INTRODUCTION
Clostridium difficile is the principal cause of infectious diarrhoea in hospitalized patients [Reference Leffler and Lamont1]. The burden of disease due to C. difficile infection (CDI) has been increasing in the past decade with marked increases in severe cases and deaths related to CDI [Reference Muto2, Reference Oake3]. A systematic review investigating the economic impact of CDI found that attributable mean CDI costs per admission ranged from AUD 8911 to AUD 30 049 for hospitalized patients globally [Reference Nanwa4]. A cross-sectional study conducted in Sydney, Australia, reported that C. difficile was one of the most frequently detected pathogens in patients who visited public hospitals for gastrointestinal illnesses, and that 69% of people infected with C. difficile were aged ⩾50 years [Reference Fletcher5]. Australian national surveillance for hospital-identified CDI has demonstrated increasing incidence since 2011, highlighting a need to further characterize the epidemiology of these infections [Reference Slimings6].
Environmental contamination and frequent antibiotic use are the most important determinants of hospital-acquired CDI internationally [Reference Badger7]. In addition, advanced age is strongly associated with infection and severe clinical presentation [Reference Khanna8]. While C. difficile is generally thought of as a hospital problem, data from industrialized countries suggest that community-acquired infections are on the rise and comprise about 27–41% of all cases of CDI in such countries [Reference Khanna8, Reference Lambert9]. Patients with community-acquired CDI tend to be younger compared to those infected in the hospital setting and they often lack exposure to antibiotics [Reference Delate10], suggesting the existence of other important risk factors for infection. Recently, C. difficile has been isolated from various foods such as red meat and minimally processed fruit and vegetables [Reference Songer11, Reference Bakri12], although further studies are necessary to confirm food as an infection source.
There have not been any previous population-based cohort studies describing the epidemiology of CDI hospitalization in Australia. The aim of this study was to describe the epidemiology of hospital-identified CDI in mid-age and older Australians. Specifically, we analysed data from a large population-based longitudinal cohort to estimate the incidence of CDI hospitalization, quantify its association with potential risk factors, and calculate the median length of hospital stay and in-hospital costs per admission with CDI.
METHODS
Data sources
The Sax Institute's 45 and Up Study is a prospective cohort study of Australian adults aged ⩾45 years, randomly sampled from the general population of the Australian state of New South Wales (NSW; population 6·8 million persons, 2006) [13]. Participants were recruited by completing a postal questionnaire, distributed from 2006 to 2009. The final cohort includes ~10% of all NSW adults aged ⩾45 years. The detailed methodology is described elsewhere [14].
Questionnaire data from the 45 and Up Study participants were linked to the NSW Admitted Patient Data Collection (APDC) (to 30 June 2012), and the NSW Register of Births, Deaths and Marriages (RBDM) (to 30 June 2012). The APDC records details of all hospital separations for NSW residents admitted to hospitals. The principal diagnosis for each hospitalization, which is the main reason for hospitalization and up to 54 additional diagnoses contributing to the hospitalization, were coded using the International Classification of Diseases, 10th revision, Australian Modification (ICD-10-AM). These data also included the Australian Refined Diagnosis Related Group (DRG) code for each hospitalization. Each DRG represents a class of patients with similar clinical conditions requiring similar hospital resources [15]. Data were linked to the RBDM to ascertain fact of death for censoring purposes. The NSW Centre for Health Record Linkage performed the data linkage independent of the study investigators and report false-positive and false-negative linkages of < 0·5% and <0·1%, respectively [16].
Case definition
We defined participants as having a CDI hospitalization if they had a linked hospitalization record where the principal diagnosis field was coded for C. difficile colitis (ICD-10-AM code A04·7) following recruitment into the study. In a sensitivity analysis, we broadened the case definition to include patients where either the principal or a secondary diagnosis field was coded with C. difficile colitis.
Definition of outcomes
The study outcomes included incident hospitalization with CDI and, in those hospitalized with incident CDI, days in hospital and costs per admission (AUD). For transfer patients, the relevant admission records were first merged together. Days in hospital per hospitalization was calculated by subtracting the discharge date from the admission date, except for same day admissions where the length of stay was assigned to be a single day. To estimate C. difficile-associated hospital costs per hospitalization, we used the DRG codes of the index hospitalization due to CDI and assigned an average cost based on DRG cost data from the National Hospital Cost Data Collection Public Sector Estimated Cost Weights Reports (NHCDC) [17]. The NHCDC documents average costs per DRG, based on patient-costed and cost-modelled information. Average DRG-specific total cost per admission in Round 14 (2009–10) NHCDC was used (version 5.2 for admissions from January 2006 to December 2009 and version 6.0x from admissions from January 2010 to June 2012).
Definition of potential risk factors
Sociodemographic information was obtained from the baseline questionnaire and included: age (grouped as 45–54, 55–64, 65–74, 75–84, ⩾85years), sex, annual household income (seven categories from <AUD 20 000 to ⩾AUD 70 000 per year), and region of residence (cities, inner regional, outer regional/remote) based on the Accessibility/Remoteness Index of Australia (ARIA) [18]. Health status and health-behaviour variables included: self-rated health (excellent, very good, good, fair, poor), body mass index (BMI: <18·5, 18·5–24·9, 25–29·9, ⩾30 kg/m2), smoking (current, past, never), alcohol (0, 1–2, >2 alcohol drinks per day), proton pump inhibitor (PPI) use (yes, no), red meat intake (0, 1–7, ⩾7 times per week), and fruit and vegetable intake (low, adequate). Fruit and vegetable intake was grouped as ‘low’ if participants reported <2 servings of fruit and/or <5 servings of vegetables per day.
In addition, the Charlson comorbidity index was used to describe comorbid illness of the participants hospitalized with CDI. This index is a well-validated measure of comorbidity burden, and has been modified to produce reliable estimates using ICD-10 codes [Reference Sundararajan19]. The 19 Charlson conditions were selected and weighted according to their potential influence on mortality (scores were categorized as 0, 1, 2, 3). Baseline data from the 45 and Up Study participants were also linked to the APDC retrospectively to obtain hospitalization records before recruitment.
Statistical analysis
Participants were excluded from the analyses if they had missing data on date of entry into the study, an invalid death date or confirmed linkage errors. Participants with a discharge diagnosis of CDI within 8 weeks prior to recruitment were excluded to remove recurrent cases.
Follow-up was calculated from the date of completing the baseline survey to the first date of admission for CDI, death, or 30 June 2012, whichever came first. The crude rate of incident CDI hospitalization in the cohort, and median days in hospital and costs per admission for those hospitalized for CDI, were calculated. Rates were also reported separately by quarter and calendar year (2009, 2010, 2011, 2012), and by the various sociodemographic factors, health characteristics and behaviors.
To identify the risk factors for CDI hospitalization, Kaplan–Meier analysis with the log-rank test was first used to determine the probability of hospitalization with CDI for all potential risk factor variables. Cox proportional hazards regressions were then used to estimate hazard ratios for each of these variables with age as the underlying time variable [Reference Thiébaut and Bénichou20]. Regression models were initially adjusted for attained age (the underlying time variable) and sex. Models were then adjusted for additional variables that were associated with CDI hospitalization (log-rank test, P < 0·3), including annual household income, region of residence, health status variables (self-rated health and body mass index), PPI use and dietary variables (red meat intake and fruit and vegetable intake).
A sensitivity analysis was conducted by repeating the analysis with the case definition modified to include patients where either the principal or additional diagnosis field was coded for CDI. We tested for violation of the proportionality assumptions for the model by inspecting the log-log plots. All analyses were performed using Stata v. 12.1 (StataCorp., USA).
Ethical approval
The conduct of the 45 and Up Study was approved by the University of New South Wales Human Research Ethics Committee. Ethics approval for this study was obtained from the NSW Population and Health Services Research Ethics Committee, and the Australian National University Human Research Ethics Committee. All participants provided written informed consent.
RESULTS
After excluding participants with invalid death records (death before recruitment, n = 12), confirmed linkage errors (n = 192), and those with a CDI hospitalization in the 8 weeks prior to recruitment (n = 3), there were 266 922 participants included in the analysis, yielding 1 126 708 years of follow-up (median 3·9 years per person). The median age of study participants at recruitment was 61·1 years (range 45·0–106·2 years), and 53·6% were women. Table 1 shows a summary of the characteristics of all study participants and those hospitalized with CDI.
Table 1. Characteristics of all participants and those hospitalized with C. difficile infection, 45 and Up Study
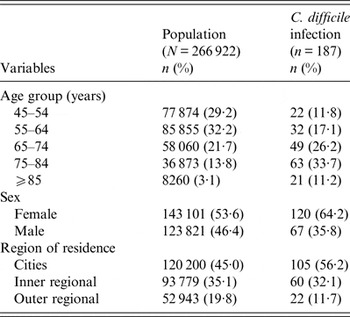
There were 187 participants with an incident CDI hospitalization, and 5·4% (10/187) died within 30 days of admission. Overall, 25·1% (47/187) of cases had a Charlson index of ⩾1, although this proportion increased with increasing age (respectively, 9·1% and 47·6% in those aged 45–54 years and ⩾85 years). We found that 37·4% (70/187) of cases had a history of hospitalization in the previous 2 weeks and 67·9% (127/187) of cases had a hospital admission in the previous 3 months.
During follow-up, the incidence of CDI hospitalization was 16·6/100 000 person-years [95% confidence interval (CI) 14·4–19·2], with a median of 6 days [interquartile range (IQR) 4–10] spent in hospital per admission, and a median hospital cost of AUD 6102 (IQR 1909–6182) per admission.
The crude incidence of CDI hospitalization increased with age as: 6·6 (95% CI 4·3–9·9), 8·7 (95% CI 6·1–12·3), 19·9 (95% CI 15·1–26·4), 41·2 (95% CI 32·9–53·9) and 69·4 (95% CI 45·3–106·4) per 100 000 person-years in those aged 45–54 years, 55–64 years, 65–74 years, 75–84 years and ⩾85 years, respectively (P <0·001). Rates also increased with year of follow-up, with a threefold increase over the study period from 10·2/100 000 person-years in 2009 to 32·0/100 000 person-years in 2012 (P < 0·001). Crude CDI hospitalization rates were higher in females (P < 0·001), in those living in cities compared to regional/remote regions (P = 0·002), in those taking PPIs (P = 0·002), and in those with poorer self-rated health (P < 0·001). Crude incidence did not differ significantly by BMI, smoking, alcohol, or food consumption.
After adjustment for age and other factors (as listed in Fig. 1), sex and self-rated health remained significant variables of CDI hospitalization. Males were 40% less likely to be hospitalized with CDI than females [adjusted hazard ratios (aHR) 0·6, 95% CI 0·4–0·7], while the aHRs increased significantly with poorer self-reported health with risks over five times greater for those with poor vs. those with excellent health (aHR 5·7, 95% CI 2·1–15·5). No statistically significant associations between other exposures and incident CDI hospitalization were observed.
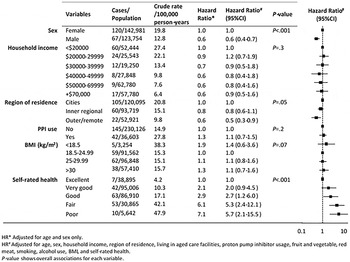
Fig. 1. Associations between baseline characteristics and incident CDI hospitalization, 45 and Up Study.
Sensitivity analysis
A total of 461 participants had a linked incident hospitalization record with a diagnosis of CDI in either the principal (n = 187) or a secondary (n = 274) diagnosis field. Compared to patients with CDI as a principal diagnosis, patients with a secondary diagnosis of CDI had higher comorbidity with 39·7% (183/461) patients having a Charlson index of ⩾1, and longer hospital stays (median of 11 days compared to 6 days).
The rate of CDI hospitalization using this alternate case definition was higher, at 39·9 (95% CI 36·5–43·8)/100 000 person-years. The rates increased significantly with calendar year from 30·7/100 000 person-years (95% CI 24·8–38·1) in 2009 to 72·0/100 000 person-years (95% CI 58·6–88·4) in 2012. We observed a similar trend of incidence in hospitalization with CDI as a principal diagnosis and hospitalization with CDI as either a principal or secondary diagnosis (Fig. 2). The quarterly incidence rates rose significantly during 2011 to 2012, with a peak in October–December 2011. The risk factor analysis yielded similar results to our main analyses, except that use of PPIs was significantly associated with CDI hospitalization (aHR 1·3, 95% CI 1·1–1·5) (Supplementary Table S1).
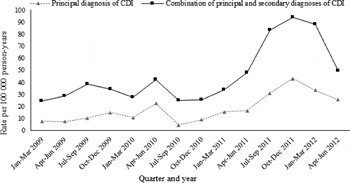
Fig. 2. Quarterly incidence of participants hospitalized with C. difficile infection in the 45 and Up Study, 2009–2012.
DISCUSSION
In this large study of middle-aged and older adults, we found a significant increase in the incidence of CDI hospitalization over 2009–2012, and an increase in CDI hospitalization with increasing age. In industrialized countries, C. difficile is one of the most frequently reported nosocomial pathogens. The elevated rates in older adults, combined with the longer duration of CDI-associated hospital stay and high hospital costs in the elderly indicate a substantial burden and excess hospital costs due to CDI in an ageing population.
Since mandatory reporting was introduced in Australia, there has been a significant increase in incidence of hospital-identified CDI [Reference Slimings6]. In our study, there is a similar trend of incidence between hospitalization with CDI as a principal diagnosis and hospitalization with CDI as either a principal or secondary diagnosis. Mandatory reporting began in 2010 while the rate increased markedly during 2011 and peaked by October–December 2011. Compared to previous years, there is a significant increase in incidence of CDI hospitalization during 2011–2012, which is unlikely to be due to changes in reporting but could be due to changes in testing practices. Changes in testing practices from enzyme immunoassay to nucleic acid amplification could result in improved detection of cases [Reference Planche21]. In addition, an Australian study using whole genome sequencing to describe the secular trends in the prevalence of hospital-identified CDI found that the introduction of new C. difficile strains, alongside rises in the incidence of established strains, may explain the observed increase in CDI [Reference Eyre22]. Although we were not able to identify the specific C. difficile strains contributing to the hospitalizations in this study, our results are in line with published Australian data [Reference Slimings6].
Notwithstanding the fact that CDI hospitalization does not exclude patients that acquire CDI during the hospitalization, nearly a third of CDI cases did not appear to be exposed to a hospital environment during the 3 months prior to their hospitalization, suggesting a community-acquired infection. Community-acquired CDI is defined as symptom onset in the community over 12 weeks after the last discharge from a healthcare facility (HCF), while HCF-acquired CDI is defined as symptom onset >48 h after admission to a HCF [Reference McDonald23]. The recommendations for surveillance of C. difficile-associated disease are to report community-onset HCF-associated CDI (defined as a patient with symptom onset in the community or ⩽48 h after admission to an HCF) in addition to HCF-onset HCF-associated CDI (defined as a patient with symptom onset >48 h after admission to an HCF) due to the delayed onset of infection in the HCF [Reference McDonald23]. In this study, we found a relatively high proportion of cases without inpatient hospital exposure in the previous 3 months. However, we were unable to assess whether these cases had contact with the healthcare environment as outpatients, although the risk of acquiring CDI in these settings has been considered low due to limited contact time [Reference Tschudin-Sutter24].
The rate of CDI hospitalization increased with age, with the highest incidence observed in persons aged ⩾85 years. This trend was in line with previous studies reporting that older people are at higher risk of experiencing severe CDI than younger people [Reference Leffler and Lamont1, Reference Khanna8]. We found that poorer self-rated health was also significantly associated with CDI hospitalization after adjustment for age, suggesting a significant impact of underlying health status. Comorbid illness and severity of underlying conditions have been reported as risk factors for CDI, partially through their association with greater healthcare contact [Reference Kyne25, Reference Ananthakrishnan26]. In addition, people with poor general health may be more likely to develop severe CDI, and therefore require hospitalization.
In our study, females were at higher risk of CDI hospitalization and the magnitude of relative risk was more extreme than published data [Reference Khanna8]. There are contradictory reports concerning sex-specific differences in CDI [Reference Foster27, Reference Fellmeth, Yarlagadda and Iyer28], although the overwhelming majority suggest a greater risk in females [Reference Wozniak, Rubin and MacIntyre29, Reference Chitnis30]. The reason for a higher risk in female observed in this study is unknown. One hypothesis relates to females being prescribed antibiotics more often than males [Reference Sun, Jew and Dasta31], and more likely to be associated with inappropriate antibiotic prescribing [Reference Barlam32]; therefore increasing risk of CDI. Sex-specific differences in the gut microbiota may also explain these findings [Reference Markle33]. Animal studies have shown that androgen levels mediate gut microbiota [Reference Yurkovetskiy34]. Sex differences in the diversity and abundance of bacterial colonization in humans’ gastrointestinal tracts may influence an individual's susceptibility to infection [Reference Mueller35]. Further research is needed to confirm the sex-specific differences in CDI and to better understand the mechanisms of this association.
We found that people living in remote or rural areas had a lower risk of CDI hospitalization and the risk decreased with increasing remoteness. This may be associated with different health services models in cities, regional/outer regional and rural areas. People living in remote areas may have less access to HCFs and therefore, are less likely to experience HCF-acquired CDI.
PPIs are associated with an increasing risk of CDI [Reference Lin36], while other studies have not confirmed this relationship [Reference Kyne25]. In our main analysis, PPI use was not associated with hospitalization with CDI as principal diagnosis (aHR 1·1, 95% CI 0·7–1·5), but was a significant risk factor for CDI hospitalization when expanding the case definition to combine principal CDI diagnosis with secondary CDI diagnoses (aHR 1·3, 95% CI 1·1–1·5). While this difference in results may in part reflect a lack of power in the main analysis, notably the combined CDI cases had longer hospital stays and more severe comorbidity than patients hospitalized with CDI as a principal diagnosis.
This is the first prospective population-based cohort study we are aware of to estimate the incidence of hospital-identified CDI and examine potential risk factors in Australia. The strengths of this study include a large sample size with linkage to hospitalization and death records, and prospectively collected data on a range of potential risk factors and confounders. The limitations include the relatively small number of cases which provide limited power to detect significant associations between certain risk factors and CDI hospitalization. The use of inpatient hospitalization data may underestimate the burden of CDI imposed on the community as cases not requiring hospitalization were not included in the analysis. Moreover, all the CDI cases were identified using coded diagnoses. The accuracy of ICD-10 codes for CDI has been assessed with 99·9% specificity and 35·6% sensitivity [Reference Jones37]. The trends in CDI rates for ICD-10 codes identified cases and laboratory-confirmed cases strongly correlated, although concordance was moderate. This may lead to an underestimate of the true population rates of CDI compared with active surveillance [Reference Jones37]. Finally, the 45 and Up Study cohort tend to be healthier and have a healthier lifestyle than the general NSW population; therefore, our results may underestimate the true incidence of CDI hospitalization in the general population. However, risk factor estimates are considered broadly generalizable from within-cohort comparisons [Reference Mealing38].
Despite concerted efforts in disease prevention, the incidence of CDI hospitalization increased significantly between 2009 and 2012 in our study. Further analysis of trends over time is needed to characterize the possible seasonality of CDI in Australia. In addition, studies are required to better understand the mechanisms underlying the increased risk of CDI hospitalization in women.
SUPPLEMENTARY MATERIAL
For supplementary material accompanying this paper visit http://dx.doi.org/10.1017/S0950268816002260.
ACKNOWLEDGEMENTS
This research was completed using data collected through the 45 and Up Study (www.saxinstitute.org.au). The 45 and Up Study is managed by the Sax Institute in collaboration with major partner Cancer Council NSW; and partners: the National Heart Foundation of Australia (NSW Division); NSW Ministry of Health; NSW Government Family & Community Services – Carers, Ageing and Disability Inclusion; and the Australian Red Cross Blood Service. We thank all the participants in the 45 and Up Study.
Y.C. received the 2014 Prime Minister's Australia Asia Postgraduate Scholarship from the Australian Government Department of Education and Training (3929_2014). B.L. is funded by an NHMRC Fellowship.
DECLARATION OF INTEREST
None.