Introduction
Throughout military history, acute gastrointestinal illness (AGI) has been a significant cause of morbidity and mortality among US service members [1]. Despite advances in medicine and improvements in basic sanitation, modern day military operations still are affected by gastrointestinal illness. In 2012, diarrhoeal diseases were responsible for more than 17 000 healthcare encounters affecting over 15 000 US service members [2]. An outbreak of AGI caused by Shiga toxin-producing Escherichia coli, a major cause of foodborne illness, sickened 244 male recruits at the Marine Corps Recruit Depot, 15 of which had life threatening complications [Reference Keaton3]. Symptoms of AGI include diarrhoea, vomiting, fever, malaise and/or weakness. Not only can AGI affect individual medical readiness, if a large proportion of the military population is affected by AGI, military operational effectiveness can be degraded [Reference Sanders4].
One important preventable cause of AGI is foodborne illness. The WHO estimates that as much as 70% of diarrhoeal diseases worldwide can be attributed to foodborne pathogens [Reference Adams and Motarjemi5]. Foodborne infections are an important cause of illness in the USA [Reference Mead6], with more than 48 million Americans becoming ill from contaminated foods annually [Reference Scallan7]. Members of the US Army are also are at risk for foodborne illness. The US Army is a unique population that is globally distributed, has its own food procurement system and a food protection system dedicated to the prevention of both unintentional and intentional contamination of food. To our knowledge, the burden of foodborne illness caused by specific pathogens among the nondeployed active duty US Army military population has not been determined. Foodborne illness burden measures are necessary for directing policy and interventions aimed at reducing the incidence of foodborne disease.
Estimating the number of foodborne illnesses caused by specific pathogens among US Army service members can be very challenging for a number of reasons. One challenge is that food can be simultaneously contaminated by a combination of agents that can cause illness including viruses, bacteria, parasites and chemicals [Reference Scallan7]. Transmission of these agents can occur through nonfood routes such as consumption of contaminated water or contact with infected animals [Reference Scallan7]. The number of infections transmitted by food depends on the level of contamination in the food, the environment in which the food is prepared, the pathogen itself and certain host factors such as immune status and age [Reference Scallan7]. Finally, we generally rely on laboratory surveillance to detect cases of foodborne illness, which results in many cases going undetected [Reference Roy, Scallan and Beach8]. Additionally, for the US Army, these issues are compounded by the fact that the US Army does not have a dedicated foodborne illness-specific surveillance system in place.
In the US Army, foodborne diseases are detected through the medical event reporting system (Disease Reporting System internet, DRSi) and only 17 of the 31 major causes of foodborne illness are included as reportable medical events [Reference Scallan7, 9]. This system relies on laboratory confirmation of illness aetiology and is not an accurate reflection of the true burden of foodborne disease. For a reportable medical event to be documented, the ill service member must seek medical care and submit a stool specimen, the laboratory must isolate and identify the organism from the sample and positive results must be entered into DRSi (Fig. 1). If any one of these events does not occur, the illness is not recorded. To gain a more accurate estimate of the number of annual foodborne illnesses among US Army service members, we need to estimate the number of cases of disease that go unrecognised at each surveillance step. Scallan et al. calculated estimates of foodborne illness in the US through the use of telephone surveys of the population, laboratory surveys, FoodNet surveillance data and data from outbreak investigations [Reference Scallan7]. Our current study uses similar methods to create pathogen-specific underreporting and underdiagnosis multipliers to estimate the true burden of disease caused by five major pathogens. Ultimately, the results of this study will be used to make recommendations for a Department of Defence (DOD)-wide foodborne illness surveillance system, to identify strategies for foodborne illness intervention and to modernise the current US Army food protection program.

Fig. 1. Burden of Illness pyramid illustrating the steps that must occur for an episode of illness in the active duty US army population to be reported through laboratory surveillance.
Methods
In 2011, the Centers for Disease Control and Prevention provided estimates of foodborne illnesses in the USA caused by 31 known major pathogens and unspecified agents [Reference Scallan7, Reference Scallan10]. We used a similar approach to estimate the annual number of foodborne illnesses among nondeployed active duty US Army service members for five major pathogens: Campylobacter, Salmonella, Shigella, non-O157 shiga-toxin-producing E. coli (STEC) and Norovirus. We used two different model structures depending on the pathogen. For all bacterial pathogens, we used models that began with the laboratory-confirmed cases counts and then scaled them up through the use of a series of underreporting and underdiagnosis multipliers (model type 1). For Norovirus, the model began with the total 2014 nondeployed active duty US Army population and used AGI incidence data to scale the population down to the estimated annual number of noroviral illnesses (model type 2).
For model type 1 we used a number of inputs, each with a measure of uncertainty. These inputs were derived from data obtained through surveys of the nondeployed active duty US Army population [Reference Mullaney11], US Army clinical laboratories [Reference Mullaney11] and data from FoodNet and Scallan et al. [Reference Scallan7]. We chose program evaluation and review technique (PERT) distributions for the majority of the model inputs. The PERT distribution is used for modelling expert estimates using the expert's minimum, most likely and maximum estimates [Reference Hauwemeiren, Vose and Bossche12]. Similar to Scallan et al., we chose this distribution because it works well when you have many estimates and sources of uncertainty that need to be combined into one model [Reference Scallan7]. The general structure for model type 1 is shown in Figure 2, followed by a general description of how each input was ascertained. Tables 1–4 display detailed model input data descriptions for each of the bacterial pathogens.

Fig. 2. Basic model structure for model type 1.
Table 1. Model inputs, data source, distribution and distribution values for Campylobacter

Table 2. Model inputs, data source, distribution and distribution values for Salmonella enterica non-typhoidal serotypes

Table 3. Model inputs, data source, distribution and distribution values for Shigella spp

Table 4. Model inputs, data source, distribution, and distribution values for Shiga-toxin producing Escherichia coli, non-O157
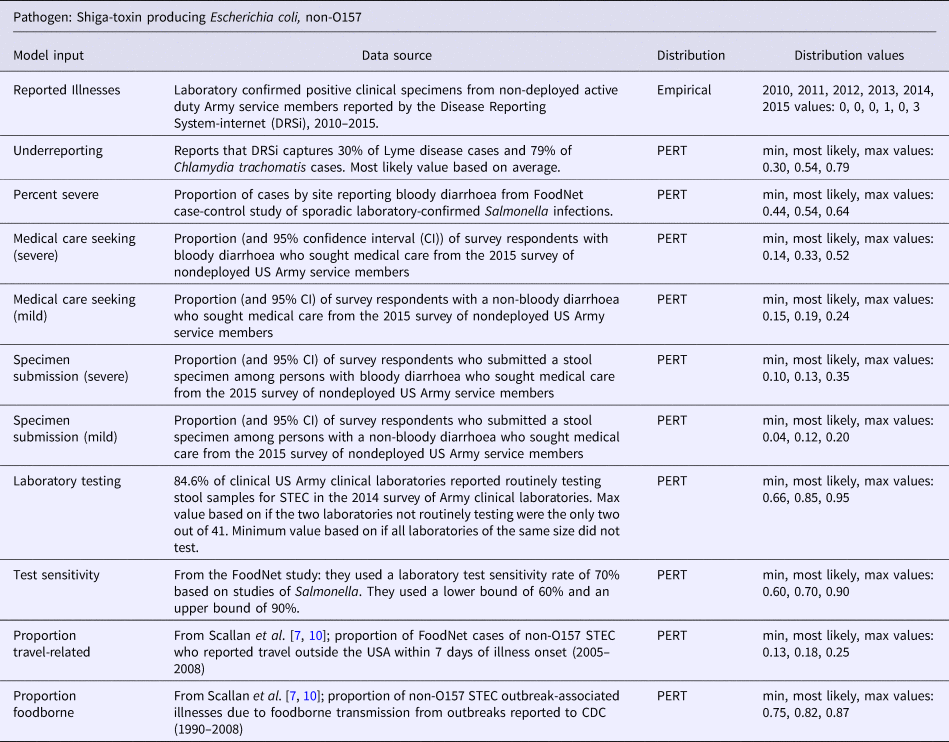
DRSi case count
Laboratory-confirmed case counts were ascertained from DRSi. The Army Public Health Center Epidemiology Service provided DRSi case counts of Salmonella, Campylobacter, STEC and Shigella from 2010 to 2015 (Z. McCormic, S. Gosine, email, 29 July 2014). All non-active duty US Army cases and all deployed cases were excluded. All cases were culture-confirmed positive. The STEC cases were not identified specifically as STEC O157:H7, so it was assumed that they were all non-O157:H7 cases. Histograms were constructed for each of the four bacterial pathogens for entry into the model. A non-parametric distribution was used because of the flexibility associated with these types of distributions [Reference Hauwemeiren, Vose and Bossche12]. The data did not meet the assumptions of parametric count distributions, such as the Poisson distribution [Reference Scallan7, Reference Hauwemeiren, Vose and Bossche12]. In particular, the annual case counts represented single count samples from distinct annual populations with different characteristics (not identically distributed) [Reference Scallan7].
Underreporting multiplier
In a study by Jordan et al. they found that DRSi case capture for Chlamydia trachomatis was 79% [Reference Jordan13]. A study by Evans et al. found that DRSi captured only 30% of Lyme disease cases [Reference Evans14]. Underreporting for the four bacterial pathogens of interest in this study likely falls somewhere between these two numbers and a PERT distribution was constructed accordingly. The same underreporting PERT distribution was used for all four bacterial pathogens. Detailed information for the underreporting model inputs are displayed in Tables 1–4.
Underdiagnosis multiplier
The underdiagnosis multiplier is made up of eight different model inputs (Fig. 2). PERT distributions were constructed for each of the eight inputs using the minimum, most likely and maximum values.
Proportion severe illness and proportion non-severe illness
The data for proportion severe illness and non-severe illness were obtained from Scallan et al., Technical Appendix 3 [Reference Scallan7]. Depending on the pathogen, these data were based on FoodNet case-control studies or FoodNet surveillance data. Detailed information and model inputs for each pathogen are displayed in Tables 1–4.
Care seeking and stool specimen submission
To adjust for medical care seeking and specimen submission, results from the 2015 survey of nondeployed active duty US Army service members were used [Reference Mullaney11]. The proportion of respondents who reported acute diarrhoeal illness in the last 30 days and sought medical care and submitted a stool sample were calculated. People with more severe illness are more likely to seek care and bloody diarrhoea is an indicator of severe disease [Reference Scallan15]. Therefore, medical care seeking and stool sample submission for bloody and non-bloody diarrhoea as surrogates for medical care-seeking and stool sample submission for severe and mild cases of illness were used. These four inputs scale up mild and severe illness care-seekers to all mild and severe illnesses in the population and scale up submitted samples from mild and severe illness care-seekers to all ill medical visits [Reference Scallan7]. Detailed information and model inputs for each pathogen are displayed in Tables 1–4.
Laboratory testing
The number of laboratories routinely testing for each of the four bacterial pathogens varied [Reference Mullaney11]. PERT distributions for each of the pathogens based on the 2014 survey of US Army clinical laboratories were constructed [Reference Mullaney11]. This factor scales tests performed up to samples submitted [Reference Scallan7]. Detailed information and model inputs for each pathogen are displayed in Tables 1–4.
Laboratory sensitivity
Laboratory specimen handling and practices across the surveyed Army laboratories met most of the recommended guidelines [Reference Mullaney11]. There were some practices that could result in decreased sensitivity, though quantification of the impact these variations in specimen handling and transport had on the number of positive samples was unable to be performed. The findings were similar to the 2004 survey of FoodNet laboratories, so the Scallan et al. data found in Technical Appendix 3 were used to construct the PERT distributions for this model input [Reference Scallan7, Reference Voetsch16]. The data are based on studies of the laboratory test sensitivity rate of Salmonella. This model input scales up positive tests to true positive specimens [Reference Scallan7]. Detailed information and model inputs for each pathogen are displayed in Tables 1–4.
Percent domestically acquired
This model input is a contractive factor to scale down case counts to those cases that are domestically acquired, excluding those cases acquired while traveling overseas [Reference Scallan7]. The data for this model input was obtained from Scallan et al., Technical Appendix 3 and is based on FoodNet studies that looked at the number of infected individuals who reported travel outside of the US within 7 days of illness to determine the number acquired during travel [Reference Scallan7]. Those who reported no travel were considered to have domestically acquired foodborne illness. This data were not available for our population, the assumption was made that our population is similar. Detailed information and model inputs for each pathogen are displayed in Tables 1–4.
Percent foodborne
This factor scales down overall illness counts to illness counts that are foodborne [Reference Scallan7]. The data for this model input were obtained from Scallan et al. Technical Appendix 3, based on FoodNet case-control studies, outbreak data and surveillance data, as outlined for each pathogen in Tables 1–4 [Reference Scallan7].
Figure 3 illustrates the model structure for Norovirus. The annual incidence of AGI among nondeployed US Army service members was estimated previously [Reference Mullaney11]. The data showed variation in incidence among geographical US Army medical regions. Estimates of the region-level incidence for each of the five different regions were calculated. Using ModelRisk 5 (VOSE Software), normal distributions of AGI incidence from each site were overlaid using the point estimate and standard error as inputs to the distribution. The distributions were averaged for entry into the model as the annual incidence of AGI. The remaining model inputs and data sources are described in detail in Table 5.

Fig. 3. Basic model structure for Norovirus.
Table 5. Model inputs, data source, distribution and distribution values for Norovirus

For both model types 1 and 2, once all model input distributions were constructed, Monte Carlo simulation was performed using ModelRisk 5 (Vose Software, 2013, Ghent, Belgium) with 100 000 iterations for each estimation. The results of each simulation were reported as a mean and range between the 5th and 95th percentile. Sensitivity analysis investigating the uncertainty introduced by each model input was conducted. All statistical analysis was performed using ModelRisk 5 (Vose Software, 2013, Ghent, Belgium).
Results
Distribution inputs and model outputs for each pathogen are displayed in Supplementary Figures S1–S9. Estimated annual number of episodes of domestically acquired foodborne illness among nondeployed active duty Army service members caused by Campylobacter jejuni, Shigella spp., Salmonella enterica non-typhoidal, STEC non-O157 and Norovirus are presented in Table 6. Due to differences in care-seeking and stool sample submission behaviours among nondeployed active duty Army service members when compared with the general US population, our under-diagnosis multipliers were much higher for the four bacterial pathogens than in the Scallan et al. study [Reference Scallan7]. Estimates are that these five major pathogens caused 158 500 (5%–95% range: 105 600–220 300) illnesses, of which 156 200 (5%–95% range: 103 600–217 800) were domestically acquired and 45 600 (5%–95% range: 30 300–64 200) were foodborne. Out of these pathogens, Norovirus (38 900, 85%) and Campylobacter (3650, 8%) caused the most illness in this population.
Table 6. Estimated annual number of episodes of domestically acquired foodborne illnesses caused by five major pathogens among nondeployed active duty US Army service members

For the sensitivity analysis, we calculated the variance and coefficient of variance for each of the model inputs to assess how much uncertainty each input added to the model. Overall, the under-diagnosis multiplier as a whole contributed the most uncertainty to the models and this was due to three model inputs: sample submission (non-severe), proportion severe illness and care-seeking (severe).
Discussion
To our knowledge, this is the first time the burden of foodborne illness caused by specific bacterial and viral pathogens has been estimated in the nondeployed active duty US Army population. Our study shows that underdiagnosis multipliers are higher in this population than in the general US population. The sensitivity analysis revealed three inputs to the underdiagnosis multipliers are contributing the most uncertainty in the models. These data come from a population-based survey of the US Army population and the uncertainty is introduced due to the small number of respondents with non-severe AGI who submitted a sample, the small number of respondents who reported severe illness and the small number of respondents with severe illness who sought medical care [Reference Mullaney11]. As previously described, repeating the survey, possibly through face-to-face administration, or through a higher level authority could improve response rate and improve the robustness of the data. This would help to reduce uncertainty in the model and improve model estimates. In addition, DRSi data are collected passively, so underreporting multipliers were required for the four bacterial pathogens of interest. This should be considered in future burden of illness calculations for the US Army population. Similar to other studies, of the five pathogens assessed, Norovirus was the leading cause of foodborne illness in our population [Reference Hall17],. In the present study, of the four bacterial pathogens, Campylobacter and Salmonella caused the most illnesses. This finding is similar to studies in England, Wales, Australia and the USA [Reference Scallan7, Reference Hall17, Reference Adak18]. The estimated number of illnesses caused by these five major pathogens is alarming. Overall, these five pathogens cause an estimated 8600 illness per 100 000 population (range: 5800–12 200 per 100 000). The illnesses caused by these pathogens can vary in duration, severity and post-infection complications (Table 7) and can impact mission readiness if numerous individuals in a unit are affected, especially in outbreak situations.
Table 7. List of pathogens, incubation period, length of illness, clinical symptoms and possible complications

Limitations
The DRSi database system only captures individuals seeking care at military medical treatment facilities. If an ill service member sought care at a civilian location, DRSi will not capture the case. It is possible that cases of illness caused by the four bacterial pathogens were missed for this reason, resulting in lower burden estimates, which were not accounted for in the models. The data for this study came from a number of sources, including our own surveys and from FoodNet surveillance and outbreak data. Limitations of our population and laboratory surveys are discussed elsewhere [Reference Mullaney11]. Limitations of the FoodNet data are discussed in the 2011 Scallan et al. burden of illness study [Reference Scallan7]. Using the FoodNet data for the US Army population may have resulted in inaccurate estimates. However, the US Army does not have an active surveillance system in place (like FoodNet), so using the FoodNet data was the best option to provide estimates. One input in particular, percent domestically acquired, may have particularly affected the outcomes. The PERT distribution for this model input came directly from FoodNet studies of cases that reported travel outside the USA within 7 days of illness onset [Reference Scallan7]. There was no access to patient records where travel history may (or may not) have been recorded. The US Army population is located worldwide and may be more likely than the general US population to travel to countries where risk of foodborne disease is higher. They also may live in overseas locations where the risk of foodborne disease is higher or even lower. That means the actual percent domestically acquired input for the US Army population could either be higher or lower than the FoodNet estimates. Regardless of these limitations, these data serve as an important baseline of the estimate of foodborne illness caused by five major pathogens. This study also shows that the military population is unique with respect to care-seeking for AGI, stool sample submission and exposure risk, so calculating military-specific underdiagnosis and underreporting multipliers to make foodborne illness burden estimates for the military population is a worthwhile undertaking. The next logical step is to start active surveillance for foodborne illness in the military population so more accurate burden estimates can be calculated in the future.
There are more than 200 known diseases transmitted through food [Reference Mead6]. Foodborne illness can be attributed to viruses, bacteria, parasites, toxins, metals and prions [Reference Mead6]. Estimating the burden of foodborne illness for all causes of foodborne illness was beyond the scope of this present study. Future studies to estimate the burden of illness for all causes of foodborne illness would be helpful to get a better idea of the total burden in the US Army population. Before this lofty undertaking is performed, however, limitations of the current study and previous should be addressed so the most accurate data are produced. Recommendations include: a DoD-sponsored survey of active duty service members across all branches of the military (Air Force, Marines, Navy, etc.); survey of deployed service members to identify unique risk factors for foodborne illness in this population; implementation of a DoD-wide active laboratory-based foodborne illness surveillance system that can monitor trends in the burden of specific foodborne illnesses in the military population over time, detect foodborne illness outbreaks in the military population and attribute the burden of foodborne illness in the military to specific foods and settings; cohort and case-control studies to provide military-specific data for disease burden model inputs; and implementation of specific foodborne illness interventions to modernise the current US Army food protection program aimed at preventing foodborne illness among members of the military.
Supplementary material
The supplementary material for this article can be found at https://doi.org/10.1017/S0950268818003199.
Author ORCIDs
S. B. Mullaney 0000-0003-0948-012X.
Acknowledgements
We thank Dr. Rebecca I. Evans (COL, US Army Veterinary Corps) for serving as the administrative and technical support lead at the Army Public Health Center, Mr. Chris Weir (Army Public Health Center) for programming multiple iterations of the survey questions into the online survey tool, Mr. Zachary D. McCormic (Army Public Health Center) and Susan Gosine (MAJ, US Army) for providing DRSi data, Dr. William F. Nauschuetz (US Army Medical Command) for providing his expertise on US Army Laboratory Services, Dr. Roy N. Emanuel II (Johns Hopkins Applied Physics Lab) for proofreading and final comments on the manuscript, Dr. Elaine Scallan (University of Colorado Denver) for providing her expertise and support throughout the entire project, and Dr. Thomas E. Honadel (COL, US Army Veterinary Corps) for his unwavering support, mentorship and guidance.
Disclaimer
The views expressed in this article are those of the authors and do not reflect the official policy or position of the US Army Public Health Center, US Army Medical Department, Department of the Army, DoD, or the US Government.