1. Introduction
The digital revolution has led since the 1950s to an acceleration of the amount of information that is harvested and processed by machines. More recently, information technologies have progressed very rapidly, allowing autonomous systems to perform increasingly complex tasks previously done only by humans and thought to rely on human forms of intelligence. Machines are becoming more powerful than human brains for a seemingly unbounded part of humanity's activities. Simultaneously, digital platforms take control of whole social and economic sectors with a quality and efficiency of service that condemns legacy institutions, private enterprises as well as public administrations, to obsolescence. This singularity (Chalmers, Reference Chalmers2009) leads to a trans-human information dynamics, an evolution of the relationship between humans and machines where humans increasingly play a subordinate role in the technosphere (Haff, Reference Haff2014). How does this profound transformation of human societies supported by a radical change of information processing relate to their main challenge, sustainability? Our aim is to investigate how the evolution of the way human societies process information and accommodate knowledge determines their sustainability, and how that process might evolve in the future, under the impact of artificial intelligence (AI) and machine learning.
Let us, at this stage, consider a general concept of sustainability, such as the safe operating space for humanity (Rockström et al., Reference Rockström, Steffen, Noone, Persson, Chapin, Lambin, Lenton, Scheffer, Folke, Schellnhuber, Nykvist, de Wit, Hughes, van der Leeuw, Rodhe, Sörlin, Snyder, Costanza, Svedin and Foley2009), which establishes biophysical thresholds that should not be crossed as a consequence of human activities. There are many different concepts, such as resilience, stability, homeostasis, sustainability, etc. to capture the behavior of adaptive social-ecological systems (SESs). These concepts have successively been refined. For example, sustainability was associated with seven dimensions (Vogt & Weber, Reference Vogt and Weber2019), resilience thinking emphasizes our embedding in the biosphere (Folke, Reference Folke2016). Moreover, as Holling noted half a century ago: ‘our traditional view of natural systems might well be less a meaningful reality than a perceptual convenience’ (Holling, Reference Holling1973). Although they might rely on different representations of the world, information processing is an issue for all.
The difficulty in adapting research to engage toward sustainability challenges has increasingly been addressed in the last two decades (Lang et al., Reference Lang, Wiek, Bergmann, Stauffacher, Martens, Moll, Swilling and Thomas2012), with an emphasis on transdisciplinarity, as well as by the introduction of new concepts such as co-creation of knowledge. Our aim is rather to consider the issue of information and knowledge processing from a much broader perspective, not restricted to research, but encompassing the complete undertaking of information processing in societies. We will make that notion more precise in what follows, but we are well aware of the difficulty in capturing the holistic concept of information. Our approach is related to the concept of ‘wicked problems’ (Rittel & Webber, Reference Rittel and Webber1973) which cannot unambiguously be defined. Rittel and Weber's initial vision of wicked problems was expanded to address the tragedy of policy planning as a ‘super wicked problem’ (Levin, Cashore, Bernstein, & Auld, Reference Levin, Cashore, Bernstein and Auld2012). Levin et al. demonstrated that analyses are pursued on a ‘narrow set of objectives’, which although important fail to capture the transformations at play. Different notions of wicked problems have been introduced, with conceptual differences on what a solution might be (Peters, Reference Peters2017). It has also been shown that many sustainability interventions target ‘highly tangible, but essentially weak, leverage points’ in Abson et al. (Reference Abson, Fischer, Leventon, Newig, Schomerus, Vilsmaier, von Wehrden, Abernethy, Ives, Jager and Lang2017), which propose to focus on alternative dimensions: ‘reconnecting people to nature, restructuring institutions and rethinking how knowledge is created and used in pursuit of sustainability’. It is thus widely agreed that a paradigmatic shift is required to address the present conundrum.
All these dimensions are of course intertwined. They are tentatively integrated in the concept of SESs (Reyers, Folke, Moore, Biggs, & Galaz, Reference Reyers, Folke, Moore, Biggs and Galaz2018). We hope to better characterize, in its generality, the global information system that underlies these approaches, taking into account more of its dimensions, whether explicitly perceived or not. In contrast to previous approaches though, we do not consider the intentional design of political solutions, but look at how the global information system evolves, co-evolves with its societal and environmental context. The recent evolution of information-processing systems, with the potential capacity for all to express themselves through platforms on the Internet, has contributed to weaken legacy institutions and adherence to a ‘truth’, leading to the rapid amplification of ‘fake’ news (Lazer et al., Reference Lazer, Baum, Benkler, Berinsky, Greenhill, Menczer, Metzger, Nyhan, Pennycook, Rothschild, Schudson, Sloman, Sunstein, Thorson, Watts and Zittrain2018; Scheufele & Krause, Reference Scheufele and Krause2019). But, the sustainability conundrum contributes probably more than technological transformation to the distrust in science and politics.
To begin with, we need to set the stage by considering a long-term perspective on knowledge processing. Fundamentally, societies define what they consider as their environments, what they see as challenges in those environments, and what might be potential solutions for these challenges. That definition process might be internally orchestrated by dominant elites in defense of limited interests, but in all cases it results in a societal dynamic equilibrium. Societies construct such representations in self-referential ways (Luhmann, Reference Luhmann1989). In this paper, sustainability will therefore be conceived as a societal endogenous challenge, rather than as an environmental, exogenous, and objectifiable one. It follows that to deal with the sustainability conundrum, it is necessary to consider the ways in which societies conceive it. That in turn involves societies' information and knowledge processing.
Feedback loops play an important role in the co-evolution of societies and knowledge. Positive feedback loops trigger chains of causality as seen in the figures below. The uninterrupted process of human individual and collective learning that has transformed our societies from small bands roaming the Earth to huge societies involving millions if not billions of people may be seen as the result of two positive feedback loops. They create shared order out of diverse experiences of the – seemingly chaotic – world, by isolating patterns, characterizing them in terms of a limited number of dimensions that are used to structure knowledge (van der Leeuw, Reference van der Leeuw, Costanza, Graumlich and Steffen2007). The first feedback loop concerns individuals and is summarized in Figure 1.

Figure 1. Knowledge generation feedback loop.
Problems that exceed individuals' capacity have an impact on the structure of the groups involved, leading to a second feedback loop as shown in Figure 2.

Figure 2. Social groups processing knowledge feedback loop.
To make sense of these diagrams, we must clarify our use of the term 'information'. Data are, in our terms, the phenomena and patterns observed by human beings. But, these provide information only as far as they are interpreted in terms of knowledge by means of information processing by the observers of the data. Information thus links data and knowledge.
Central in the evolution of information processing is the acquisition of ever growing numbers of cognitive dimensions. The more cognitive dimensions exist, the more, and more complex, problems can be tackled, and the more quickly knowledge is accumulated and novel techniques are developed to reduce the effort involved.
This accumulation of information-processing capacity enables a concomitant increase in matter, energy, and information flows through a group or society, enabling its members to grow in numbers. Hence, one can conceive of human societies as ‘dissipative structures’ in the sense of Prigogine (Reference Prigogine1980) – dynamic structures dependent for their existence on flows of energy, matter, and information which, as part of their information processing, dissipate entropy. All societies organize themselves and their environment to attract enough matter and energy resources for their individual members to survive. To do that, they depend on an outward flow of information which structures the relationship between them and their environment, and on inward flows of matter and energy. To maintain some sort of homeostasis, they also handle the dual flows. In the process of structuring knowledge by information processing, they expand and align the understanding, know-how, and skills of the individuals in the society involved, including their technical and organizational means of solving problems, so as to generate and maintain group cohesion. That process is responsible for the long-term co-evolution of human knowledge, language, technology, social institutions, and the environments in which societies develop.
Knowledge processing systems embedded in societies evolve together with the challenges societies try to address, and the technologies used to do so. They also condition the possible paths societies might take. They define the conditions of the preservation of sustainability and constrain the possibilities of adaptation. In this paper, we would focus on the most recent evolution of information and knowledge processing, and situate it in the long-term history of human societies to better understand how ensuring sustainability is related to information processing.
2. Information processing and environment
The acceleration of the capacity to process information automatically on technological systems and the growing awareness of the threats that the environment poses to humanity have developed synchronously (Grumbach & Hamant, Reference Grumbach and Hamant2018). But, in contemporary societies the co-evolution of knowledge generation and problem solving seems to be dysfunctional. Our societies develop increasingly precise, but fragmented, knowledge on the global environment, but that knowledge does not sufficiently serve its purpose: to maintain a functional articulation of human societies with their environments. Isaac Asimov expressed that trouble long ago: ‘The saddest aspect of life right now is that science gathers knowledge faster than society gathers wisdom’ (Asimov & Shulman, Reference Asimov and Shulman1988).
The digital revolution has of course played a fundamental role in the awareness of the complexity of the environmental dynamics, thanks to the capacity to harvest large quantities of environmental data and to simulate ecosystems in computerized models (Lynch, Reference Lynch2008). This has allowed the development of increasingly robust predictions of the evolution of the climate as well as other dimensions of ecosystems over decades (e.g. Sachs et al., Reference Sachs, Nakicenovic, Messner, Rockström, Schmidt-Traub, Busch, Clarke, Gaffney, Kriegler, Kolp, Leininger, Riahi, van der Leeuw, van Vuuren and Zimm2018). But, as we will argue below, the digital revolution involves fundamental changes in the information processing of human societies, which impact the control of the flows of matter, energy, and information as well as the processing of knowledge, and thus affects our understanding of the nature of sustainability and the potential ways to deal with the conundrum.
The turn of the 1950s is fundamental. It is the beginning of the ‘Great Acceleration’, a rapid evolution of both social and environmental parameters (Steffen et al., Reference Steffen, Sanderson, Tyson, Jäger, Matson, Moore, Oldfield, Richardson, Schellnhuber, Turner and Wasson2004). From the information processing point of view, two fundamental aspects change: (1) the growing consciousness of the intricacy of the interactions of human societies with their natural environments, and the risks thereof for global stability, clearly stated by Edward Teller for the American Petroleum Institute in 1959 (Franta, Reference Franta2018), and (2) the increasingly rapid development of digital processing, which has been envisioned in particular by Gordon Moore (Reference Moore1965).
The information-processing system of a society deals with the environmental dynamics the society is embedded in. It handles changes of the environment, including when they result from the sometimes critical unintended consequences of human actions (Merton, Reference Merton1936). Ideally, the information-processing system should contribute to maintain sustainable conditions. If it does not, the ‘dissipative structure’ can be seen as dysfunctional. It seems that that is currently the case with the mismatch between scientific knowledge and societal action, resulting in the degradation of their living environment by human societies. What causes such a deviation from dynamic equilibrium? We claim that the difference between human perception and human action might be a decisive element (cf. van der Leeuw, Reference van der Leeuw2019; van der Leeuw & Folke, Reference van der Leeuw and Folke2021). We will attempt to capture it in terms of the mismatch between the limited set of dimensions perceived and measured, and the unbounded set of dimensions impacted by action. This is not a new approach, it has been considered in philosophy (Schopenhauer, Reference Schopenhauer1819), but we will try to demonstrate how it could help characterize the sustainability conundrum.
Perception, whether individual or societal, concerns only a limited and biased set of dimensions, while action confronts that limited perception with the unlimited dimensionality of the environment's dynamics. In that interaction, newly emergent problems often trigger the manifestation of additional, unforeseen, dimensions. This is well illustrated by the famous example of the Tacoma Narrows Bridge in the USA, a suspension bridge which collapsed a few months after its opening in 1940, for an, at that time, surprising reason: moderate winds produced self-exciting aeroelastic flutter. That phenomenon then entered textbooks, introducing new dimensions in engineering (Malík, Reference Malík2013; Pasternack, Reference Pasternack2015).
From an external point of view, a crisis is a temporary dysfunctional moment in a system's trajectory which, if the system is resilient, can subsequently be resumed after often only minor changes. But, the present disorder seems of a different nature, requiring a fundamental adaptation, much like a paradigmatic change. From the endogenous perspective developed here, such events involve ‘an incapacity of a society's information processing to deal with the (environmental and other) dynamics in which that society is involved’ (van der Leeuw, Reference van der Leeuw2020, p. 334). The corona virus disease 2019 (COVID-19) pandemic, for example, is often considered an exogenous event, but it is also endogenous. Until it occurred, information was ignored, not yet available, or unobtainable, so that the society's information processing was not in tune with the dynamics occurring in its environment (Gans, Reference Gans2020). Once the virus was included in most countries' information-processing apparatus, subsequent differences in the speed and efficiency of societies' information processing led to mortality gaps (Rahmandad, Lim, & Sterman, Reference Rahmandad, Lim and Sterman2020). Missing tools responsible for those differences include digital means to monitor people's interactions (contact tracing), and to aggregate the data to map the propagation of the virus.
The concept of Anthropocene (Crutzen, Reference Crutzen2002) was introduced to capture the present time. If it originates as a geological epoch, the beginning of significant human impact on our planet's geology and ecosystems, it also designates the political setting around the sustainability conundrum, which we try to capture as an endogenous information-processing challenge. Societies learn from events and change how they interact with their environment. Most of their adaptation requires adding relevant dimensions to the society's information-processing apparatus.
But, interestingly, the role of this learning process can only be understood by considering also the information that is not processed. What is generally considered as ‘noise’ rather than as ‘signal’ is potential meaning except for the fact that there is not yet a successful way to define the structure that would allow it to be transformed into meaningful signals. By extricating from a set of observations those that ‘make sense' in a particular conceptual framework, one is also discarding observations that could make sense in another framework which remains to be implemented. What is the role of those unprocessed observations? What is their relationship to the observations that were processed? How do both kinds contribute to beliefs, rituals, and other forms of knowledge processing that condition the interaction with the environment?
3. Perception, unprocessed information, resonance, and asymmetries
‘Unprocessed’ information is rarely considered when studying information systems. It is the case, though, in fields which distinguish various levels of information processing such as anthropology and ethnology, which consider myths and beliefs, or neurosciences and psychology, which consider unconscious information processing. Yet, a quick scan of the socio-economic and industrial models that currently underpin the organization of much of the world, reveals how much unprocessed information is embedded in mostly ‘Western’ norms (Williamson, Reference Williamson2009) that are not only perceived as the inevitable outcome of history, but as objective reality itself. They are the result of a history that could have taken alternative paths, and are therefore not inevitable. Over parts of the system's trajectory, its cohesion and feedbacks were so strong that it moved in a specific direction, but there were points in time at which new perspectives emerged, involving new dimensions, so that there was a choice between trajectories. Over time, the knowledge system thus moved between necessity and choice.
For most of the Early Middle Ages, for example, Western European society held a cyclical, vitalist perspective in which human beings were an integral part of Nature and life and death were seen as a continuous cycle. Following the mid-14th century plague epidemics, a linear perspective developed that saw a life as a trajectory from birth to death, and de-emphasized the link between death and birth. This led to a distinction between living (changing) things and dead (non-changing) ones, and then to the distinction between ‘nature’ and ‘society’ (Evernden, Reference Evernden1992), and between the natural sciences and the social ones (van der Leeuw, Reference van der Leeuw, Ducros, Ducros, Joulian and .1998).
The discovery of other continents in the 15th and 16th centuries, the emergence of Protestantism, the Enlightenment, and the Industrial Revolution in the 18th century and the current information and communication technology revolution are other examples of such breaking points (Castells, Reference Castells1996). Each involved a systemic choice that over time transformed huge domains of noise (potential information) into signals (knowledge).
Henrich (Reference Henrich2020) shows how the information-processing apparatus of Western civilization has grown by selecting an evolving set of increasingly many dimensions, which contributed to the global diffusion of its science, technology, and social organization. Although there are similarities between cultures and historical periods in the development of science, such as the production of a surplus of knowledge that is not directly useful, variations in the fundamental role of the societal embedding of science suggest the existence of alternatives (Berlin, Reference Berlin2002; Schemmel, Reference Schemmel2020). The underlying question is: ‘Why and how are specific innovations generated and adopted, rather than others, thus leading to certain systemic developments in information processing?’ We must look in greater detail at how the dynamics of human information-processing systems generate novelty and thereby impact on their trajectory.
The interaction between a society and its (natural, social, technological, institutional, etc.) environment can be conceived as an interaction between two dynamic niches, an internal, conceptual one (the human information-processing apparatus, including linguistic, cognitive, institutional, and physical elements) and an external, material one (the world humans interact with), which resonate with, and shape, each other (Iriki, Reference Iriki2019). The two niches co-evolve to shape both the information processing of a society and the structure of the environment with which it interacts.
But, while the cognitive categories derived from observations selectively reduce the unbounded complexity of the observed environment in the internal niche, these simplified conceptions are then confronted with the much more complex dynamics in the outside niche. Because of the difference in dimensionality, any human action upon the environment has unperceived, unintended consequences and is subject to ‘ontological uncertainty’ (Lane & Maxfield, Reference Lane and Maxfield2005) – the impossibility to fully predict the outcome of such actions. The rationality of homo economicus constitutes an example of such bias. Keynes noted more generally in the conclusion of his general theory (Keynes, Reference Keynes1936) ‘the ideas of economists and political philosophers, both when they are right and when they are wrong are more powerful than is commonly understood’. The evolution of a society's knowledge structure thus drives the trajectory of human–environmental interaction, but only partly dicts it.
Societal information-processing capacity has expanded through the development of the inside (knowledge) niche. In the process, some of that capacity has been delegated to language, technology, institutions, etc. For that phenomenon, Iriki (Reference Iriki2019) proposes the concept of ‘extended epigenetic evolution’. Concepts, institutions or artifacts, for example, fix certain kinds of information processing in the conceptual, linguistic, material, and technological or institutional realm by ‘crystallizing’ them as specific ‘tools for thought and action’. Bureaucratic systems, taxation principles, as well as many other aspects of the rules of nations direct the behavior of people. They determine that certain actions follow set patterns, short-circuiting part of the information processing required and alleviating the overall information-processing load, as in the case of the many routines based on outdated ideas that are being developed to deal with anticipated catastrophes.
The increasing delegation to technological means constitutes an important aspect of information processing in contemporary societies (e.g. Diamond, Reference Diamond1997). Computers and digital systems are concomitant with an exponential explosion of all aspects of information processing: diversity of data harvested, storage capacity, computational power, real-time processing, instant communication, application domains, etc. An increasing part of information processing is thus routinized and displaced outside the mind, aligning the members of a society. The automatization of information processing is such a paradigm shift, with an intense interplay between ‘memory’ (conservatism) and ‘novelty’ (progressivism) (Gunderson & Holling, Reference Gunderson and Holling2002). What happened? How does this development relate to the mismatch between knowledge and action mentioned above, and to the sustainability conundrum?
4. Computers and automatic information processing
The industrial revolution led to a surge in the complexity of technological, societal, and environmental processes. To cope with that complexity, the information-processing capacity of society had to adapt (Beniger, Reference Beniger1986). Among the numerous industrial challenges of the 19th century, some involved informational ones. Railroad systems could not have grown independently of telegraph networks for example. Both co-evolved in an accelerating feedback loop that resonated between the two niches (Iriki, Reference Iriki2019) through flows of information and matter.
The advent of electronic devices in the mid-20th century discharged humans of an increasing part of their information processing. Two very distinct periods can be distinguished in the way dimensions of knowledge were affected by digitization. In the first period, running essentially since the 1950s, mostly existing categories were being transposed to computers. In the second, starting in the 1990s, new categories emerge from radically different usage of digital processing. This led to two concurrent approaches to digitization, which could respectively be described as conservative and disruptive.
Conservative digitization had a considerable impact on two major sectors: administrative data manipulation and scientific modeling. It led to deep transformations of these sectors themselves and of society as a whole, but without generating radically new cognitive dimensions. Repetitive bureaucratic activities, such as census or accounting, consist essentially of the manipulation of tables with a fixed number of columns corresponding to categories of interest and rows representing the entities, such as individuals. These were represented under abstract formalisms that allowed both automatic manipulation by machines and easy query by humans (Maier, Reference Maier1983). Tasks previously performed by humans were thus done by machines, with a considerable gain in efficiency and reliability, but without fundamental transformation of the tasks themselves or of the information processed, thus maintaining the purpose of the initial sector of activity. The relationship between signals and noise did not change. Only the ways in which signal was encoded, did.
As such computers progressively penetrated all sectors, the necessity to store the corresponding data led to the development of ‘hard’, quantifiable parameters, based on known, well-defined categories, which therefore gained importance at the expense of more subjective appreciations. New signals were created out of thus far ignored noise, further specifying an existing interpretative scheme, rather than modifying that scheme. In the process, the aspiration for a reinforced transparency of public action did contribute to focalization on some specific categories of parameters which, because they had (and have) the public's interest, did get emphasized through an adapted metric.
As for the relation between society and the economy (Polanyi, Reference Polanyi1944), the direction of the interaction has been reversed, humans are increasingly serving the technosystem. Humans devoted considerable amounts of time (they still do) to transfer information to machines.
This trend re-enforced the idea that, within the measured dimensions, everything is under control. In case of obvious inadequacy and to avoid irrelevance, legacy socio-economic sectors attempted to include additional categories to further diminish ontological uncertainty.
One of the intrinsic challenges of such apparently objective mechanisms is that they reinforce the tendency for the resulting categories to represent mostly, often exclusively, phenomena progressively identified by human observers. This can be done by ignoring their interaction with other systems or cognitive dimensions, potentially threatening sustainability itself. Moreover, the search for increased optimization might reach limits, and benefit individual entities to the detriment of the whole system (Grumbach & Hamant, Reference Grumbach and Hamant2020).
A radical shift occurred in the 1990s, when digital platforms were designed to learn from humans by observing their behavior when they use their services. This created new categories, new information out of what until then had been considered noise. This paradigmatic shift has important consequences for the mastery of categories and the control of the flows of information and matter, as we will discuss in a subsequent section.
5. Scientific modeling and environmental knowledge
The construction of the meteorological system from the mid-19th century until now is an excellent example of a positive information-processing feedback loop that involves all activity sectors, leading to the progressive constitution of new signals, new scientific fields, new technological capacities, and entrepreneurial investments as well as international cooperation. All these involve the identification of new signals and the establishment of appropriate metrics. Its success led to the extension of its scope from the weather (short term) to the climate (long term) and the capture of yet completely new cognitive dimensions and signals, institutionalized by the creation in 1988 of the Intergovernmental Panel on Climate Change (IPCC) (Yamin & Depledge, Reference Yamin and Depledge2004).
An important increase in computing power enabled the modeling of the atmosphere as a complex system, further improving the accuracy of predictions. In 1967, a physically realistic digital climate model (Forster, Reference Forster2017), took into account radiative forcing, confirming the greenhouse effect of carbon dioxide (CO2). In 1975, a model of the global climate in three dimensions, integrating the ocean and the ice caps, confirmed that a doubling of the quantity of CO2 in the atmosphere would lead to an increase of more than 2°C (Manabe & Wetherald, Reference Manabe and Wetherald1975). Further progress in modeling enabled the extension of climate models progressively including all the categories known from natural sciences (Edwards, Reference Edwards2011).
After 50 years of modeling, current factual observations confirm the tendency predicted by previous models. The capacity to evaluate predictive models with hindsight ensures confidence in the predictions made by current models for the coming decades on the increase of temperatures resulting from the increase of the proportion of greenhouse gases. Our understanding thus combines real observations and accumulated sensor data with computerized models that include an increasing set of thus far unknown dimensions, such as the complex modeling of albedo and radiative forcing for instance. This was made possible by the capacity to develop new scientific categories (signals), and to progressively include them in computer models. What should be noted is the contemporaneity of the acceleration of the human activity and its dramatic impact on ecosystems with the emergence of the scientific capacity to be aware of such transformations. But, it does not lead to a paradigmatic shift in the organization of societies and their interaction with their environment.
Models were also developed for the societal dynamics. Since Forrester’ initial models used for the Meadows report (Meadows, Meadows, Randers, & Behrens, Reference Meadows, Meadows, Randers and Behrens1972), other alternatives to current economic models have been proposed to capture notions of resilience, which allows the inclusion of yet more dimensions that were initially not perceived at all, considered as negligible, or too complex to deal with.
These models changed the understanding of both the natural environment and the interaction humans have with their environment, but they are conservative in the sense that their mission is to inform humans, but not to interact with the environment whether natural or social. This is in sharp contrast to the digital platforms that emerged since the late 1990s, which radically disrupted society, by ensuring the mediation between human as well as non-human actors on essentially all multi-sided markets (Rochet & Tirole, Reference Rochet and Tirole2003), from knowledge access to ride-sharing. They partially invert the relation between humans and machines in the direction of autonomous machine information processing and take control of resource allocation, as we will see in more detail in what follows. Such cybernetic digital platforms are not designed to enable human control of processes.
The knowledge produced is at the center of heated controversies, but has only a moderate impact on policies. There is a clear mismatch between the dramatic conclusions of, for instance, IPCC reports and the mild policies of nations, exhibiting a serious limitation of our societies' knowledge processing capacity. We claim that information that is currently not processed, either by omission or by inaccessibility, is absolutely central to remedy this policy deficiency. To deepen our insight in the role of both noise and signal, we need to deal with the process of categorization.
6. The role of categorization
In our opinion, a fundamental aspect of the discrepancy between the masses of information gathered, mainly by the natural and life sciences, and the lag in proactive measures to deal with the sustainability conundrum is due to the fact that the sciences collect data within a relatively narrow spectrum that is missing much information that is necessary to deal with the issues. Why is that the case, and how has that bias developed? We argue that this is due to the manner in which data are transformed into information by means of categorization.
A human information-processing system cannot be defined exclusively by the knowledge it explicitly contains and the processes which have been implemented. The phenomena outside its cognitive sphere, which the society has never thought about and of which it might be completely unaware, need also to be taken into account. Here, we label that domain the ‘unknown unknown’. If it is by definition impossible to give examples for today, it is possible for the past. DNA forensics belonged to the unknown unknown a century ago.
Another domain, of which the society is aware but has not managed coherent information processing, is here called the ‘known unknown’ – it is the domain of cognized noise out of which signals could emerge. There, we find beliefs that are not, or only feebly, related to observations, such as the Ancient Greeks' ideas about the god Helios (the sun) as the regulator of day and night. Modern examples abound, such as the ‘laws’ of market equilibrium, or the belief in the superiority of western democratic systems, rationalized by invoking semi- or pseudo-scientific arguments.
Once the links between phenomena, observations, and the human knowledge system densify, effective structuration of the cognitive framework is beginning in the form of categorization. That process has been studied in detail by Kahneman and others (Kahneman, Reference Kahneman2012; Kahneman, Slovic, & Tversky, Reference Kahneman, Slovic and Tversky1982). It is based on pattern identification, a comparison between similarities and dissimilarities among the phenomena observed. Initially, the category to be created is the subject and the phenomena studied to do so are the referent. According to Tverski and Gati (Reference Tverski, Gati, Rosch and Lloyd1978), this is done with a bias in favor of similarity, uniting phenomena into ‘open’ categories (groups) by distinguishing similar characteristics. In the next stage, the process is inverted: the categories become the referents, the phenomena the subjects, and the comparisons are biased toward dissimilarity, thus determining which phenomena among those initially included do not belong to the categories established. That allows the remainder of the phenomena to be transformed into closed categories (classes) transformed into definitions. These definitions then co-determine further investigations and classifications.
The balance between the closed categories, anchoring the tradition that shaped the society's existing world view and the open categories for which hypotheses are under construction decides between continuity and change. The choices made in both phases of the categorization process are fundamental for the resultant information-processing structure, as they determine which phenomena are taken into account in sustainability investigations, and which are considered ‘noise’. The exploration of noise and the creation of new categories are thus essential to enable socio-environmental transformations relative to the sustainability conundrum.Footnote 1
As the interaction between open and closed categories proceeds, information processing is structured, enriched, and refined. At certain points transitions occur between different conceptions of people's mental map of the world, such as scientific paradigm shifts (Kuhn, Reference Kuhn1962) changing the appreciation of the outside world, as well as the method by which signals are identified and information is organized. A perception of the outside world that reckons with the undefined and the existence of unknown dynamics, alternates with one that is deemed more predictable because it takes more defined dimensions of variability into account. This fundamentally changes the course the system's trajectory may take. Taleb (Reference Taleb2008) argues that as a result of that process, our current perception of the world is simplified and regularized into ‘knowledge’ dominated by closed categories, which no longer takes the unforeseen sufficiently into account, and might be driven apart from the actual dynamics. By implication the sustainability conundrum is to an important extent due to an increasing dependency on closed categories that do not explore uncertainty and noise, part of the mid-20th century dream that ultimately everything could be understood by science.
It is therefore worthwhile to consider some of the implications of Henrich's (Reference Henrich2020) idea that Western science has created a very particular cognitive system. Taleb (Reference Taleb2008) argues that this increasingly generally accepted vision overemphasizes the controllability of the human and natural environments in which our societies are functioning. He draws upon the ‘ex ante’ perspective that is inherent in the complex adaptive systems approach, focusing on identifying novelty by looking at system trajectories from the past to the future, in contrast to most of science's ‘ex post’ perspective which looks from the present to the past in an attempt to identify the origins of the present. In that latter light, most, if not all, emergent phenomena are unanticipated. The world is thus subject to ontological uncertainty, even though the scientific perspective implies that those phenomena are fully understood and predictable.
In other words, in the course of its evolution, Western science has exchanged a high-dimensional perspective, formulated in open categories, for a low-dimensional one, formulated in closed categories. The former was part understood, ‘fuzzy’ and polythetic, while the latter thought to be fully understood. This has fundamentally changed our appreciation of the uncertainties and risks involved in our interactions with the environment, resulting in a false sense of control and, over time, an accumulation of unanticipated consequences of those interactions. Moreover, as part of the feedback loop that Taleb points to, the very closure of the system of categories permitted (engineering and social) interventions which eroded the very assumption of controllability by contributing their own unintended consequences – which then intensified the search for enhanced control. Sustainability which belongs to a sphere with ontological uncertainty becomes a conundrum when reduced to a sphere of fictitious control. One can summarize this loop in the figure above. Moreover, while current Western science has made an important contribution to our understanding of part of the world around us, it also contributed to the fragmentation of our human perspective on the environment, as is highlighted by a study of Scoones (Reference Scoones2016), which shows how the environmental, political, and economic domains that are clearly relevant to the sustainability debate have been isolated in different conceptual ‘boxes’ depending on combinations of technology-led, market-led, state-led, and citizen-led processes, and how this has affected our perspective on the central issue of resource availability that is crucial to sustainability. In that process, many part-understood dimensions of the ‘real’ world have been ignored, and its complexity over-simplified. One of these is the relationship between sustainability and societal equity (Leach et al., Reference Leach, Reyers, Bai, Brondizio, Cook, Díaz, Espindola, Scobie, Stafford-Smith and Subramanian2018); another relationship between sustainability and the impact of colonialism. These are both examples where the replacement of open, multidimensional, and polythetic categories by closed ones has relegated important aspects of sustainability to ‘noise’ (Figure 3).

Figure 3. Open/closed categories feedback loop.
How does the digital disruption affect this situation? As we will see in the next sections, (1) the volume of information processed has expanded exponentially, (2) the structure of human information processing has changed, and (3) so have some of the tools that explore the known unknown, and potentially even the unknown unknown. This is a transformation whose qualitative impact might exceed its quantitative one, and whose long-term repercussion is underestimated. But, more importantly, neither of them can be considered independently of the sustainability issue.
7. The emergence of digital platforms and autonomous information processing
The exponential growth of data collection and processing capacities radically changed the structure of information-processing systems (Bratton, Reference Bratton2015). It became possible to instantiate data that had previously never been revealed, such as the continuous move of a person in space, or variation of temperature at a given geographical location, not to mention the DNA of living organisms. Such data are now stored on material means in data centers. That led to an increasing hierarchization of the information, in the non-human processing sphere in which humanity has embedded itself.
Today, close to a hundred billion (1011) objects (humans, machines, places, etc.) are now connected continuously. Super nodes, mostly intermediation platforms such as Google, Amazon, Apple, Tencent, or Alibaba, enjoy extremely high centrality connecting billions of people and objects thanks to decision-making based on algorithmic processing. Such an information processing toolkit never existed before. It surpasses the potential of statistics by orders of magnitude, leading to an increasing information asymmetry with other components of society, such as legacy power systems (Grumbach, Reference Grumbach2019). How does this work?
Intermediation platforms connect players with each other to exchange directly in two- or even multi-sided markets (Langley & Leyshon, Reference Langley and Leyshon2017; Rochet & Tirole, Reference Rochet and Tirole2003), such as the relations between producers and consumers of goods or services (e.g. Amazon) or drivers and passengers (e.g. Uber), etc. These new digital markets revolutionize whole sectors of activity, removing the producers of these services from their position as mediators and loosening their connections with their clients. The emergence of the new digital intermediaries generates new signals, new dimensions of understanding and new categories, and changes the ways in which they are handled and valued. It also promotes new means of communication and new services to produce and access knowledge, such as social networks or search engines. Their importance in domains such as education and health forces traditional actors to reposition themselves. The COVID-19 pandemic accelerated this evolution (Kenney & Zysman, Reference Kenney and Zysman2020).
In general, such intermediation platforms are purely digital, they do not produce the goods or the services, but ensure only the connection and if necessary the payment. Platforms thus further dis-embed information from matter and energy, thanks to their overall penetration of the datasphere in the social, economic, and political sector.Footnote 2 The platforms’ physical infrastructures, such as the data centers, are not necessarily in the territory in which the exchanges take place.
The overall architecture of the datasphere is structured into three levels: (1) the core formed by large data centers; (2) the connection infrastructure and the intermediary companies; and (3) the periphery formed by terminals such as PCs, smartphones, sensors, and on-board equipment of all kinds. That global architecture is regulated and managed through complex interactions between States and corporations (Nye, Reference Nye2014).
This architecture has created two extreme opposites, the gigantic data centers and the tiny terminals and sensors. Terminals have benefited from increasing miniaturization and integration; they could reach 150 billion by 2025 (among which 1 billion video surveillance cameras as of 2020), twenty times more terminals of all kinds than humans. According to IDC (Rydning et al., Reference Rydning, Reinsel and Gantz2018), digitization affects all information flows, regardless of the activities involved, causing massive growth in the volume of data generated, from 33 zetta-bytes (a byte is a unit of digital information consisting of 8 bits) in 2018 to 175 in 2025. The recently introduced unit of zetta, (1021), corresponds to the diameter of the Milky Way in meters! Most of these data come from systems of control and interaction with people, machines, and spaces. An autonomous car, for example, can generate 3 terabytes per h.
The novel information generated includes some that until now was in the sphere of the known–unknown, if not the unknown–unknown. Financial market information, in the past in the known–unknown solely ruled by humans, is now largely under the control of algorithms. The alchemy of social norms and beliefs, rooted in the unknown–unknown, is now transformed by digital platforms, although resulting from explicit processing of information.
Many new categories thus emerge with algorithmic interaction fundamentally supported by new operating modes. Unlike legacy organizations, which extend the number of categories after analysis and ask humans to inform the machines by filling forms, platforms compute the new categories automatically. Recommendations are also made automatically, based on profile comparisons. Open categories are thus created by machines and evolve dynamically with sophisticated algorithmic techniques, such as A/B testing (Siroker & Koomen, Reference Siroker and Koomen2013), and can nudge people on markets as well as in politics (Morozov, Reference Morozov2014). Humans no longer determine what is signal, and what is noise, and this fundamentally changes the means at societies' disposal to deal with environmental or societal challenges. In the field of sustainability, most of the information is provided by automatic monitoring systems, and is then analyzed by AI to find relevant patterns, estimating the evolution of climate or agriculture for instance.
Systems initially developed for commercial purposes are thus increasingly used to make, or at least help make, decisions regarding people on fundamental issues such as health, justice, or education for instance. On what ground an algorithm makes choices is unclear, as is which human actors would be responsible for a given decision and its consequences (Diakopoulos, Reference Diakopoulos2016). Increasingly, humans lose understanding of what algorithms do, and therewith insight in, or control of, social processes. Although initially designed by humans, algorithms are increasingly hard to apprehend in their design, behavior, and outcome at runtime. Google's Internet services software, for instance, has 2 billion lines of code (Metz, Reference Metz2015). Moreover, algorithms can hardly ever be proven correct.
In the last decade, AI has enjoyed important successes due to the exponential growth of computing power, the availability of huge datasets, and a complete methodological turnaround. After years of unsuccessful attempts to tell the machine what to do with categories introduced by humans, the idea is now to let the machine learn how to solve the problem from innumerable examples and follow its own strategy, creating its own categories. The methodology differs from the scientific approach, searching for simple correlations rather than causality.
Therefore, systems which are mostly driven by algorithms, control an increasing part of the exchanges humans have. These systems' power challenges the power of States. It seems no accident that these new forms of control of human interactions emerge at a time when humanity reaches hard environmental limits (Rockström et al., Reference Rockström, Steffen, Noone, Persson, Chapin, Lambin, Lenton, Scheffer, Folke, Schellnhuber, Nykvist, de Wit, Hughes, van der Leeuw, Rodhe, Sörlin, Snyder, Costanza, Svedin and Foley2009), and must introduce new measures to ensure sustainability. Platforms probably have the greatest potential to ensure new operational modes.
8. An inexorable information machinery driven by powerful feedback loops
Where will this lead to in the future? Some platforms enjoy extremely dominant positions (Argenton & Prüfer, Reference Argenton and Prüfer2012). Google, for instance, has about 95% of the search engine market in most of Europe. Some powerful feedback loops contribute to this concentration, which differ from marginal cost considerations and are more powerful than network effects (Belleflamme & Peitz, Reference Belleflamme, Peitz, Corchón and Marini2018). The positive feedback loops for search engines constitute a good illustration of how platforms grow. The fundamental loop is shown in Figure 4.
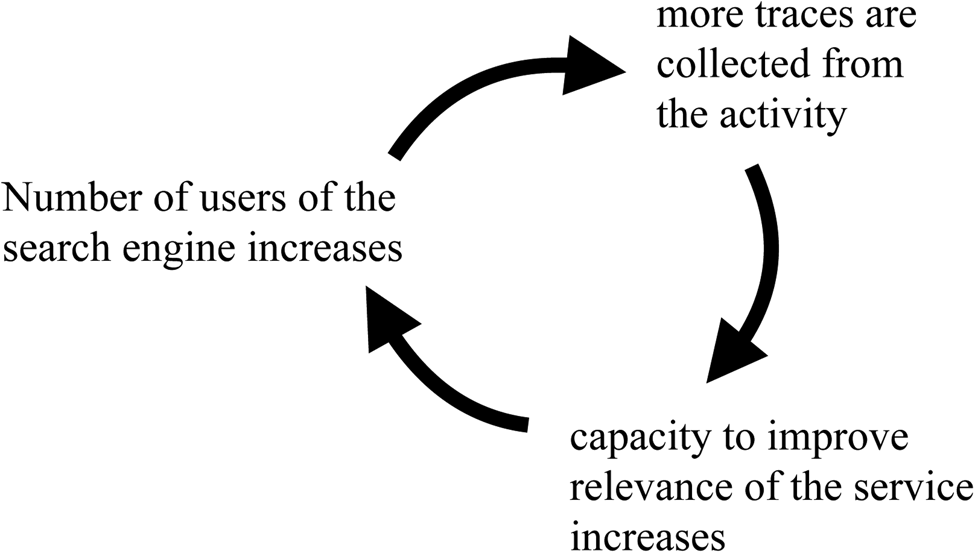
Figure 4. Search engine feedback loop.
Other feedback loops reinforce the basic one. They concern for instance the economic model of the engine, which in most cases relies on services involving secondary data, such as the traces of the engine's users, which are under the full control of the platforms (Figure 5).

Figure 5. AdWords feedback loop.
The number of users of course impacts the interest of advertisers. Since AdWords are sold using an auction system, prices go up with the interest in the platform (Mehta et al., Reference Mehta, Saberi, Vazirani and Vazirani2007). As prices go up, advertisers invest in a larger spectrum of words, exploiting less expensive ones. The monetization of searches thus increases, and so does the profit. A complex nexus of feedback effects is exploited, which reshape the structure of information processing in societies, with very strong information asymmetries. The search engine can derive global knowledge, such as the incidence of an epidemic, political inclinations, economic trends, as well as specific knowledge about individuals and communities such as leading groups or governments, unless these protect themselves from foreign or adverse intelligence. Special modules for research or patents provide a unique, unchallenged picture of the state of the art in science, technology, or intellectual property.
Similar positive feedback loops apply to intermediation, which co-evolve driven by the platforms. They deploy invasive forms of control of people, based on criteria that are only partly decided by humans. They concern progressively all dimensions of human life. Although this phenomenon has been widely described and provokes heated controversies (Zuboff, Reference Zuboff2015), it continues to surprise entire sectors of society, which are then completely disrupted. Education, for example, will almost certainly undergo massive restructuring in the coming decades, of equal importance to the Humboldtian revolution (Nybom, Reference Nybom2003), as will health systems and the intermediation between patients, research, pharmacy, insurance, etc. (Van Dijck & Poell, Reference Van Dijck and Poell2016). In both sectors, a shift toward sustainability might occur, favoring long-term objectives. In transportation systems, global platforms might balance individual interest with global constraints related, among others, to meteorological, pollution, or traffic conditions, favoring common interests as well as long-term sustainability goals.
This quest for digitization seems inexorable, shaking the fundamental equilibria of society. It is unclear what equilibria might emerge from interaction with counter-forces. Regulation has had marginal effects. The digital revolution is driven by very powerful feedback loops that reflect tensions between what is known and what needs to be known for the correct functioning of societies. These loops currently favor generalization of control, centrality of its main actors, and their distance from the ground.
Let us now consider in more detail some of the feedback loops that drive this evolution and entail major changes in social norms, with potential impact on sustainability.
Some loops are related to the venture capital strategy, contributing to the growth of a selected actor in a sector relative to others, and explaining the domination of one app for each service. Others are related to technological structures. Their feedback is powerful enough to sustain the irresistible growth of these systems. The increasingly sensitive nature of data poses growing problems of security for hosts as well as of trust for suppliers, individuals, administrations, or companies, which strengthens specialization in data storage and processing (Singh & Chatterjee, Reference Singh and Chatterjee2017), as well as delegation of data management to third parties. This contributes to further concentration of the industry. The development of data centers is accelerating and, conversely, local storage needs are decreasing. If in 2010 approximately one-third of data was stored in data centers, and two-thirds in edge capacity, this ratio is expected to reverse by 2025, while the majority of data will continue to be created at the periphery.
Interestingly, such concentration also plays at the geographical level. Very different strategies have been implemented in the USA, East Asia, and Europe, with diverging visions of the role of States and platforms, although in all regions the trend toward biopolitics (Haraway, Reference Haraway2020) is growing very fast. Biopolitics promotes new forms of governance, relations between governments and people based on dimensions continuously monitored and thus allowing real-time interactions, from nudging to coercion. These dimensions can favor the short-term equilibrium of society (e.g. control of debt, health, etc.), as well as its resilience and long-term sustainability (e.g. control of ecosystemic interactions, personal carbon rationing, etc.).
Science fiction has for a long time considered potential consequences of singularity (Vinge, Reference Vinge1993), in which information processing by machines becomes superior to – and independent from – humans. Such singularity is more and more taken seriously in the industrial realm, as a potentially harsh future reality. Machines are enjoying an increasing independence from human inputs. One striking example is that 20 years after the success of IBM's machine Deep Blue against Kasparov in chess, DeepMind produced the machine AlphaGo that beat the Go champion Ke Jie in 2017. AlphaGo, which learned Go from games played by humans, was later beaten by AlphaGo Zero, which was trained against itself without human experience of the game.
Deep-learning techniques allow playing with variable amounts of human knowledge, from supervised learning, which uses pre-established human categories, to unsupervised learning, where machines create categories without such knowledge. Code and integrated circuit design are increasingly conceived by machines, of which humans have limited understanding due to the complexity of the systems. Machines evolve into dealing with the known unknown and potentially the unknown unknown without human grasp of the categories involved, thus creating and expanding knowledge structures independent of the human categorization process. This evolution will further accelerate. In a seminal paper, Bloom et al. (Reference Bloom, Jones, Van Reenen and Webb2020) show that while research efforts are rising substantially, research productivity is declining sharply, particularly in information technology, agriculture, or medical innovation. This results in the emergence of new cognitive dimensions, which matter for human societies but are not understood or controlled by humans.
This question of control is important in a large spectrum of very sensitive legal, ethical, economic, or political issues, such as determining if a war machine can decide to kill a human without human interaction. But, beyond the issue of control and beyond problematic applications, there is the question of the societal acceptance of decisions taken by machines that are beyond human explanation. That question is directly related to how societies deal with the sphere of the known–unknown and the unknown–unknown, and might have very different answers depending upon the culture involved.
9. Conclusion
We are currently facing a unique new challenge: how to integrate into human information-processing sets of categories that emerged independent from human observation and were processed by machines beyond human control. The question we need to ask is how the balance between the known and the unknown, between phenomena under control, and those outside our cognitive sphere, might evolve and trigger transitions to future dynamic equilibria.
We have seen how the expansion of human information-processing frameworks leads to the creation of new categories, which can then be fitted into the extended knowledge system. Integrating machine-created categories into existing knowledge systems might constitute an important challenge. Could it result in discontinuities in the information-processing systems, or in our cognitive capacities? Would we adapt our human information processing, and the behavior that is based on it, to the knowledge generated by machines? What would be the time-frame to do so? Since most human behavior is deeply anchored in very ancient cultures, shifting to a different category- and knowledge system might require a long time-frame. But, on the other hand, could the need to adapt to a deteriorated environment and to transition toward more resilient organizations force changes to occur in a relatively short time-frame? Could this lead to divergent paths in different cultures, or bring such cultures closer together?
Different transitions are currently operating in East Asia and North America, the two regions with the strongest leadership in the digital transformation. Could the less emphasis on the nature/culture divide in Asia (Callicott & Ames, Reference Callicott and Ames1989) favor the extension of the digital domain and its categories? East Asia might be setting new norms that foster harmony and co-existence between humans, nature, and autonomous machines, while the Western perspective adheres to the notion of progress for human society and control by technological means. The outcomes might be different. One possible path could be a progressive weakening of the current world order, with its norms and institutions established by Western cultures, toward new normative frameworks promoted by Asia (Chu & Zheng, Reference Chu and Zheng2020; Dalio, Reference Dalio2020; Ramo, Reference Ramo2004).
Acknowledgments
The authors wish to thank Gary Dirks and Pablo Jensen as well as the two anonymous reviewers for their precious comments and fruitful suggestions on an earlier draft of the paper.
Author contributions
Both authors contributed equally to the conception and the writing of the present paper.
Financial support
Arizona State University provided travel support for this project.
Conflict of interest
None.