INTRODUCTION
During outbreaks of hospital-acquired influenza (HAI) or hospital-acquired influenza-like illness (HA-ILI), morbidity and mortality increase in patients, especially those with severe underlying diseases [Reference Salgado1]. Healthcare workers (HCWs) are also at risk of influenza through extra-hospital exposure (general population) or intra-hospital exposure (patients, visitors, or colleagues); they then become, inside the hospital, possible sources of infection for other patients or colleagues. These intra-hospital transmissions impose direct and indirect costs associated with additional laboratory tests, unnecessary or inappropriate antibiotic use, disrupted hospital service due to absenteeism and increased workload on uninfected HCWs, and, possibly, ward closure [Reference Salgado1–Reference Fralick3].
HA-ILI is transmitted by contagious persons to susceptible patients. The literature reviews of hospital outbreaks [Reference Salgado1, Reference Voirin2] indicate that each HCW, patient, or visitor is a potential source of infection that may remain unidentified. Quantifying the contribution of each source of HA-ILI transmission through statistical modelling should help to understand the intra-hospital spread of HA-ILI and implementation of preventive and control measures during seasonal epidemics or pandemics [Reference Thompson, Comanor and Shay4].
However, for valid statistical inference, some features of communicable diseases should be considered; e.g. the natural history and the occurrence of dependent events [Reference Nishiura, Kakehashi, Inaba, Chowell, Hyman, Bettencourt and Castillo-Chavez5]. First, in case of HA-ILI, transmission is likely to have occurred through exposure during the previous days. It would therefore be useful to identify: (i) the most probable source of infection (patient, HCW, visitor) in order to choose appropriate interventions; and (ii) the delay between exposure and HA-ILI in order to time the interventions. Second, after infection by ILI viruses, an infected person may become contagious well before the onset of the first symptoms (unobservable contagious period) and remain contagious during the course of the disease (observable contagious period). It is therefore important to know the extent to which exposure during the unobservable contagious period contributes to HA-ILI transmission in order to determine the duration of the interventions.
A statistical model was previously developed to integrate these features in order to assess the risk of HA-ILI associated with multiple exposures [Reference Payet6]. Here, data collected prospectively during three influenza outbreaks [Reference Vanhems7] are used to study the influence of observable exposure to contagious patients and HCWs and of unobservable exposure to other sources on the patient's risk of HA-ILI, and to quantify the contribution of each source of transmission.
METHODS
Data sources
The data came from a prospective observational study performed during three influenza outbreaks between 15 November 2004 and 15 April 2007 at Edouard Herriot Hospital, Lyon, France [Reference Vanhems7].
Briefly, each participating ward was followed-up daily to detect ILI cases. A case was defined as an adult patient or HCW presenting with fever (⩾37·8 °C) and cough or sore throat. The data collected on each patient hospitalized in the participating wards included admission and discharge dates, the date of symptom onset, and data on HCW work periods. Overall, the data concerned 64 incident ILI cases among 21 417 patients at risk of ILI with a median length of stay of 9 days (range 2–77 days) [Reference Vanhems7]. The French Institut de Veille Sanitaire provided data on the incidence of ILI in the general population [Reference Costagliola8].
Statistical analysis
The present analysis developed a previous statistical model [Reference Payet6] built to link the patient's risk of communicable disease (with multiple exposures) to recorded and unrecorded sources of transmission in healthcare settings. However, this model did not consider the role of the source; i.e. patient or HCW. Here, the new model assesses the risk of HA-ILI conditionally on exposure to recorded contagious patients or HCWs or to unrecorded symptomatic or asymptomatic patients, HCWs, or visitors.
Unless serious effort is put into microbiological testing, only the onset of HA-ILI symptoms can be directly observed during an outbreak; exposures that lead to infection and the start of contagiousness cannot be easily observed. However, in infectious disease epidemiology this is an important feature that should be incorporated in the modelling process. We therefore needed to translate observable information gathered during investigation into unobservable information that is essential for modelling transmission [Reference Nishiura, Kakehashi, Inaba, Chowell, Hyman, Bettencourt and Castillo-Chavez5]. In the specific case of influenza, the contagious period starts 1 day before symptom onset and lasts for a maximum of 6 days [Reference Carrat9]. The unobservable contagiousness period was thus defined as the day before symptom onset and the observable contagiousness period as a maximum of 5 days following symptom onset. Moreover, considering that the maximum length of incubation period is 5 days [Reference Lessler10], it was assumed that exposure to a contagious source within a given ward during the 5 days preceding a given time point t was compatible with potential transmission. This allowed us to distinguish, for each susceptible patient at a given time t, the exposures that occurred the day before from those that occurred 2–5 days before. We therefore relaxed the assumption that the risk of infection is uniform over the contagious period and the moments of exposure.
Consequently, the following effects can be separately estimated: (1) exposure to recorded vs. unrecorded sources; (2) exposure to contagious patients vs. contagious HCWs; (3) exposure 1 day vs. 2–5 days before time t; and, (4) exposure during observable vs. unobservable contagious periods of the recorded sources.
The analysis considered that the infected patients could not become susceptible anew; therefore, the follow-up was censored at the day of HA-ILI onset for cases and the day of discharge for HA-ILI-free persons.
The risk of HA-ILI was modelled at the patient level and for each half-day of hospitalization. The half-day time unit was convenient because: (i) at our hospital, the patients are admitted in the afternoon and discharged in the morning whereas the HCWs work either in the morning or the afternoon, and, (ii) the risk and the exposure appear to be more constant with the day time unit.
Bayesian inference was used to estimate the parameters and the relative contributions of the various exposures [Reference Gelman11]. Markov Chain Monte Carlo (MCMC) methods run with OpenBUGS and the coda package in R [12] provided the means and 95% credible intervals (95% CrIs) from the posterior distributions of the parameters. The attributability of HA-ILI to the different types of exposure was also calculated. The details of the model and of these calculations are provided in the Supplementary material.
Sensitivity analyses were performed to study how parameter estimates varied according to various assumptions about the contagious and incubation periods. For this, we varied the maximum length of the contagious period from 5 days to 4 days, and the maximum of the incubation period from 5 days to 2 days. We present this sensitivity analysis in the Supplementary material.
RESULTS
Risk of HA-ILI associated with exposure to recorded and unrecorded sources
Table 1 shows that the patients' risk of HA-ILI was 78·9/10 000 patient-days (95% CrI 42·8–131·1) in case of exposure to recorded contagious patients and 57·0 (95% CrI 18·3–112·7) in case of exposure to recorded contagious HCWs. In case of exposure to an unrecorded source of infection, the risk of HA-ILI for the patients was fivefold higher during the epidemic than during the sporadic period of the disease (4·2 vs. 0·8/10 000 patient days). The attributability of HA-ILI to any recorded source was 97% (95% CrI 94–98) during the epidemic period and 99% (95% CrI 99–100) during the sporadic period.
Table 1. Risk of hospital-acquired influenza-like illness (HA-ILI) associated with exposure to recorded and unrecorded sources
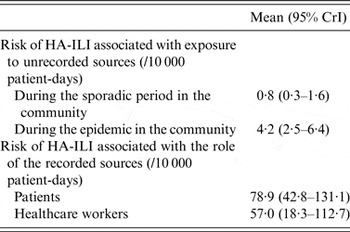
CrI, Credible interval.
Attributability of HA-ILI to the role of the source
Among recorded sources, the study estimated that 59% (95% CrI 34–83) of all HA-ILIs could be attributed to exposure to contagious patients and 41% (95% CrI 17–66) to exposure to contagious HCWs (Fig. 1). Details of the calculations are provided in the Supplementary material.
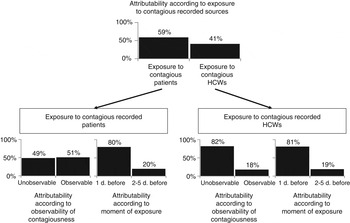
Fig. 1. Attributability of HA-ILI among patients according to various recorded sources of exposure.
Attributability of HA-ILI to observability of contagiousness and the moment of exposure
Figure 1 details attributability results. Table 2 shows that exposure to contagious patients the day before a given time t was more likely to be compatible with transmission than exposure 2–5 days before [80% (95% CrI 59–96) vs. 20% (95% CrI 4–41)]. In addition, the risks of HA-ILI were similar during the observable and unobservable contagiousness period of the recorded source [49% (95% CrI 19–75) vs. 51% (95% CrI 25–81)].
Table 2. Analysis of the attributability of hospital-acquired influenza-like illness (HA-ILI) according to observability of contagiousness and to the moment of exposure
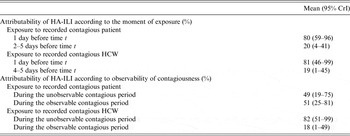
CrI, Credible interval.
Exposure to contagious HCWs the day before a given time t were more likely to be compatible with transmission than exposure occurring 2–5 days before [81% (95% CrI 46–99) vs. 19% (95% CrI 1–45)]. In case of exposure to contagious HCWs, the risk of HA-ILI was much greater during the unobservable than during the observable contagiousness period [82% (95% CrI 51–99) vs. 18% (95% CrI 1–49)].
Sensitivity analyses
Sensitivity analyses are presented in the Supplementary material and show that similar estimates and 95% CrIs were obtained for each parameter.
DISCUSSION
The present work investigated the changes in the risk of HA-ILI transmission to hospitalized patients according to the exposure to recorded contagious patients or HCWs or unrecorded sources.
The study yielded two important findings. First, about 81% of HA-ILIs were associated with exposure 1 day earlier. This short incubation period of ILI is in agreement with a recent discussion on the definition of HAI [Reference Vanhems, Landelle and Benet13], underlines the explosive nature of HA-ILI spread, and suggests that secondary cases might appear as soon as 24 h after a primary case. Second, 49% and 82% of HA-ILIs can be attributed to not yet symptomatic or asymptomatic contagious patients and HCWs, respectively. This highlights that unobservable transmissions may precede HA-ILI outbreaks and suggests that symptom-based control measures alone might fail to halt HA-ILI and influenza transmission in the acute-care setting.
Previous reports on HAI outbreaks [Reference Salgado1, Reference Voirin2] have indicated that HCWs, patients, and visitors are all potential sources of infection and that, sometimes, no source of infection could be identified suggesting transmission from asymptomatic individuals. The present results are in agreement with these reports and show that unrecorded sources may account for 1–3% of all HA-ILIs.
In the present study, the unrecorded sources could include symptomatic patients or HCWs missed by survey, asymptomatic contagious carriers, and contagious visitors (when not under study). However, it seems unlikely that symptomatic patients or HCWs could have been missed here because the ILI definition had a high sensitivity and because of the active surveillance [Reference Monto14]. The unobserved transmission may be explained by the presence of asymptomatic contagious individuals. Indeed, the proportion of asymptomatic influenza carriers has been estimated at 30% even if their infectiousness is believed to be reduced. Moreover, the effect of unrecorded exposure seemed to increase fivefold during the epidemic vs. the sporadic period. This increase may be due to an increase of the number of contagious visitors during the epidemic period. This result is in favour of exposure-limiting measures during the epidemic or pandemic period in order to avoid unwanted introduction of influenza to hospitals.
In the present study, the majority of HA-ILIs were attributable to symptomatic contagious patients and HCWs because 97% and 99% of HA-ILIs were associated with recorded exposure during the epidemic and sporadic period, respectively. Interestingly, the distribution of the risk indicates that about half of HA-ILI cases were associated with exposure to contagious patients. Two hypotheses might explain this result. First, while direct patient-to-patient transmission cannot be ruled out, especially in wards where contact between patients is frequent, patient-to-patient transmission could also occur indirectly through HCWs acting as vectors [Reference Grundmann and Hellriegel15, Reference Temime16]. Second, airborne transmission from patient to patient in confined settings might be as important as direct transmission through close contact [Reference Bischoff17, Reference Brankston18].
On the basis of the present results, a number of prevention and control measures may be discussed. In France, the Institut de Veille Sanitaire declare the start of the influenza epidemic annually [Reference Costagliola8]. Hospitals should then watch the progress of the epidemic in the general population and start active surveillance when ILI incidence exceeds the epidemic threshold. This helps in avoiding unnecessary introduction of influenza to hospitals and in preparing for increase of admissions of potentially influenza-infected patients. Given the short period between exposure and HA-ILI and given the possibility of transmission during the unobservable contagiousness period, isolation of symptomatic patients or contacts and contact tracing should be the first measures taken to prevent secondary cases.
In practice, control of airborne transmission is difficult; however, control of indirect transmission via HCWs can be easily achieved through enhanced promotion of hand washing, use of face-masks and vaccination. The prophylactic antiviral chemotherapy of patients exposed to contagious persons and the treatment of infected persons could reduce transmission during the observable and unobservable contagious periods. However, this action may be limited by the small number of antiviral drugs available, the emergence of resistance and the possible side-effects. Visiting restrictions and ward closures can also help reduce the impact of the outside epidemic on HA-ILI transmission. Moreover, interventions, particularly non-pharmaceutical interventions, aiming at controlling transmission should be started as soon as the first case is suspected because even short delays before implementation of interventions may increase HA-ILI and influenza spread through unobserved transmission.
The approach presented here has some limitations. First, the definition of exposure ignored actual contact between persons. In practice, it is difficult to follow each person to find out with whom, when, and for how long he/she came into contact with another person. Second, the definition of the contagious period we used ignored individual variation; although we performed sensitivity analyses, it would be interesting to integrate this variation into a future improved model. Third, the study did not consider a follow-up of potential patients to collect the occurrence of HA-ILI after hospital discharge; thus, transmission might well have been underestimated. Finally, the analysis was about HA-ILI; its transposition to influenza remains debatable despite a strong correlation between the incidence of ILI and the epidemic activity of influenza. Here, only 13 (20%) out of 64 patients with HA-ILI had laboratory-confirmed influenza.
In conclusion, the present results indicate that the majority of HA-ILIs in patients was equally attributable to exposure to contagious patients and contagious HCWs. The very short period between exposure and HA-ILI and the large transmission during the unobservable contagiousness period are strong indicators of the explosive nature of HA-ILI and influenza at hospital. The above-mentioned, well-known prevention and control measures should be discussed in continuing education and reminders given to patients, HCWs, and visitors, especially during seasonal and pandemic influenza.
SUPPLEMENTARY MATERIAL
For supplementary material accompanying this paper visit http://dx.doi.org/10.1017/S0950268816000145.
ACKNOWLEDGEMENTS
The authors express their gratitude to Jean Iwaz (Hospices Civils de Lyon) for the thorough revisions of the latest versions of the manuscript. We also thank the two referees for their very interesting comments that have allowed us to clarify and strengthen our paper.
This work was partly supported by FINOVI Foundation (Lyon), a Programme Hospitalier de Recherche Clinique (PHRC).
DECLARATION OF INTEREST
None.