No CrossRef data available.
Article contents
Interpreting validity evidence: It is time to end the horse race
Published online by Cambridge University Press: 31 August 2023
Abstract
An abstract is not available for this content so a preview has been provided. Please use the Get access link above for information on how to access this content.
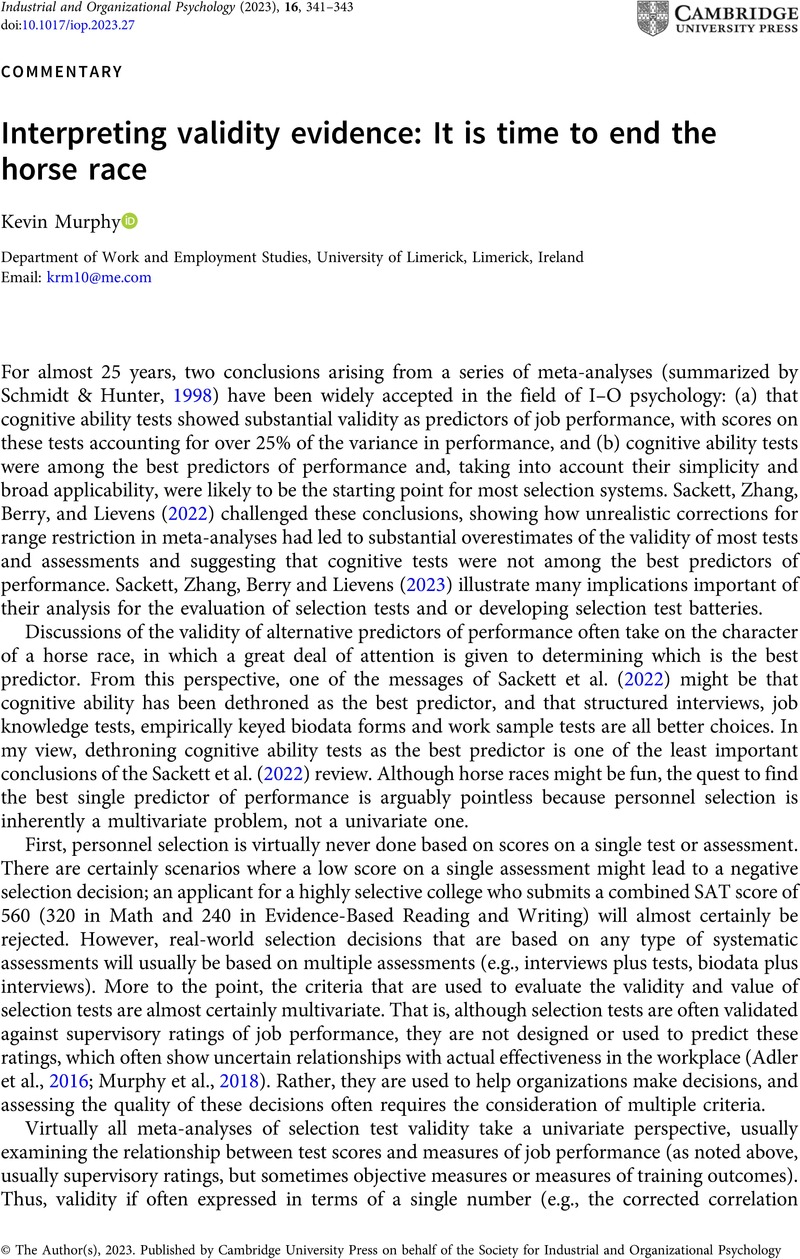
- Type
- Commentaries
- Information
- Copyright
- © The Author(s), 2023. Published by Cambridge University Press on behalf of the Society for Industrial and Organizational Psychology
References
Adler, S., Campion, M., Colquitt, A., Grubb, A., Murphy, K. R., Ollander-Krane, R., & Pulakos, E. D. (2016). Getting rid of performance ratings: Genius or folly. Industrial and Organizational Psychology: Perspectives on Science and Practice, 9, 219–252.CrossRefGoogle Scholar
Murphy, K., Cleveland, J. & Hanscom, M. (2018). Performance appraisal and management. Sage.Google Scholar
Murphy, K. R. (2019). Understanding how and why adding valid predictors can decrease the validity of selection composites: A generalization of Sackett, Dahlke, Shewach, and Kuncel (2017). International Journal of Selection and Assessment, 27, 249–255.CrossRefGoogle Scholar
Murphy, K. R. & Shiarella, A. (1997). Implications of the multidimensional nature of job performance for the validity of selection tests: Multivariate frameworks for studying test validity. Personnel Psychology, 50, 823–854.CrossRefGoogle Scholar
Sackett, P. R., Dahlke, J. A., Shewach, O. R., & Kuncel, N. R. (2017). Effects of predictor weighting methods on incremental validity. Journal of Applied Psychology, 102, 1421–1434.CrossRefGoogle ScholarPubMed
Sackett, P. R., Zhang, C., Berry, C. M., & Lievens, F. (2022). Revisiting meta-analytic estimates of validity in personnel selection: Addressing systematic overcorrection for restriction of range. Journal of Applied Psychology, 107, 2040–2068.CrossRefGoogle ScholarPubMed
Sackett, P. R., Zhang, C., Berry, C. M., & Lievens, F. (2023). Revisiting the design of selection systems in light of new findings regarding the validity of widely used predictors. Industrial and Organizational Psychology: Perspectives on Science and Practice, 16(3), 283–300.CrossRefGoogle Scholar
Schmidt, F. L., & Hunter, J. E. (1998). The validity and utility of selection methods in personnel psychology: Practical and theoretical implications of 85 years of research findings. Psychological Bulletin, 124(2), 262.CrossRefGoogle Scholar