No CrossRef data available.
Article contents
The importance of representativeness as well as timeliness in studying technology: Three additional suggestions
Published online by Cambridge University Press: 09 September 2022
Abstract
An abstract is not available for this content so a preview has been provided. Please use the Get access link above for information on how to access this content.
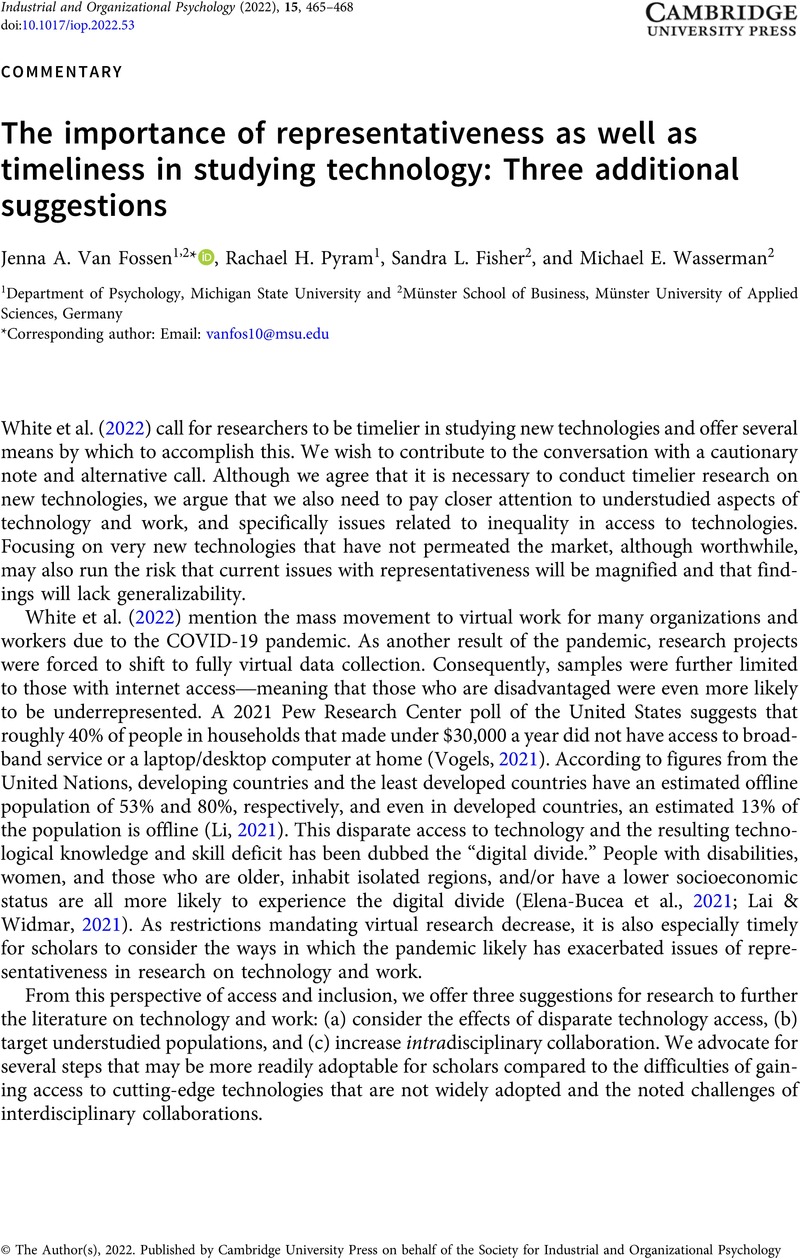
- Type
- Commentaries
- Information
- Copyright
- © The Author(s), 2022. Published by Cambridge University Press on behalf of the Society for Industrial and Organizational Psychology
References
Attaran, M., Attaran, S., & Kirkland, D. (2019). The need for digital workplace: Increasing workforce productivity in the information age. International Journal of Enterprise Information Systems, 15(1), 1–23.CrossRefGoogle Scholar
Balkmar, D., & Mellström, U. (2018). Masculinity and autonomous vehicles: Adegendered or resegregated future system of automobility? Transfers, 8(1), 44–63.CrossRefGoogle Scholar
Elena-Bucea, A., Cruz-Jesus, F., Oliveira, T., & Coelho, P. S. (2021). Assessing the role of age, education, gender and income on the digital divide: Evidence for the European Union. Information Systems Frontiers, 23(4), 1007–1021.CrossRefGoogle Scholar
Fisher, S. L., & Howardson, G. N. (2022). Fairness of artificial intelligence in human resources-held to a higher standard? In Strohmeier, Stefan (Ed.), Handbook of research on artificial intelligence in human resource management (pp. 303–322). Edward Elgar Publishing.Google Scholar
Harper, S., & Yesilada, Y. (2012). Emerging technologies and web accessibility: Research challenges and opportunities focusing on vision issues. Disability and Rehabilitation: Assistive Technology, 7(1), 1–19.Google Scholar
Jones, K. S., Newman, D. A., Su, R., & Rounds, J. (2022). Vocational interests and adverse impact: How attraction and selection on vocational interests relate to adverse impact potential. Journal of Applied Psychology, 107(4), 604–627.CrossRefGoogle ScholarPubMed
Lai, J., & Widmar, N. O. (2021). Revisiting the digital divide in the COVID-19 era. Applied Economic Perspectives and Policy, 43(1), 458–464.CrossRefGoogle ScholarPubMed
Li, C. (2021, October 11). Worsening global digital divide as the US and China continue zero-sum competitions. Brookings. https://www.brookings.edu/blog/order-from-chaos/2021/10/11/worsening-global-digital-divide-as-the-us-and-china-continue-zero-sum-competitions/
Google Scholar
Ng, T. W., & Feldman, D. C. (2012). Evaluating six common stereotypes about older workers with meta-analytical data. Personnel Psychology, 65(4), 821–858.CrossRefGoogle Scholar
Ravid, D. M., Tomczk, D. L., White, J. C., & Behrend, T. S. (2020). EPM 20/20: Areview, framework, and research agenda for electronic performance monitoring. Journal of Management, 46(1), 100–126.CrossRefGoogle Scholar
Tarafdar, M., Tu, Q., Ragu-Nathan, B. S., & Ragu-Nathan, T. S. (2007). The impact of technostress on role stress and productivity. Journal of Management Information Systems, 24(1), 301–328.CrossRefGoogle Scholar
U.S. Census Bureau. (2021, October 8). ASE tables. https://www.census.gov/programs-surveys/ase/data/tables.html
Google Scholar
Vogels, E. A. (2021, June 22). Digital divide persists even as Americans with lower incomes make gains in tech adoption. Pew Research Center. https://www.pewresearch.org/fact-tank/2021/06/22/digital-divide-persists-even-as-americans-with-lower-incomes-make-gains-in-tech-adoption/
Google Scholar
White, J., Ravid, D., Siderits, I., & Behrend, T. S. (2022). An urgent call for I-O psychologists to produce timelier technology research. Industrial and Organizational Psychology: Perspectives on Science and Practice, 15(3), 441–459.CrossRefGoogle Scholar