1 Introduction
Let
$(X,f)$
be a topological dynamical system (t.d.s. for short), that is,
$(X,d)$
is a compact metric space and
$f:X\rightarrow X$
is a continuous self-map. Preimage entropies were introduced and studied by Langevin and Przytycki [Reference Langevin and Przytycki6], Hurley [Reference Hurley5], Nitecki and Przytycki [Reference Nitecki and Przytycki7], and Fiebig, Fiebig and Nitecki [Reference Fiebig, Fiebig and Nitecki3]. These quantities give relevant information of how ‘non-invertible’ a system is. Among these entropy-like invariants, there are two kinds of pointwise preimage entropies:


where
$s(n,{\epsilon },Z)$
(or
$s(n,{\epsilon },Z,f)$
) denotes the largest cardinality of any
$(n,{\epsilon })$
-separated set of
$Z\subset X$
. An important question is: can one introduce the counterpart of
$h_m(f)$
or
$h_p(f)$
from the measure-theoretic point of view, and obtain a variational principle relating them?
The first progress on this research was made by Cheng and Newhouse [Reference Cheng and Newhouse1]. They defined a new notion of topological preimage entropy:

On the measure-theoretic side, they defined a corresponding measure-theoretic preimage entropy:

where
${\alpha }$
ranges over all finite partitions of X,

and
$\mathcal {B}^-$
is the infinite past
$\sigma $
-algebra
$\bigcap _{n\geq 0}f^{-n}\mathcal {B}$
related to the Borel
$\sigma $
-algebra
$\mathcal {B}$
. In addition, they stated a variational principle:

where
$\mathcal {M}(X,f)$
denotes the set of all f-invariant Borel probability measures on X.
Recently, Wu and Zhu [Reference Wu and Zhu9] developed a variational principle for
$h_m(f)$
under the condition of uniform separation of preimages. They introduced a new version of pointwise metric preimage entropy:

where
${\alpha }$
ranges over all finite partitions of X and

For f with uniform separation of preimages, the authors [Reference Wu and Zhu9] established the following variational principle relating
$h_{m,\mu }(f)$
and
$h_m(f)$
:

In fact, it was shown in [Reference Wu and Zhu10, Proposition 3.1] that

For related definitions of topological and measure-theoretic entropies, we refer to the books [Reference Downarowicz2, Reference Glasner4, Reference Walters8].
In this note, we shall give an example to show that

So the variational principle in equation (1.1) is not true.
2 Main result
In this section, we will state and prove our main result.
Lemma 2.1. Let
$A=\{0, 1, 2\}$
and endow
$A^{\mathbb {N}\times \mathbb {N}}$
with the product topology of the discrete topology on A. Denote by
$f: A^{\mathbb {N}\times \mathbb {N}}\to A^{\mathbb {N}\times \mathbb {N}}$
the left shift map on rows; that is,

For each array
$x=(x_{m,i})_{m,i\geq 0}$
, denote by
$i_0(x)$
the minimal
$i\geq 0$
such that
$x_{0,i}=0$
. If such an i does not exist, then we set
$i_0(x)=\infty $
. Let
$X\subset A^{\mathbb {N}\times \mathbb {N}}$
consist of arrays such that:
-
(1) for all
$i\geq i_0(x)$ and all
$m\geq 0$ , we have
$x_{m,i}=0$ ;
-
(2) for all
$0\leq i< i_0(x)$ and all
$m\geq 0$ , we have
$x_{m,i}\in \{1,2\}$ and if both
$m\geq 1$ and
$i\geq 1$ , then
$x_{m,i}=x_{m-1,i-1}$ .
For the t.d.s
$(X,f)$
, we have
$h_{\mathrm {pre}}(f)\geq \log 2$
and
$h_{\mathrm {pre},\mu }(f)=0$
for any
$\mu \in \mathcal {M}(X,f)$
.
Proof. For
$0\leq n\leq \infty $
, let
$A_n$
denote the set of points
$x\in X$
with
$i_0(x)=n$
and
$\textbf {0}$
denote the array consisting of just zeros. Then we have the following observations.
-
(1)
$A_0=\{\textbf {0}\}$ and the element
$\textbf {0}$ has infinitely many preimages.
-
(2) Any element
$x\in X\setminus A_0$ has exactly one preimage.
-
(3)
$(A_\infty ,f)$ is an invertible subsystem.
Let
${\epsilon }_0>0$
be so small that
$x,y\in X$
with
$x_{0,0}\neq y_{0,0}$
implies
$d(x,y)\geq {\epsilon }_0$
. Note that if we just observe the zero-row of
$f^{-n}(\textbf {0})$
, we will see elements starting with any block of any length
$0\leq k\leq n$
over
$1,2$
(followed by zeros). So we have

Hence,
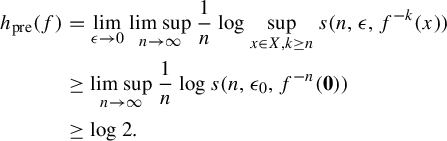
Now we pass to evaluating the measure-theoretic preimage entropy. Notice that for each
$0<n<\infty $
, the set
$A_n$
is visited by any orbit at most once implying that
$\mu (A_n)=0$
for any
$\mu \in \mathcal {M}(X,f)$
. So, any invariant measure
$\mu $
is supported by
$A_0\cup A_\infty $
. Fix
$\mu \in \mathcal {M}(X,f)$
. Without loss of generality, we may assume that
$\mu (A_0)>0$
and
$\mu (A_\infty )>0$
. Consider the conditional measures

It is easy to verify that both
$\mu _{A_0}$
and
$\mu _{A_\infty }$
are invariant and
$\mu =\mu (A_0)\mu _{A_0}+\mu (A_\infty )\mu _{A_\infty }$
. By the affinity of measurable conditional entropy (see, for example, [Reference Downarowicz2, Theorem 2.5.1], [Reference Cheng and Newhouse1, Theorem 2.3] or [Reference Wu and Zhu9, Proposition 2.12]), we have
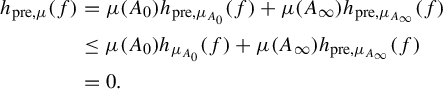
By Lemma 2.1, we can get our main result.
Theorem 2.2. There exists a t.d.s.
$(X,f)$
such that

Thus, the Cheng–Newhouse variational principle in equation (1.1) fails.
3 Another definition of preimage entropy
In [Reference Cheng and Newhouse1], the authors show that
$h_{\mathrm {pre}}(f)$
can also be defined as

This result is based on their variational principle in equation (1.1). Now we shall give a topological proof of equation (3.1). In fact, it is a consequence of the following result.
For
$Z\subset X$
, let
$r(n,{\epsilon },Z)$
denote the smallest cardinality of any
$(n,{\epsilon })$
-spanning set of
$Z\subset X$
. It is clear that the above topological notions of entropies defined by separated sets can also be defined by spanning sets.
Theorem 3.1. Let
$f:X\to X$
be a continuous map. Then,
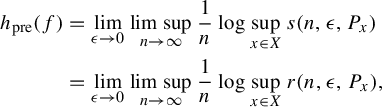
where
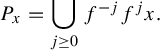
Proof. Fix
$y\in X$
,
$n\in \mathbb {N}$
and
$k\geq n$
. If
$f^{-k}y\neq \emptyset $
, then pick
$x\in f^{-k}y$
. So,

which implies

Next, we show the remaining inequality. Fix
$s>h_{\mathrm {pre}}(f)$
. For any
${\epsilon }>0$
, there exists
$N\in \mathbb {N}$
such that

for all
$x\in X$
,
$n\geq N$
and
$k\geq n$
.
Fix
$x\in X$
,
$n\geq N$
. For
$k\geq n$
, let
$E_k\subset X$
be an
$(n,{\epsilon })$
-spanning set of
$f^{-k}f^kx$
with
$\#E_k=r(n,{\epsilon },f^{-k}f^kx)\leq e^{sn}$
. Let
$K(X)$
be the space of non-empty closed subsets of X equipped with the Hausdorff metric. Then we have
$E_k\in K(X)$
. As X is compact,
$K(X)$
is also compact. So there exists a subsequence
$\{k_j\}_{j\geq 1}$
such that
$E_{k_j}\rightarrow E(j\to \infty )$
. Then we have
$\#E\leq e^{sn}$
.
We claim that
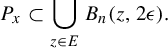
To see this, pick
$y\in P_x$
. Then there exists
$J\geq n$
such that for any
$j\geq J$
, one has
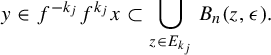
Furthermore, we can pick
$z_{k_j}\in E_{k_j}$
to get

Without loss of generality, we assume that
$\lim _{j\to \infty }z_{k_j}=z$
. Then it is easy to see that
$z\in E$
and

So the claim is true. Hence, we have
$r(n,2{\epsilon },P_x)\leq \#E\leq e^{sn}$
, from which one can get

By the choice of s, we obtain the reversed inequality.
Acknowledgements
I would like to thank the referee for valuable suggestions that greatly improved the manuscript. This work was supported by National Nature Science Foundation of China (Grant No. 12001192)