INTRODUCTION
Campylobacteriosis, mainly caused by the zoonotic bacterial species Campylobacter jejuni and Campylobacter coli, is the most frequent form of acute bacterial gastroenteritis in humans worldwide and is therefore a major public health burden [Reference Coker1, Reference Adak2].
New Zealand had an average annual rate of notified human campylobacteriosis cases of 354/100 000 between 2002 and 2006 [3] with a peak of >380/100 000 population in 2006 [3]. This was followed by a sharp decline which has persisted to the present day. The reduction in notified campylobacteriosis cases occurred after a range of control measures to reduce Campylobacter spp. contamination in the poultry supply chain [Reference Sears4, 5] were introduced. Major objectives were described in the Poultry Processing Code of Practice and included mandatory Campylobacter performance targets for broiler chicken carcasses at the end of primary processing. The regulatory target was officially implemented on 1 April 2008 [5]. However, initiatives such as the development of a voluntary Broiler Growing Biosecurity Manual by industry (building on existing manuals and codes of practice), improvements in procedures for catching and transporting birds and monitoring/reporting prevalence of Campylobacter spp. in caecal samples took place throughout 2007 [Reference Sears4].
Campylobacter spp. are commensals in many wild, farmed and domestic animals. The risk factors for human infection range from consumption of undercooked chicken [Reference Muüllner6, Reference Kapperud7], consumption of offal (mainly chicken and sheep liver) [Reference Lake8], raw dairy products [Reference Lake8] and untreated water [Reference Adak9], to contact with pets and farmed animals [Reference Kapperud7–Reference Adak9].
Seasonal patterns in Campylobacter infections in humans have been identified in many temperate countries. Studies examining incidence rates identified a consistent peak in summer across all temperate countries [Reference Nylen10, Reference Kovats11], some countries also showed an early spring peak [Reference Nylen10, Reference Kovats11]. Kovats and colleagues [Reference Kovats11] identified the timing of the peak as being least variable from year to year in England and Wales, Greece, Denmark and The Netherlands. The pattern of seasonality was shown to be quite different in Australia and New Zealand, showing less consistency over time and a prolonged summer peak [Reference Nylen10, Reference McCarthy12]. Hearnden and colleagues [Reference Hearnden13] identified marked differences in seasonality patterns across New Zealand, in particular identifying three different groups of seasonality and incidence patterns between the North and South Islands of New Zealand. The first group, the Far North and rural areas of the North Island, showed a relatively low summer incidence and small inter-seasonal variation. The second group, comprised of urban areas (Auckland, Hamilton, Napier) in the North Island as well as some areas in the South Island, exhibited a higher summer incidence and more seasonality compared to the first group. The highest summer incidences and strongest inter-seasonal variations were found in Wellington and surrounding areas (North Island) and Christchurch, Dunedin and much of the rest of the South Island [Reference Hearnden13]. These differences between rural and urban areas could be due to the proportion of poultry- to non-poultry-associated cases in these areas, with urban areas showing a higher risk of poultry-associated infections [Reference Muellner14]. A study by Spencer and colleagues [Reference Spencer15] examined spatial and temporal determinants of campylobacteriosis notified over 7 years (2001–2007) in New Zealand. In this study [Reference Spencer15] they not only described differences in the spatial distribution between Canterbury (most seasonal variation with severe but short epidemics in the summer), Manawatu (lowest rate of notifications but increased numbers of winter cases) and Auckland (notifications appear to come in short bursts during summer); but they also found an association between social deprivation and a decreased risk of notification.
A recent study by McCarthy et al. [Reference McCarthy12] examining UK, Finnish, Australian and New Zealand datasets, sought to explain the seasonality and other aspects of C. jejuni epidemiology. The study discovered a clade formed by clonal complex (CC)45 and CC283 which showed a summer peak and is present in the UK and the Finnish dataset but not in the Australian or New Zealand dataset [Reference McCarthy12]. However, the McCarthy et al. study [Reference McCarthy12] used a small-scale dataset from New Zealand which was focused on a prolonged outbreak in the winter of one year [Reference McTavish16] instead of one of the larger published datasets [Reference Muüllner6].
In order to examine possible transmission routes of campylobacteriosis in New Zealand, a source attribution study was initiated in the Manawatu sentinel surveillance site of New Zealand in 2005 [Reference Bolwell17]. The data collected as part of the Manawatu study between 2005 and 2013 is used in this study to explore strain-type-associated seasonality. The main questions addressed in this study are: ‘Do the C. jejuni clonal complexes identified in human cases in this region show a seasonal pattern’; and ‘How do these compare with seasonality-associated clonal complexes in other countries?’
MATERIALS AND METHODS
Data
Multi-locus sequence typing (MLST) data of laboratory-confirmed cases of human campylobacteriosis were obtained from the sentinel surveillance site in the Manawatu region of New Zealand's North Island between 2005 and 2013.
Human faecal swabs were cultured on modified charcoal cefoperazone deoxycholate agar (mCCDA) plates (Fort Richard Laboratories, New Zealand) and in Bolton Campylobacter enrichment broth (Lab M, UK) and incubated under microaerobic conditions (85% N2, 5% O2, 10% CO2) generated by a MACS-VA500 microaerobic workstation (Don Whitley Scientific, UK) at 42 °C for 48 h. Single colonies resembling Campylobacter spp. were subcultured onto Columbia horse blood agar (BA) (Fort Richard Laboratories) and incubated microaerobically at 42 °C for an additional 24 h. Boiled lysate DNA preparations were made and cultures were frozen in glycerol broth at −80 °C. Campylobacter spp. isolates were identified by the method outlined in [Reference Linton, Owen and Stanley18] and further speciated using the mapA gene which has been shown to be unique in C. jejuni [Reference Stucki19]. The forward (5′-CTTGGCTTGAAATTTGCTTG-3′) and reverse (5′-GCTTGGTGCGGATTGTAAA-3′) primers were designed to target this gene and the amplification protocols were based on the methods outlined in [Reference Linton, Owen and Stanley18] and [Reference Stucki19] with slight modifications [Reference Muüllner6].
The isolates were sequenced at seven housekeeping loci (aspA, glnA, glt A, gly A, pgm, tkt, uncA) by the MLST technique outlined in [Reference Dingle20]. A total of 3309 nucleotides were sequenced per isolate. The 1215 isolates were grouped by CC and the four most prevalent CCs were used for further analysis.
Seasonal decomposition of time-series by loess (STL)
STL is an iterative procedure that decomposes time-series into overall trend, seasonal, and residual components. Both overall trends and seasonal subseries are estimated using loess local smoothing, which allows the trend and seasonality to change smoothly through time [Reference Cleveland21]. We allowed seasonality to change through time using a loess smoothing window of 7 years for the seasonal subseries. This allows the seasonal pattern to change between the early pre-intervention period and later post-intervention period. The procedure is part of the ‘stl’ package [22].
Poisson regression
In addition to the seasonal decomposition, a Poisson model with an autoregressive error term was fitted to monthly case data by CC. Seasonality was assessed via monthly factors for each CC, and an indicator variable was included to assess the effect of the poultry intervention on case numbers. For each CC (i = 1, …, 4), we model case numbers count ikt in month k at time t using

where z t = 1 if the intervention was in effect (t ⩾ 2008) and w kt = 1 if the time t is in month k.
The residuals E ikt were modelled using an AR1 process:

with correlation ρ and variance σ 2.
The Poisson model was fitted via an integrated nested Laplace approximation (INLA) using the INLA package in R [Reference Rue, Martino and Chopin23]. INLA is a Bayesian approach to statistical inference for latent Gaussian Markov random field (GMRF) models, an example of which are Poisson time-series models with autocorrelated errors. For methodological details of the INLA approach, see Rue et al. [Reference Rue, Martino and Chopin23].
RESULTS
The 1215 isolates were grouped by CC; the four most prevalent CCs were CC48 (n = 354, 29%), CC21 (n = 317, 26%), CC45 (n = 170, 14%) and CC61 (n = 65, 5%).
In New Zealand, CC48 is a poultry-associated CC [Reference Müllner24], whereas CC45 and CC21 are associated with a wider range of hosts and environmental sources [Reference Gripp25, Reference Levesque26] and CC61 is a ruminant-associated CC [Reference Manning27].
Figure 1 displays the multiple time-series plots visualizing the number of cases for each examined CC for each month over 9 years. The time of the poultry intervention is indicated by a vertical dotted line at the beginning of 2008. Comparing the pattern of the four CCs for the pre- and post-intervention period, it is noticeable that the intervention had the greatest influence on CC48 where the human campylobacteriosis incidences dropped notably (Fig. 1 a). The intervention appears to have had little effect on the number of CC21, CC45 or CC61 cases, no change in the pattern of disease notifications being apparent.

Fig. 1. Each panel displays the pattern of human campylobacteriosis cases over 9 years (2005–2013). (a) CC48, (b) CC45, (c) CC61, (d) CC21. The vertical dotted line at the beginning of 2008 indicates the defined starting point of the intervention aimed at the poultry industry.
The numbers of isolates of the four CCs were summarized over 9 years and plotted as a proportion of the number of all cases occurring in the same month over 9 years (2005–2013), are displayed in Figure 2. The summarized number of cases per month for CC45 shows a pattern of seasonality with a rising proportion of cases in the late spring to early summer (November and December, Fig. 2). For CC21 the month with the highest proportion of case notifications on average was April (autumn), but this varied considerably between years (from 0 to 1). Both plots for CC45 (Figs 1 and 2) show signs of a seasonal peak in late spring to summer (November–January) each year, whereas the pattern for the other CCs is less obvious.
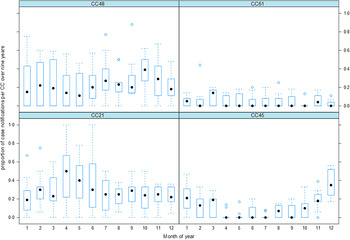
Fig. 2. The box plots show the pattern of notified human campylobacteriosis incidences divided by the four most common clonal complexes (CC48, CC61, CC21, CC45). Each box summarizes the proportions of case notifications for a given clonal complex and month between 2005 and 2013.
Seasonal decomposition
The output of STL is shown in Figure 3. It is apparent that CC61 and CC21 do not show a clear seasonal pattern or trend, as the majority of variation is in the residual. The output is different for the other two CCs where CC45 displays a clear seasonal variation, and CC48 cases describe a strong trend (related to the 2007–2008 intervention) with little seasonality.
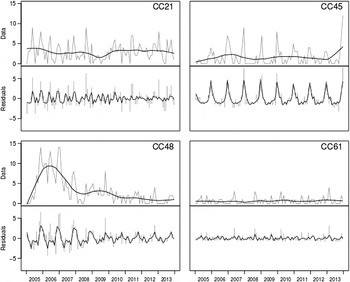
Fig. 3. Seasonal decomposition. For each clonal complex, the top plot corresponds to the data (grey) and trend (black), with the bottom showing seasonality (black) and residuals (grey).
Poisson regression model
The results for the fitted Poisson model are summarized in Table 1. The Poisson model indicates that the intervention was associated with a sharp decline in CC48 [rate ratio (RR) 0·31, 95% credible interval (CrI) 0·16–0·61]. The RR for the intervention-related CC48 drop indicates that three times fewer cases of CC48 occurred after 2008 (Table 1). This drop can also be seen in the time-series plot (Fig. 1 a).
Table 1. Results of the Poisson regression analysis estimating the effect of intervention and season for each clonal complex (CC)

Values given are rate ratio (95% credible interval).
Results that differ significantly from baseline (>1 is larger, <1 is smaller) are highlighted in bold.
There is an increase of numbers of cases associated with CC45 from October to March, with January cases having a RR of 14·3 (95% CrI 3·81–73·5) compared to July. This suggests a seasonal peak from spring to early autumn.
DISCUSSION
This study presents a 9-year time-series of genotyped human campylobacteriosis cases from the Manawatu region of New Zealand. The data were used to explore seasonal patterns in specific CCs.
Studies exploring the seasonality of C. jejuni within host animal populations or the environment in European countries such as Sweden, Finland and Iceland have reported either a strong peak in broiler flocks in August [Reference Jore28]; or no observed seasonal pattern as in the case of Poland [Reference Andrzejewska29]. There are no published studies examining the seasonality of C. jejuni within animal host populations in New Zealand. However, Lal et al. [Reference Lal30] reviewed historical data from 1973 to 2010 and calculated an overall monthly seasonality index for campylobacteriosis incidences which showed seasonal peaks in May and September for Oceania (New Zealand and Australia). Hearnden et al. [Reference Hearnden13] examined regional and temporal variations in C. jejuni notification rates and identified, apart from a marked difference in seasonality of campylobacteriosis between the North and South Islands of New Zealand, different seasonal incidences depending on the urban or rural nature of the region.
Prior to 2008, New Zealand had a particularly high and increasing incidence of human campylobacteriosis, peaking in 2006 [3]. The high numbers of campylobacteriosis cases led to recommendations for new measures to reduce the risk [Reference Baker31] and thus the New Zealand Food Safety Authority announced the implementation of the Campylobacter Risk Management Strategy.
Sears and colleagues [Reference Sears4] used source-attribution techniques, notification, hospitalization and other data to explore the 2007–2008 drop in campylobacteriosis incidence. The annual notification rate in 2008 represented a 54% decline (161·5/100 000) compared to the average annual rate from 2002 to 2006 (353·8/100 000) and the source-attribution findings demonstrated a 74% reduction in the poultry-associated cases.
We found that after the intervention the cases linked to the poultry-associated ST474 (CC48) dropped by 50% (165 cases between January 2005 and December 2007, compared to 71 cases between January 2008 and December 2013). Human campylobacteriosis cases associated with other sequence types belonging to CC48 decreased less (ST48: 45 cases pre-intervention, 31 cases post-intervention; ST38: 16 cases pre-intervention, 11 cases post-intervention). This drop can be seen in the time-series of CC48 (Fig. 1 a) and was also detected by the regression model (P < 0. 00 001). By contrast to CC48, the intervention had little effect on the other CCs. In New Zealand, CC48 is a poultry-associated CC [Reference Müllner24] whereas CC45 and CC21 are associated with a wider range of hosts and environmental sources [Reference Gripp25, Reference Levesque26, Reference Sheppard32] and CC61 is a ruminant associated CC [Reference Manning27]. This might explain why there was no corresponding decline in the other prevalent CCs following the intervention.
The Poisson regression model displayed a seasonal peak for CC45 from spring to autumn with weaker significances for spring and autumn (Table 1). The CC45 peak in summer is in concordance with the displayed seasonal decomposition for CC45 (Fig. 3), the time-series (Fig. 1) and boxplots (Fig. 2), showing a seasonal peak around December each year. The Poisson regression model also indicates significant associations for CC45 in spring and autumn. These might coincide with the findings of Lal and colleagues [Reference Lal30], which estimated a peak in May and October each year [Reference Lal30]. However, our results do not show a peak in May and show only a weak association with respect to October.
CC21 showed a relatively high degree of variability in proportions of notified human campylobacteriosis incidences over the years 2005–2013 (Fig. 2) whereas CC48 was strongly influenced by the intervention which may have obscured or eliminated existing patterns. The plot for CC21 (Fig. 2) shows an apparent peak in April; however, the interval (dashed lines) is quite large, indicating a high amount of variation over the 9 years. The highest amount of variation is over the months of April, May and June (late autumn to early winter). Precipitation has previously been shown to be associated with an increased incidence of campylobacteriosis [Reference Febriani33] and environmental surface water has been shown to carry higher numbers of Campylobacter spp. during the winter months [Reference Whiley34]. However, examination of the association between meteorological data and human C. jejuni cases was beyond the scope of this study.
MLST data have been used to examine seasonal patterns of C. jejuni and C. coli in human disease [Reference McCarthy12, Reference Cody35, Reference Sopwith36] in several countries, identifying a consistent CC45 peak in summer across all examined temperate countries. These regular, re-occurring patterns could indicate an environmental influence [Reference Febriani33] or frequent host–pathogen interactions [Reference Gilpin37]. Seasonality has previously been shown in New Zealand [Reference Nylen10, Reference Kovats11, Reference Hearnden13, Reference Spencer15] but only one study [Reference McCarthy12] has used MLST data to identify the specified genotypes involved. McCarthy et al. [Reference McCarthy12] examined the data from a 3-year longitudinal study in the UK and compared this to international datasets (Finland, Australia, New Zealand). The study identified CC45 and CC283 as being associated with a summer peak, but although they reported that the genotypes isolated in the UK are more similar to the New Zealand and the Australian samples than to the Finnish samples, McCarthy et al. [Reference McCarthy12] did not identify a marked seasonal peak in New Zealand or Australia. The present study did not identify any isolates belonging to the CC283, but the non-identification of the CC45 summer peak by McCarthy and colleagues [Reference McCarthy12] may be explained by the fact that they used a New Zealand dataset which was based on an outbreak in the Southern hemisphere winter of 2006, whereas our data spans the 9-year period 2005–2013. Nylen et al. [Reference Nylen10], who examined the seasonal distribution of campylobacteriosis across nine European countries and New Zealand, concluded that the seasonality in New Zealand was less consistent, as the week in which the peak occurred was more variable from year to year and the summer increase more prolonged. As displayed in Figure 2, the proportion of cases of CC45 over 9 years shows a distinct peak in December with a comparatively small interval varying over the 9 years, which indicates a relatively strong and consistent seasonal pattern. Possibly the inconsistent seasonality detected by Nylen and colleagues [Reference Nylen10] is related to delays between the illness, sampling, processing and official submission. Our data is subject to similar constraints, but whereas Nylen and colleagues [Reference Nylen10] reported weekly incidences, our data is summarized monthly and therefore minimizes this variation (e.g. it is not important if the incident occurred in the first or last week of the month). Several studies examining the seasonality of C. jejuni isolates from human cases identified CC45 as being strongly associated with an early summer peak [Reference McCarthy12, Reference Cody35, Reference Sopwith36] and to be frequently isolated from chickens, wild birds and environmental water [Reference Sheppard32, Reference Sopwith36, Reference French38]. These findings suggest that CC45 is adapted to survival outside the host and makes this adaptation a key driver of transmission between livestock, the environment and humans [Reference Sopwith36]. The present study showed that CC45 was not affected by the poultry industry-specific intervention, suggesting that even though it is frequently isolated from poultry [Reference Müllner24] the isolates causing the human campylobacteriosis may have been of a different origin or survived the intervention. Sopwith and colleagues [Reference Sopwith36] sampled recreational surface water and urban and rural river systems and suggested that there may be an association between CC45 isolated from water and reported campylobacteriosis incidences in humans, presenting a potential environmental transmission route for CC45. Additionally, Sopwith and colleagues [Reference Sopwith36] found that persons infected with CC45 were more likely to live in rural areas, to be aged <5 years, to have been involved in outdoor activities (e.g. fishing), to have consumed home-delivered milk, and were less likely to have eaten chicken in the 2 weeks prior to illness. CC45 has also, compared to another generalist CC21, been associated with greater resilience to oxidative and freezing stress although a poorer survival response to heat or chilling [Reference Habib, Uyttendaele and De39]. These findings support the hypothesis that isolates belonging to CC45 are more likely to infect humans through transmission routes other than food, e.g. through indirect exposure to pets or cattle or through exposure to untreated water during outdoor activities. This is further supported by the association of human CC45 with more rural areas of residence [Reference Sopwith36].
After the intervention in the poultry industry the number of poultry-associated cases has fallen, and source-attribution studies have estimated that there has been a relative increase in the importance of ruminant-associated strains [Reference Muellner14]. However, although the intervention reduced the number of poultry-associated cases, the risk has not been eliminated. New strategies need to be developed to control campylobacteriosis cases acquired from both poultry and non-poultry sources [5] and an examination of the seasonality of the CCs associated with these cases may help to focus on specific time-frames to determine the sources and transmission routes. Once source-attribution studies identify specific sources, new intervention strategies can be developed to reduce the human disease burden.
In conclusion, this study has shown that genetic analytical approaches (MLST) in epidemiological analysis can successfully identify seasonality in campylobacteriosis. CC45 was identified as a prevalent CC showing a consistent summer peak over the 9 years 2005–2013, in contrast to previous studies that found limited seasonality in New Zealand. It has also shown that the intervention had a significant effect on poultry-associated CC48.
ACKNOWLEDGEMENTS
We are grateful to all members of the Hopkirk Molecular Epidemiology Laboratory for their support and helpful discussions. The collection and typing of human isolates was performed by members of the Hopkirk Molecular Epidemiology Laboratory (Rukhshana Akhter, Julie Collins-Emerson, Errol Kwan, Sarah Moore, Petra Muellner, Lynn Rogers) and funded by the Ministry for Primary Industries. This work was funded by the Allan Wilson Centre for Molecular Ecology and Evolution.
DECLARATION OF INTEREST
None.