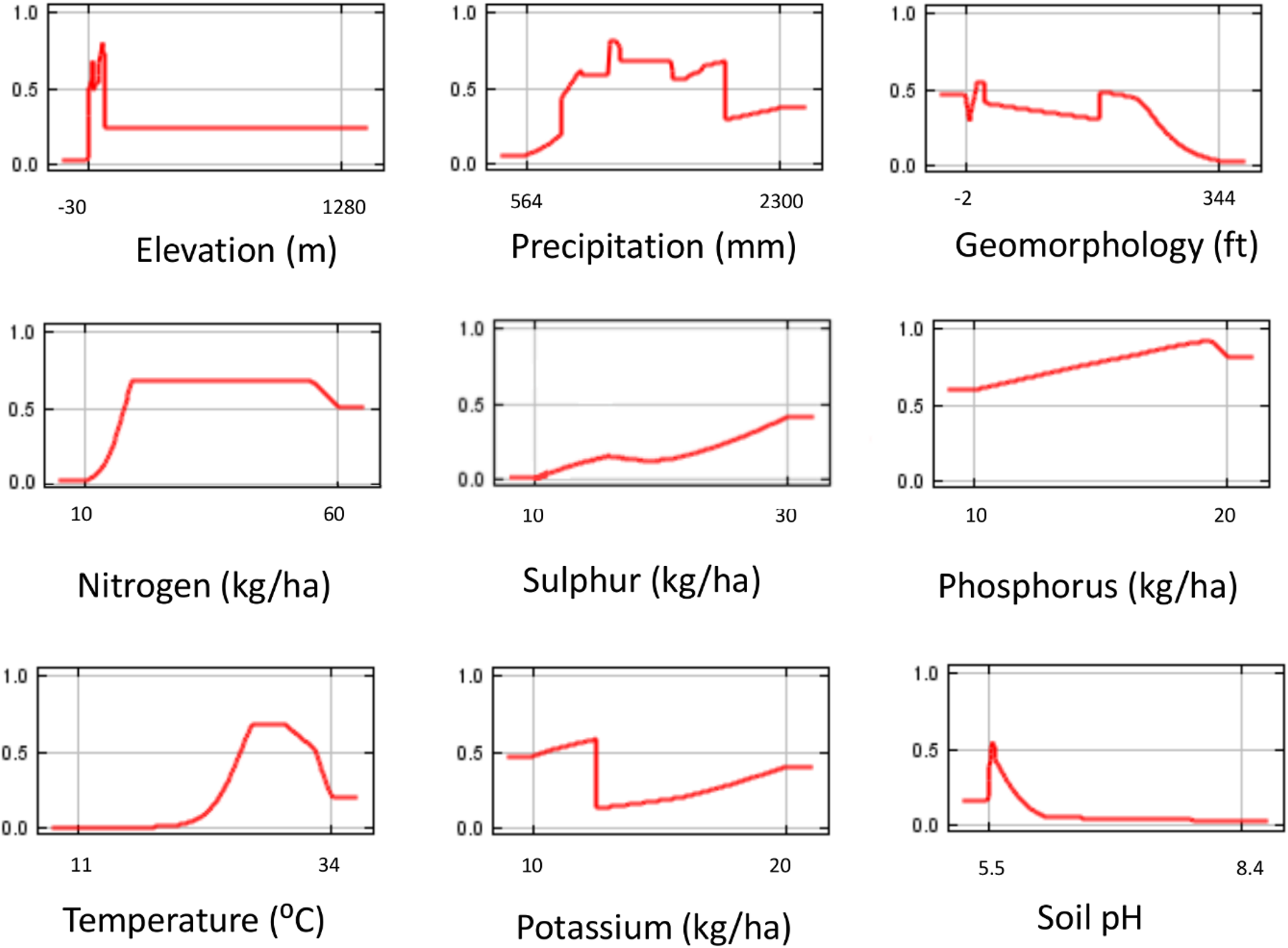
Fig. 3. Colour online. The response curves of the environmental variables influencing the suitable locations of Tossa species in Bangladesh. *The 0–0.8 legend on the horizontal axis represents the per cent contributions of the environmental variable.
A Jackknife test was checked to measure the relative importance of each environmental variable (Fig. 4). The environmental variable with the highest gain, when used in isolation, was N, which therefore appeared to have the most useful information by itself. The environmental variable that decreased the gain the most when it is omitted was the temperature, which therefore appeared to have the most information that wasn't present in the other variables. Maxent measured this by ‘gain’, which represented how much better the distribution fitted the sample points.
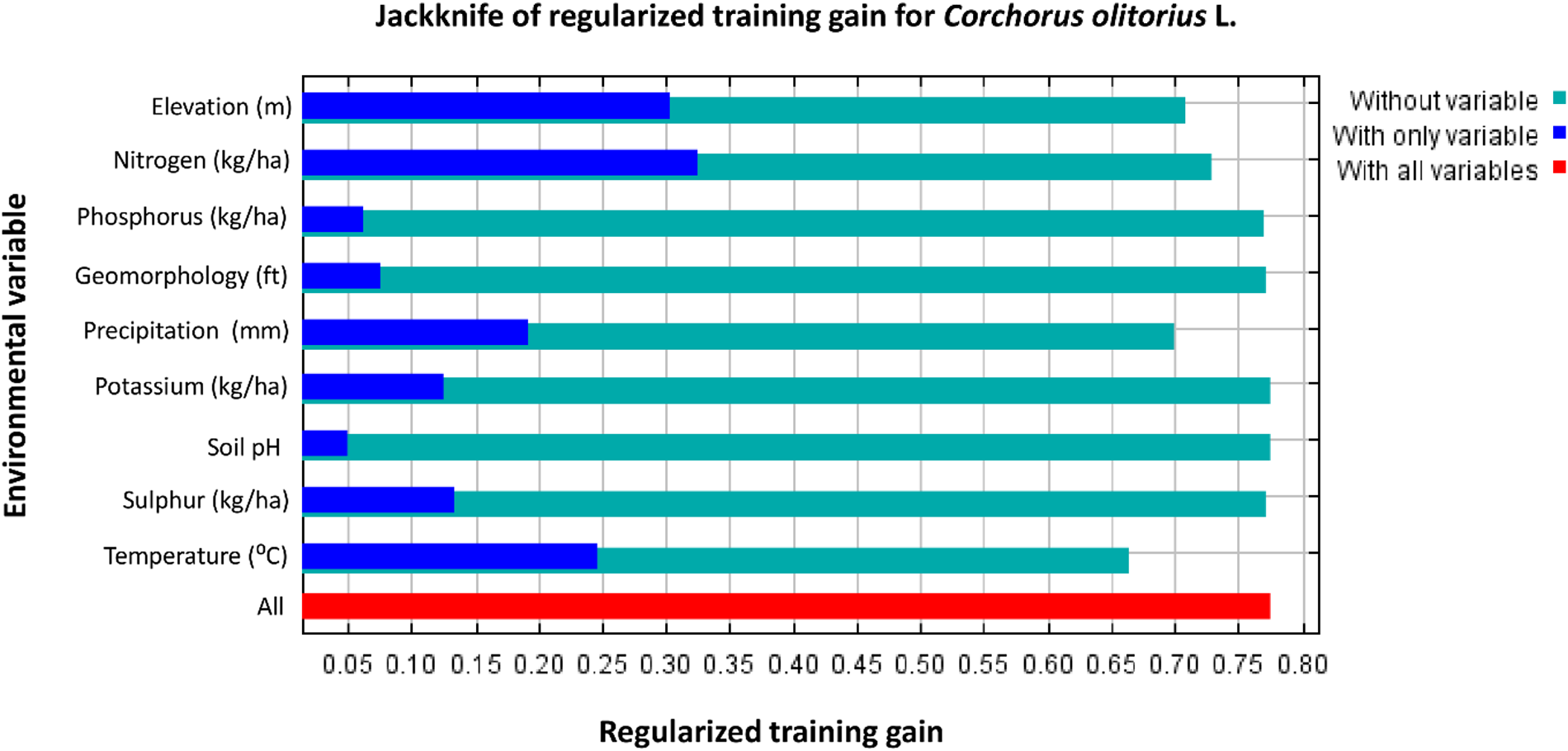
Fig. 4. Colour online. The Jackknife test of the environmental variables influencing for identifying the importance of all the variables for Tossa species.
Current potential distribution of Tossa species
The Maxent predictions showed a continuous potential distribution for Tossa in Bangladesh. The new high suitability areas occur primarily in the central part of Bangladesh including moderately suitable areas to the south-central part, central-eastern, central-western and central-northern part (Fig. 5). The results showed a larger suitable area of the potential habitat for Tossa in Bangladesh which is 5.1% greater than the present area. A low, medium and high habitat suitability of Tossa was predicted with different land cover types. Maxent output was categorized into the frequency distribution classes for the habitat suitability as low 0.00–0.23, medium 0.23–0.38, medium-high 0.38–0.62 and high 0.62–1.00 (Yang et al., Reference Yang, Kushwaha, Saran, Xu and Roy2013).
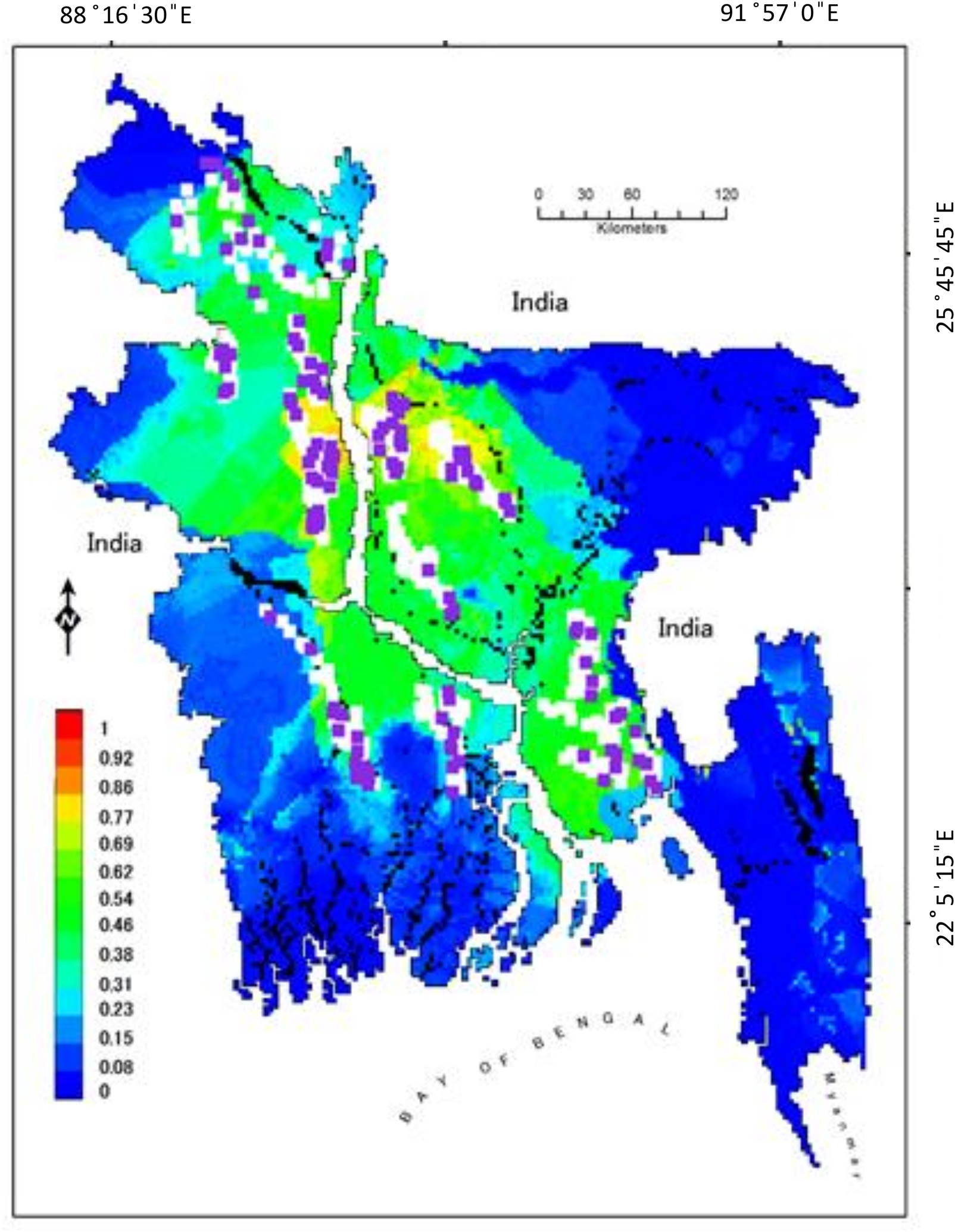
Fig. 5. Colour online. The current potential distributions of Tossa species in Bangladesh. The modelling was conducted using 239 sample location points. The warmer colour shows better-predicted areas for Tossa.
Global climate impacts on the habitats of Tossa to the year 2100
The two RCP scenarios have varied influences on the distributions of Tossa in Bangladesh. Maxent models predicted that the climate suitability for Tossa will decline 2% for the RCP4.5 and 9% for the RCP8.5 to the 2100 year (Fig. 6). The RCP4.5 scenario showed the least decrease in the Tossa habitat because of the slight projected mean temperature rise (1.8°C), while the RCP8.5 scenario predicted a reasonable decrease in the Tossa habitat with the projected mean temperature rise of 3.7°C. The decline for the RCP8.5 scenario was severe because the temperature increase exceeds the tolerable temperature of 30°C, while the decline due to the RCP4.5 scenario was understated. The northern and south-eastern part was predicted as unsuitable with RCP4.5 and RCP8.5 scenarios respectively for the Tossa habitat.

Fig. 6. Colour online. The impact of RCP4.5 and RCP8.5 scenarios of the ACCESS1-0 GCM for the distribution predictions of Tossa species in Bangladesh to the 2100 year.
RCP4.5 exhibited the three highest-contributing bioclimatic variables as BIO11 (mean air temperature during the coldest quarter; 47.5%), BIO14 (precipitation during the driest month; 17.7%) and BIO8 (mean air temperature during the wettest quarter; 8.8%) (Table 2). BIO17 (precipitation during the driest quarter; 38.6%), BIO18 (precipitation during the warmest quarter; 13.7%) and BIO7 (annual temperature range; 10.5%) presented the highest contributions for RCP8.5. There were no contributions of BIO6 (Min Air Temperature of Coldest Month), BIO15 (seasonal precipitation) and BIO16 (precipitation during the wettest quarter).
Table 2. The contribution percentages of bioclimatic variables used to predict the future distribution of Tossa in Bangladesh

The results of EdGCM showed a 4.2°C rise in mean annual temperature and a 1.5 mm decline in rainfall in Bangladesh to the year 2100 (Fig. 7). This increase in temperature and reduced rainfall caused a 10.2% deterioration of the Tossa habitat from the existing distribution in Bangladesh.
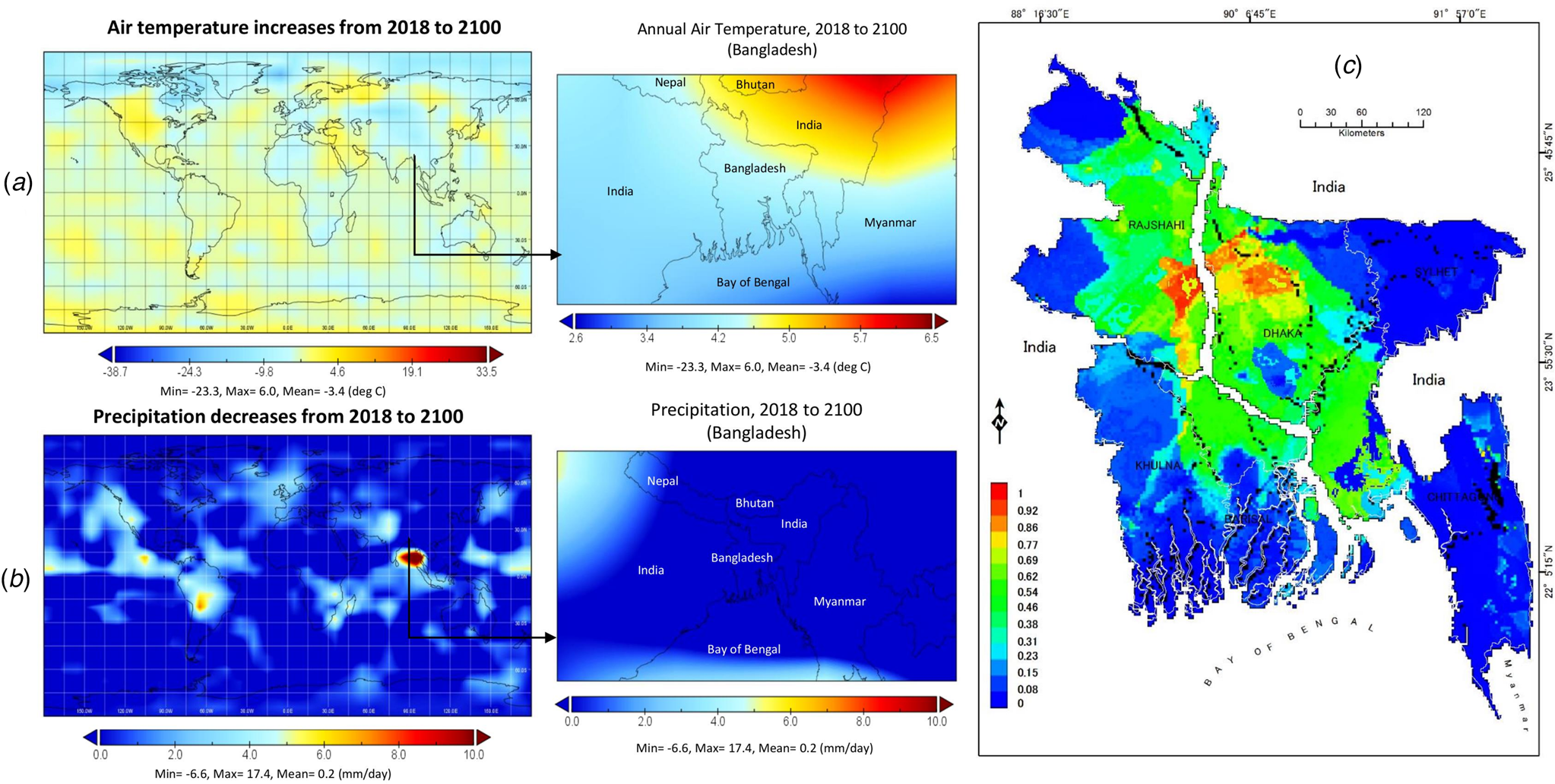
Fig. 7. Colour online. Changes in temperature and rainfall from the output of EdGCM predicted for the year 2100; (a) shows the increase of annual air temperature from 2018–2100 in a global scale, (b) demonstrates the precipitation variability in Bangladesh from 2018–2100 in a global scale and (c) displays the existing habitat distribution of Tossa via the EdGCM prediction in Bangladesh.
Discussion
Habitat suitability and environmental variables
The distribution of Tossa was mostly influenced by the elevation, N, temperature and precipitation (Table 1). It responded slightly to soil S and K, where geomorphology, soil P and pH influenced faintly. Percentage of elevation, N, temperature and precipitation were considered the four most important environmental variables to the model prediction. They had the highest relative contributions, the highest regularized training gain when used in isolation in the Jackknife test and also the highest AUC values when used in isolation. Maxent's projections of predicted Tossa's habitat suitability in restoration scenarios showed an increase of high suitability areas (>0.62 per grid cell, where habitat suitability ranges from 0–1 per grid cell, wherein the typical is near to 0.5) of 0.5% of total country land in all substantial environmental variables (Elith et al., Reference Elith, Phillips, Hastie, Dudík, Chee and Colin2011). Nabil and Ashraf (Reference Nabil and Ashraf2011) and Musa et al. (Reference Musa, Ezenwa, Oladiran and Akanya2010) stated N as an important factor for the growth, yield, nutrient use efficiency and quality of Tossa. They also stated that N excites the new leaves formation and increases the size and height of the plant. Precipitation was found significant for growth, yield, stem diameter and the number of branches per plant by Fasinmirin and Olufayo (Reference Fasinmirin and Olufayo2009) and Whitfield et al. (Reference Whitfield, Wright, Gyles and Taylor1986). The presence of K and P offered adequate nutrients for leaf area development and plant stem diameter. These improved the plant height, the number of leaves, stem diameter, total leaf area and the number of branches of Tossa (Aliyu, Reference Aliyu2000; Katé et al., Reference Katé, Tovihoudji, Batamoussi-Hermann, Sossa, Idohou, Agbangba and Sinsin2020).
The result of the Maxent showed a strong relationship between Tossa probability of distributions and low elevated land in Bangladesh because Tossa grows well in low-lying and plain land. The elevation was linked to a range of environmental factors and temperature differences, influencing the growth of Tossa (Benor et al., Reference Benor, Fuchs and Blattner2011). The modelling showed very low suitability for Tossa in high elevated land (Fig. 5). The projected habitat suitability for Tossa species is in the central and central-northern regions of Bangladesh. The conservation also depends on the preservation of necessary environmental variables in Bangladesh.
Climate change impact on the Tossa
The two scenarios of RCP4.5 and RCP8.5 both have a negative impact on the future distributions of Tossa in Bangladesh. The distributions of the Tossa will decrease the least percentage (2%) with the RCP4.5 scenario. As well, RCP8.5 will cause a sensible decline of the Tossa area of 9% to the year 2100 due to altered average temperatures, rainfall and increased extreme events of heat and cold waves, flooding, etc. (Maheswari et al., Reference Maheswari, Sarkar, Vanaja, Srinivasa, Srinivasa, Venkateswarlu and Sikka2015). The vital bioclimatic variables elucidating the future suitable Tossa distribution were BIO11, BIO17, BIO14, BIO18 and BIO1 for both of the climatic models, and results suggested that BIO14, BIO7 and BIO11 were the most common contributors (Shishir et al., Reference Shishir, Mollah, Tsuyuzaki and Wada2020). On the other hand, the EdGCM modelling results showed a comparable negative impact on the Tossa distributions. The habitat suitability will be condensed by 10.2% owing to the increased mean temperature of 4.2°C. A reduced annual rainfall of 1.5 mm to the 2100 year also will cause a decline of Tossa in Bangladesh because the water supports plants morphological development (plant height, stem diameter, number of green leaves, leaf elongation, leaf area and dry weights) (Franco et al., Reference Franco, Arreola, Vicente and Martinez-Sanchez2008; Yakoub et al., Reference Yakoub, Benabderrahim and Ferchichi2016).
Thus, land and management executives should pay additional consideration to Mean Air Temperature of Coldest Quarter; Precipitation of Driest Month, Driest Quarter and Warmest Quarter; and Temperature Annual Range including Max and Min air temperature. Therefore, this is a vital period to promote awareness against the vulnerability of Tossa. In addition, there is a varied range of uncertainties in climate change due to multi-model climate scenarios including scale variabilities. However, additional research is required for a more accurate model using precise climate analysis considering similar spatial resolution data.
Conservation planning of Tossa species
The results presented in the current study suggested a larger area can be protected for the cultivation of Tossa in Bangladesh in association with the presence of sufficient rainfall and temperature including N content in the soil. Total 7533.32 km2 highly suitable areas might be protected for the conservation of Tossa species in Bangladesh. Therefore, highly suitable areas for Tossa might be considered for newly potential protected sites via the expansion of the existing areas. Besides, areas of medium and medium-high suitability can be protected which are correspondingly important areas for Tossa cultivation. These medium, medium-high and high suitable areas should be considered in the protected areas management plans as necessary to conserve the Tossa species.
Conclusion
In this study, two different models, MaxEnt and EdGCM were used to predict the habitat suitability of Tossa in Bangladesh using 239 occurrence records and 19 bioclimatic variables. Both of the two models predicted the future potential distributions towards the 21st century. Results suggested that Tossa in Bangladesh is susceptible due to the worst state of climate change. Maxent flourished the precise distributions of Tossa using RCP4.5 scenarios based on its presence data and the effective environmental factors used in the current study. MaxEnt (RCP8.5) predicted that Tossa will decline much under the RCP8.5 scenario by the end of the 21st century due mostly to intensifying temperatures resulting in precipitation inconsistency and extreme drought conditions. This also may affect the morphological development of Tossa. Additionally, Maxent identified elevation, N, temperature and precipitation as the prime determinants of Tossa distribution, which was controlled by both edaphic and climatic factors. In the case of EdGCM, the results are comparable to the MaxEnt to predict the habitat suitability of Tossa. The results indicated that the most alarming issue was the intensified mean annual temperature of >4°C which may cause precipitation variability and extreme drought condition ensuing decline of the Tossa habitat in Bangladesh.
Acknowledgements
We acknowledge the organization of BBS (Bangladesh Bureau of Statistics) and BCA (Bangladesh Country Almanac) for providing essential data for this research.
Financial support
This research received no specific grant from any funding agency, commercial or not-for-profit sectors.
Conflict of interest
The authors declare there are no conflicts of interest.
Ethical standards
Not applicable.