Introduction
Influenza is a common seasonal respiratory infection with a wide range of severity, from low (asymptomatic/subclinical infection and illness not requiring medical care) to more severe (hospitalisation and death). In addition to seasonal epidemics, which can vary widely in severity from season to season, influenza viruses also have the potential to cause pandemics, such as the 2009 H1N1 pandemic [Reference Garten1], or limited animal-to-human outbreaks, such as influenza A(H3N2) variant virus infections at agricultural fairs [Reference Jhung2]. In each of these situations it may be critical to rapidly characterise the severity and potential impact of the situation to inform preparedness and response efforts [Reference Holloway3]. Sometimes, as was seen with the 2009 H1N1 pandemic, the first indication of a novel influenza virus might be increased reports of severe illness [4]; however, seasonal epidemics can also vary widely in the incidence of hospitalisations and deaths from year to year [5]. Since the overall number of hospitalisations and deaths from influenza are related to both the clinical severity of illness and the incidence in the population [Reference Reed6], distinguishing between increases in incidence and increases in severity becomes an important epidemiological activity.
An example of such a situation occurred in Arizona during the 2015–2016 influenza season. During January–March 2016, the Arizona Department of Health Services identified unusually high levels of influenza activity, influenza-associated complications and influenza-associated mortality. Reports from Arizona's Medical Electronic Disease Surveillance Intelligence System, which tracks the total number of positive influenza test results for the state, showed a greater total number of positive tests during the 2015–2016 season than for any season since the 2009 pandemic [7]. The majority of influenza viruses detected in Arizona were influenza A(H1N1)pdm09. Maricopa County had received anecdotal reports of increased severe illnesses and deaths from influenza A(H1N1)pdm09 during the same time period, including clusters of severe influenza-associated complications of cardiomyopathy and severe pneumonia associated with methicillin-resistant Staphylococcus aureus [8]. Pima County also identified an increase in patients with severe influenza-associated illness requiring extracorporeal membrane oxygenation, some of whom had influenza viruses that were not able to be subtyped at the state public health laboratory; these viruses were sent to the Centers for Disease Control and Prevention (CDC) and eventually identified as seasonal influenza A(H1N1)pdm09 viruses. This raised concerns that small genetic changes might have resulted in a poor match between the polymerase chain reaction (PCR) testing kit primers and the currently circulating H1N1 viruses.
During this same time period, early in the 2015–2016 influenza season, there were sporadic reports of severe disease associated with influenza A(H1N1)pdm09 virus infection in other jurisdictions in the USA [9]; however, the national influenza surveillance data at that time was showing that the USA as a whole was experiencing a mild to moderate season [10].
Given the discrepancy in reports of disease severity and burden in Arizona compared with the rest of the USA and concern for possible genetic changes in the viruses, state-level burden estimates were quickly needed to determine if the increase in reports of influenza associated complications and mortality were due to changes in the virus resulting in increased severity or to an overall increase in annual influenza activity in Arizona. Existing surveillance data had been used in previous settings to rapidly quantify disease burden in a similar way during the 2009 pandemic [Reference Dawood11–Reference Lipsitch13]. Using existing data sources that were potentially available in near real-time, we estimated state level influenza burden and severity for Arizona during the 2015–2016 influenza season to compare with prior recent seasons.
Methods
We used a variety of existing data sources in Arizona to calculate community influenza-like illness (ILI) rates, outpatient medically-attended ILI rates, pneumonia and influenza hospitalisation rates and pneumonia and influenza mortality rates for Arizona during the time periods of Morbidity and Mortality Weekly Report (MMWR) weeks 40–12, which corresponds to October through March of the following year, as these data were available at the start of the investigation (Table 1). We obtained data for this time period for the 2015–2016 influenza season and two recent prior seasons for comparison – 2013–2014 (an H3N2-predominant season) and 2014–2015 (an H1N1pdm09-predominant season). To estimate 95% confidence intervals, rates at each level were re-calculated using a Monte Carlo simulation with 1000 iterations, assuming the sample of observed cases from each source followed a Poisson distribution.
Table 1. Data sources
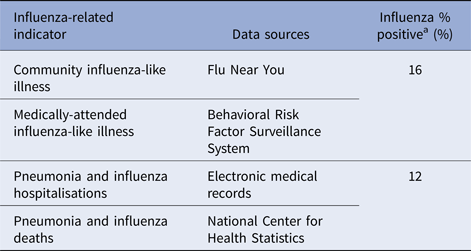
a Percent positive on testing by Sonora Quest laboratories between weeks 40–12 of the 2015–16 influenza season. Testing from outpatient clinics was used to determine the percent positivity for community and medically-attended ILI whereas testing from emergency rooms and inpatient hospital testing were used to determine the percent positivity for hospitalisations and deaths.
Community ILI
To calculate community ILI rates, we used Arizona specific data obtained from the community-based online survey ‘Flu Near You.’ Flu Near You was established in 2011 and monitors ILI among participants across the USA [Reference Smolinski14]. When registering for Flu Near You, participants provide year of birth, zip code of residence and their email address. Participants can register and report for themselves and other household members including children. Participants are then emailed weekly surveys asking if they have had any of a list of symptoms, including fever, cough and sore throat, or not. We defined ILI as fever with either cough or sore throat. There was no requirement for measured fever. Data are provided weekly at a zip-code level. To calculate the person-time in weeks contributed by each participant, we took the time from the participant's first report and censored at the date of their last report within our study period. We used the total number of ILI episodes reported in Flu Near You and divided by the calculated person-time to obtain a community ILI rate. We calculated age-specific rates and an overall age-adjusted rate for Arizona.
Medically-attended ILI
There were no direct measurements of incidence of outpatient influenza available for Arizona during the period of this investigation. However, data were available from the 2010–2011 influenza season from the Behavioral Risk Factor Surveillance System (BRFSS), a nationwide telephone survey of health-related behaviors and care seeking [15]. From 2009 to 2011, questions were added that asked participants if they had recent ILI symptoms (fever with either cough or sore throat) and, if so, whether they sought care for those symptoms [Reference Biggerstaff16]. In order to demonstrate how this data could be used to calculate outpatient disease burden if current season data was available, we pulled Arizona specific data from this BRFSS dataset. In order to estimate the rate of medically-attended ILI, we multiplied the percentage of Arizona BRFSS respondents who sought care for ILI as reported by the 2011 BRFSS survey by the community ILI rate for each season by age category. This multiplier was used across all three seasons.
Pneumonia and influenza hospitalisations
A sample of hospitals from Maricopa and Pima counties supplied electronic medical record (EMR) data on pneumonia and influenza-related hospitalisations for the three seasons based on any listed International Classification of Diseases (ICD) codes (Supplementary Appendix 1). The sample hospitals made up 17.4% of inpatient discharges for Arizona in 2015 based on the Arizona Hospital Discharge Database [17], which tracks all hospital discharges from non-federal facilities in Arizona. The total number of hospitalisations was calculated by dividing the total number of hospitalisations reported by the market share of hospitals reporting (percentage of inpatient discharges, 17.4%), assuming similar age-distribution across the state. This provided an estimated number of hospitalisations for the state of Arizona which was divided by the 2014 census population to provide a pneumonia and influenza-associated hospitalisation rate. Data provided included information about age, length of stay, intensive care unit (ICU) admission and length of ICU stay.
Pneumonia and influenza mortality
Pneumonia and influenza-associated mortality rates were calculated using Arizona death certificate data obtained from the National Center for Health Statistics (NCHS). Pneumonia and influenza mortality is reported weekly to the CDC Influenza Division through NCHS as a percentage of all deaths that are due to pneumonia and influenza [18]. We included all deaths in Arizona that listed an ICD code for pneumonia or influenza among any of the causes of death during MMWR weeks 40–12 and divided by the 2014 census population of Arizona to calculate season mortality rates for Arizona.
Severity ratios
Severity ratios were calculated as the estimated number of hospitalisations or deaths per estimated community case. State level ratios were compared across seasons. To estimate 95% confidence intervals, ratios were re-estimated using each of the 1000 iterations from the Monte Carlo simulation.
Virologic data
We obtained information on the total number and results of influenza tests performed by Sonora Quest Laboratories between MMWR weeks 40 and 12 of the 2015–2016 season. Sonora Quest Laboratories perform testing for inpatient hospitals, emergency room and outpatient clinics primarily from Maricopa County. We used the percentage positive of inpatient and emergency room testing to estimate influenza-attributable hospitalisation and mortality rates and outpatient testing to estimate influenza-attributable community and outpatient visit rates. Testing results included those of PCR, rapid antigen detection, viral culture and direct fluorescent antibody staining.
Results
Community ILI
There were a total of 963 persons from Arizona who reported to Flu Near You at least once during weeks 40–12 of the 2015–2016 influenza season. The median number of ILI reports in Flu Near You per week was 188 reports (interquartile range 167–241 reports per week). This represented a community-ILI rate during MMWR weeks 40–12 of the 2015–2016 season of 112 episodes per 100 person-seasons, compared with 65 for the 2014–2015 season and 67 for the 2013–2014 season (Table 2). Rates were highest in the youngest age group (children <5 years of age) and lowest in the oldest age group (⩾65 years) for all seasons. Each age-specific ILI rate was highest during the 2015–2016 season as compared with the prior two seasons.
Table 2. Estimated influenza-like illness (ILI) and pneumonia and influenza (P&I) rates per 100 000 person-seasonsa, by age
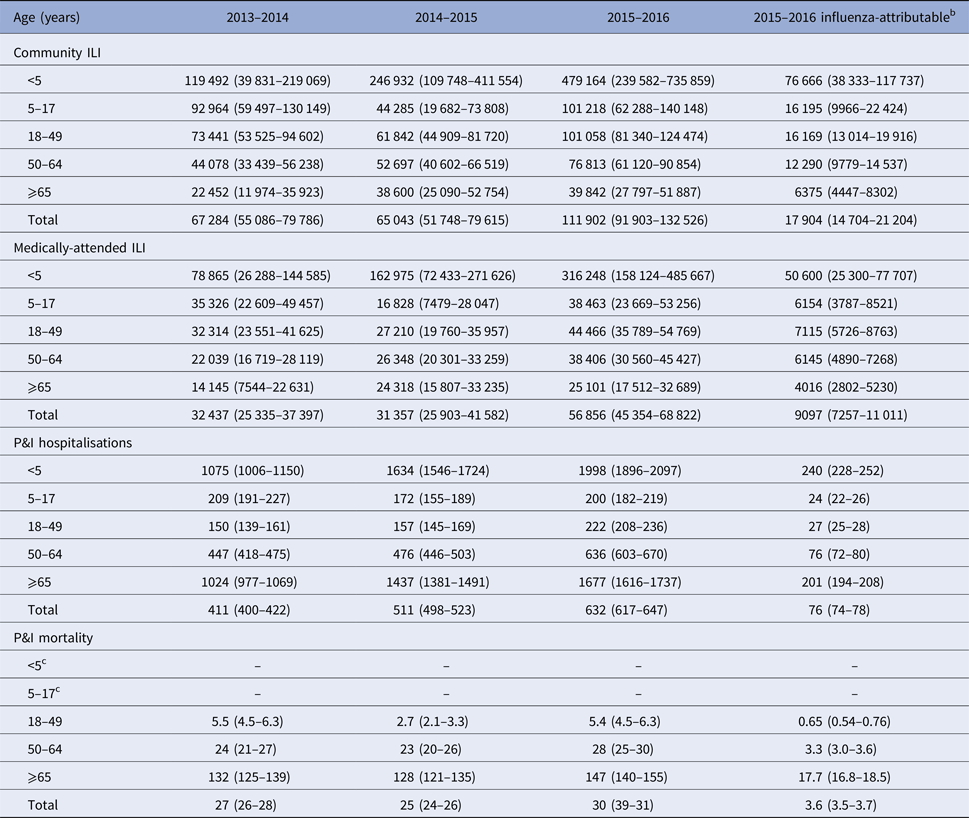
a Seasons include MMWR weeks 40–12 of the 2013–2014, 2014–2015, and 2015–2016 influenza seasons and influenza-attributable rates for MMWR weeks 40–12 of the 2015–2016 season.
b Influenza-attributable rates were calculated for 2015–2016 based off of the percentage of testing positive for influenza from either outpatient laboratories (for community and medically-attended cases) or emergency department and hospital testing (for hospitalisations and deaths). Data on percentage of testing positive for influenza was not available for the 2013–2014 or 2014–2015 seasons.
c There were too few deaths for children <5 years of age and children 5–17 years of age to be able to calculate mortality rates for these categories.
Medically-attended ILI
Care-seeking as reported among the 2011 Arizona BRFSS respondents varied by age group, with 66% of children <5 years of age seeking care, 38% of persons 5–17 years, 44% of persons 18–49 years, 50% of persons 50–64 years and 63% of persons 65 years and older. The age-weighted average, based on the 2014 Arizona census data, was 48%. This gave a medically-attended overall rate of 57 per 100 person-seasons for 2015–2016, 31 for 2014–2015 and 32 for 2013–2014 (Table 2). The ratio of medically-attended illness to community illness was 0.48 for all seasons since the same care-seeking rate was used for each season (Fig. 1).

Fig. 1. Visual representation of the burden of influenza-like illness (ILI) and pneumonia and influenza severity ratios showing the relative magnitude of medically-attended illness, hospitalisations and deaths by season. Triangle areas are proportional to the number of events. *Number labeling shows the severity ratio (ratio of deaths, hospitalisations and medically-attended cases to community cases) for each level of disease severity.
Pneumonia and influenza hospitalisations
From the sample of hospital EMR records, there were 7348 hospitalisations for pneumonia and influenza during MMWR weeks 40–12 of the 2015–2016 season, 5948 during the 2014–2015 season and 4784 during the 2013–2014 season. The sample of hospitals contributing EMR data accounted for 17.4% of all discharges in 2015 (including 14% of all paediatric discharges). Based on this, there was an estimated pneumonia and influenza rate of 632 hospitalisations per 100 000 person-seasons in Arizona during the 2015–2016 season, 511 in 2014–2015 and 411 in 2013–2014 (Table 2). Hospitalisation rates were highest for children <5 years of age and adults ⩾65 years of age for all seasons and age-specific hospitalisation rates were highest in 2015–2016 compared with prior seasons for each age group. The ratio of hospitalisation to community cases were similar across seasons (0.0057 in 2015–2016, 0.0079 in 2014–2015 and 0.0061 in 2013–2014) (Table 3, Fig. 1).
Table 3. Influenza-like illness (ILI) and pneumonia and influenza (P&I) severity ratiosa, by age
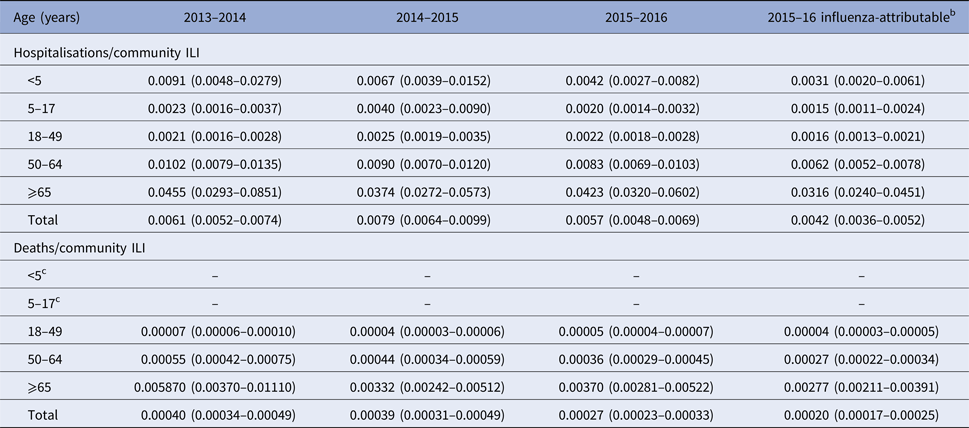
a Calculated as the ratio of either deaths or hospitalisations to community cases. Seasons include MMWR weeks 40–12 of the 2013–2014, 2014–2015, and 2015–2016 influenza seasons and influenza-attributable rates for MMWR weeks 40–12 of the 2015–2016 season.
b Influenza-attributable rates were calculated for 2015–2016 based off of the percentage of testing positive for influenza from either outpatient laboratories (for community and medically-attended cases) or emergency department and hospital testing (for hospitalisations and deaths). Data on percentage of testing positive for influenza was not available for the 2013–2014 or 2014–2015 seasons.
c There were too few deaths for children <5 years and children 5–17 years of age to be able to calculate mortality rates and therefore severity ratios for these categories.
Pneumonia and influenza mortality
There were 2001 pneumonia and influenza coded deaths in Arizona during MMWR weeks 40–12 of the 2015–2016 influenza season reported by the National Center for Health Statistics, 1682 during weeks 40–12 of the 2014–2015 season and 1798 during weeks 40–12 of the 2013–2014 season. The pneumonia and influenza mortality rate was 30 per 100 000 person-seasons for 2015–2016, 25 for 2014–2015 and 27 for 2013–2014 (Table 2). Case-fatality ratios were similar across the three seasons (0.00027 in 2015–2016, 0.00039 in 2014–2015 and 0.00040 in 2013–2014) (Table 3, Fig. 1).
Virologic data
Overall during 2015–2016, 16% of influenza tests from outpatient providers tested at Sonora Quest Laboratories between MMWR weeks 40–12 were positive for influenza. Thirteen percent of tests were positive for influenza A and 5% were positive for influenza B. Of tests performed from emergency departments or on inpatients, 12% were positive for influenza. Ten percent of inpatient and emergency department tests were positive for influenza A and 3% were positive for influenza B. These percentages were used to calculate influenza-attributable rates (Table 2) and severity ratios (Table 3). Similar trends for influenza-attributable rates and severity ratios were seen as for pneumonia and influenza rates and severity ratios.
Discussion
There was an increase in overall influenza activity in Arizona during the 2015–2016 season with higher rates observed at each severity level compared with recent prior seasons; however, severity ratios (hospitalisations and deaths per community case) were similar across the three seasons, with the lowest point estimates during the 2015–2016 season. This indicates that there was an increase in the burden of disease at all levels of disease severity, consistent with the reports of increased numbers of severe influenza cases seen in Arizona, but there was no corresponding increase in the severity of disease. Disease burden was increased among all age groups, but persons aged <50 years saw the greatest increase compared with the 2014–2015 season.
The reasons for an increase in disease burden could include changes in virologic characteristics leading to increased transmissibility or host factors leading to increased susceptibility. Two genetic subgroups of influenza A(H1N1)pdm09 virus emerged during the 2015–2016 influenza season, 6B.1 and 6B.2, with the majority of samples characterised by CDC belonging to 6B.1 [Reference Davlin19]. After further characterisation of viruses specifically from Arizona, submitted samples were found to be similar to the seasonal circulating influenza A(H1N1)pdm09 viruses in the USA, the majority belonging to 6B.1 (Personal communication, Rebecca Garten, CDC). These viruses were antigenically similar to the A/California/2009 component of the 2015–2016 vaccine [Reference Davlin19]. Significant changes in vaccination coverage in the population (percentage of the population vaccinated in the appropriate time frame) could also affect incidence of influenza. However, overall influenza vaccination coverage rates for Arizona did not decrease in 2015–2016 as compared with prior seasons, as reported by the National Immunization Survey (NIS-Flu), with (41.6% in 2014–2015 vs. 42.4% in 2015–2016) [20].
An alternative explanation might be that the disease burden seen in Arizona during the 2015–2016 season, though elevated compared with prior years, was still within the range of seasonal variation in influenza activity and severity seen in other states. Although national influenza activity during the 2015–2016 season was lower than the previous three seasons, individual states each experience the influenza season differently, not only in timing of season onset and peak activity, but also in total influenza burden. Data collected from the US Outpatient ILI Surveillance Network (ILINet) are used to produce a measure of ILI activity (from minimal to high) by jurisdiction (all 50 states, District of Columbia, New York City, Puerto Rico, US Virgin Islands) [21]. During the last six influenza seasons, the maximum number of jurisdictions experiencing high ILI activity in a single week has ranged from 4 of 54 in 2011–2012 to 45 of 54 in 2014–2015 [Reference Davlin19]. In addition, during the 2015–2016 influenza season, only 23 out of 54 jurisdictions ever reported at least 1 week of high ILI activity during the season [Reference Davlin19]. This variation in influenza activity between states during the same season is currently poorly understood, but insight by individual states into their own average influenza burden and severity could help inform preparation for seasonal influenza epidemics as well as the ability to respond quickly in pre-pandemic or outbreak situations.
There are data limitations at each level of disease severity in our analysis which could influence our severity ratios, though likely not the comparison between seasons. Flu Near You might underrepresent persons with limited access to computers or smartphones and the total number of reports for younger children is low. In addition to selection bias, there may be recall bias. Persons in Flu Near You are more likely to report when ill which affects the person-time calculations [Reference Reed22]. Care-seeking for ILI has not been asked in BRFSS since 2011, though we do not have reason to expect that that health-seeking behaviors for ILI have changed since that time. Flu Near You is now collecting this data and in future, state and local health departments may be able to utilise more current data to estimate the percentage of care seeking. Finally, neither the ILI case definition, nor pneumonia and influenza ICD codes, are specific for influenza. Though we account for this by also including an influenza-attributable rate calculated by using the percentage testing positive, the influenza-attributable rates might be an underestimate as many persons with ILI or who are hospitalised with pneumonia do not undergo influenza testing [Reference Reed6]. Also, the percentage that were laboratory positive from outpatient practices was applied to community illness and hospitalisations and the ED percentage positive was applied to pneumonia and influenza mortality. The true percentage positive may be different among levels of disease severity. Each of these limitations might affect our rate estimates; however, we expect them to affect each year similarly and therefore the severity ratios are likely still comparable between years. In addition, the overall number of paediatric deaths from pneumonia and influenza in Arizona used to calculate age-specific mortality rates for children under 18 years of age was low and thus estimates for the paediatric age groups were not estimated. Given the desire for rapid public health response, our goal was to compare general ratios between several levels of disease severity and as ratios were very similar between seasons, we did not further quantify uncertainty around our severity ratios. When synthesising data from multiple various sources, methods to estimate appropriate confidence intervals become increasingly complex. Future refinements to our approach should address this challenge so that tools will exist when needed to help interpret potential changes in severity ratios.
Public health implications
Timely state-level burden estimates, though subject to the above limitations, are possible to obtain within a matter weeks through utilisation of existing data sources. Given the geographic variation in influenza rates during ‘typical’ influenza seasonal epidemics, as well as pandemics, it is important for state or local health departments to be able to perform their own burden estimates [Reference Biggerstaff23–Reference Burkom25]. Methods similar to those detailed in this report could be used by individual states to determine state-level disease burden for typical seasonal activities, such as public health messages, evaluation of prevention campaigns and resource allocation, but also to respond during novel influenza outbreaks or early in a pandemic. The timing of data made available during our investigation varied by data source. Flu Near You data is available within 1 week as it is collected from participants directly on a weekly basis. Preliminary NCHS data is provided weekly to CDC with a 2 week delay but becomes more complete over time. State and local health departments may have earlier access to this data. Timing of the availability of EMR data varied from weeks to months depending on the facility and was likely affected by the familiarity of the hospital systems with data requests of this nature and by competing priorities. However, advanced planning and collaboration between health departments and hospitals and potentially use of systems such as BioSense [Reference Benoit26], these data could be obtained in a time-frame relevant for mid-season decision making needs. Public health agencies at all levels would benefit from determining potential data sources in advance and forming relationships with hospitals in order to rapidly assess disease burden and severity in a potential outbreak or pandemic situation.
Supplementary material
The supplementary material for this article can be found at https://doi.org/10.1017/S0950268818001516.
Acknowledgements
The authors would like to thank Dignity Health Arizona, Chandler Regional Medical Center, Mercy Gilbert Medical Center, Banner University Medicine Tucson, Tucson Medical Center, St. Joseph's Hospital, St. Mary's Hospital, Phoenix Children's Hospital (in particular Esther Munoz, Tunu Shum and Jess Perlmutter), Flu Near You, Sonora Quest Laboratories and the National Center for Health Statistics.
Human participation protection
Institutional review board was not required as only secondary, de-identified information was used for this report.
Disclosure
The findings and conclusions in this report are those of the authors and do not necessarily represent the views of the CDC.