Introduction
Severe acute respiratory syndrome coronavirus 2 (SARS-CoV-2) is a highly transmissible respiratory pathogen (Li et al., Reference Li, Lu and Zhang2020) that causes coronavirus disease 19 (COVID-19). Although COVID-19, as of May 2023, is not a global emergency anymore (Solis et al., Reference Solis, Franco-Paredes, Henao-Martínez, Krsak and Zimmer2020; World Health Organization, 2022), it remains a pandemic (World Health Organization, 2023) causing distress, morbidity and mortality. Furthermore, the condition of long COVID, referring to persistent symptoms such as cognitive impairment, fatigue and low mood, poses an emerging challenge (Suran, Reference Suran2023). Little is known about the risk factors of long COVID, and the role of mental health in the modulation of that risk is unclear, given that although a pre-existing mental health diagnosis may be an independent risk factor (Lam et al., Reference Lam, Damant, Ferrara, Lim, Stickland, Ogando, Power and Smith2023), it may also mitigate the psychological burden of long COVID (Rastogi et al., Reference Rastogi, Cerda, ElTohamy, Chen, Stevens and Liu2023).
There are inter-individual differences when it comes to SARS-CoV-2 susceptibility, the course that COVID-19 takes and its outcome. Compromised immune functioning, poor health behaviour, sleep, somatic comorbidities and exposure to chronic stress, all related to infection risk and disease course, are often present in people with poor mental health (Chireh et al., Reference Chireh, Li and D’Arcy2019; Chrousos, Reference Chrousos2009). Therefore, they may be susceptible to SARS-CoV-2 and a relatively poor COVID-19 course (Simon et al., Reference Simon, Schenk, Palm, Faltraco and Thome2021).
An early meta-analysis on the potential effects of (pre-existing) mood disorders on SARS-CoV-2 infection risk found no evidence for the existence of this association (Ceban et al., Reference Ceban, Nogo, Carvalho, Lee, Nasri, Xiong, Lui, Subramaniapillai, Gill, Liu, Joseph, Teopiz, Cao, Mansur, Lin, Rosenblat, Ho and McIntyre2021). However, the authors did find that having a pre-existing mood disorder was associated with increased chances of COVID-19-related hospitalization and death. This is in line with 3 other meta-analyses reporting a relatively poor COVID-19 course in people with (pre-existing) mental disorders (Fond et al., Reference Fond, Nemani, Etchecopar-Etchart, Loundou, Goff, Lee, Lancon, Auquier, Baumstarck, Llorca, Yon and Boyer2021a; Toubasi et al., Reference Toubasi, AbuAnzeh, Tawileh, Aldebei and Alryalat2021; Vai et al., Reference Vai, Mazza, Delli Colli, Foiselle, Allen, Benedetti, Borsini, Casanova Dias, Tamouza, Leboyer, Benros, Branchi, Fusar-Poli and De Picker2021). The meta-analyses unequivocally found high levels of between-study heterogeneity in outcome, which remained unexplained. Given that behavioural parameters related to susceptibility (e.g., poor social distancing) and health behaviour may be different in people with various kinds of mental disorders, we considered an updated meta-analysis stratified by type of mental disorder valuable at this stage. An additional reason for an update is that dozens of primary studies have become available since the publication of the earlier meta-analyses. Therefore, we here aim to optimize and specify the findings of earlier studies using a new and broader systematic review and meta-analysis. We will apply a frequentist (i.e., classical) and Bayesian approach to meta-analysis. The frequentist approach will be used to provide effect-size estimates and for significance testing of the null hypothesis. The Bayesian approach complements this with the strength of evidence estimates for the null and the alternative hypotheses (Heck et al., Reference Heck, Boehm, Böing-Messing, Bürkner, Derks, Dienes, Fu, Gu, Karimova, Kiers, Klugkist, Kuiper, Lee, Leenders, Leplaa, Linde, Ly, Meijerink-Bosman, Moerbeek, Mulder, Palfi, Schönbrodt, Tendeiro, van den Bergh, Van Lissa, van Ravenzwaaij, Vanpaemel, Wagenmakers, Williams, Zondervan-Zwijnenburg and Hoijtink2022; Keysers et al., Reference Keysers, Gazzola and Wagenmakers2020).
We hypothesize that SARS-CoV-2 infection risk, including breakthrough infection, and COVID-19 course, including long COVID, are worse and that mortality rates and the need for COVID-19-related care are high for people with mental disorders relative to people without these disorders. We will explore and review potential explanations, with an emphasis on variables that are open to change such as stress coping and dietary behaviour. Finally, we will discuss the implications for prevention and treatment.
Methods
We followed the MOOSE (Stroup et al., Reference Stroup, Berlin, Morton, Olkin, Williamson, Rennie, Moher, Becker, Sipe and Thacker2000) and PRISMA guidelines (Moher et al., Reference Moher, Liberati, Tetzlaff and Altman2009), and drafted and preregistered a review protocol at the website of the Open Science Foundation (https://osf.io/35jhm/registrations).
Identification and selection strategy
Web-based searches were performed in PubMed, EMBASE, Web of Science and Google Scholar, which is the optimal database combination for a systematic literature search according to Bramer et al. (Reference Bramer, Rethlefsen, Kleijnen and Franco2017). Database-specific search strings are presented in the online supplement. The final date for the systematic search was 27 June 2023. Reference lists of reviews and meta-analyses were hand-searched for eligible data. A grey literature search on the preprint servers PsyArXiv.org and MedArXiv.org was also performed. Decisions on eligibility were based on titles and abstracts of candidate papers, and ultimately on full-text assessment. At least two members of the review team made a final decision on the eligibility of these articles, based on the in- and exclusion criteria.
Inclusion and exclusion criteria
Articles were included when they (1) reported SARS-CoV-2 infection risk and/or course of COVID-19 for people with pre-existing mental disorders versus controls without these disorders, and (2) were written in English, German, French, Spanish, Arabic or Dutch. Articles were excluded when (1) no relevant outcome data could be extracted, even after we had been in contact (or had made reasonable attempts hereto) with the corresponding author of the article, or when (2) no original data were reported (e.g., opinion pieces). When articles reported on overlapping data sets, we included the article that was most informative for our purposes (see supplementary material, Box S1 for the rules that we set for article selection and the efforts that were undertaken to avoid the multiple inclusion of a single dataset).
Exposure and outcome variables
Exposure variables were pre-existing anxiety, depressive, neurodevelopmental, schizophrenia spectrum and substance use disorders (SUDs) assessed according to diagnostic systems such as Diagnostic and Statistical Manual of Mental Disorders, Fourth or Fifth Edition (DSM-IV or V) (American Psychiatric Association, 2013; World Health Organization, 1992) or International Statistical Classification of Disease and Related Health Problems, Tenth Revision (ICD-10) (WHO, 1992). Control conditions were composed of people without the mental disorders of our interest (e.g., the comparison of people with a SUD vs. people without a SUD) and not per se people without any disorder (i.e., the healthy control). Outcome variables included (1) infection risk – including break-through infection –, presented as a percentage of SARS-CoV-2 positive tests/self-reports in the populations under study and (2) COVID-19 course variables, further specified as (a) indicators of the severity of the disease (e.g., symptomatic vs. non-symptomatic, requiring respiratory assistance or not), (b) hospitalization rates, (c) intensive care unit (ICU) admission rates, (d) presence of symptoms of long-COVID/persistent COVID-19 symptoms of any kind (Byrne, Reference Byrne2022) and (e) COVID-19-related mortality rates. Please note that at the time of preregistration at the OSF, we considered the outcome variable long COVID as part of the course variables and not as a separate one.
Data extraction
From included papers, we extracted demographic data (e.g., gender distribution), clinical data (e.g., diagnosis), suspected virus type (estimated from the time frame in which the data were collected), methodological data (e.g., study types) and outcome data (i.e., raw numbers or effect-size estimates and corresponding 95% confidence intervals (95% CIs) on outcome data). Data extraction was performed independently by two members (AR, TY (research assistant), MM) of the review team.
Measures of effect
We extracted odds ratios (ORs) and corresponding 95% CIs as measures of effect. Where reported, we extracted data from analyses that controlled for the largest number of potential confounders or that came from propensity-matched samples. When results were reported as hazard ratios (HRs) or risk ratios (RRs) and raw data were not available, we interpreted these as an OR when the incidence of the reported outcome was <20%. HRs and RRs based on data reporting on an incidence of the outcome of ≥20% were transformed (Davies et al., Reference Davies, Crombie and Tavakoli1998; Grant, Reference Grant2014; Zhang and Yu, Reference Zhang and Yu1998).
Assessment of methodological quality
The methodological quality of input studies was scored by two members of the review team using the Quality Assessment Tool for Observational Cohort and Cross-sectional Studies recommended by the United States National Institutes of Health (2021). The items of this instrument are presented as supplement (Table S1).
Statistical analysis
We performed analyses in Stata version 17 (StataCorp, 2021) and JASP (Jeffreys’s Amazing Statistics Program; JASP team, 2017) and created summary tables on the characteristics of the included studies. Random-effects meta-analyses were used to pool the data on SARS-CoV-2 infection rates, breakthrough infection rates, COVID-19 course, hospitalizations, ICU admissions, long COVID and mortality rates to pre-existing mental disorders. Statistical significance was set at P < 0.05. Heterogeneity among studies was quantified using the I 2 measure and assessed for statistical significance using the Q 2-statistic (Sterne et al., Reference Sterne, Bradburn and Egger2001). When heterogeneity in outcome was present, subgroup and meta-regression analyses were performed to identify study characteristics that might explain the heterogeneity. Potential continuous variables that served as candidates were gender (% females), average age, methodological quality scores and sample size (N). Sensitivity analyses were used to assess the potential effects of controlling for confounders and the time frame of diagnostic assessment (e.g., lifetime vs. current) and virus variant (e.g., Alpha vs. Beta). Data on the latter variable were estimated based on the time frame in which the data were gathered and the geographic location where this was done. We followed the advice of the Cochrane handbook to interpret results from sensitivity and moderator only when there were at least 10 studies available per meta-regression analysis (Higgins and Thomas, Reference Higgins and Thomas2021). Note that analyses on main outcomes (e.g., mortality) were all a priori registered. Most meta-regression analyses and subgroup analyses were not. Publication bias was assessed using visual inspection of funnel plots and the Eggers regression test (Sterne et al., Reference Sterne, Bradburn and Egger2001). All frequentist meta-analyses were repeated by making use of a Bayesian random-effects approach to confirm the robustness of results and to present, using a Bayes Factor (BF10), the strength of evidence for the null or the alternative hypothesis. BFs were calculated for an effect-size estimate (logOR) of 0.00 with a standard deviation of 0.50. We used the thresholds suggested by Heck et al. (Reference Heck, Boehm, Böing-Messing, Bürkner, Derks, Dienes, Fu, Gu, Karimova, Kiers, Klugkist, Kuiper, Lee, Leenders, Leplaa, Linde, Ly, Meijerink-Bosman, Moerbeek, Mulder, Palfi, Schönbrodt, Tendeiro, van den Bergh, Van Lissa, van Ravenzwaaij, Vanpaemel, Wagenmakers, Williams, Zondervan-Zwijnenburg and Hoijtink2022) for the interpretation of the BF10s.
Results
Table S2 (online supplement) lists all the articles that were included for full-text assessment as well as reasons for inclusion and exclusion. See Figure 1 for a flow chart and further information on the identification, screening and inclusion of studies. Eighty-one of the 23,194 candidate articles (0.4%) reported data that met the eligibility criteria. Fourteen of the articles reported data from a prospective design (17%) and 68 from a retrospective design (83%) (see Table S3). More than three-quarter of the data included here were not included in any of the four previous meta-analyses (Ceban et al., Reference Ceban, Nogo, Carvalho, Lee, Nasri, Xiong, Lui, Subramaniapillai, Gill, Liu, Joseph, Teopiz, Cao, Mansur, Lin, Rosenblat, Ho and McIntyre2021; Fond et al., Reference Fond, Nemani, Etchecopar-Etchart, Loundou, Goff, Lee, Lancon, Auquier, Baumstarck, Llorca, Yon and Boyer2021a; Toubasi et al., Reference Toubasi, AbuAnzeh, Tawileh, Aldebei and Alryalat2021; Vai et al., Reference Vai, Mazza, Delli Colli, Foiselle, Allen, Benedetti, Borsini, Casanova Dias, Tamouza, Leboyer, Benros, Branchi, Fusar-Poli and De Picker2021; see Table S4). The surplus of articles that we report on results from an accretion of primary articles and from differences in inclusion criteria. In Box S2, we describe some specific actions that were undertaken to avoid data overlap within the analysis.
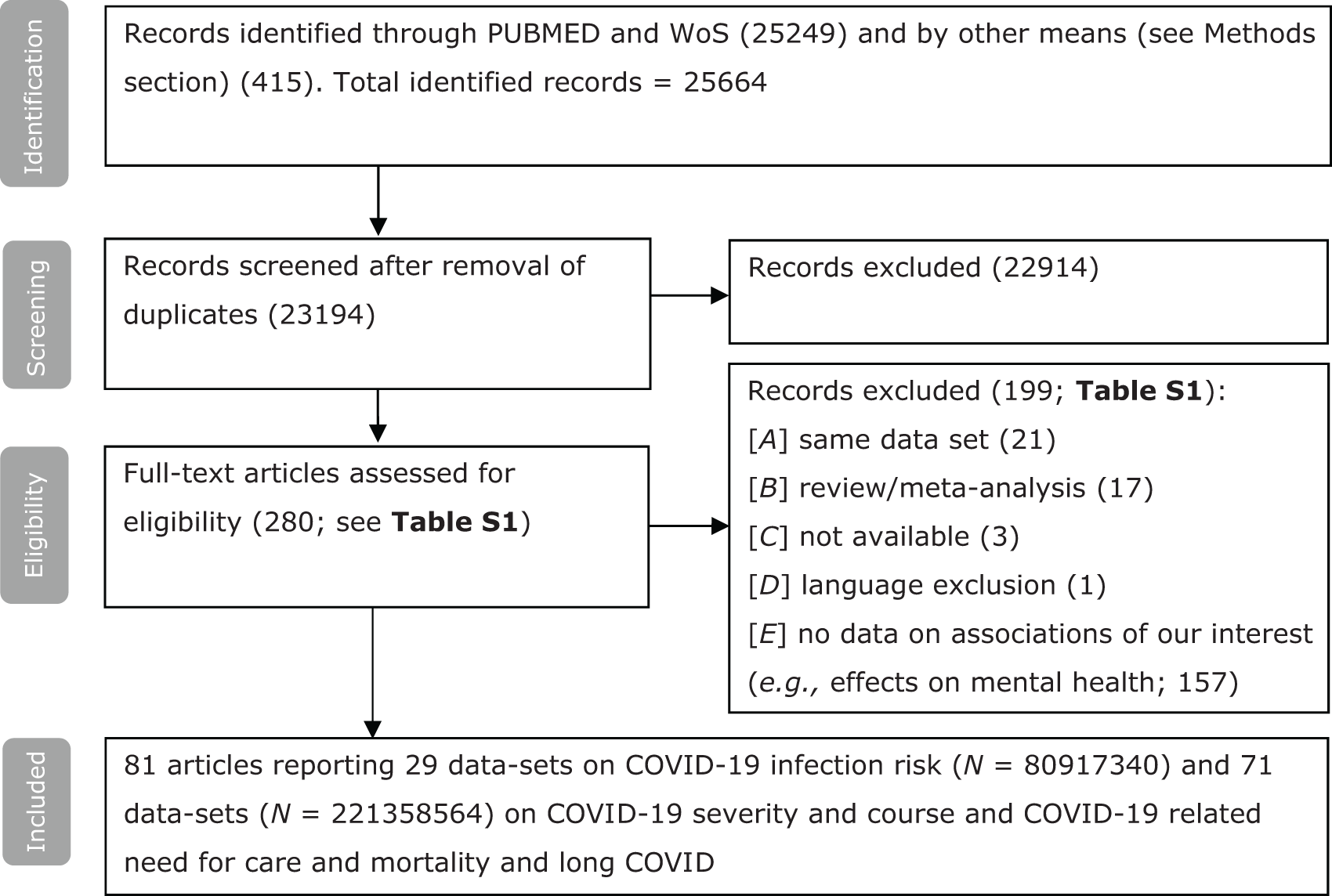
Figure 1. Flow chart on identification, screening and inclusion of eligible publications.
Table 1 provides demographic and clinical information on the samples from the input studies that we included. Tables S3–S5 provide further information on the method and assessment of predictor variables, outcome variables (S5), control conditions (S3), virus variants (S4), COVID-19 diagnostics (S5) and covariates used in analyses (S3). All except three studies reported on the comparison of people with a specific disorder versus people without this disorder. COVID-19 data were serologically confirmed in all studies, with two exceptions in which the diagnosis was gathered by self-diagnosis or through self-report. Psychiatric diagnostic spectra were most often defined by ICD-10 criteria (World Health Organization, 1992) followed by DSM-IV and V criteria (American Psychiatric Association, 1994, 2013).
Table 1. Characteristics of the studies included and samples by outcome

Abbreviations: AUD = alcohol use disorder; AV = average, MED = median, NK = not known, SUD = substance use disorder; Mental disorders; the study groups and reports on several mental disorders as 1 group; Several categories, the study reports separate associations on several categories of mental disorders.
a Includes data on breakthrough infection.
b Includes data on long COVID/persistent symptoms.
The median and mean ages of the included samples ranged between 9 and 81 years. The percentage of females per sample ranged from 16% to 86%. The median sample size per analysis was 114837 (range, 61–73,099,850). The methodological quality of most input studies was moderate to high (see Table S6 for scores per study).
SARS-CoV-2 infection risk
SARS-CoV-2 infection risk was not significantly different in populations of people with a mental disorder versus those in populations without a mental disorder (see Table 2). Subcategories of neurodevelopmental disorders (i.e., autism spectrum disorder and attention-deficit/hyperactivity disorder (ADHD)) and mood disorders (i.e., depressive disorder and bipolar disorder) were tested with regard to outcome, but neither of these was associated with an increased risk. Bayesian meta-analyses yielded BFs suggesting either anecdotal or moderate evidence favouring the null hypothesis of no effect of mental disorder on the risk to acquire SARS-CoV-2 (see Table 2).
Table 2. Results from frequentist and Bayesian meta-analyses

* P < 0.05, **P < 0.01, ***P < 0.001.
a Estimates come from analyses including nationwide data, at the expense of local data. Estimates from analyses favouring local data (e.g., data from studies performed in North-East London, Manchester and Bristol) over data from the UK Biobank over nationwide data (e.g., the UK Biobank) in different combinations is presented in Tables S6 and S7. In all cases, pooled effect sizes fell within in the 95% CIs when the nationwide data was replaced by more local data.
b Evidence category for the results from Bayesian analyses: + anecdotal evidence for H0 or H1; ++ moderate evidence for H0 or H1; +++ strong evidence for H0 or H1; ++++ very strong evidence for H0 or H1
Between-study heterogeneity in outcome was evident in all analyses (see Table 2). Analysis by time frame of diagnostic assessment (e.g., lifetime vs. past year/current) and statistical control for confounders by input studies (categorical; yes vs. no) yielded similar results. The continuous variables that were tested as effect moderators (i.e., percentage of females, average age, methodological quality and sample size) were not associated with outcome (see Table S8). We found evidence for publication bias in the data on psychosis spectrum disorders and infection risk (see Table 2). Accounting for this using trim-and-fill methods did not result in a different estimate. We estimated per sample the dominant virus variant for each specific study. About 80% of studies investigated the Alpha variant and the remaining studies were either a mix of the Alpha and Beta variant or the Beta variant. There was no evidence that the observed associations were driven by a specific virus variant. Overall, results from analyses on local and nationwide data were similar (see Table S7 and dataset S1).
Two studies specifically compared breakthrough infection risk in people with a mental disorder versus controls without the mental disorders under investigation (Nishimi et al., Reference Nishimi, Neylan, Bertenthal, Seal and O’Donovan2022; Wang et al., Reference Wang, Quan, Ding, Kang, Koenen, Kubzansky, Branch-Elliman, Chavarro and Roberts2022c). Both studies gathered nationwide data in the United States. To avoid pooling of dependent data, statistical analyses were not performed and a narrative review of the results will be presented. Nishimi et al. (Reference Nishimi, Neylan, Bertenthal, Seal and O’Donovan2022) show, based on data from the department of veteran affairs (N = 263,697), a small significant increase in the risk of breakthrough infection in patients with several kinds of mental disorders (range adjusted relative RRs: 1.03–1.16). Wang et al. (Reference Wang, Quan, Chavarro, Slopen, Kubzansky, Koenen, Kang, Weisskopf, Branch-Elliman and Roberts2022b) report data on 579,372 individuals from the TriNetX network showing an overall increased breakthrough infection risk in patients with several types of SUDs relative in comparison to propensity-matched controls who were free of SUDs (range HRs: 1.06–2.06).
COVID-19 severity
Consistent over all categories, we find that people with pre-existing mental disorders show higher odds to experience a severe COVID-19 course, once infected, relative to people in control conditions (pooled ORs range, 1.26–2.32; see Table 2). Bayesian meta-analysis aligned with these results and show that the evidence favouring the alternative hypothesis is in the range from anecdotal (for anxiety disorders) to very strong (for SUDs) (see Table 2).
COVID-19-related hospitalization, ICU admission and mortality
Associations with COVID-19-related hospitalization, ICU admission and mortality were also largely consistent over predictor categories, with higher odds for people in the patient categories relative to people in the control condition who were free of the specific diagnosis that we related to outcome (pooled ORs range, 1.05–1.93). Results from Bayesian meta-analys in line with the results from those derived through the classical frequentist approach. The evidence for the hypotheses of the existence of an association between having mental disorders and COVID-19-related hospitalization and morbidity was notably strong in most cases. For the outcome of ICU admission, the level of evidence was only anecdotal or moderate (see Table 2).
Between-study heterogeneity in outcome was evident in most analyses (see Table 2). Results over subcategories (e.g., bipolar and unipolar depression for mood disorders) were largely similar. Moreover, results were not driven by a particular virus variant. The time frame of psychiatric assessment (e.g., lifetime vs. past year/current) and statistical control for confounders by input studies (categorical; yes vs. no) was not differentially associated with changes in outcome. There were two exceptions. One, the association between mood disorders and hospitalization was stronger for people with a current versus a lifetime disorder (z = 2.85, P < 0.01). Two, uncontrolled studies investigating the association between psychosis spectrum disorder and COVID-19-related mortality yielded higher effect-size estimates relative to studies that applied statistical control (e.g., matching, the use of co-variates) (z = 5.83, P < 0.001). Analyses of local and nationwide data yielded consistent results (see Table S9 and dataset S1). Associations were not moderated by geographic location, average age, percentage of females and methodological quality (see Table S10). In a few analyses, we found evidence of publication bias (Table 2). Controlling for this using trim-and-fill procedures did not result in substantially different estimates (data not shown).
Long COVID
Eight studies reported on the association between pre-existing mental disorders and long COVID/persistent symptoms. [Al-Aly et al., Reference Al-Aly, Bowe and Xie2022; Bellan et al., Reference Bellan, Apostolo, Albè, Crevola, Errica, Ratano, Tonello, Minisini, D’Onghia, Baricich, Patrucco, Zeppegno, Gramaglia, Balbo, Cappellano, Casella, Chiocchetti, Clivati, Giordano, Manfredi, Patti, Pinato, Puricelli, Raineri, Rolla, Sainaghi and Pirisi2022; de Miranda et al., Reference de Miranda, Gomes, Filgueiras, Corsini, Almeida, Silva, Medeiros, Vilela, Fernandes and Grenfell2022; Durstenfeld et al., Reference Durstenfeld, Peluso, Peyser, Lin, Knight, Djibo, Khatib, Kitzman, O’Brien, Williams, Isasi, Kornak, Carton, Olgin, Pletcher, Marcus and Beatty2023; Hedberg et al., Reference Hedberg, Granath, Bruchfeld, Askling, Sjöholm, Fored, Färnert and Naucler2023; Jones et al., Reference Jones, Davis, Stanley, Julious, Ryan, Jackson, Halpin, Hickman, Pinnock, Quint, Khunti, Heaney, Oliver, Siddiqui, Pavord, Jones, Hyland, Ritchie, Young, Megaw, Davis, Walker, Holgate, Beecroft, Kemppinen, Appiagyei, Roberts, Preston, Hardjojo, Carter, van Melle and Price2021; Thompson et al., Reference Thompson, Williams, Walker, Mitchell, Niedzwiedz, Yang, Huggins, Kwong, Silverwood, Di Gessa, Bowyer, Northstone, Hou, Green, Dodgeon, Doores, Duncan, Williams, Steptoe, Porteous, McEachan, Tomlinson, Goldacre, Patalay, Ploubidis, Katikireddi, Tilling, Rentsch, Timpson, Chaturvedi and Steves2022; and Wang et al., Reference Wang, Wang, Davis, Volkow and Xu2022a (total N = 6,350,939)]. Relative to the other outcomes, these studies on long COVID quite often applied a prospective design (50% vs. 11%). Both Jones et al. and Thompson et al. report on UK nationwide primary care data. To avoid overlapping data, we once ran a meta-analysis with Jones et al. included and Thompson et al. excluded and one with Jones et al. excluded and Thompson et al. included. Al-Aly et al. (Reference Al-Aly, Bowe and Xie2022) and Wang et al. (Reference Wang, Wang, Davis, Volkow and Xu2022a) report on two large US-based cohorts. These however were not deemed to present overlapping data due to the populations under study, which were composed of veterans and healthcare professionals respectively. All the included data sets showed that pre-existing mental disorders are associated with the presence of long COVID, although between-study heterogeneity in outcome was present (P-values < 0.001). Pooled ORs ranged between 1.68 and 1.75, with corresponding P-values < 0.0001. Bayesian analyses showed that the evidence for the alternative hypothesis of a negative effect of pre-existing mental disorders on long COVID symptoms is extremely strong (BF10 range, 205–421). Due to a lack of data, we were not able to run analyses per type of mental disorder.
Discussion
Our meta-analysis of 81 original studies and a total of 304 effect size estimates shows that people with pre-existing mental disorders are not more likely to acquire SARS-CoV-2 relative to control conditions of people without these disorders. However, people with pre-existing mental disorders have increased morbidity and mortality rates and need for care related to COVID-19 once infected, and long COVID symptoms relative to people without pre-existing mental disorders. Although effect-size estimates are small to medium, Bayesian analyses showed that in many cases the evidence favouring the alternative hypothesis is very strong (e.g., mortality and hospitalization rates for people with psychosis spectrum disorder and SUDs).
Pre-existing mental disorders and susceptibility to SARS-CoV-2 infection
We expected increased SARS-CoV-2 infection risk in people with pre-existing mental disorders relative to people in control conditions without these disorders. Increased susceptibility however was not evident in the data. Results from Bayesian meta-analyses showed either anecdotal or moderate evidence favouring the null hypothesis of no effect of mental disorder on the risk to acquire SARS-CoV-2 (see Table 2).
A reason for not finding the expected associations could be that infection is less likely in case of loneliness and social deprivation, which are relatively common in people with mental disorders and even more so during the pandemic (Pai and Vella, Reference Pai and Vella2022). Future studies are needed to address the relevance and contributions of these factors and their potential interactions with risk factors for infection that are evident in people with mental disorders, amongst which are poor physical fitness/co-morbid somatic conditions (Barton et al., Reference Barton, Zagler, Engl, Rihs and Musil2020), malnutrition (Mahboub et al., Reference Mahboub, Rizk, Karavetian and de Vries2021), smoking (Yuan et al., Reference Yuan, Yao and Larsson2020), risk-taking behaviours and impulsivity (Kreek et al., Reference Kreek, Nielsen, Butelman and LaForge2005) and socioeconomic and minority status (Butler, Reference Butler2021; Mena et al., Reference Mena, Martinez, Mahmud, Marquet, Buckee and Santillana2021).
Pre-existing mental disorders and morbidity and mortality rates for COVID-19 and long COVID
Another main finding of the current study is that people with most types of pre-existing mental disorders are disproportionally affected by COVID-19 once infected relative to people without pre-existing mental disorders. Our group found similar associations for pre-existing neurodegenerative diseases (Smadi et al., Reference Smadi, Kaburis, Schnapper, Reina, Molero and Molendijk2023). Theoretically, this might be a direct result of relatively low socioeconomic status, especially when it comes to limitations on access to care. An in-depth analysis of COVID-19-related mortality in the city of Santiago, Chile, showed that people with low socioeconomic status had fewer testing opportunities, faced relatively long waiting lists and struggled with delayed test results. These circumstances correlate with increased morbidity and mortality rates (Mena et al., Reference Mena, Martinez, Mahmud, Marquet, Buckee and Santillana2021).
A complementary explanation (Wang et al., Reference Wang, Ahorsu, Lin, Chen, Yen, Kuo, Griffiths and Pakpour2021) may be that people with (especially severe) mental disorders often lack the motivation, energy and/or insight to go out for testing (e.g., due to disorganization, negative symptoms, cognitive dysfunction or delusional thinking). This may result in late detection and, with that, delayed medical care. Moreover, in this group, too, compromised immune functioning and poor physical fitness probably contribute (Beurel et al., Reference Beurel, Toups and Nemeroff2020; Chireh et al., Reference Chireh, Li and D’Arcy2019; Wei et al., Reference Wei, Chen, Zhang and Cheng2020). Studies indicate that, on average, people with mood, schizophrenia spectrum and SUDs have increased inflammatory markers (Beurel et al., Reference Beurel, Toups and Nemeroff2020; Dowlati et al., Reference Dowlati, Herrmann, Swardfager, Liu, Sham, Reim and Lanctôt2010; Fraguas et al., Reference Fraguas, Díaz-Caneja, Ayora, Hernández-Álvarez, Rodríguez-Quiroga, Recio, Leza and Arango2019) relative to control conditions of people without these disorders and hence an increased vulnerability to SARS-CoV-2 infection.
Clinical data show that a dysregulated – pro-inflammatory – immune status predicts a poorer course and higher mortality rate in patients with COVID-19. Although the exact mechanisms that underlie this association are yet unknown, it has been suggested that a compensatory inflammatory response that dysregulates the adaptive immune response might play a role here (Henry et al., Reference Henry, Benoit, Vikse, Berger, Pulvino, Hoehn, Rose, Santos de Oliveira, Lippi and Benoit2019). Therefore, testing the cellular immune response and blood glucose levels has potential prognostic value in COVID-19 patients with pre-existing mental disorders and/or SUDs. In the chronic pro-neuroinflammatory environment that characterizes at least some subcategories of mental disorders, T cells show maladaptive characteristics in terms of a higher CD4/CD8 ratio, along with a decreased cellular immune response in depressive disorder (Toben and Baune, Reference Toben and Baune2015). A lymphocyte-mediated mechanism with an altered CD4/CD8 ratio has been implicated in the pathogenesis of alcohol-related liver injury (Batey et al., Reference Batey, Cao and Gould2002), and an altered CD4/CD8 ratio has been observed in people with schizophrenia spectrum disorders (Al-Diwani et al., Reference Al-Diwani, Pollak, Irani and Lennox2017). Interestingly, an imbalance of the lymphocyte subpopulations, characterized by reduced counts of CD4+ and CD8+ T cells and an increase of natural-killer lymphocytes has been implicated as an early marker of mortality in inpatients with COVID-19 (Cantenys-Molina et al., Reference Cantenys-Molina, Fernández-Cruz, Francos, Lopez Bernaldo de Quirós, Muñoz and Gil-Herrera2020).
Allostatic overload
To contextualize the mechanisms referred to above, the concept of allostatic overload may be useful. Allostatic overload refers to the damage sustained by biological systems due to repeated and/or prolonged stress (McEwen, Reference McEwen2013). Chronic stress plays an important role in the aetiology and disease course of many types of mental disorders (Juster et al., Reference Juster, McEwen and Lupien2010; Spitzer et al., Reference Spitzer, Barnow, Völzke, John, Freyberger and Grabe2009). Compromised immune functioning and poor physical fitness often go hand in hand with exposure to chronic stress through direct neuro-endocrine routes or indirect routes through stress effects on health behaviour and sleep (Glaser and Kiecolt-Glaser, Reference Glaser and Kiecolt-Glaser2015; McEwen, Reference McEwen2013). Prolonged stressors and excessive activity of stress mediators are associated with glucocorticoid-receptor resistance, which interferes with the appropriate regulation of inflammation, and this is an important aspect in the onset and progression of a wide range of diseases (Cohen et al., Reference Cohen, Janicki-Deverts, Doyle, Miller, Frank, Rabin and Turner2012; De Kloet et al., Reference De Kloet, Joëls and Holsboer2005), including COVID-19. Glucocorticoid-receptor resistance is linked to higher levels of stress and inflammatory mediators, and hence a sustained pro-inflammatory state (De Kloet et al., Reference De Kloet, Meijer, de Nicola, de Rijk and Joëls2018; Glaser and Kiecolt-Glaser, Reference Glaser and Kiecolt-Glaser2015; Keller et al., Reference Keller, Gomez, Williams, Lembke, Lazzeroni, Murphy and Schatzberg2017), including immune dysregulation of brain microglia (Wohleb et al., Reference Wohleb, Franklin, Iwata and Duman2016), a characteristic feature of, in particular, atypical or a hypothesized immune-metabolic form of depression (Lamers et al., Reference Lamers, Milaneschi, de Jonge, Giltay and Penninx2018).
In addition, high blood glucose levels (e.g., due to metabolic syndrome in people with schizophrenia spectrum disorders and major depressive disorders) may be important mediators of COVID-19 progression and severity (Logette et al., Reference Logette, Lorin, Favreau, Oshurko, Coggan, Casalegno, Sy, Monney, Bertschy, Delattre, Fonta, Krepl, Schmidt, Keller, Kerrien, Scantamburlo, Kaufmann and Markram2021). Stress hormones are major and direct determinants of blood glucose levels and as such, stress can set the stage for insulin resistance. Hence, in a worst-case scenario, chronic stress can mediate a COVID-19-vulnerable phenotype (Pal and Bhadada, Reference Pal and Bhadada2020). Another potentially relevant factor is major histocompatibility complex variation, which has been implicated in the development of schizophrenia spectrum disorders and bipolar disorder (The International Schizophrenia Consortium 2009). This variability may cause dysfunctional T-cell–mediated immune responses, which can contribute to COVID-19 progression, and hence to higher severity and mortality of the infection (Chen and John Wherry, Reference Chen and John Wherry2020; Müller and Schwarz, Reference Müller and Schwarz2010).
Clinical and policy implications
The results of this study have several clinical implications. From a preventive perspective, patients with mental disorders should be considered at high risk for a poor COVID-19 prognosis. This should inform vaccination policies and educational campaigns, especially in areas with limited access to care for these population groups. Given that high levels of blood glucose and pro-inflammatory markers appear to be important mediators of COVID-19 progression and severity (Lamers et al., Reference Lamers, Milaneschi, de Jonge, Giltay and Penninx2018; Logette et al., Reference Logette, Lorin, Favreau, Oshurko, Coggan, Casalegno, Sy, Monney, Bertschy, Delattre, Fonta, Krepl, Schmidt, Keller, Kerrien, Scantamburlo, Kaufmann and Markram2021), our results are also relevant to the pharmacotherapy of depressive disorders, schizophrenia spectrum disorders and SUDs.
Healthy blood glucose profiles depend at least partly on the type of antidepressant or antipsychotic prescribed. Regarding antidepressants, serotonin reuptake inhibitors exert a moderate beneficial effect on the glucose levels of patients with diabetes mellitus and depression (Baumeister et al., Reference Baumeister, Hutter and Bengel2012). Likewise, serotonin–noradrenaline reuptake inhibitors (McIntyre et al., Reference McIntyre, Soczynska, Konarski and Kennedy2006), agomelatine and bupropion seem safe (Roopan and Larsen, Reference Roopan and Larsen2017), whereas tricyclic antidepressants elevate the risk of type-2 diabetes mellitus (Wang et al., Reference Wang, Kaelber, Xu and Volkow2021c). Since monoaminoxidase inhibitors may promote hypoglycaemia (McIntyre et al., Reference McIntyre, Soczynska, Konarski and Kennedy2006), with these compounds there is a need for optimal control in patients with diabetes. As for antipsychotic drugs, healthier blood glucose profiles can be obtained by prescribing some classic rather than atypical antipsychotics (Libowitz and Nurmi, Reference Libowitz and Nurmi2021; Zhang et al., Reference Zhang, Gallego, Robinson, Malhotra, Kane and Correll2013), such as fluphenazine or haloperidol, except for aliphatic phenothiazines (chlorpromazine and levomepromazine (Haupt and Newcomer, Reference Haupt and Newcomer2001). In case atypical antipsychotics are indicated, healthy profiles can be promoted by prescribing aripiprazole (Baker et al., Reference Baker, Pikalov, Tran, Kremenets, Arani and Doraiswamy2009; van Winkel et al., Reference van Winkel, De Hert, Wampers, Van Eyck, Hanssens, Scheen and Peuskens2008), ziprasidone (Sacher et al., Reference Sacher, Mossaheb, Spindelegger, Klein, Geiss-Granadia, Sauermann, Lackner, Joukhadar, Müller and Kasper2008; Simpson et al., Reference Simpson, Glick, Weiden, Romano and Siu2004) or lurasidone (McEvoy et al., Reference McEvoy, Citrome, Hernandez, Cucchiaro, Hsu, Pikalov and Loebel2013), especially in comparison with olanzapine (Koller and Doraiswamy, Reference Koller and Doraiswamy2002), risperidone (Koller et al., Reference Koller, Cross, Doraiswamy and Schneider2003), quetiapine (Koller et al., Reference Koller, Schneider, Bennett and Dubitsky2001) and clozapine (De Hert et al., Reference De Hert, Hanssens, van Winkel, Wampers, Van Eyck, Scheen and Peuskens2007). In cases of antipsychotic-related diabetes, the use of standard antidiabetic medication may be helpful (Cernea et al., Reference Cernea, Dima, Correll and Manu2020). This seems especially important in first psychotic episodes and for young patients (Saddichha et al., Reference Saddichha, Manjunatha, Ameen and Akhtar2008).
From a nutritional perspective, there is preliminary evidence that ketosis induced by a dietetic intervention may contribute to the mitigation of neuro-inflammation via the inhibition of glutamate activity in astrocytes (Morris et al., Reference Morris, Maes, Berk, Carvalho and Puri2020). Dietary interventions therefore should also be considered as a protection against COVID-19. Perez-Araluce et al. (Reference Perez-Araluce, Martinez-Gonzalez, Fernández-Lázaro, Bes-Rastrollo, Gea and Carlos2021) indicate the relevance of this in a study that reports relatively large risk reductions in people adhering to high-quality Mediterranean diets. A similar point can be made for stress-reduction techniques (Callus et al., Reference Callus, Bassola, Fiolo, Bertoldo, Pagliuca and Lusignani2020).
Our study also underlines the importance of using independent data (Cheung, Reference Cheung2019). A re-analysis that we made of previous studies indicates that Toubasi et al. (Reference Toubasi, AbuAnzeh, Tawileh, Aldebei and Alryalat2021) may have included UK Biobank data twice (Batty and Gale, Reference Batty and Gale2021; Yang et al., Reference Yang, Li, Zhang, Zhang, Cheung, Ng and Xiang2021). We suspect that Ceban et al. (Reference Ceban, Nogo, Carvalho, Lee, Nasri, Xiong, Lui, Subramaniapillai, Gill, Liu, Joseph, Teopiz, Cao, Mansur, Lin, Rosenblat, Ho and McIntyre2021) also double-counted UK Biobank data (Van der Meer et al., Reference Van der Meer, Pinzón-Espinosa, Lin, Tijdink, Vinkers, Guloksuz and Luykx2020; Yang et al., Reference Yang, Chen, Hu, Chen, Zeng, Sun, Ying, He, Qu, Lu, Fang, Valdimarsdóttir and Song2020), as well as US nationwide data (Taquet et al., Reference Taquet, Luciano, Geddes and Harrison2021; Wang et al., Reference Wang, Liu, Li, Liu and Wu2021d), while Fond et al. (Reference Fond, Nemani, Etchecopar-Etchart, Loundou, Goff, Lee, Lancon, Auquier, Baumstarck, Llorca, Yon and Boyer2021a) did so with nationwide data from Korea (Jeon et al., Reference Jeon, Kwon, Park and Shin2020; Lee et al., Reference Lee, Yang, Moon, Yoo, Ha, Kim, Park, Choi, Lee, Ahn, Kim, Koh and Yon2020) and Vai et al. (Reference Vai, Mazza, Delli Colli, Foiselle, Allen, Benedetti, Borsini, Casanova Dias, Tamouza, Leboyer, Benros, Branchi, Fusar-Poli and De Picker2021) in their analysis on mortality (An et al., Reference An, Lim, Kim, Chang, Choi and Kim2020; Lee et al., Reference Lee, Yang, Moon, Yoo, Ha, Kim, Park, Choi, Lee, Ahn, Kim, Koh and Yon2020). This double-counting data, can give a false impression of precision (Cheung, Reference Cheung2019) and we put great effort in avoiding this (e.g., see Box S1 in the online supplement).
Strengths and limitations
Our analyses on SARS-CoV-2 infection rate by mental disorder have precedence in the literature, and the findings that we report on COVID-19 course variables are roughly similar to those reported by previous meta-analyses (Ceban et al., Reference Ceban, Nogo, Carvalho, Lee, Nasri, Xiong, Lui, Subramaniapillai, Gill, Liu, Joseph, Teopiz, Cao, Mansur, Lin, Rosenblat, Ho and McIntyre2021; Fond et al., Reference Fond, Nemani, Etchecopar-Etchart, Loundou, Goff, Lee, Lancon, Auquier, Baumstarck, Llorca, Yon and Boyer2021a; Toubasi et al., Reference Toubasi, AbuAnzeh, Tawileh, Aldebei and Alryalat2021; Vai et al., Reference Vai, Mazza, Delli Colli, Foiselle, Allen, Benedetti, Borsini, Casanova Dias, Tamouza, Leboyer, Benros, Branchi, Fusar-Poli and De Picker2021). The dataset that we composed and our analytical approach, however, have some strengths over them. First, we present results stratified by mental disorder and do so for different outcomes. Second, we believe that our approach stands out in the extensive efforts that we took to avoid reporting on overlapping data sets.
Our results indicate the existence of associations between various pre-existing mental disorders and a relatively poor COVID-19 course but do not prove these associations, because the underlying data was purely observational and often was cross-sectional. Vaccination status is a key determinant for all outcomes that we report on. On the one hand, studies indicate that people with mental disorders are less likely to have themselves fully vaccinated (Hartonen et al., Reference Hartonen, Jermy, Sõnajalg, Vartiainen, Krebs, Vabalas, Leino, Nohynek, Sivelä, Mägi, Daly, Ollila, Milani, Perola, Ripatti and Ganna2023; Mazereel et al., Reference Mazereel, Van Assche, Detraux and De Hert2021). On the other hand, some countries, (e.g., the Netherlands) prioritized vaccination of people with mental disorders. Future studies are necessary to investigate the extent to which vaccination moderates the associations between pre-existing mental disorders and COVID-19 course.
The control conditions that were used in most of the input studies also constitute a limitation. All studies (with three exceptions) reported data on convenience samples in which people with a disorder were compared to people without the disorder (e.g., SUD vs. no SUD). The use of such control conditions comes with limitations. The concept of psychiatric comorbidity is ignored. Associations that we now assign to for instance a SUD, can easily reflect associations that are due to a SUD comorbid with depression or psychosis. Data exist that indeed suggests that patterns of comorbidity can be the driving force underlying the relatively poor COVID-19 course observed in people with mental disorders (Schieber et al., Reference Schieber, Dunphy, Schieber, Lopes-Cardozo, Moonesinghe and Guy2023).
The publication time frame of the input studies makes that by far most studies that we included investigated the original SARS-CoV-2 Wuhan Alpha virus. This is an important advantage regarding homogeneity, but also a disadvantage because new variants (i.e., Gamma, Delta, Omicron) could not be considered. The predictor categories in our study were more fine-grained than those in earlier meta-analyses (e.g., Toubasi et al., Reference Toubasi, AbuAnzeh, Tawileh, Aldebei and Alryalat2021), but still represent heterogeneous categories (Feczko et al., Reference Feczko, Miranda-Dominguez, Marr, Graham, Nigg and Fair2019). Possibly, this resulted in at least some of the between-study differences in outcome. Large samples were included, and our meta-analyses were well-powered, but for moderator and subgroup analyses this may have not been the case. The Cochrane handbook advises interpreting the results derived from meta-regression only when there are ≥10 studies available per analysis (Higgins and Thomas, Reference Higgins and Thomas2021). Sometimes, we reported results based on fewer studies. Furthermore, we show that covariate-adjusted and matched analyses yield similar results relative to uncontrolled analyses. However, results from controlled and matched analyses still can be confounded in case the full set of covariates is not considered. A good example here is medication status. The use of many types of psychotropic medication could be related to outcome (see above). This however is not specifically addressed in most records for us to exclude this as an alternative explanation. It should be noted here that while the analyses on main outcomes (e.g., mortality) were all a priori registered, most meta-regression and subgroup analyses were not. Finally, we included studies that were written in English, German, French, Spanish, Arabic or Dutch and may have missed relevant data because they are written and published only in, e.g., Mandarin.
Summary and conclusion
The infection risk for SARS-CoV-2 infection is rather similar in people with mental disorders relative to control conditions. Yet, once infected, a more severe COVID-19 course was observed for all mental disorders that we studied. Hospitalization rates were relatively high in people with mental disorders; moreover, they are more likely to die from COVID-19 relative to controls. We conclude that patients with pre-existing mental disorders are (behaviourally and biologically) disadvantaged when it comes to coping with the disease. This conclusion underscores an eminent element in current definitions of poor mental health: “not having the (full) ability to cope with events and challenges” (Galderisi et al., Reference Galderisi, Heinz, Kastrup, Beezhold and Sartorius2015). The documented increase in the prevalence of mental disorders in the wake of the COVID-19 pandemic (the COVID-19 Mental Disorders Collaborators, 2021; Simon et al., Reference Simon, Schenk, Palm, Faltraco and Thome2021; Taquet et al., Reference Taquet, Luciano, Geddes and Harrison2021) is disturbing, but especially so in the light of our findings. Together, they suggest a dangerous interaction loop. Based on our data, we predict that the stress exerted by the COVID-19 pandemic (including interpersonal stress due to lockdowns, constraints imposed by school or work, and loss) (Acuff et al., Reference Acuff, Tucker and Murphy2020) will make this loop even more dangerous because of its effects on disease moderators, e.g., allostatic overload. All in all, our findings underline the importance of vaccine priority and health surveillance in people with mental disorders, in the current and possibly a next pandemic or if vaccine escape mutants arise.
Supplementary material
The supplementary material for this article can be found at https://doi.org/10.1017/S2045796023000719.
Availability of data and materials
The data presented in this manuscript will be made freely available at the OSF webpage dedicated to this project (https://osf.io/35jhm/) upon acceptance.
Acknowledgements
We thank the following authors for providing us with additional data upon request (in alphabetical order): Charles Bailey, Allen Bennett, Massimo Cavallaro, Benedicto Crespo Facorro, Olivera Djuric, Nick Francis, Lars Fritsche, Sang Joon Son, Jaehun Jung, Morgan Katz, David Kolin, Jacob Lebin, Iris Manor, Roy Perlis, Maxwell Salvatore, Maxime Taquet, Jack Tsai, Dana Tzur-Bitan, Carolina Varela, Kalliopi Vrotsou, Carly Welch, Ursula Werneke, and Chen Yanover.
We thank our colleagues at the Universities of Leiden and Navarra and Ari Maite Molendijk for all the discussions and their input on the topic. We acknowledge some assistance by Tugce Yurtsever in data collection, data extraction and methodological quality assessment.
Author contributions
MM had full access to the data and takes responsibility for the integrity of the data and the accuracy of the results presented in this manuscript.
Concept and design: all authors.
Data acquisition, quality grading, classification and analysis: PM, AR, TY and MM.
Drafting of the manuscript: MM.
Critical revision of the manuscript for important intellectual content: all authors.
Financial support
There is no funding for this project
Competing interests
PM reports to have received research grants from the Ministry of Education (Spain), the Government of Navarra (Spain), the Spanish Foundation of Psychiatry and Mental Health, and AstraZeneca; he is a clinical consultant for MedAvanteProPhase and Worldwide Clinical Trials Limited and has received lecture honoraria from or has been a consultant for AB-Biotics, Guidepoint, Janssen, Novumed, Roland Berger, and Scienta. GR declares to have received a research grant or lecture honoraria from Roche, MSD, and GSK. The other authors report not to have any potential conflict of interest.