Introduction
Randomised-controlled experiments represent the gold standard for ascertaining causation. This is not to say that experiments are free of limitations and, as will be clarified, some of them contributed to a certain reluctance towards the usage of experimental methods in political science. While acknowledging the merit of experimentation in the empirical investigation of causal claims, political scientists long lagged behind psychologists and economists in the use of the experimental approach. It was only with the development of (population-based) survey experiments, facilitated by new advancements in survey techniques, that some of these limitations, and in particular the scarce generalisability of the results from an experimental sample to a population of interest, were mitigated, unveiling the virtues of the experimental design to a wider audience of social and political scientists.
This article provides an overview of the use of survey experiments in political research and an illustration of how experimental data can be modelled and interpreted. Then, it describes some key concepts for the understanding and application of experiments in the discipline, clarifying the basic theoretical issues tied to causal inference and the most common experimental designs to achieve it. In the following section, we focus on survey experiments and their ambition to combine internal and external validity. By presenting the results of a content analysis of experimental articles published by leading academic journals over the last two decades, we show a lag in the use of experimentation in European research as compared to the American one, but also an increasing interest in survey experiments from European scholars. Based on this and with the purpose of introducing the main challenges and possibilities encountered in designing, planning and implementing survey experiments, we then contrast a traditional factorial and a more innovative conjoint design, considering how treatments are usually formulated and assigned. In this regard, we illustrate how survey experimental data can be analysed to estimate average treatment effects and conditional treatment effects across subgroups of subjects by means of two original examples on attitudes towards asylum seekers and preference towards political candidates, respectively. We conclude with some considerations on the merits, limits and implications of experimental designs in political research.
A primer to experiments
Green (Reference Green, Lewis-Beck, Bryman and Futing Liao2004) points to two main characteristics to distinguish the experimental design from other methods of social investigation: a planned intervention and a random assignment. The first refers to the treatment administered to the units of analysis and whose impact on the outcome variable the researcher is interested to estimate. The treatment represents the independent variable and, in controlled experiments, is manipulated under the direct control of the researcher. The second characteristic, instead, has to do with the process through which subjects are allocated to one (or more) treatment group(s). Randomisation ensures that all groups are balanced across potential covariates – an assumption that the experimental analyst should nonetheless demonstrate – and that no systemic relationships exist between the treatment factor(s) and other observed or unobserved variables.
Because of these two key features, experimental studies are considered to be better equipped to address causal questions than observational studies. Contrary to observational research, where the researcher usually relies on statistical modelling to avoid problems of endogeneity and unobserved heterogeneity, in an experimental setting confounding variables are controlled by design, resulting in unbiased causal inference if randomisation has been properly implemented. Since in an experimental study each subject has the same probability to be assigned to a treatment condition, the final outcome will solely depend on the stimulus received, while the effect of possible confounding factors will be balanced across all the considered groups.Footnote 1
Experiments usually achieve superior internal validity – the possibility of establishing a causal effect (McDermott, Reference McDermott, Druckman, Green, Kuklinski and Lupia2011) – through a ‘between-subject’ design in which the subject is assigned either to a treatment condition (i.e., the one that receives the intended manipulation) or to a control condition (i.e., the one that is used as a counterfactual to estimate what would have happened if the intervention had not been administered).
However, experiments also allow to vary treatments while holding subjects constant and controlling for subject-specific effects. This is the case of ‘within-subject’ designs, in which each participant receives one or more treatments (or controls) and causal estimates are obtained by comparing the same subjects' behaviour across the different conditions over the experiment duration, generally before and after each treatment. Within-subject designs, which require independence of exposure to multiple treatments, have greater statistical power than between-subject designs, as more data points are offered for the same subjects. Moreover, they are more adequate in environments where an individual faces more than one choice during a sequence of events (e.g., as in bargaining and collective action research). Still, ‘within-subject’ designs may introduce possible confounds by exposing the same subject to multiple interventions and more likely produce spurious results due to a ‘demand effect’, i.e., participants understand the experimenter's intention and behave accordingly to satisfy her or his expectations (Charness et al., Reference Charness, Gneezy and Kuhnc2012).
The standard procedure to estimate the treatment effect is to compare the differences for the outcome of interest across the different groups or experimental conditions, also known as the average treatment effect (ATE). However, experimenters may also decide to test for possible moderators and baseline covariates that might affect the relationship between the treatment and the outcome, thus assessing possible heterogeneity of a treatment effect depending on another treatment or one or more characteristics of the participants. This is commonly done using a regression model with interaction terms (moderators) to estimate the conditional average treatment effect (CATE).
Across disciplines, experiments have been more concerned with the identification of treatment effects than the generalisability of findings across different subjects and groups – the external validity (McDermott, Reference McDermott, Druckman, Green, Kuklinski and Lupia2011). This is specifically the case of ‘laboratory’ experiments, which generally occur in a more artificial but highly controlled environment. Laboratory experiments have the advantage of flexibility, as they can easily be conducted at low costs with convenience samples, including students and volunteers. However, participants in such experiments are generally viewed as unrepresentative of any target population (Iyengar, Reference Iyengar, Druckman, Green, Kuklinski and Lupia2011). Moreover, laboratory experiments imply some sort of interaction between participants and researchers, so that results may be biased by demand effects (Zizzo, Reference Zizzo2010). Last, in spite of better control, the artificial nature of the setting may prevent results to be extended to real-world situations. It is worth mentioning, however, that this kind of experiments can be moved from a typical university laboratory to a more naturalistic one (township, households), so to have a hybrid ‘lab-in-the-field’ design (Morton and Williams, Reference Morton and Williams2010).
When treatments are randomly administered in a naturalistic setting under the direct control of the researcher, these are classified as ‘field’ experiments. By evaluating the effect of the treatment in a real-world setting, the analyst has the possibility to make unbiased and externally valid causal claims. One can argue that estimates derived from one setting at a given time cannot be applied easily to another context or time period. Still, as Green and Gerber (Reference Green and Gerber2003: 101) pointed out, ‘extrapolation from one field setting to another involves less uncertainty than the jump from lab to field or from non-experimental correlations to causation’. When researchers take advantage of random assignments that occur naturally but not under their direct planned intervention (e.g., use of a lottery to allocate resources, policies or duties), instead, we are in the presence of a randomised ‘natural’ experiment (Dunning, Reference Dunning2012).Footnote 2
Last, experiments may be embedded in a survey through the manipulation of different elements of a questionnaire (Gaines et al., Reference Gaines, Kuklinski and Quirk2007). These experimental settings combine treatment manipulation and random assignment with survey sampling, ensuring a broader variation of the pool of subjects being considered and helping bring experimental research outside of the lab. Survey experiments may be conducted with either non-probability or probability samples of participants; when administered to a randomly selected, representative sample of a target population, they are referred to as ‘population-based survey experiments’ (henceforth PBSE) and allow the researcher to make population inferences about causal relationships drawn from experimental findings (Mutz, Reference Mutz2011).
Survey experiments in political research
Development of survey experiments
Experiments for long remained an almost uncharted territory for political scientists, given their interest in the generalisability of findings to target populations, the asserted artificiality of the laboratory setting and unrepresentativeness of the experimental subjects (Iyengar, Reference Iyengar, Druckman, Green, Kuklinski and Lupia2011). It was only in the 1970s, with the emergence of political psychology as an interdisciplinary field, that a certain interest in the experimental approach started to develop (McGraw and Hoekstra, Reference McGraw, Hoekstra, Delli Carpini, Huddy and Shapiro1994).
Nevertheless, we had to wait until the 1990s to observe a real growth in the number of experimental studies in political science, especially in the US. As Druckman and colleagues have reported, more than half of the experimental articles appeared in the American Political Science Review (henceforth APSR) between its foundation in 1906 and the late 2000s was published after 1992 (Druckman et al., Reference Druckman, Green, Kuklinski and Lupia2006, Reference Druckman, Green, Kuklinski, Lupia, Druckman, Green, Kuklinski and Lupia2011). Political scientists' increasing reliance on experimental methods was later confirmed by Dunning and Rosenblatt (Reference Dunning and Rosenblatt2016), who found a further increase in the number of APSR articles reporting experimental research in the early 2010s. This was the result of technological improvement connected to computer assisted telephone interview as well as ambitious projects, such as the Multi-Investigator Study and the following Time-Sharing Experiments for the Social Sciences, which allowed researchers to administer complex randomised experiments to large probability samples of participants (Sniderman and Grob, Reference Sniderman and Grob1996; Mutz Reference Mutz2011).
Building on Druckman and colleagues' criteria to classify research articles presenting ‘primary data from a random assignment study with participants’ (Druckman et al., Reference Druckman, Green, Kuklinski and Lupia2006: 628–629), we can observe a remarkable increase (33.3%) in the number of APSR articles using randomised experiments in the last five years as compared to the 2010–2014 period (Figure 1), with a peak between 2018 and 2019 (N = 21). Indeed, the percentage of experimental manuscripts over the total number of articles published in an issue of this journal has gradually grown over the last 15 years, so that experimental articles accounted for about one-fifth of APSR manuscripts in 2019.Footnote 3
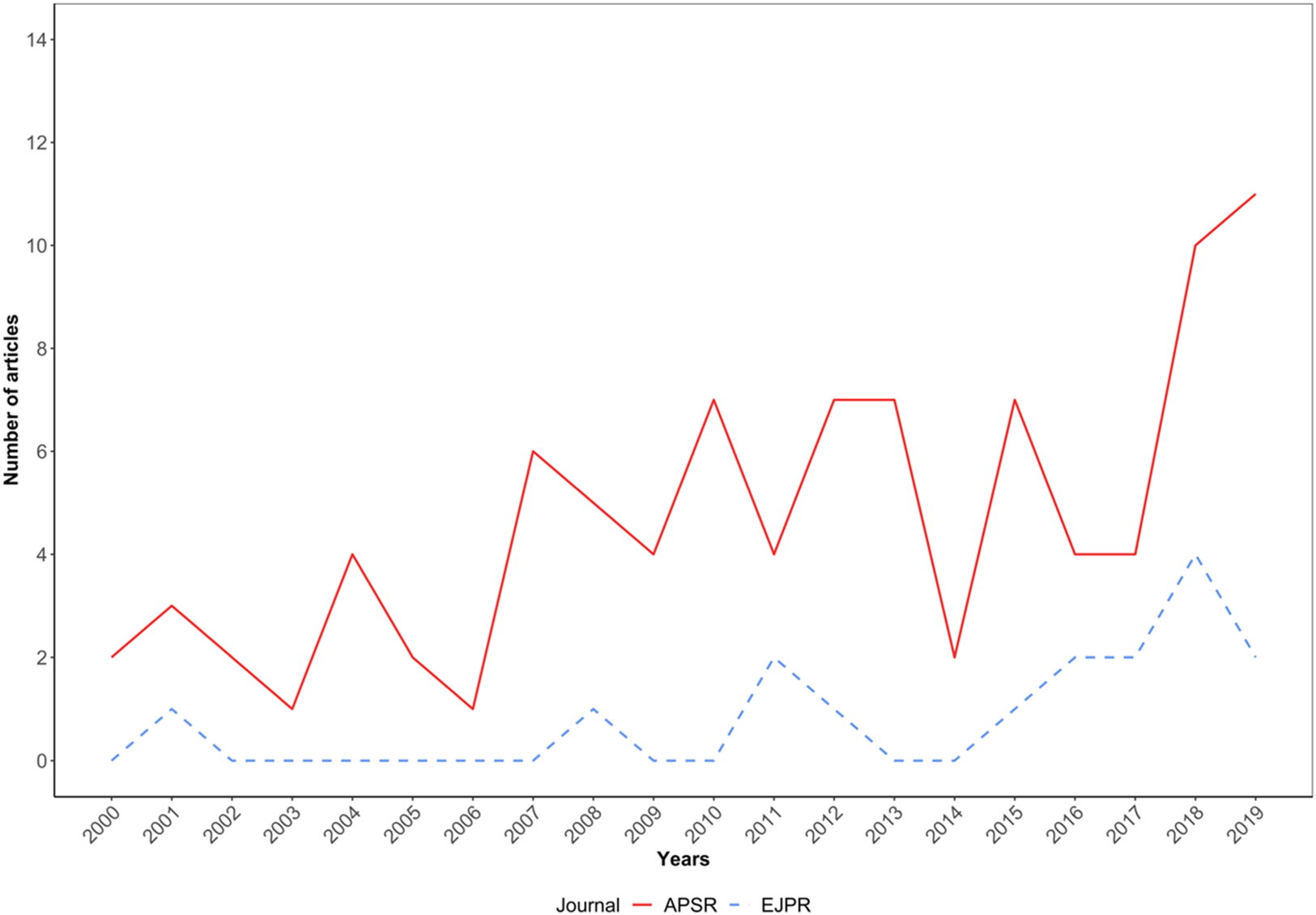
Figure 1. Number of experimental articles in APSR and EJPR, 2000–2019.
A similar upward trend, albeit of different magnitude, is observed in European political research. Using the European Journal of Political Research (henceforth EJPR) as a benchmark to assess whether and to what extent experimentation has also gained acceptance in Europe,Footnote 4 we found that about two-thirds (63%) of the articles making use of randomised experiments between 2000 and 2019 have been published in the last four years. The volume of experimental manuscripts, however, has remained quite low if compared with APSR. Experimental articles, on average, still account for only 5.1% of total manuscripts published each year by EJPR, signalling that the use of experimental methods is not only relatively newer to European scholars but also less prominent than in the American context.
European political scientists' hesitation with the use of experimental methods is also confirmed by a content analysis of the manuscripts. While the typology of experiments in APSR articles is quite various, with more than one-third of the cases (37.6%) making use of a survey experiment, followed by laboratory (32.3%), field (30.1%) and natural experiments (3.2%), almost the totality of EJPR experimental articles (93.8%) relied on survey experiments. Only in a few instances field (12.5%) and laboratory experiments (6.3%) appeared in the journal, whereas natural experiments have not been published in a volume of EJPR over the last two decades. Yet, these figures also highlight a large interest towards survey experiments and their primacy over other type of settings. In this respect, it is interesting to note that PBSE account for 62.9% and 40% of APSR and EJPR survey experiments published in the 2000–2019 period, respectively.
Survey experiments in practice: vignette factorial and conjoint designs
Initially, the use of survey experiments was mostly limited to address measurement issues through the manipulation of the presence, wording or order of questionnaire items and their random allocation to interviewees. In one of the earliest examples of the ‘split ballot’ experiment, for instance, Rugg (Reference Rugg1941) found that Americans were more likely to support freedom of speech against democracy when asked whether the US should ‘forbid’ (46% of the interviewees answered ‘yes’) rather than ‘allow’ (62% of the sample answered ‘no’) public speeches against democracy. Over the years, survey experiments based on question wording have then been employed to address more substantive issues, testing, for instance, how framing may affect citizens' opinions about public policies (e.g., Kinder and Sanders, Reference Kinder and Sanders1996).
However, the most common paradigm to formulate treatments has been the use of vignettes, in which a short text describes a situation, a policy proposal or a political stance often in combination with pictures and/or videos. Vignette experiments are very well-suited to determine the extent to which multiple factors contribute to attitude formation or the occurring of certain behaviours. Overcoming the main weakness of the simple ‘split ballot’, in which levels (values) of different factors (attributes) may vary only one at a time, ‘vignette factorial designs’ allow to estimate the joint effect of multiple attributes at their different levels (Mutz, Reference Mutz2011), with the number of treatment groups being determined by the number of combinations of factor levels.
The first advantage of this design is that factors are randomly assigned and orthogonal to each other, thus allowing a researcher to estimate the effect of each single treatment while ignoring the others if these are shown to be insignificant. Otherwise, s/he needs to take into account that the average effect of one factor is weighted across the levels of the others (Gerber and Green, Reference Gerber and Green2012). Second, factorial designs can be used to estimate not only how two or more treatments interact among them, but also the extent to which a given factor (or the combination of some factors) hinges on a third characteristic of the respondent, which is uncorrelated by randomisation. In this respect, a ‘full factorial design’, in which all the combinations of the factor levels are examined, has to be distinguished from a ‘fractional factorial design’, in which only a subset of these combinations is considered either because their number is too large or because resources for testing them all are not available. This last consideration leads us to one of the disadvantages of factorial designs, which is the trade-off between the number of conditions to be considered and the sample size. Researchers may increase the number of experimental factors only at the cost of efficiency. The number of conditions has, in fact, to be taken into account in relation with the number of subjects per experimental group and statistical power, that is, the probability of being able to reject the null hypothesis of no treatment effect (Gerber and Green, Reference Gerber and Green2012).
Such a disadvantage can be avoided in ‘conjoint analysis’, a prime technique of preference elicitation introduced at the beginning of the 1980s (Alves and Rossi, Reference Alves and Rossi1978) and commonly used in marketing research, whose recent formalisation by Hainmueller et al. (Reference Hainmueller, Hopkins and Yamamoto2013) has contributed to its popularity among political scientists. In conjoint experiments, respondents are requested to choose (discrete-choice conjoint analysis) and/or to rate (rating-based conjoint analysis) sets of possible alternatives (e.g., candidates to vote for, policy proposal to pass) resulting from the random variation of an indefinite number of factors, orthogonal among each other, that can assume multiple values. The analysis consists of estimating the simultaneous independent causal effects – average marginal component effect (AMCE) – of many features of multidimensional objects on the respondent's decision.
Contrary to common survey research, conjoint analysis starts from the assumption that social and political phenomena come in different facets that individuals are likely to simultaneously evaluate in real-world situations. Asking the respondent to judge a given object by introducing trade-off costs among different aspects helps reduce the problem of social desirability and the artificiality of the task while increasing the level of external validity (Hainmueller et al., Reference Hainmueller, Hangartner and Yamamoto2015).
Conjoint experiments are not exempt from criticism. First, they are cognitively demanding, as conducting such complex experiments with many attributes evaluated at once requires the repetition of the designed task. In this respect, there is no agreement on the ideal number of attributes to consider, nor on the number of tasks to be implemented. Researchers need to balance among the theoretical aspects to investigate, the respondents' fatigue, as well as sample size and statistical power (Bansak et al., Reference Bansak, Hainmueller, Hopkins and Yamamoto2018, Reference Bansak, Hainmueller, Hopkins and Yamamoto2021). A second criticism is that conjoint analysis may lead scholars to less formalised and more inductive forms of research, posing less restrictions with respect to the number of factors under observation. As some have argued (e.g., Sniderman Reference Sniderman2018), however, this would represent more a pro than a con, since the whole scope of conducting an experiment is actually to evaluate countervailing explanations.
Remarkably, more than one-third of survey experiments appeared in APSR (5 out of 14) and EJPR (3 out of 10) between 2015 and 2019 were based on a conjoint approach, with the remaining two-thirds largely relying on a traditional factorial design. Given the widespread use of these two techniques in contemporary political research and in order to offer a valuable help to scholars interested in modelling this kind of data, in the next section we present the experimental protocol and results of a full factorial experiment on respondents' evaluation of asylum applications, moving then to a conjoint experiment on preferences towards ideal political candidates. First, however, we describe some problems to internal and external validity a researcher may face when conducting survey experiments. These problems will then be addressed in the analyses of our case studies.
Potential problems in survey experiments
When conducting survey experiments researchers need to address some challenges. One of the most relevant has to do with ‘noncompliance’ and its impact on internal validity (Druckman et al., Reference Druckman, Green, Kuklinski, Lupia, Druckman, Green, Kuklinski and Lupia2011). This problem occurs when the subjects assigned to a certain treatment (including control) do not receive it. This might take place as a result of either the respondent's active behaviour (e.g., not accomplishing a given task or dropping out during the survey) or an involuntary action (e.g., the participant receives a different treatment from that to which s/he was assigned or eventually s/he is not exposed to any stimulus).
To tackle active noncompliance, a researcher may evaluate participants' level of attention via the recorded duration of the experiment or the use of screening questions posed during the interview and asking respondents to select a certain response option to check for their cooperation (Berinsky et al., Reference Berinsky, Margolis and Sances2014). Lower attention, however, does not necessarily imply no treatment. An alternative to address this problem is to include ‘manipulation checks’, that is, additional questions placed at the end of the experiment to evaluate whether or not the subject received the treatment as intended. Overall, there is still a debate on the utility of these types of questions since the post-treatment exclusion of subjects with low levels of attention or failing to pass manipulation checks may add a bias rather than help the analyst establish the treatment's causal effect (Gerber et al., Reference Gerber, Arceneaux, Boudreau, Dowling, Hillygus, Palfrey, Biggers and Hendry2014; Mutz and Pemantle, Reference Mutz and Pemantle2015).
When noncompliance is passive and related to failure in random assignment, the researcher should carefully discuss the number of subjects initially eligible for the study, the size of groups assigned to a certain treatment, how many did not receive the planned intervention, how the statistical analysis was handled and if any subject was excluded after the experiment. Ideally, researchers should provide intent-to-treat analysis of outcome variables considering all subjects assigned to a group regardless of whether the treatment was assigned or not (Gerber et al., Reference Gerber, Arceneaux, Boudreau, Dowling, Hillygus, Palfrey, Biggers and Hendry2014).
Another important challenge to internal validity has to do with ‘treatment spill-over effects’ (Transue et al., Reference Transue, Lee and Aldrich2009), namely the possible contamination between treatments pertaining to different experiments included in the same survey. Given the greater complexity of modern surveys, it is always a good practice to randomise the order of experiments (if more than one is included) and evaluate a possible order effect when analysing data.
Turning to external validity, the first challenge deals with ‘sampling issues’. Available studies have not detected significant differences between experimental treatment effects (both ATE and CATE) obtained through non-probabilistic and representative population samples (e.g., Mullinix et al., Reference Mullinix, Leeper, Druckman and Freese2015; Coppock et al., Reference Coppock, Leeper and Mullinix2018). Still, it should be emphasised that PBSE are one of the most effective tools to combine causal inference and external validity. In this case, it is always recommended to report sample characteristics (Gerber et al., Reference Gerber, Arceneaux, Boudreau, Dowling, Hillygus, Palfrey, Biggers and Hendry2014) and, if applied, the employed weighting scheme.
Ultimately, external validity has also to do with the extent to which outcomes observed in an experiment resemble real-world situations. One common criticism raised towards laboratory and survey experiments concerns the lack of realism as they offer a stylised setting and treatments that are often deemed to mirror complex everyday situations only imperfectly (Sniderman, Reference Sniderman2018). In a survey context, moreover, the stimuli might be more easily received than in the real world where competing frames are present (Barabas and Jerit, Reference Barabas and Jerit2010). Thus, researchers should provide a justification of the stimuli and settings used in the experiment and carefully evaluate the results. Eventually, they might also try to validate them with similar situations in real-world environments (Hainmueller et al., Reference Hainmueller, Hangartner and Yamamoto2015). That said, and although not being exempt from limitations, survey experiments represent a useful tool to test theories about a broad range of political phenomena. As in the case of any other research endeavour, drafting, conducting and analysing an experiment is a difficult task of which each step should be discussed in detail and in accordance with shared standards (Gerber et al., Reference Gerber, Arceneaux, Boudreau, Dowling, Hillygus, Palfrey, Biggers and Hendry2014; Mutz and Pemantle, Reference Mutz and Pemantle2015).
A factorial experiment on preferences towards asylum seekers
Following the so-called refugee crisis, the issue of immigration has become increasingly relevant in Europe, contributing to the development of a climate of insecurity and cultural threat among the European publics (Basile and Olmastroni, Reference Basile and Olmastroni2020). Given its proximity to the Libyan coasts, the crisis has been even harsher in Italy. In the last few years, anti-immigrant sentiments have spread among citizens, with populist and right-wing parties often resorting to anti-Muslim rhetoric and capitalising on people's resentment (Guidi and Martini, Reference Guidi and Martini2019).
Yet, while Western citizens may tend to oppose more open immigration policies, some experimental studies show that they are far less reluctant to admit individual immigrants. This person-positivity bias seems to vary according to the immigrant's profile, with preferences over asylum-seekers structured by economic, humanitarian and ethnic concerns. Specifically, asylum seekers with a high-skill background (i.e., a better occupational standing) are more likely to be accepted than those with low-skill profiles (Iyengar et al., Reference Iyengar, Jackman, Messing, Valentino, Aalberg, Duch, Hahn, Soroka, Harell and Kobayashi2013). Immigrants who fear political prosecution are more likely to be favoured than those who move for economic reasons (Bansak et al., Reference Bansak, Hainmueller and Hangartner2016), whereas individuals from Muslim-majority countries would have less chances to see their asylum request accepted (Valentino et al., Reference Valentino, Soroka, Iyengar, Aalberg, Duch, Fraile, Hahn, Hansen, Harell, Helbling, Jackman and Kobayashi2019). Interestingly, leftists seem to be more sensitive to humanitarian vis-à-vis instrumental reasons and less concerned about immigrants' religious identity than their right-wing fellow citizens (Bansak et al., Reference Bansak, Hainmueller and Hangartner2016). Coming to the Italian context, experiments covering this topic are rare, with exceptions showing a link between ideology and party alignments, on the one hand, and partisan cue-taking and ethnic prejudice, on the other (Barisione, Reference Barisione2020).
Thus, one factorial experiment might help disentangle what factors matter the most for asylum-seeker acceptance, whether there is an interplay among instrumental, humanitarian and ethnic considerations, and to what extent, if any, political ideology moderates their relationships. Although the following example is merely illustrative, available research (Bansak et al., Reference Bansak, Hainmueller and Hangartner2016; Barisione, Reference Barisione2020) leads us to believe that Italians would look more favourably at asylum applications pursued by skilled immigrants rather than low-skilled ones (h1); by those escaping war as compared to those coming for economic opportunities (h2); or by subjects from Christian-majority countries against Muslim-majority ones (h3).
We also can assume that the applicant's skills and ethnicity might moderate the differential effect of the motivation for migrating, so that the gap in the approval of an asylum application presented by an individual escaping from war and one looking for better economic conditions would be reduced when the subject is skilled and from a Christian-majority country (h4).
Last, we might expect instrumental, humanitarian and ethnic concerns to hinge on the ideology of the respondent, such that the approval gap between skilled and non-skilled migrants will be larger among right-wing participants than among left-wing ones (h5a); the approval gap between migrants coming for economic and humanitarian reasons will be smaller among right-wing voters compared to left-wing ones (h5b); and the approval gap between migrants coming from Christian-majority vis-à-vis Muslim-majority countries will be larger among right-wing respondents compared to left-wing ones (h5c).
Data
The experiment we consider in this section was embedded in the second wave of the EUENGAGE online panel survey, conducted between 6 July and 6 October 2017. Respondents were approached through an opt-in online panel provided by Research Now using quota sampling to reflect the general population's characteristics (see Appendix A). While the whole sample includes individuals from 10 EU member states, our analysis is limited to the Italian sample (n = 1278). Since weighting non-probability samples may be a problematic task (Mullinix et al., Reference Mullinix, Leeper, Druckman and Freese2015), we decided to present analysis on unweighted data, thus focusing on causal relationships without any claim of representativeness or generalisability.
Vignette
Respondents began the experiment by reading a short introduction about an asylum applicant interested in migrating to Italy. Then, each interviewee was invited to examine his background along with a picture of the applicant. While the picture remained constant, subjects were randomly assigned to one of the eight different experimental groups resulting from the combination of three treatments. Figure 2 shows one possible vignette generated through the random assignment of the examined conditions (the full protocol is in Appendix B).
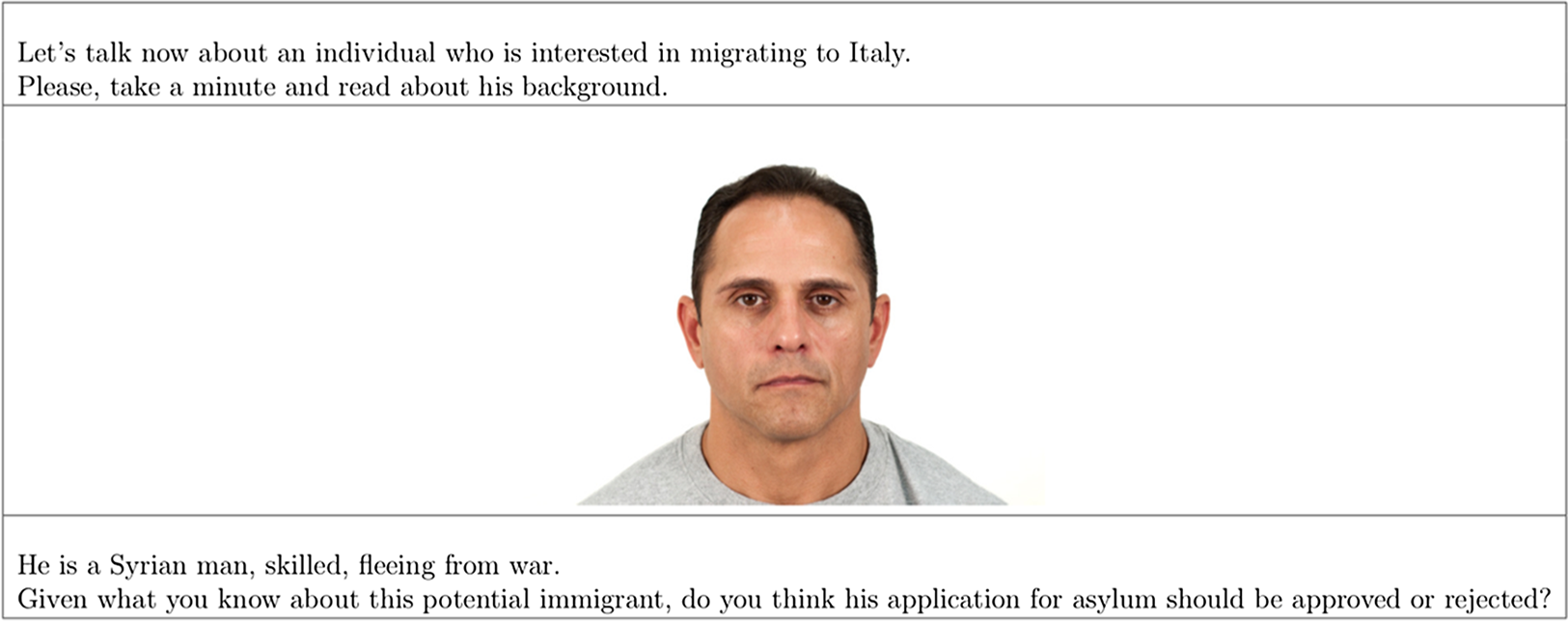
Figure 2. Stimuli: an illustration of the factorial experiment.
The picture comes from the Chicago Face Database (Ma et al., Reference Ma, Correll and Wittenbrink2015), a free source of high-resolution pictures standardised and validated via subjective evaluations and objective physical measurements. In our case, the results of subjective ratings classify the picture as a man, rated between Latino and white ethnic origin, aged around 43 years, with a neutral expression. The use of a fixed picture contributed to increase the credibility of the task while holding a broad range of conditions (gender, age and emotional facial expressions) constant across all respondents.
The experiment manipulated three conditions, providing us with a 2 × 2 × 2 full factorial design in which each hypothetical scenario presented the respondents with varying information about the migrant's qualification (low skilled or skilled), the reasons for leaving his country (looking for job or fleeing from war) and his ethnic origins (Syrian or Ukrainian). Differently from Iyengar et al. (Reference Iyengar, Jackman, Messing, Valentino, Aalberg, Duch, Hahn, Soroka, Harell and Kobayashi2013) and Valentino et al. (Reference Valentino, Soroka, Iyengar, Aalberg, Duch, Fraile, Hahn, Hansen, Harell, Helbling, Jackman and Kobayashi2019), the vignette did not specify what types of skills the applicant had, while it introduced the reason for migrating. When it comes to the country of origin, the vignette included two ethnic groups among which we might have asylum applicants, Syria and Ukraine being two contexts of conflict at the time of fieldwork. Moreover, the former constitutes a Muslim-majority country while the latter a Christian-majority one. Last, Ukrainians are a more familiar type of foreign immigrants in Italy, being the 5th most represented group out of 169 nationalities present in the country, than Syrians, who rank 69th (ISTAT 2017). This should elicit different degrees of cultural contrast between the two groups (higher for Syrians and lower for Ukrainians). Table 1 summarises all the experimental conditions and lists the number of respondents assigned to each group. Finally, after reading the scenario, the respondents had to express whether the migrant's application for asylum ought to be approved or rejected, so answers were collected in a dichotomous format, mimicking a real-world choice by a public official.
Table 1. Randomly assigned conditions in the factorial experiment
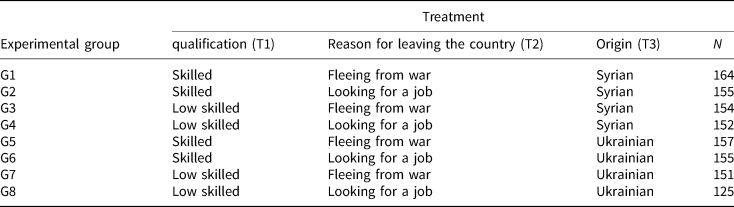
To check for the robustness of the random assignment, we performed balance tests by multinomial regression, regressing assignment to a certain experimental group on a set of socio-demographic characteristics (gender, age, educational attainment). Moreover, since our experiment involves the respondents' reaction to the admission of asylum seekers, we also checked for balance in ideology, party identity and attitudes towards immigration. The results confirm that the random procedure was correct with no variable being statistically different across the treatment groups (see Appendix C). Hence, any difference between conditions should be attributed to treatment manipulation only and not to other confounding factors.
It has to be noticed that this was not the only experiment included in the survey. In fact, other two experiments on the topics of the economy and globalisation were present, implying potential spill-overs among the three. However, the experiments were presented in a randomised order, a procedure that, as discussed above, can alleviate this type of bias.
Empirical analysis
To begin, it is worth mentioning that the general approval rate of the proposed asylum application is fairly high, with around 63% of respondents willing to accept the assigned request. This result aligns with studies conducted in other countries (Iyengar et al., Reference Iyengar, Jackman, Messing, Valentino, Aalberg, Duch, Hahn, Soroka, Harell and Kobayashi2013; Valentino et al., Reference Valentino, Soroka, Iyengar, Aalberg, Duch, Fraile, Hahn, Hansen, Harell, Helbling, Jackman and Kobayashi2019), though we take it with caution since it comes from a non-representative sample.
Given that our dependent variable is dichotomous in format, we estimated a logistic regression model. By reason of the experimental setting, we do not need to build a complex model with a battery of control variables. Rather, we identify the effects of our treatments by plugging dummy variables in as well as their interactions. For the sake of simplicity, we display results showing predicted probabilities of approval of asylum applications as a function of our covariates. To get the ATEs, we computed average marginal effects and performed Wald tests (formal notations and full models are reported in Appendix D).
We start by considering the main effect of our three treatments as they stand alone. It should be noted that approval clearly depends on profile features, confirming our first expectation (h1). Specifically, skilled applicants have 18% more probability to get accepted than non-skilled applicants (χ 2 = 45.45; P < 0.001). Similarly, people fleeing from war have 17% more probability to see their application approved than individuals coming to Italy to find a job, thus corroborating our second anticipation (h2) (χ 2 = 43.37; P < 0.001). Last, contrary to our third expectation (h3), a Syrian applicant has higher chances to be granted asylum, though the difference is small and non-significant (5% difference; χ 2 = 3.38; P = 0.065) (for a graphical representation, see Appendix D).
However, each of these values constitutes the effect of a single treatment on the dependent variable averaged across the levels of the other experimental conditions. The second step of our analysis is to explore the interplay among our three independent variables. The idea, here, is to test whether the gap in the approval rate between applicants escaping from war and those looking for better economic conditions is reduced when the applicant is skilled and from a Christian-majority country. Figure 3 shows the results of our model plotting the effects of two conditions – the level of qualification and the reason for leaving the country – and split them into two panels, depending on whether the potential refugee is from Syria (left panel) or from Ukraine (right panel).
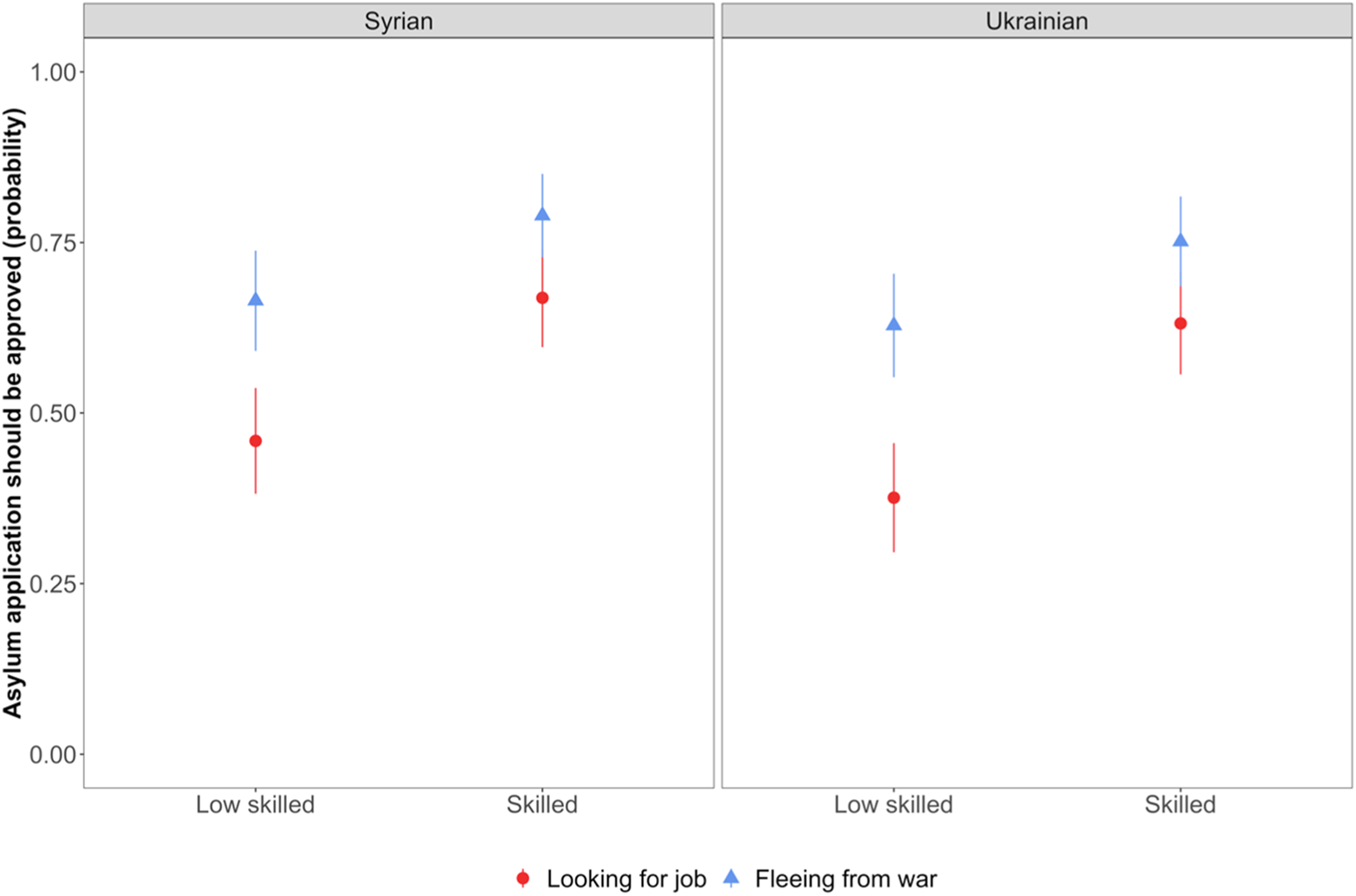
Figure 3. The effect of skills and reasons for leaving the country on the probability to accept asylum applications by immigrant's ethnic group.
Note: Graph shows predicted probabilities based on logit regression. Lines on both sides of the points represent 95% confidence intervals.
In the case of a Syrian applicant, we do not find a statistically significant interaction effect between skills and motivation for migrating. A detailed examination of the predicted probabilities from the combination of treatment conditions reveals that approval rates are always higher when the applicant has a humanitarian reason for requesting asylum (fleeing from war) compared to those who come for economic opportunities (looking for job). This is so for an unskilled applicant escaping from a war context, who has 20 percentage points (χ 2 = 14.20; P < 0.001) more probability to get a final approval than a similar unskilled applicant looking for a new job position. Similarly, skilled applicants fleeing from a conflict have more chances of being approved than skilled individuals who are job seeking (12% difference; χ 2 = 6.26; P < 0.05). Moreover, if we keep the reason of moving for economic opportunities as a reference and compare low skilled and skilled individuals, this increases the probability of the latter of being accepted (21% higher for skilled refugees; χ 2 = 15.04; P < 0.001); yet, not enough to close the gap with skilled subjects coming for political reasons.
Turning to the case of an Ukrainian applicant, we find the same pattern, meaning that we do not find an interaction between qualification and reason for leaving the country, a result that also extends to the ethnic origins.Footnote 5 Shortly, in contrast with our fourth expectation (h4), we might say that skills temper the effect of migrating for economic opportunities; however, this effect is not strong enough to significantly reduce the gap in the acceptance rate of skilled applicants moving for humanitarian reasons.
We conclude by examining whether the effects of the applicant's skills, reason for migrating and ethnicity are moderated by the ideology of the respondent. Figure 4 displays the results of a two-way interaction model between our treatments and the ideological positioning of the respondent. As we can see, although left-wing participants tend to express higher levels of approval than right-wing respondents, we do not find different patterns for ideology. In fact, when the applicant is either skilled or migrates for humanitarian reasons, approval rates improve to the same degree across ideological groups, so we do not detect any larger or smaller gap in these conditions depending on ideology, rejecting our expectations (h5a, h5b). When it comes to the ethnicity of the applicant, in contrast with our last hypothesis (h5c), rightists do not seem to be more sensitive to the Muslim−Christian divide as far as the two selected national groups are concerned.Footnote 6
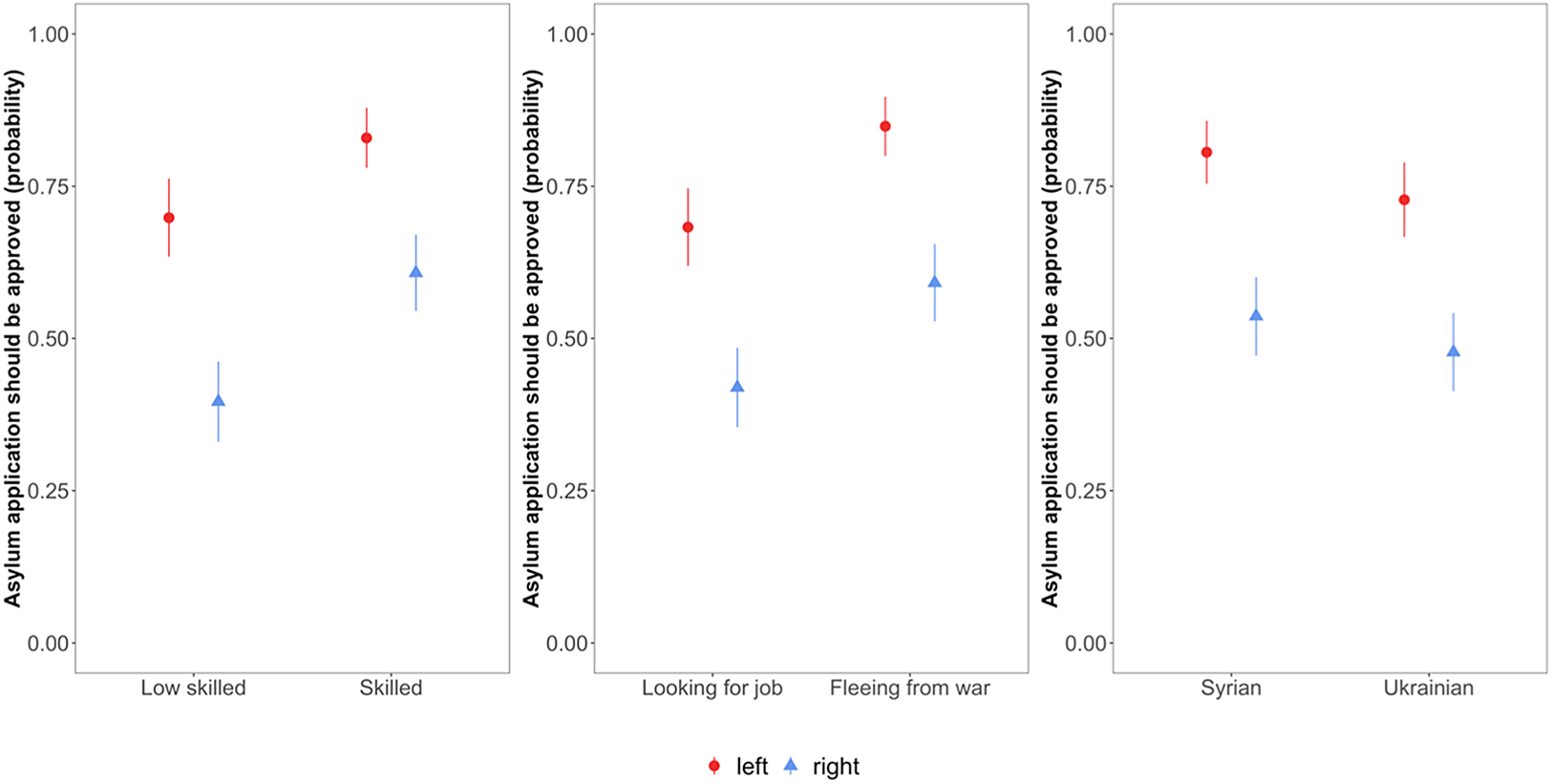
Figure 4. The effect of skills and reasons for leaving the country on the probability to accept asylum applications by respondent's ideology.
Note: Graph shows predicted probabilities based on logit regression. Lines on both sides of the points represent 95% confidence intervals.
To sum up, in line with previous studies (Iyengar et al., Reference Iyengar, Jackman, Messing, Valentino, Aalberg, Duch, Hahn, Soroka, Harell and Kobayashi2013; Bansak et al., Reference Bansak, Hainmueller and Hangartner2016; Valentino et al., Reference Valentino, Soroka, Iyengar, Aalberg, Duch, Fraile, Hahn, Hansen, Harell, Helbling, Jackman and Kobayashi2019), Italians take into account both instrumental and humanitarian motives when asked about specific individual applications for asylum. However, these factors do not seem to interact among each other, neither they do with the migrant's ethnic origins, which, at least in our experiment, turn out being irrelevant. Nor we do find an interaction between ideology and reasons behind approving a hypothetical application for asylum, so future research should be conducted on this link. Last, our results hold to some robustness checks (e.g., removal of speeders, weighting, controlling for possible spill-over effects among experiments in the same survey; see Appendix D).
A conjoint analysis on candidate preference
Personalisation of politics, that is, the gradual shift in the electorate's attention from political parties and issues to specific candidate features, has been the result of broad underlying social and political processes, including the individualisation of social life, the de-freezing of traditional cleavages and the emergence of parties as campaign organisations in a new media environment (Costa Lobo and Curtice, Reference Costa Lobo and Curtice2015). These patterns have prompted observational research to look at the role of candidate features to explain voting choices, unravelling the importance of some basic traits, among which: competence (being intelligent and knowledgeable), leadership (being inspiring), integrity (being honest) and empathy (being compassionate and caring) (Pancer et al., Reference Pancer, Brown and Barr1999).
The topic has become even more relevant with the success of (neo-)populist parties and leaders. In the ideal type, populist voters would attribute larger importance to valence issues (e.g., corruption) (Curini, Reference Curini2018), oppose professional politicians (Akkerman et al., Reference Akkerman, Mudde and Zaslove2014), favour candidates who act as ‘delegates’ (who care only about the interests of her/his electorate) rather than ‘trustees’ (who are independent and care about the interests of the nation), and, finally, advocate strong leadership (Caramani, Reference Caramani2017).
However, existing research needs to address some relevant problems. First, standard survey measures are prone to social desirability bias, with respondents likely to score as important all the above-mentioned personality traits. Second, interviewees are usually asked to rate each trait individually and not to evaluate candidate profiles characterised by both more and less positive features. In fact, politicians' profiles are multidimensional in nature and that explains the proliferation of conjoint analysis on candidate preferences (e.g., Teele et al., Reference Teele, Kalla and Rosenbluth2018; Franchino and Zucchini, Reference Franchino and Zucchini2015; for a critical view, see Incerti, Reference Incerti2020).
Still, none of the available studies have analysed extensively the role of personality or valence traits suggested by observational research.Footnote 7 Therefore, one possible conjoint experiment on the topic could explore what personality traits make a politician a good candidate in the eyes of the citizens and whether the importance of these traits vary across subgroups of voters based on their populist attitudes. Drawing on the available theoretical and empirical research, we can develop some expectations.
First, we might expect Italians to rate more favourably candidates who have higher levels of competence than those who have less (h1); who show higher moral integrity than apparently dishonest candidates (h2); who are more compassionate than cold and distant (h3). Second, we anticipate populist attitudes to moderate the importance of some traits on candidate favourability. Specifically, we hypothesise populist citizens to dislike professional politicians more than non-populists (h4a). Moreover, as compared to non-populist voters, populists will more likely favour candidates with high moral integrity (h4b), strong leadership (h4c) and acting as delegates (h4d).
Data
Our data come from a panel survey carried out by the Department of Social, Political and Cognitive Sciences at the University of Siena and collected on a sample of the Italian population aged 14 years or older selected within a probability panel held and managed by GfK Italy. The first wave of the survey (n = 3411) was conducted between 6 and 25 May, 2019, right before the last European elections and included the key covariate used in this example, that is, a scale eliciting populist attitudes. The second wave (n = 3179) was administered in the post-electoral period, from 28 May to 26 June 2019, and contained both the populist scale and the conjoint experiment. We restrict our analysis to subjects aged 18 years or over at the time of the interview (n = 3096). When conducting sub-group analysis on populism, we primarily use the populist scale included in the second wave, conducting some robustness tests with the first-wave measure and concentrating on the adult respondents who participated in both waves. Since we have a probability sample resembling the general population on several socio-demographic features – albeit more skewed towards the highly educated (see Appendix C) – we have decided to run models on weighted data.
Vignette
To minimise the effect of response fatigue on the quality of our experimental data and eliminate potential spill-over effects, the conjoint experiment was embedded at the beginning of the questionnaire after a few introductory questions. This is a forced, paired-choice, fully randomised conjoint with discrete and rating choices. Respondents began the experiment by reading a short introduction in which they were invited to reflect about ‘the characteristics a candidate should have to enter politics at the European level’, and then informed that they would have been provided ‘with several pieces of information about people who might have run for the European elections’. Then, for each pair of hypothetical politicians, participants were asked ‘which of the two candidates would you personally have preferred to win a seat in the European Parliament’. The experiment builds on the one proposed by Hainmueller et al. (Reference Hainmueller, Hopkins and Yamamoto2013). Figure 5 shows one possible conjoint vignette generated through random assignment to the considered macro-traits and relative attributes (for the full stimuli, see Appendix B).
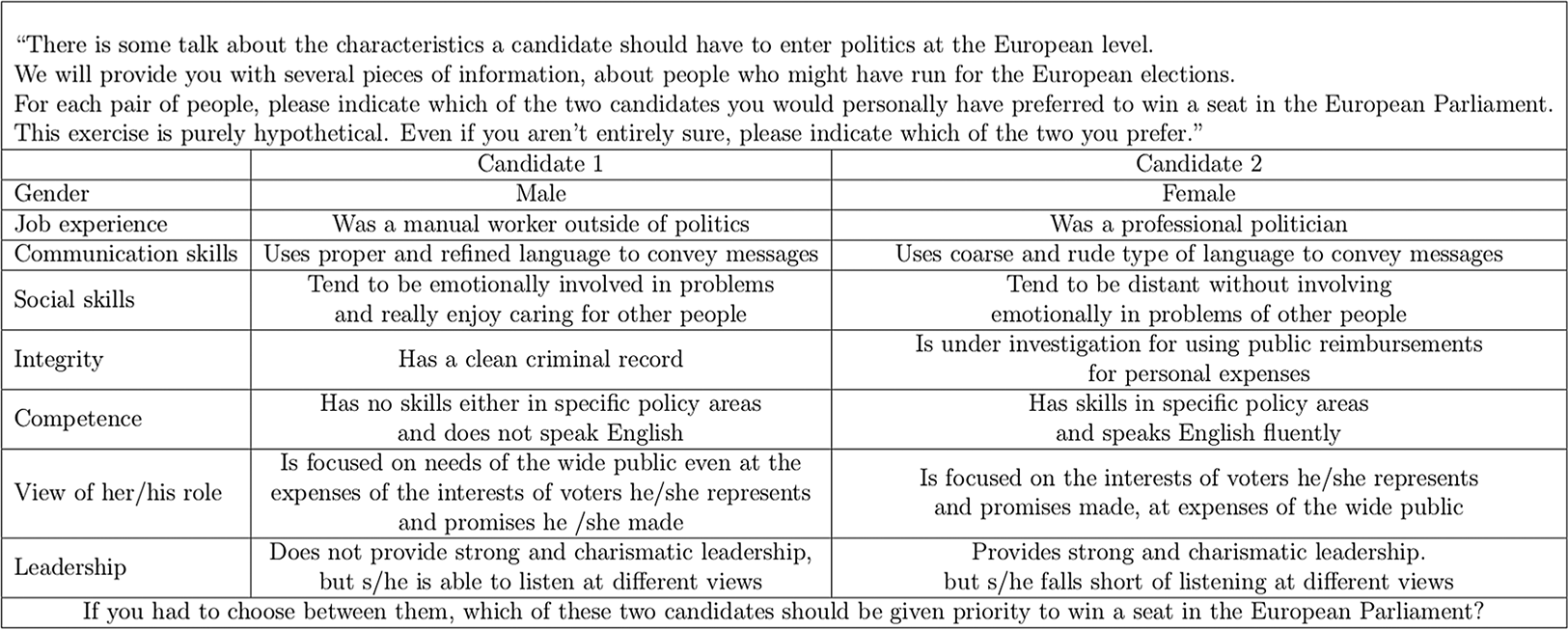
Figure 5. Stimuli: an illustration of the conjoint experiment.
Since respondents had to choose either one or the other candidate, the outcome variable is dichotomous, coming close to a real-world situation in European elections, at least in Italy, in which voters can express a preference among a list of pre-selected candidates. After this choice, participants were also asked to rate each profile on a 7-point favourability scale.
Overall, we manipulated eight macro-traits, implying that our hypotheses are tested in a broad context of candidate features. Moreover, each respondent was exposed to two pairs of candidates, therefore facing the same task twice. Two traits elicited basic sociodemographic characteristics, such as the role of gender and job position. Five traits considered personality features and skills, namely: communication skills, social skills, integrity, competence and leadership. The remaining trait elicited view of role (see Appendix B for the full list of attributes).
Profiles were generated so that the order of macro-traits was randomised and fixed across the two pairings to minimise recency effect and priming. The assignment of attributes, instead, followed an independent fully randomised approach, meaning that all attributes were randomly assigned without restrictions on their possible combination. To check for the correct implementation of the experiment, we first analysed the distribution of considered attributes in the sample – results ensure fair distribution – and then tested for balance in our main covariate (the populist scale) – results suggest that imbalance should not be a matter of concern (see Appendix B).
Empirical analysis
In our experiment, 2676 respondents rated 10,704 profiles (5352 pairings), with a design yielding 1536 possible profile combinations. In conjoint analysis, as mentioned above, the causal quantity of interest is the average marginal component effect (henceforth AMCE). In our example, this corresponds to the average difference in the probability effect of being preferred for winning a seat in the European Parliament when comparing two different attribute levels – e.g., a candidate with a ‘clean criminal record’ versus a candidate being ‘under investigation’ – while keeping all other attributes constant. Since attributes are randomised, profiles with a ‘clean criminal record’ will have, on average, the same distribution on all other attributes as compared to profiles ‘under investigation’ (as we positively tested). In the subsequent analysis, the dependent variable will be a dichotomous variable measuring people's choice.
Following Hainmueller et al. (Reference Hainmueller, Hopkins and Yamamoto2013), we estimated a linear probability model to assess the role of the different profile traits and the relative assigned attributes on people's candidate choice. In this case, the explanatory variables are a series of dummies for each of the attributes of the macro-traits under consideration. Since each participant carried out two different tasks, observations are not independent, so we clustered standard errors by respondent. As said, AMCE conveys information on the marginal causal effect of an attribute against a reference category. Following Leeper et al. (Reference Leeper, Hobolt and Tilley2019), we also computed unadjusted marginal means (henceforth MM) to give a more detailed description of the respondents' preference for all feature levels. Again, we will only show graphical results to ease interpretation (see Appendix D for full models).
Which of the considered traits does meet the favourability of the respondents, increasing the probability of choosing a certain candidate? Figure 6 shows the AMCE of each attribute against the baseline, so that when the point estimate and confidence intervals cross the zero line, the attribute has no effect. When the coefficient leans towards the right, taking positive values, it produces a positive change in the probability of choosing a certain candidate. On the contrary, when the coefficient leans towards the left, taking negative values, it yields a negative change in the probability. As can be seen, being a woman does not exert an effect compared to being a man. Conversely, many job positions outside politics – manual worker ( + 6%, P < 0.01), engineer ( + 5%, P < 0.01), university professor ( + 4%, P < 0.01) – increase the probability of being chosen if compared with a professional politician. This would confirm a negative bias towards a long-term political career.
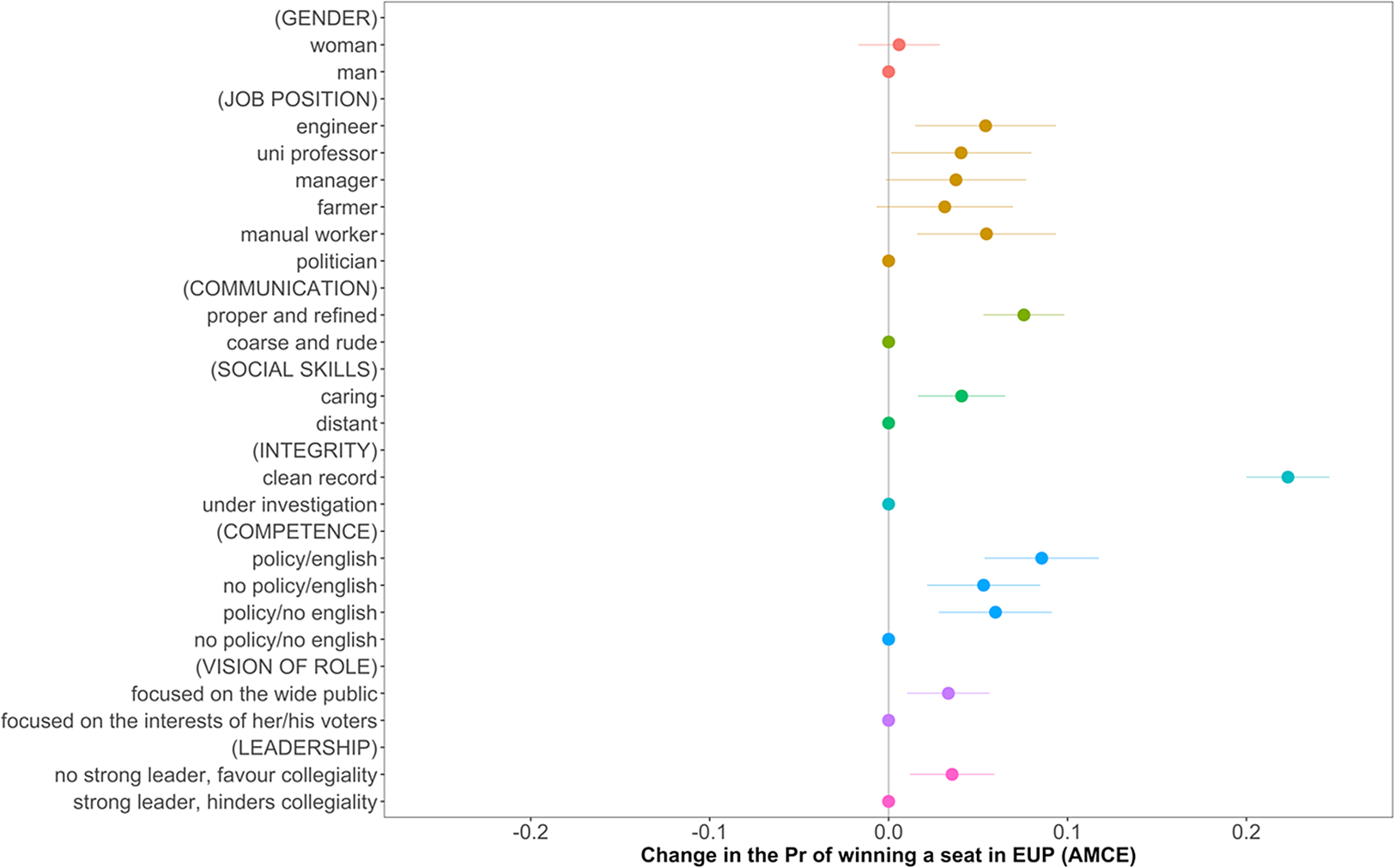
Figure 6. Average marginal component effect: effects of candidate traits on preference for election.
Note: Lines on both sides of the points represent 95% confidence intervals. The points without horizontal bars denote the attribute value used as a reference category.
Considering the way a candidate might conceive her/his role, being a trustee, who is focused on the national interest, seems to be favoured over a candidate who interprets the role as a delegate focused on the mere interest of her/his voters ( + 3%, P < 0.01). Similarly, a candidate who favours collegiality is preferred over a strong leader ( + 4%, P < 0.01), and, reasonably, proper and refined communication skills exert a positive effect on candidate selection ( + 8%, P < 0.001).
Coming to the traits on which our attention is focused, competence increases the probability of being favoured as compared to less expertise in specific policies or fluency in English ( + 6%, P < 0.001). Moreover, caring about the problems of other people and being emotionally involved exert a positive effect on candidate selection as compared to the baseline category for this trait ( + 4%, P < 0.01). Still, the most important trait by far is integrity, with candidates showing a clean criminal record being supported much more than those under investigation. The increase in probability is equal to 22 percentage points, a strong and statistically significant effect (P < 0.001). Overall, our first three expectations (h1, h2, h3) are corroborated, albeit with differences in the magnitude of effects. All these conclusions are largely substantiated by MM results (see Appendix D).
Now, are these results conditioned by individuals' populist attitudes? To evaluate this, we first need to distinguish our respondents according to their level of populism. To do this, we rely on a scale developed by Akkerman et al. (Reference Akkerman, Mudde and Zaslove2014) and derived from a six-item battery aimed at capturing a latent attitudinal dimension characterised by three main aspects: people-centrism, anti-elitism and Manichaeism. We tested it via factor analysis and computed factor scores to get a synthetic measure of populism (full results are reported in Appendix D). Then, we ran separate models for respondents who were either above or below the median value of the resulting populist score to gauge whether the effect of attributes changed according to their level of populism.
Figure 7 summarises the results in three panels. Moving from left to right, it displays the AMCEs of attributes when populism is high, low and the difference between the two, thus allowing to detect any subgroup difference. The first thing we can notice is that, if compared with non-populist respondents, populists seem to favour candidates with some job experience (especially farmers, manual workers) over professional politicians. Looking at MMs (Appendix D), however, this is not the product of a strong preference for working-class positions or manual jobs, but of a general disapproval of professional politicians. Specifically, populists tend to punish this type of candidates 7% more than non-populists (P < 0.01), confirming our hypothesis (h4a). Moreover, populists appear to be more sensitive to moral integrity, with a 7% increase in the probability of choosing a candidate with a clean criminal record over a candidate under investigation, compared to non-populists. Therefore, also in this case, our expectation is corroborated (h4b). On the other hand, against popular accounts (h4c and h4d), we do not find populists to prefer strong leaders or candidates who act as delegates.
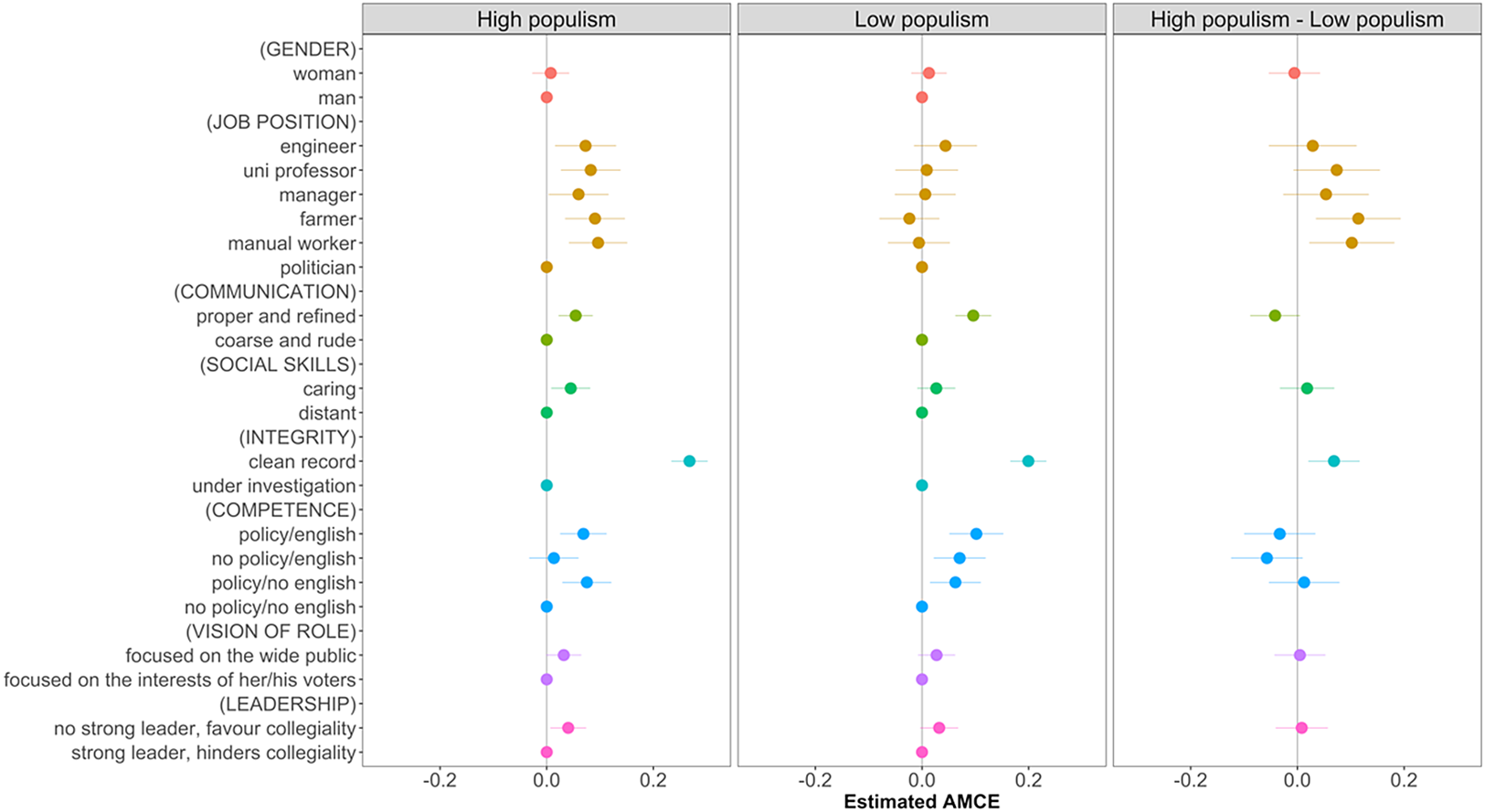
Figure 7. Average marginal component effect for populists and non-populists.
Note: Lines on both sides of the points represent 95% confidence intervals. The points without horizontal bars denote the attribute value used as a reference category.
We might conclude that citizens take into account personality traits eliciting valence features when evaluating candidate fit for elections. These have to do with communication and social skills, view of the role and leadership. There is an opposition towards professional politicians and, in line with previous research (Franchino and Zucchini, Reference Franchino and Zucchini2015), moral integrity is by far the most important aspect, with both results more pronounced in the case of populist respondents. A series of robustness checks confirm the reliability of our results (i.e., changing the way we measure the dependent variable, removing subjects depending on their level of attention during the survey, performing sub-group analysis using a measure of populism from the first survey wave, handling randomisation problems; see Appendix D).
Conclusions
The experimental approach constitutes the prime method in the quest for causality. Nowadays, political scientists willing to embark in experimental research may take advantage of a growing number of studies and choose the strategy that best fits their objectives among a wide menu of designs and settings. Of course, none of these options is free of limitation, so that doing experiments needs a good deal of creativity together with a deep awareness of the potential trade-offs between the control of the experimental setting and the external validity of the results.
This article has tried to give an overview of the basic concepts underneath the experimental method, highlighting the amount of scholarly interest in the field and possible designs to use. It has addressed the main differences among various experimental settings and discussed the main applications and potential problems in the use of survey experiments in particular. When combining randomised assignment and representative samples, survey experiments allow the researcher to make population inferences about causal relationships between variables of interest. Yet, survey experiments are not necessarily the final remedy for the study of causality and researchers should problematise each single step in their design, planning and implementation. For practical guidance, we have presented the full protocol for a traditional factorial design on individuals' attitudes towards migrants and a more innovative conjoint experiment on candidate preferences, including a set of research questions, the experimental stimuli used to address them, and the way to analyse experimental data.
The exposure and acquaintance of political scientists to experimental methods have gradually fuelled experimental publications, with a rapid spread in the number and influence of these manuscripts in the last few decades. We hope that this study, besides offering a general overview on the merit, success and use of experimental designs in international scholarly literature, will contribute to stimulate an increasing interest and usage of the experimental method among the Italian political science community. Bearing in mind the limitations that we have outlined, we can now take advantage of the possibility of experimentation in political research and shook off the idea that the study of politics is only an observational science.
Supplementary material
The supplementary material for this article can be found at https://doi.org/10.1017/ipo.2021.20
Funding
This research received financial support from the project ‘Bridging the gap between public opinion and European leadership: Engaging a dialogue on the future path of Europe EUENGAGE’ (H2020-EURO-2014-2015/H2020-EURO-SOCIETY-2014, Grant no. 649281) funded by the European Union's Horizon 2020 research and innovation programme (www.euengage.eu) and the Department of Excellence 2018-2022 (2272-2018-IA-PROFCMIUR001) (https://interdispoc.unisi.it/en/). No conflict of interest.
Data
The replication dataset is available at http://thedata.harvard.edu/dvn/dv/ipsr-risp
Acknowledgments
We thank James N. Druckman for having shared with us the list of experimental articles published by the American Political Science Review over the last six decades and for his encouragement and support in our comparative project on experimental research in Europe and the United States. We also thank Mattia Guidi and Thomas Leeper for their feedback on the analysis. Usual disclaimers apply.