Dietary exposure to xenobiotics in food and water
The gut microbiota maintains physiological processes that promote host health (Ayres, Reference Ayres2017). A typical vertebrate gastrointestinal tract contains an average of 1013 microbial cells and approximately 3,000 microbial species in total (Li et al., Reference Li, Ni, Li, Wang, Li, Wu, Zhang, Yu and Yan2014; Sender et al., Reference Sender, Fuchs and Milo2016). The microorganisms that inhabit the intestine are classified into four major groups (ie. archaea, bacteria, viruses, and fungi) and organised into functional communities that vary in density and structure along the digestive tract (Hiippala et al., Reference Hiippala, Jouhten, Ronkainen, Hartikainen, Kainulainen, Jalanka and Satokari2018; Milani et al., Reference Milani, Duranti, Bottacini, Casey, Turroni, Mahony, Belzer, Palacio, Montes, Mancabelli, Lugli, Rodriguez, Bode, de Vos, Gueimonde, Margolles, van Sinderen and Ventura2017). The variation among gut microbial communities could be associated to the function of the microbiota, which expresses more than 3.3 million genes related to metabolic regulation, biotransformation, nutrient absorption, molecule biosynthesis, and the modulation of immune responses and behaviour (Claus et al., Reference Claus, Guillou and Ellero-Simatos2016; Qin et al., Reference Qin, Li, Cai, Li, Zhu, Zhang, Liang, Zhang, Guan, Shen, Peng, Zhang, Jie, Wu, Qin, Xue, Li, Han, Lu, Wu, Dai, Sun, Li, Tang, Zhong, Li, Chen, Xu, Wang, Feng, Gong, Yu, Zhang, Zhang, Hansen, Sanchez, Raes, Falony, Okuda, Almeida, Le Chatelier, Renault, Pons, Batto, Zhang, Chen, Yang, Zheng, Li, Yang, Wang, Ehrlich, Nielsen, Pedersen, Kristiansen and Wang2012). Thus, gut microbiota functions are fundamental to the well-being of host organisms, yet all microbial communities are unique. This uniqueness is established from birth and can be altered through life due to the diet, immune system, genotype, age, and lifestyle of the host and by external factors such as habitat characteristics and xenobiotic exposure (Jin et al., Reference Jin, Wu, Zeng and Fu2017).
Xenobiotics are chemical substances of synthetic origin that are generally not present in the environment (Abdelsalam et al., Reference Abdelsalam, Ramadan, ElRakaiby and Aziz2020; Stefanac et al., Reference Stefanac, Grgas and Landeka Dragičević2021). However, this term is flexible and can also include endobiotics and other naturally occurring substances if their concentrations are higher than average (Soucek, Reference Soucek and Scwab2011). These compounds, such as environmental contaminants and pharmaceuticals, have been identified in various foods and interact with gut microbes (Garcia and Gotah, Reference Garcia and Gotah2017; Koppel et al., Reference Koppel, Maini Rekdal and Balskus2017). Introducing xenobiotics into the environment due to natural and anthropogenic processes has resulted in growing concerns regarding their impacts on human and animal health.
The levels of residues in food items are beyond the scope of this study but may affect consumers by inducing allergic reactions, hormonal imbalances, and bacterial resistance to antibiotics (Pugajeva et al., Reference Pugajeva, Ikkere, Judjallo and Bartkevics2019). Contaminated water also constitutes another source of xenobiotic exposure (Amoatey and Baawain, Reference Amoatey and Baawain2019). However, while the toxicity of xenobiotics and their effects on human health have been extensively reported (Rodríguez et al., Reference Rodríguez, Gallampois, Haglund, Timonen and Rowe2021), evidence indicates that the toxicity of non-target gut microbiota due to dietary exposure could also affect host health (Claus et al., Reference Claus, Guillou and Ellero-Simatos2016; Jin et al., Reference Jin, Wu, Zeng and Fu2017). Due to the conserved metabolic pathways among animal models, their use in toxicological studies allows us to extrapolate the measurable effects of xenobiotic exposure to humans (Hansen, Reference Hansen, Harris and Hansen2012). However, evaluations of the effects of xenobiotics on the intestinal microbiota must consider multiple factors, including the genetic individual variability of the host given that genetic variants and associated gene expression could control the composition of the gut microbiota (Bubier et al., Reference Bubier, Chesler and Weinstock2021). Therefore, using model species in pre-clinical trials that use translational approaches to evaluate xenobiotic effects in the gastrointestinal tract could provide novel insights due to the integration of pathologies (Johnson and Greenwood-Van Meerveld, Reference Johnson, Greenwood-Van Meerveld and Greenwood-Van Meerveld2017; Robinson et al., Reference Robinson, Krieger, Khan, Huffman, Chang, Naik, Yongle, Hameed, Krieger, Girardi and Gaudino2019).
To summarise the known interactions between xenobiotics and the intestinal microbiota, we performed a systematic search in the PubMed database. Published articles were searched with the terms “effects,” “xenobiotics,” “gut microbiota,” “ecotoxicology,” “microbiota,” and “metagenomics” in different combinations. In all, 672 results were returned. Experimental approaches are required to assess possible changes in the composition and function of the intestinal microbiota (Franklin and Ericsson, Reference Franklin and Ericsson2017; Hörmannsperger et al., Reference Hörmannsperger, Schaubeck and Haller2015) and microbiota dynamics (Ranjan et al., Reference Ranjan, Rani, Metwally, McGee and Perkins2016). Thus, we selected the 77 papers encompassing experimental exposure assessments, of which 45 articles deal exclusively with dietary-oral exposure and gut microbiota assessments (Table 1). Our search indicated that although the effects of xenobiotics are diverse, dysbiosis patterns, which are recurring changes in particular microbial groups due to exposure to xenobiotics, are present that may be related to the experimental organism and its core microbiota (Figure 1) such as (1) gut dysbiosis in the murine model, (2) gut dysbiosis in pollinators, (3) gut dysbiosis in fish models (eg. zebrafish and tilapia), and (4) some relevant cases of gut dysbiosis in poultry and insects. Moreover, when analysing studies with different experimental models, similar dysbiosis patterns were revealed that were mainly described using the 16S sequencing approach. In general, if these patterns are permanent or persist for extended periods, they may correlate with pathologies such as metabolic syndrome and obesity (Kocełak et al., Reference Kocełak, Zak-Gołąb, Zahorska-Markiewicz, Aptekorz, Zientara, Martirosian, Chudek and Olszanecka-Glinianowicz2013; Turnbaugh et al., Reference Turnbaugh, Ley, Mahowald, Magrini, Mardis and Gordon2006; Verdam et al., Reference Verdam, Fuentes, de Jonge, Zoetendal, Erbil, Greve, Buurman, de Vos and Rensen2013) and cardiovascular (Lam et al., Reference Lam, Su, Koprowski, Hsu, Tweddell, Rafiee, Gross, Salzman and Baker2012, Reference Lam, Su, Hsu, Gross, Salzman and Baker2016) and gastrointestinal diseases (Becker et al., Reference Becker, Neurath and Wirtz2015; Gevers et al., Reference Gevers, Kugathasan, Denson, Vázquez-Baeza, Van Treuren, Ren, Schwager, Knights, Song, Yassour, Morgan, Kostic, Luo, González, McDonald, Haberman, Walters, Baker, Rosh, Stephens, Heyman, Markowitz, Baldassano, Griffiths, Sylvester, Mack, Kim, Crandall, Hyams, Huttenhower, Knight and Xavier2014). However, microbial communities have an intrinsic ability to adapt to adverse conditions and recover without severe consequences to the host (Weiss and Hennet, Reference Weiss and Hennet2017). In this regard, research on dietary xenobiotic exposure is highly relevant due to the underlying relationship between the gut microbiota and host health (Jin et al., Reference Jin, Wu, Zeng and Fu2017).
Table 1. Overview of the main features of the studies that have evaluated gut dysbiosis due to xenobiotic dietary-oral exposure.

Abbreviations: C, chronic; ERC, environmentally realistic concentrations; GBI, gut barrier integrity; HH, host health; LS, long-term study; m, mouse; Me, metabolites; Mi, microbiota; NEW, newly emerged workers; NPs, nanoparticles; r, rat; RS, recovery study; SC, sub-chronic; SS, short-term study; W, worker bees.
* Other microbial groups changes were found.

Figure 1 Relative frequencies of 45 studies with dietary-oral exposure and gut microbiota evaluations published between 2013 and 2022. Information on the kind of experimental organism, methodological approach to the microbiota evaluation, main bacterial groups that are affected after exposure, range of experimental concentrations (ER Concentration [environmentally realistic concentration] vs. Hi Concentration [high concentration when assessing action modes]), and evaluations of host health (HH Evaluation [host health evaluation] vs. Mi Evaluation [microbiota evaluation only]). The figure was made with sankeymatic.
Dysbiosis and gut physiology
A well-balanced gut ecosystem promotes feedback among the microbial species that reside within, which in turn regulates mucous epithelial immunity. For instance, anti-bacterial peptides secreted by mucous membranes influence the composition of gut microbiota, and this communication is essential to maintain the integrity of the epithelial barrier and the immunological niche. However, xenobiotics and other antigens in food may interact with epithelial mucous and trigger pathological states associated with dysbiosis, which is defined as the loss of microbiota homeostasis that is characteristic of inflammatory, metabolic, neurological, and autoimmune diseases and disorders (DeGruttola et al., Reference DeGruttola, Low, Mizoguchi and Mizoguchi2016). Changes in feedback processes may or may not induce inflammatory responses, although they can modify the permeability of the epithelial barrier and permit the translocation of bacteria from the intestinal lumen to the circulatory system. This disrupts cytokine production, T cell pro-inflammatory proliferation, and T cell anti-inflammatory inhibition, which provokes intestinal immunological niche dysfunction that ultimately results in a chronic inflammatory state within the host (Pagliari et al., Reference Pagliari, Gambassi, Piccirillo and Cianci2017; Tilg et al., Reference Tilg, Zmora, Adolph and Elinav2020).
Although epithelium-microbiota interactions that maintain homeostasis are highly complex, exposure to xenobiotics can result in distinctive dysbiotic patterns (Figure 2a). For instance, a decrease in the abundance of Bifidobacterium spp. and Firmicutes bacteria, particularly those of the Clostridiaceae family, precedes inflammatory conditions and may possibly play an important role in the development of obesity due to the overexpression of pro-inflammatory cytokines, which interrupts insulin signalling (Malaisé et al., Reference Malaisé, Menard, Cartier, Gaultier, Lasserre, Lencina, Harkat, Geoffre, Lakhal, Castan, Olier, Houdeau and Guzylack-Piriou2017). These alterations lead to the reduction of anti-microbial activities and the disruption of IgA synthesis, a critical component that supports the integrity of the epithelial barrier. Additionally, xenobiotic-induced changes have been reported in the abundance of butyrate-producing bacteria, which are considered to be energy sources for epithelial cells in the colon that promote proliferation and differentiation (Weiss and Hennet, Reference Weiss and Hennet2017). In this regard, members of the Lactobacillaceae family are reportedly the most affected by exposure to benzo-ɑ-pyrene, glyphosate, and arsenic (Aitbali et al., Reference Aitbali, Ba-M’hamed, Elhidar, Nafis, Soraa and Bennis2018; Gaulke et al., Reference Gaulke, Rolshoven, Wong, Hudson, Ho and Sharpton2018; Ribière et al., Reference Ribière, Peyret, Parisot, Darcha, Déchelotte, Barnich, Peyretaillade and Boucher2016; Tang et al., Reference Tang, Tang, Ren and Li2020). Also, changes in Bacteroidetes and Proteobacteria (Zhou et al., Reference Zhou, Xu, Huang, Liu, Chen, Hu, Li, Liu and Guo2020) have also been associated with pro-inflammatory conditions that are similar to those observed with Paraprevotellaceae, Desulfovibrionaceae (Ribière et al., Reference Ribière, Peyret, Parisot, Darcha, Déchelotte, Barnich, Peyretaillade and Boucher2016), and Enterobacteriaceae (Stedtfeld et al., Reference Stedtfeld, Stedtfeld, Fader, Williams, Bhaduri, Quensen, Zacharewski, Tiedje and Hashsham2017). Of note, few studies incorporate evaluations of metabolite and gut barrier integrity changes, and most of these only discuss these effects (Table 1).
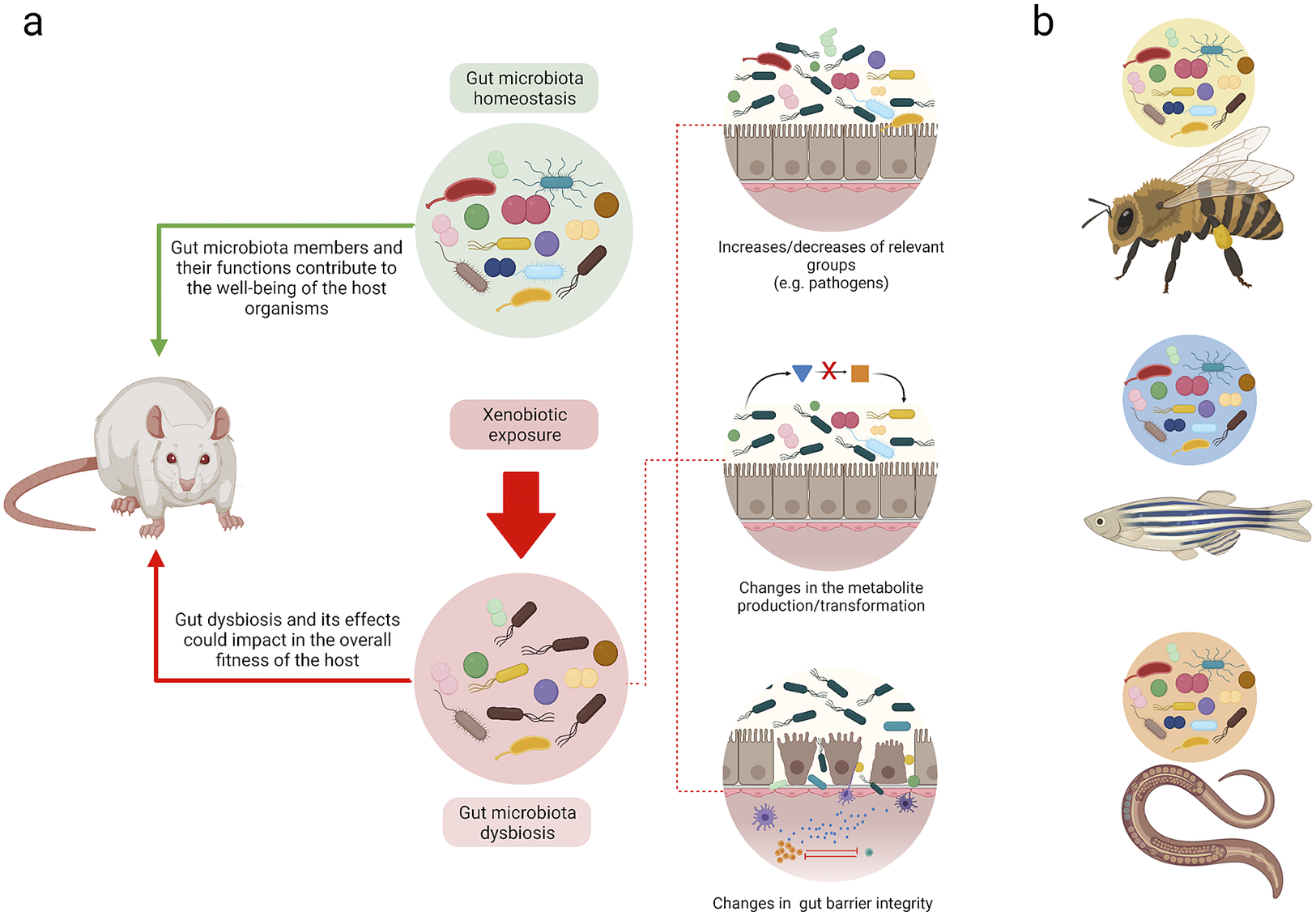
Figure 2 Understanding homeostasis and dysbiosis in different biological models. (a) Summary of the loss of gut homeostasis due to xenobiotic exposure. The feedback between the gut microbiota and host could promote host health. Alternatively, at the onset of dysbiosis due to xenobiotic exposure, different mechanisms may be elicited that may result in different outcomes that affect the host. (b) Although gut microbiota studies have been performed with different species, extrapolating the results among them is not viable. Each model has its own genetic, metabolic, and gut microbiome particularities. The figure was made with Biorender.
Changes in the intestinal architecture constitute a primary focus of multiple experiments that have aimed to evaluate the effects of dietary xenobiotics at a systemic level. In a murine model, the modification of intestinal structures was related to different inflammatory states that were the result of polynuclear and inflammatory cell (IL-6, IL-6ß) infiltration due to oral methylmercury exposure (Lin et al., Reference Lin, Zhao, Zhang, He, Wang, Chang, Cui, Gao, Li, Chen and Li2020). Furthermore, evidence of changes to epithelial tissues in regions of the small and large intestines have been reported with benzo[a]pyrene (Lin et al., Reference Lin, Zhao, Zhang, He, Wang, Chang, Cui, Gao, Li, Chen and Li2020; Ribière et al., Reference Ribière, Peyret, Parisot, Darcha, Déchelotte, Barnich, Peyretaillade and Boucher2016), in addition to reductions in villi height, modifications to the proportions between these structures, and changes in crypt depth due to glyphosate exposure (Tang et al., Reference Tang, Tang, Ren and Li2020). In the worst-case scenario, a full depletion of epithelial tissue has been reported (Ribière et al., Reference Ribière, Peyret, Parisot, Darcha, Déchelotte, Barnich, Peyretaillade and Boucher2016). In poultry exposed to mercuric chloride, a complete absence of villi in some intestinal regions and cell infiltrations in the stratrum propri zone associated with inflammatory effects have been found (Zhou et al., Reference Zhou, Xu, Huang, Liu, Chen, Hu, Li, Liu and Guo2020). In addition to the intestines, the kidneys and liver have been evaluated with histological analyses, and evidence of glyphosate dose-dependent lesions has been found in the latter (Mesnage et al., Reference Mesnage, Teixeira, Mandrioli, Falcioni, Ducarmon, Zwittink, Mazzacuva, Caldwell, Halket, Amiel, Panoff, Belpoggi and Antoniou2021). These effects are similar to those observed in other biological models. However, dysbiosis patterns are unique for each model due to genetic, metabolic, and gut niche particularities (Figure 2b).
Linking gut dysbiosis and physiological effects due to xenobiotics
The murine model has been widely used in pharmaceutical and medical research. Twenty-seven of the studies included in this review employed rodents (Table 1). However, when evaluating and analysing gut dysbiosis, some key aspects must be considered to better understand the processes involved. For instance, the inherent variability within the microbiomes of the same species, particularly with regard to resident microorganisms, depends on the physiology of the digestive system; therefore, the identification and standardisation of external factors capable of modifying microbial communities should be considered (Franklin and Ericsson, Reference Franklin and Ericsson2017; Hugenholtz and de Vos, Reference Hugenholtz and de Vos2018). In this sense, knowledge gaps also need to be considered. However, one of the main limitations appears to be that functional predictions of metabolic routes and affected genes are only possible at the in-silico level without a subsequent step to evaluate systemic effects. Complete studies should evaluate the general effects associated with gut dysbiosis using biochemical approaches to determine specific biomarkers and histopathology techniques and, if possible, to evaluate mechanistic approaches in terms of immunology, behaviour, and survival rates.
Only 6 of 45 papers included a biochemistry analysis of blood serum. In these experiments, molecules related to inflammatory processes were quantified, including inflammatory and neurotrophic factors (Lin et al., Reference Lin, Zhao, Zhang, He, Wang, Chang, Cui, Gao, Li, Chen and Li2020), interleukins (Nielsen et al., Reference Nielsen, Roager, Casas, Frandsen, Gosewinkel, Bester, Licht, Hendriksen and Bahl2018a), and C-reactive protein (van den Brule et al., Reference Van Den Brule, Ambroise, Lecloux, Levard, Soulas, De Temmerman, Palmai-Pallag, Marbaix and Lison2016). In the same way, molecules associated with lipid metabolism (one of the most important routes affected by gut dysbiosis), adiponectin (Gaulke et al., Reference Gaulke, Rolshoven, Wong, Hudson, Ho and Sharpton2018), and triglycerides (Lai et al., Reference Lai, Ng, Wan, Wong, Leung, Li and Wong2018) were determined and quantified. The results from these analyses allow xenobiotic concentrations in the bloodstream to be determined (Zhan et al., Reference Zhan, Liang, Liu, Ma, Li, Liu, Liu, Wang and Zhou2018). Histopathological techniques were used in 5 out of 45 studies. The intestine was the target organ in these approaches, and the main aim of these studies was to identify structural changes in different intestinal regions as well as the infiltration of inflammatory cells (Lin et al., Reference Lin, Zhao, Zhang, He, Wang, Chang, Cui, Gao, Li, Chen and Li2020; Mesnage et al., Reference Mesnage, Teixeira, Mandrioli, Falcioni, Ducarmon, Zwittink, Mazzacuva, Caldwell, Halket, Amiel, Panoff, Belpoggi and Antoniou2021; Ribière et al., Reference Ribière, Peyret, Parisot, Darcha, Déchelotte, Barnich, Peyretaillade and Boucher2016; Tang et al., Reference Tang, Tang, Ren and Li2020; Zhou et al., Reference Zhou, Xu, Huang, Liu, Chen, Hu, Li, Liu and Guo2020). Finally, some organism-level changes were considered in the experimental approaches such as behavioural changes due to the critical role of the gut–brain axis (Aitbali et al., Reference Aitbali, Ba-M’hamed, Elhidar, Nafis, Soraa and Bennis2018; Dechartres et al., Reference Dechartres, Pawluski, Gueguen, Jablaoui, Maguin, Rhimi and Charlier2019) and survival rates after exposure to xenobiotics (Lee et al., Reference Lee, Seo, Ha, Kim, Choi, Oh, Lee, KimY, Park and Yoon2020; Motta et al., Reference Motta, Raymann and Moran2018; Wang et al., Reference Wang, Berdy, Velasquez, Jovanovic, Alkhalifa, Minbiole and Brucker2020). In the previous experiments, omics tools were used to explore these changes, including metabolomics to evaluate changes in gut and faeces metabolites (Chi et al., Reference Chi, Gao, Bian, Tu, Ru and Lu2017; Lin et al., Reference Lin, Zhao, Zhang, He, Wang, Chang, Cui, Gao, Li, Chen and Li2020; Nielsen et al., Reference Nielsen, Zhang, Curran, Magnuson, Venables, Durrer, Allen and Roberts2018b), transcriptomics (Wang et al., Reference Wang, Berdy, Velasquez, Jovanovic, Alkhalifa, Minbiole and Brucker2020; Yang et al., Reference Yang, Wang, Lv, Tian, Peng, Peng, Xu, Song, Lv, Chen, Sun and Wang2018), and proteomics (Li and Cui, Reference Li and Cui2018; Yang et al., Reference Yang, Wang, Lv, Tian, Peng, Peng, Xu, Song, Lv, Chen, Sun and Wang2018).
Only 7 of 45 studies in this review dealt with the effects of pesticides on pollinators (Blot et al., Reference Blot, Veillat, Rouzé and Delatte2019; Botina et al., Reference Botina, Vélez, Barbosa, Mendonça, Pylro, Tótola and Martins2019; Dai et al., Reference Dai, Yan, Ma, Yang, Wang, Hou, Wu, Liu and Diao2018; Daisley et al., Reference Daisley, Trinder, McDowell, Collins, Sumarah and Reid2018; Motta et al., Reference Motta, Raymann and Moran2018; Rouzé et al., Reference Rouzé, Moné, Delbac, Belzunces and Blot2019; Wang et al., Reference Wang, Berdy, Velasquez, Jovanovic, Alkhalifa, Minbiole and Brucker2020). This topic is of particular importance because the ecological roles of pollinators appear to be threatened by non-target exposure to xenobiotics (Cullen et al., Reference Cullen, Thompson, Carolan, Stout and Stanley2019). Likewise, the prevalence of contaminants in water bodies highlights the importance of studies that assess the effects of using fishes as research models. In this study, eight publications employed fish models, of which most were zebrafish (Bao et al., Reference Bao, Zhao, Wu, Lou, Lu, Yu, Fu and Jin2020; Buerger et al., Reference Buerger, Dillon, Schmidt, Yang, Zubcevic, Martyniuk and Bisesi2020; Cheng et al., Reference Cheng, Zhang, Gao, Xu and Wang2022; González-Penagos et al., Reference González-Penagos, Zamora-Briseño, Cerqueda-García, Améndola-Pimenta, Pérez-Vega, Hernández-Nuñez and Rodríguez-Canul2020; Jiang et al., Reference Jiang, Chen, Liu, Wang, Wu and Zhao2021; Keerthisinghe et al., Reference Keerthisinghe, Wang, Wang, Wang, Yang, Li, Yang, Xi, Dong and Fang2020; Sherif et al., Reference Sherif, El‐Sharawy, El‐Samannoudy, Sabry, Eldawoudy, Abdelsalam and Younis2021; Xie et al., Reference Xie, Zhou, Xu, Feng, Pan, Junaid, Wang and Zou2020). In addition, other studies considered other organisms such as spiders (Yang et al., Reference Yang, Wang, Lv, Tian, Peng, Peng, Xu, Song, Lv, Chen, Sun and Wang2018), arthropods (Wang et al., Reference Wang, Qiao, Wang and Zhu2019), and poultry (Zhou et al., Reference Zhou, Xu, Huang, Liu, Chen, Hu, Li, Liu and Guo2020).
Gut dysbiosis in the murine model
Most mammal studies that have assessed the impacts of xenobiotics on gut microbiota have been conducted in murine models. However, even if the same species is used in different studies, various biological factors could induce variability among responses. In addition, some responses have shown a pollutant-type dependency. Nevertheless, some evidence has emerged indicating possible patterns at the taxonomic level. This is the case of the Firmicutes phyla. In this phyla, changes in abundance that are mainly due to changes in the abundance of the Lachnospiraceae family have been associated with heavy metals (Breton et al., Reference Breton, Massart, Vandamme, De Brandt, Pot and Foligné2013b). Similarly, Lactobacillaceae abundance has shown responsiveness to exposure to methylmercury (Lin et al., Reference Lin, Zhao, Zhang, He, Wang, Chang, Cui, Gao, Li, Chen and Li2020), benzo[a]pyrene (Ribière et al., Reference Ribière, Peyret, Parisot, Darcha, Déchelotte, Barnich, Peyretaillade and Boucher2016), glyphosate and its commercial formulations (Aitbali et al., Reference Aitbali, Ba-M’hamed, Elhidar, Nafis, Soraa and Bennis2018; Mao et al., Reference Mao, Manservisi, Panzacchi, Mandrioli, Menghetti, Vornoli, Bua, Falcioni, Lesseur, Chen and Belpoggi2018; Tang et al., Reference Tang, Tang, Ren and Li2020), and trichloroethylene (Khare et al., Reference Khare, Gokulan, Williams, Bai, Gilbert and Blossom2019). Interestingly, these bacteria are essential for butyrate synthesis. Butyrate is related to gut barrier integrity and is a source of energy for epithelial cells in the colon (Silva et al., Reference Silva, Bernardi and Frozza2020).
In the previously mentioned studies (Aitbali et al., Reference Aitbali, Ba-M’hamed, Elhidar, Nafis, Soraa and Bennis2018; Khare et al., Reference Khare, Gokulan, Williams, Bai, Gilbert and Blossom2019; Lin et al., Reference Lin, Zhao, Zhang, He, Wang, Chang, Cui, Gao, Li, Chen and Li2020; Tang et al., Reference Tang, Tang, Ren and Li2020), decreases in Firmicutes abundance were observed, whereas in experiments conducted with polybrominated diphenyl ethers (Li et al., Reference Li, Dempsey, Wang, Lee, Weigel, Fei, Bhatt, Prasad, Raftery, Gu and Cui2018) and bisphenol A (Malaisé et al., Reference Malaisé, Menard, Cartier, Gaultier, Lasserre, Lencina, Harkat, Geoffre, Lakhal, Castan, Olier, Houdeau and Guzylack-Piriou2017), increases in Firmicutes abundance were observed after exposure. Furthermore, Chi et al. (Reference Chi, Gao, Bian, Tu, Ru and Lu2017) demonstrated that changes in Firmicutes abundance were sex-dependent due to manganese exposure, with increases and decreases observed in males and females, respectively. Likewise, Lozano et al. (Reference Lozano, Defarge, Rocque, Mesnage, Hennequin, Cassier, de Vendômois, Panoff, Séralini and Amiel2018) described changes in Lactobacillaceae abundance associated with exposure to roundup, although they were only present in females. However, although several studies in murine models indicate changes in this phylum, Wang et al. (Reference Wang, Tang, Glenn and Wang2016) reported that no significant changes in the abundance of this group were observed after exposure to aflatoxin B1. Similarly, the phylum Bacteroidetes also presents different outcomes depending on the exposure. Pollutants, such as trichloroethylene (Khare et al., Reference Khare, Gokulan, Williams, Bai, Gilbert and Blossom2019), aflatoxin B1 (Liew et al., Reference Liew, Mohd-Redzwan and Than2019), methylmercury (Lin et al., Reference Lin, Zhao, Zhang, He, Wang, Chang, Cui, Gao, Li, Chen and Li2020), benzo[a]pyrene (Ribière et al., Reference Ribière, Peyret, Parisot, Darcha, Déchelotte, Barnich, Peyretaillade and Boucher2016), and glyphosate (Dechartres et al., Reference Dechartres, Pawluski, Gueguen, Jablaoui, Maguin, Rhimi and Charlier2019; Nielsen et al., Reference Nielsen, Roager, Casas, Frandsen, Gosewinkel, Bester, Licht, Hendriksen and Bahl2018a), could promote positive or negative changes in Bacteroidetes abundance that are mainly associated with changes in Bacteroides abundance. It is worth noting that both Firmicutes and Bacteroidetes play important roles within mammalian microbiota ecosystems. It has been reported that imbalances in their abundance have been involved in different intestinal disorders while also being associated with an obesogenic phenotype (Buerger et al., Reference Buerger, Dillon, Schmidt, Yang, Zubcevic, Martyniuk and Bisesi2020; Lozano et al., Reference Lozano, Defarge, Rocque, Mesnage, Hennequin, Cassier, de Vendômois, Panoff, Séralini and Amiel2018).
Dysbiotic changes may also be linked to physiological effects that affect the overall fitness of the host, with manifestations such as changes in body composition, survival rates, immune conditions, metabolic production, and behaviour as well as additional transgenerational effects. Changes in metabolism may be identified through blood biochemistry and metabolomics analyses, which can also help to identify biotransformations of target xenobiotics and the synthesis of enzymes and transport molecules such as the ones associated with bile acid metabolism (Li et al., Reference Li, Dempsey, Wang, Lee, Weigel, Fei, Bhatt, Prasad, Raftery, Gu and Cui2018). Metabolic changes can also be identified by the production of neurotransmitters such as serine, GABBA, L-tyrosine, and aspartic acid (Lin et al., Reference Lin, Zhao, Zhang, He, Wang, Chang, Cui, Gao, Li, Chen and Li2020). Other impacts of xenobiotic exposure have been associated with changes in oxidative stress and glutathione (GSH), glutathione peroxidase (GSH-Px), and adiponectin levels (Gaulke et al., Reference Gaulke, Rolshoven, Wong, Hudson, Ho and Sharpton2018; Tang et al., Reference Tang, Tang, Ren and Li2020). Also, studies evaluating inflammatory processes have reported changes in the production of metabolites, such as IL-33, IFN-gamma, and IL-3, and changes in cytokine levels (eg. IL-12, IL-17F, GM-CSF, eotaxin, and MIP-1-α chemokine) that are directly associated with intestinal immunoregulation (Khare et al., Reference Khare, Gokulan, Williams, Bai, Gilbert and Blossom2019). Furthermore, exposure to glyphosate-based herbicides promotes changes in the gut microbiota that result in anxiogenic and depressive behaviour in murine models (Aitbali et al., Reference Aitbali, Ba-M’hamed, Elhidar, Nafis, Soraa and Bennis2018), which confirms that chemical pollutants can alter animal social behaviour at individual and group levels (Michelangeli et al., Reference Michelangeli, Martin, Pinter-Wollman, Ioannou, McCallum, Bertram and Brodin2022). Moreover, exposure to bisphenol A induced a significant and gradual increase in weight over the experimental period, even without changes in the food intake rate (Malaisé et al., Reference Malaisé, Menard, Cartier, Gaultier, Lasserre, Lencina, Harkat, Geoffre, Lakhal, Castan, Olier, Houdeau and Guzylack-Piriou2017). This outcome is one of the main reasons to classify bisphenol A as an obesogen toxicant and thus one of the most studied endocrine-disrupting xenobiotics (Chiu et al., Reference Chiu, Warner, Nowak, Flaws and Mei2020). Body composition measurements are determining factors that must be considered when attempting to understand the overall impact that a xenobiotic compound may have on an organism.
Gut dysbiosis in insect pollinators
Worldwide, ecotoxicological research on pollinating insects arises from their inevitable chronic exposure to pesticides and the fundamental roles they play within ecosystems (Cullen et al., Reference Cullen, Thompson, Carolan, Stout and Stanley2019). Pollinating insects play critical roles in plant reproduction cycles, which can influence biodiversity and ecosystem services with impacts that transcend agriculture (Lautenbach et al., Reference Lautenbach, Seppelt, Liebscher and Dormann2012; Potts et al., Reference Potts, Imperatriz-Fonseca, Ngo, Aizen, Biesmeijer, Breeze, Dicks, Garibaldi, Hill, Settele and Vanbergen2016). Recent studies have shown the effects on gut microbiota due to exposure to glyphosate (Blot et al., Reference Blot, Veillat, Rouzé and Delatte2019; Dai et al., Reference Dai, Yan, Ma, Yang, Wang, Hou, Wu, Liu and Diao2018; Motta et al., Reference Motta, Raymann and Moran2018), spinosad and cooper sulphate (Botina et al., Reference Botina, Vélez, Barbosa, Mendonça, Pylro, Tótola and Martins2019), chlorpyrifos (Daisley et al., Reference Daisley, Trinder, McDowell, Collins, Sumarah and Reid2018), atrazine (Wang et al., Reference Wang, Berdy, Velasquez, Jovanovic, Alkhalifa, Minbiole and Brucker2020), fipronil, imidacloprid, thiamethoxam, and coumaphos (Rouzé et al., Reference Rouzé, Moné, Delbac, Belzunces and Blot2019). However, similar to what has been observed with murine models, major changes in the abundance of Proteobacteria, Firmicutes, and Actinobacteria have been reported, although patterns are difficult to identify given that responses are likely dependent on exposure conditions. For instance, glyphosate exposure has been linked to microbiota changes, such as those observed within the Actinobacteria phylum that are characterised by a reduced abundance of Bifidobacterium spp. (Blot et al., Reference Blot, Veillat, Rouzé and Delatte2019), or changes in Firmicutes abundance that are mostly driven by the increased abundance of Clostridia, Ruminococcaceae (Dai et al., Reference Dai, Yan, Ma, Yang, Wang, Hou, Wu, Liu and Diao2018), Staphylococcus spp., and Lactobacillus spp. (Blot et al., Reference Blot, Veillat, Rouzé and Delatte2019). Motta et al. (Reference Motta, Raymann and Moran2018) reported a decrease in the abundance of Lactobacillus spp. In this sense, it is unclear if the role of Lactobacillus spp. is just as relevant as it is in the murine model. However, these studies are mostly based on 16S rRNA (Dai et al., Reference Dai, Yan, Ma, Yang, Wang, Hou, Wu, Liu and Diao2018), and thus fail to include detailed taxonomic identifications or even functional inferences. Despite these limitations, when the studies have also included qPCR techniques, they have been able to identify specific species of interest. For example, within the Proteobacteria phylum, it was observed that glyphosate exposure promoted a decrease in the abundance of Snodgrasella alvi, which is a characteristic member of the core microbiota of bees (Blot et al., Reference Blot, Veillat, Rouzé and Delatte2019; Motta et al., Reference Motta, Raymann and Moran2018). Remarkably, bees with conventional microbiota that have been exposed to glyphosate exhibited an overall susceptibility to opportunistic pathogens (Motta et al., Reference Motta, Raymann and Moran2018). Other studies have reported changes in the abundance of Gilliamella apicola, a dominant gut Gammaproteobacteria in honey bees and bumblebees, after exposure to pesticides such as fipronil, spinosad, and glyphosate (Blot et al., Reference Blot, Veillat, Rouzé and Delatte2019; Botina et al., Reference Botina, Vélez, Barbosa, Mendonça, Pylro, Tótola and Martins2019; Motta et al., Reference Motta, Raymann and Moran2018; Rouzé et al., Reference Rouzé, Moné, Delbac, Belzunces and Blot2019).
Aside from the direct effects on host fitness, such as dose-dependent survival rates, Wang et al. (Reference Wang, Berdy, Velasquez, Jovanovic, Alkhalifa, Minbiole and Brucker2020) reported that changes in gut microbiota derived from atrazine exposure could exert inherited effects on the progeny, which suggests that legacy microbiota could provide protective effects due to the enrichment of Serratia marcescens and Pseudomonas protegens, which are associated with atrazine metabolism. In this sense, research using biological models with short reproductive cycles, like insect pollinators, allows for the crucial aspects of the overall effects of a particular xenobiotic to be assessed at higher organisational levels. Examples of these assessments include survival rate analysis, linking dysbiosis with disease and xenobiotic exposure, and evaluating transgenerational effects due to inherited microbiota with population-level consequences.
Gut dysbiosis in fishes
Although there is an increase in publications related to gut microbiota dysbiosis, studies using aquatic organisms, such as fishes or amphibians, are scarce compared to murine model investigations (Evariste et al., Reference Evariste, Barret, Mottier, Mouchet, Gauthier and Pinelli2019). Among aquatic model organisms used in dysbiosis experiments, fish models stand out, particularly the zebrafish model. Zebrafish are particularly interesting models due to the homology of their genome and intestinal structure and functionality with those of mammals (Howe et al., Reference Howe, Clark, Torroja, Torrance, Berthelot, Muffato, Collins, Humphray, McLaren, Matthews, McLaren, Sealy, Caccamo, Churcher, Scott, Barrett, Koch, Rauch, White, Chow, Kilian, Quintais, Guerra-Assunção, Zhou, Gu, Yen, Vogel, Eyre, Redmond, Banerjee, Chi, Fu, Langley, Maguire, Laird, Lloyd, Kenyon, Donaldson, Sehra, Almeida-King, Loveland, Trevanion, Jones, Quail, Willey, Hunt, Burton, Sims, Mclay, Plumb, Davis, Clee, Oliver, Clark, Riddle, Elliot, Threadgold, Harden, Ware, Begum, Mortimore, Kerry, Heath, Phillimore, Tracey, Corby, Dunn, Johnson, Wood, Clark, Pelan, Griffiths, Smith, Glithero, Howden, Barker, Lloyd, Stevens, Harley, Holt, Panagiotidis, Lovell, Beasley, Henderson, Gordon, Auger, Wright, Collins, Raisen, Dyer, Leung, Robertson, Ambridge, Leongamornlert, McGuire, Gilderthorp, Griffiths, Manthravadi, Nichol, Barker, Whitehead, Kay, Brown, Murnane, Gray, Humphries, Sycamore, Barker, Saunders, Wallis, Babbage, Hammond, Mashreghi-Mohammadi, Barr, Martin, Wray, Ellington, Matthews, Ellwood, Woodmansey, Clark, Cooper, Tromans, Grafham, Skuce, Pandian, Andrews, Harrison, Kimberley, Garnett, Fosker, Hall, Garner, Kelly, Bird, Palmer, Gehring, Berger, Dooley, Ersan-Ürün, Eser, Geiger, Geisler, Karotki, Kirn, Konantz, Konantz, Oberländer, Rudolph-Geiger, Teucke, Lanz, Raddatz, Osoegawa, Zhu, Rapp, Widaa, Langford, Yang, Schuster, Carter, Harrow, Ning, Herrero, Searle, Enright, Geisler, Plasterk, Lee, Westerfield, de Jong, Zon, Postlethwait, Nüsslein-Volhard, Hubbard, Roest Crollius, Rogers and Stemple2013; Lickwar et al., Reference Lickwar, Camp, Weiser, Cocchiaro, Kingsley, Furey, Sheikh and Rawls2017). Zebrafish have been used to evaluate changes in microbial richness and diversity, the general structure of the microbiota, and possible disruptions in the intestinal tract due to aquatic exposure to environmental pollutants (Zhong et al., Reference Zhong, Li, Lu, Zhang and Guo2022). For instance, carbendazim exposure elicited decreases in the abundance of Firmicutes, Bacteroidetes, and some members of α- and γ-Proteobacteria (Bao et al., Reference Bao, Zhao, Wu, Lou, Lu, Yu, Fu and Jin2020), which is similar to what has been reported with tetracycline exposure (Keerthisinghe et al., Reference Keerthisinghe, Wang, Wang, Wang, Yang, Li, Yang, Xi, Dong and Fang2020). In contrast with these changes, exposure to diethylhexyl phthalate and benzo[a]pyrene increased the abundance of Proteobacteria and Firmicutes, respectively (Buerger et al., Reference Buerger, Dillon, Schmidt, Yang, Zubcevic, Martyniuk and Bisesi2020; Xie et al., Reference Xie, Zhou, Xu, Feng, Pan, Junaid, Wang and Zou2020). Another group with significant changes in abundance is Fusobacteria. In this taxa, exposure to α-cypermethrin promoted an increase of specific taxa (Cheng et al., Reference Cheng, Zhang, Gao, Xu and Wang2022), in contrast to the observations described by Buerger et al. (Reference Buerger, Dillon, Schmidt, Yang, Zubcevic, Martyniuk and Bisesi2020) and Jiang et al. (Reference Jiang, Chen, Liu, Wang, Wu and Zhao2021) in which exposure to diethylhexyl phthalate and triazole fungicides decreased the abundance of Fusobacteria.
As with the murine model, different authors have indicated that changes in the Firmicutes:Bacteroidetes ratio in the gut microbiota serve as an obesity biomarker in zebrafish. These studies detected changes in body weight after exposure to carbendazim (Bao et al., Reference Bao, Zhao, Wu, Lou, Lu, Yu, Fu and Jin2020) and diethylhexyl phthalate (Buerger et al., Reference Buerger, Dillon, Schmidt, Yang, Zubcevic, Martyniuk and Bisesi2020). Also, in the study conducted by Xie et al. (Reference Xie, Zhou, Xu, Feng, Pan, Junaid, Wang and Zou2020), the associations between zebrafish gut microbiota and inflammatory responses after benzo[a]pyrene exposure were described, emphasising increases of IL1β cytokine, IL6, IL8, and Ifn1 protein, which could be related to the inherent genetic background of the zebrafish intestine.
Another fish species widely used in xenobiotic experiments is the Nile tilapia (Oreochromis niloticus). However, only Sherif et al. (Reference Sherif, El‐Sharawy, El‐Samannoudy, Sabry, Eldawoudy, Abdelsalam and Younis2021) explored changes within the Aeromonas, Lactobacillus, and Pseudomonas genera after exposure to titanium dioxide nanoparticles, which induced higher mortalities when fish were challenged with Aeromonas hydrophila. However, the study relies on traditional bacterial counting and not on molecular approaches or technologies.
Gut microbiota study criteria
Experimental considerations
An experimental design for assessing alterations in the gut microbiota must consider key aspects to produce accurate results. Among these is establishing the proper population size for statistical purposes. However, socio-ecological restrictions must be also considered to determine the proper group size within a specific space (Shülke and Ostner, Reference Shülke, Ostner, Mitani, Call, Kappeler, Palombit and Silk2012). This is crucial in rodents and other social species, such as zebrafish, which are gregarious animals that establish complex relationships within their groups (Green, Reference Green1966). These dynamics, specifically any dominance hierarchy, play an essential role in feeding behaviour and could affect stress responses, as some of the main characteristics of dominance include chasing, fighting, and avoidance behaviour (Mondragón et al., Reference Mondragón, Mayagoitia, López-Luján and Díaz1987). The feeding strategy is another relevant variable that must be defined in these studies, especially considering that subordinate organisms take longer to feed than dominant organisms. In rodents, ad libitum feeding is typically employed. However, this method makes it difficult to control xenobiotic dosing, and differences in feeding could also influence the susceptibility to these compounds (Hart et al., Reference Hart, Newman and Robertson1995). An alternative that compensates for ingestion differences, is timed feeding, which grants animals illimitable access to food in a specific period and considers the social dynamics within a group (Carey and Merrill, Reference Carey and Merrill2012). These feeding periods can be flexible and may depend on the experimental species and their biological rhythms. Timed feeding can synchronise many behavioural variables such as anticipatory food activity (Satoh et al., Reference Satoh, Kawai, Kudo, Kawashima and Mitsumoto2006). Dietary exposure to xenobiotics must also be addressed. For instance, in studies using murine models, ensuring that the proper concentration of xenobiotics is supplied is achieved by orally gavaged dosing, but employing this method may not be feasible with other organisms such as fish and invertebrates. Nevertheless, it is important to consider possible stress arising from the methodology employed.
Other considerations must also be considered when establishing a dysbiosis experimental design. The sampling protocol for gut content must be standardised to yield comparable results, as the composition and dynamics of the microbiota depend on specific target (tissue and/or organ) conditions such as the enzymes involved in nutrient metabolism, the molecular patterns associated with cell-surface attachment, and the ability to avoid immune responses from the host (Adak and Khan, Reference Adak and Khan2019). In turn, these factors are related to specific gastrointestinal tract regions. Therefore, this is also applicable to establishing comparisons between histological analyses that are mainly due to the microanatomy of every intestinal region and each species (Mowat and Agace, Reference Mowat and Agace2014). Only a few studies include autocritiques on possible experimental artefacts that will help to improve future experiments such as the importance of estimating exposure periods and origin of the recollected samples to extract microbial DNA (Chi et al., Reference Chi, Bian, Gao, Ru, Tu and Lu2016), sample size, and bioinformatic parameters (Wang et al., Reference Wang, Tang, Glenn and Wang2016).
Sequencing strategies
In this review, 35 of the 45 selected studies used the taxonomic 16S rRNA amplicon fingerprinting approach to evaluate changes in bacterial communities due to the dietary intake of xenobiotics (Table 1). Notably, the amplification of the V3 − V4 conserved region was the most frequently employed approach. The 16S method is widely used in gut dysbiosis studies due to its cost-effectiveness and various bioinformatics tools. However, this method comes with some limitations that should be considered. These considerations include (1) a putative association of the 16S gene with taxa defined as operational taxonomic units and the resulting difficulty of assessment (Poretsky et al., Reference Poretsky, Rodriguez-R, Luo, Tsementzi and Konstantinidis2014); (2) a lack of reproducibility as a result of different experimental variables, including sample management, the 16S gene variable region selected, and the bioinformatic pipelines employed (Han et al., Reference Han, Gao, Li, Tan, Xie, Zhang and Li2020); and (3) an inability to establish reliable species or strain functional predictions (Ranjan et al., Reference Ranjan, Rani, Metwally, McGee and Perkins2016).
The 16S rRNA amplicon fingerprinting method classifies the identified taxa from phylum to genus, with an observable decrease in specificity at the lower levels, making it particularly unreliable at the species level (similar to the difference in results obtained using OTUs vs. ASVs). In this sense, whole-genome sequencing (WGS) approaches, also known as metagenomics or shotgun sequencing, allows for more information to be retrieved from genera with low abundance (Durazzi et al., Reference Durazzi, Sala, Castellani, Manfreda, Remondini and De Cesare2021). Moreover, WGS can be used to infer functional features such as the genes present and metabolic routes involved in responses to xenobiotic exposure (Mesnage et al., Reference Mesnage, Teixeira, Mandrioli, Falcioni, Ducarmon, Zwittink, Mazzacuva, Caldwell, Halket, Amiel, Panoff, Belpoggi and Antoniou2021; Stedtfeld et al., Reference Stedtfeld, Stedtfeld, Fader, Williams, Bhaduri, Quensen, Zacharewski, Tiedje and Hashsham2017). In addition to functional assessments, the WGS approach allows for diversity indexes to be more accurately estimated and for the taxonomic structures of complex microbial communities to be elucidated, taking into consideration species and even strains of bacteria, archaea, viruses, fungi, and protozoans (Han et al., Reference Han, Gao, Li, Tan, Xie, Zhang and Li2020; Poretsky et al., Reference Poretsky, Rodriguez-R, Luo, Tsementzi and Konstantinidis2014; Ranjan et al., Reference Ranjan, Rani, Metwally, McGee and Perkins2016). For example, in a study by Mesnage et al. (Reference Mesnage, Teixeira, Mandrioli, Falcioni, Ducarmon, Zwittink, Mazzacuva, Caldwell, Halket, Amiel, Panoff, Belpoggi and Antoniou2021), increases in the abundance of three species (Eggerthella isolate HGM04355, Acinetobacter johnsonii, and Akkermansia municiphila) were detected after glyphosate and MON 52276 exposure. Also, the authors characterised and quantified the abundance of the EPSPS gene encoding the target enzyme of this herbicide and performed a functional analysis using KO assignments specific to their biological model. However, exploring multivariate and multidimensional microbial communities with this approach is highly expensive for large-scale studies with deep sequencing. A more affordable alternative is to adjust the metagenomic sequencing depth according to the experimental hypothesis of the study, focusing on the required reads to detect the species, functions, and biomarkers expected as outcomes. In this regard, shallow shotgun sequencing is a viable option to determine common species and functional profiles in samples at a slightly higher cost than that of 16S fingerprinting without losing valuable information (Hillmann et al., Reference Hillmann, Al-Ghalith, Shields-Cutler, Zhu, Gohl, Beckman, Knight and Knights2018). Although the WGS method usually provides complete information about the structure and functionality of the microbiomes, this approach cannot necessarily be generalised, as particular changes may be required for specific depth adjustments. This was the case with the study of Stedtfeld et al. (Reference Stedtfeld, Stedtfeld, Fader, Williams, Bhaduri, Quensen, Zacharewski, Tiedje and Hashsham2017), who were unable to determine variations in the abundance of antibiotic-resistance genes with lower abundance. They then used qPCR to overcome this limitation.
Data analysis reproducibility
The different methodological approaches used in microbiota experiments create substantial difficulty when integrating the resulting data of different studies. Restrictions when comparing outcomes stem from the different methods used to extract and store DNA/RNA material, the sequencing strategies and platforms used in each study, and the bioinformatics pipelines and databases employed. It is desirable to use a specific database to reference sequence assignments such as the Murine Microbiome Database (MMDB). However, it is also necessary to be able to compare among general databases to avoid size, updating, or management-related biases, which are mainly due to the search space or the quantity of undiscovered microbial groups, as this can lead to false positive and negative classifications, respectively (Ye et al., Reference Ye, Siddle, Park and Sabeti2019). This also applies when exploring the effects of xenobiotic exposure that may induce changes associated with new or unclassified microbial groups, which may be dismissed because databases may not include abnormal or disturbed microbiota. In addition, a standardisation of available metadata is important to provide additional context for the samples and raw reads available in public repositories (Dundore-Arias et al., Reference Dundore-Arias, Eloe-Fadrosh, Schriml, Beattie, Brennan, Busby, Calderon, Castle, Emerson, Everhart and Eversole2020). For some experiments, such as evaluations of dysbiosis under conditions of xenobiotic exposure, their outcomes require a clear workflow with sufficiently detailed information on the algorithms and parameters used for data processing in order to ensure reproducibility. Thus, data accessibility is crucial for creating a robust conceptual framework to increase our understanding of the dynamics of microbiota changes due to xenobiotic exposure.
Conclusions
The gut microbiota was explored as a dynamic interaction system, which can be perturbed due to xenobiotic exposure. According to the studies reviewed herein, impacts on specific gut microbial communities could be associated with pathologies and changes at intestinal, metabolic, and systemic levels. Thus, the overall health of the organism may be at stake due to xenobiotic exposure. In this sense, using bioinformatics tools and biological models is a relevant strategy to explore dysbiosis mechanisms. Murine models are the most studied in these experiments. Exploring the effects of xenobiotic exposure on the structure and composition of the gut microbiota from organisms that are exposed to several contaminants in natural environments, such as pollinators and fishes, could extend our knowledge of the main microbial groups that are susceptible to xenobiotics and the overall impacts that these compounds have. While 16S approaches could be used as exploratory approaches, more WGS and physiological studies with standardised and specific experimental designs are needed to understand how xenobiotics can perturb the gut microbiota and how the results may be extrapolated.
Acknowledgements
We thank Andrea Lievana MacTavish for English language editing. Figures were created with BioRender and SankeyMATIC.
Data availability statement
The data for this study are available from the corresponding author upon reasonable request.
Author contribution
Conceptualization: B.I.-M, B.Y.-R; Data curation: B.I.-M.; Funding acquisition: M.B.-L.; Supervision: B.Y.R.; Visualisation: B.I.-M., B.Y.-R.; Writing – original draft: B.I.-M.; Writing – review and editing: B.Y.-R., B.G.-G., M.B.-L., L.R.
Disclosure statement
The authors declare no conflict of interest.
Funding
Partial funding for this study was provided by CONACYT (CB2016-288306) and Doctoral grant to B.I.-M. (#CVU 720840). The funders had no role in the design of the study; in the collection, analyses, or interpretation of data; in the writing of the manuscript; or in the decision to publish the results.