INTRODUCTION
Forest disturbance, both due to natural processes and harvesting, affects carbon flux, climate and biodiversity (Dixon et al. Reference Dixon, Solomon, Brown, Houghton, Trexier and Wisniewski1994; Ishii et al. Reference Ishii, Tanabe and Hiura2004; Echeverria et al. Reference Echeverria, Coomes, Salas, Rey-Benayas, Lara and Newton2006). Forest disturbance rates vary greatly depending on forest type, institutional differences and local conditions. For example, average annual deforestation in Russia, which harbours a large portion of the world's forests (Hansen et al. Reference Hansen, Potapov, Moore, Hancher, Turubanova, Tyukavina, Thau, Stehman, Goetz, Loveland, Kommareddy, Egorov, Chini, Justice and Townshend2013), is about 0.56%, and forest disturbance rates vary greatly among Russian regions (Potapov et al. 2011). Within protected areas, disturbance rates are generally lower than outside, but even forests within protected areas are sometimes disturbed by logging (Sieber et al. Reference Sieber, Kuemmerle, Prishchepov, Wendland, Baumann, Radeloff, Baskin and Hostert2013; Bragina et al. Reference Bragina, Radeloff, Baumann, Wendland, Kuemmerle and Pidgeon2015), depending on factors such as the level of protection and regional governance (Wendland et al. Reference Wendland, Baumann, Lewis, Sieber and Radeloff2015). Given the importance of protected areas to safeguarding forest ecosystems, it is important to monitor forest disturbance within protected areas carefully.
Protected areas are essential conservation tools for safeguarding forests, ecosystems and wildlife habitats. However, protected status does not necessarily provide efficient protection (Sieber et al. Reference Sieber, Kuemmerle, Prishchepov, Wendland, Baumann, Radeloff, Baskin and Hostert2013; Bragina et al. Reference Bragina, Radeloff, Baumann, Wendland, Kuemmerle and Pidgeon2015), for reasons such as legal loopholes and weak enforcement (Kuemmerle et al. Reference Kuemmerle, Chaskovskyy, Knorn, Radeloff, Kruhlov, Keeton and Hostert2009). For example, within Russian wildlife sanctuaries (International Union for Conservation of Nature (IUCN) category IV), clearcuts are generally prohibited; however, sanitary clearcuts (i.e. removing trees or stands affected by insects or diseases) and various types of selective logging are allowed (e.g. Forest Management Plan of Altai Krai 2008; Regulations of Beisky Forest Division 2011). The regulations governing wildlife sanctuaries do not define the terms and conditions under which timber is to be harvested, but rather refer to guidelines that are part of the general forest legislation (e.g. Forest Management Regulations 2007). This lack of clear guidance about the conditions under which harvests can occur and the lack of clear harvest limits raises the concern that logging may cause the degradation of wildlife habitats and ecosystems (Fredericksen Reference Fredericksen1998; Matricardi et al. Reference Matricardi, Skole, Pedlowski and Chomentowski2013), especially when the extent of selective logging is unknown. It is important to map forest disturbance both within and outside of protected areas in order to evaluate how well protected areas perform their role of protecting habitats for biodiversity.
Landsat satellite imagery is a powerful data source for detecting forest disturbances (e.g. Healey et al. Reference Healey, Cohen, Zhiqiang and Krankina2005; Kuemmerle et al. Reference Kuemmerle, Hostert, Radeloff, Perzanowski and Kruhlov2007; Masek et al. Reference Masek, Huang, Wolfe, Cohen, Hall, Kutler and Nelson2008; Griffith et al. Reference Griffith, Kuemmerle, Baumann, Radeloff, Abrudan, Lieskovsky, Munteanu, Ostapovicz and Hostert2013). However, most Landsat-based studies have focused on full canopy removal (e.g. Hansen et al. Reference Hansen, Potapov, Moore, Hancher, Turubanova, Tyukavina, Thau, Stehman, Goetz, Loveland, Kommareddy, Egorov, Chini, Justice and Townshend2013; Sieber et al. Reference Sieber, Kuemmerle, Prishchepov, Wendland, Baumann, Radeloff, Baskin and Hostert2013), and few have mapped selective logging (e.g. Lippitt et al. Reference Lippitt, Rogan, Li, Eastman and Jones2008), which is a key forest disturbance type worldwide (Hill et al. Reference Hill, Hamer, Lace and Banham1995; Asner et al. Reference Asner, Keller, Pereira and Zweede2002; Lippitt et al. Reference Lippitt, Rogan, Li, Eastman and Jones2008; Plotnikov Reference Plotnikov2010). Landsat's 30-m resolution makes low-intensity logging difficult to detect, especially when individual disturbances are less than a pixel in size (Lippitt et al. Reference Lippitt, Rogan, Li, Eastman and Jones2008). Examples of accurate mapping of selective logging exist (e.g. in Brazil) (Matricardi et al. Reference Matricardi, Skole, Pedlowski and Chomentowski2013), but detecting selective logging generally remains challenging. Although fewer trees are removed in a selective harvest than in a clearcut, selective logging is a concern; it fragments and changes the structure of wildlife habitat, removing critical elements such as cavity trees and leading to changes in species richness and abundance (Hill et al. Reference Hill, Hamer, Lace and Banham1995; Fredericksen Reference Fredericksen1998). Accurate maps of forest disturbance including both clearcut and selective logging substantially improve estimates of both legal and illegal forest harvesting.
Traditionally, forest disturbance detection has been based on summer growing season satellite imagery, rather than on winter images (e.g. Healey et al. Reference Healey, Cohen, Zhiqiang and Krankina2005; Potapov et al. Reference Potapov, Turubanova, Zhuravleva, Hansen, Yaroshenko and Manisha2012). However winter satellite images can be very useful when mapping forest types (Liira et al. Reference Liira, Püssa and Peterson2006; Tirpak & Giuliano Reference Tirpak and Giuliano2010; Wang et al. Reference Wang, Skidmore, Toxopeus and Liu2009) and harvests in coniferous forests (Tirpak & Giuliano Reference Tirpak and Giuliano2010). Indeed, in European Russia, winter imagery improved the classification of temperate forest disturbance substantially (Baumann et al. Reference Baumann, Ozdogan, Kuemmerle, Wendland, Esipova and Radeloff2012). Including winter images is challenging, because the sun elevation angle at the time of image acquisition is much lower and because differences in snow depth and weather conditions at the time of image acquisition can affect classification accuracy (Püssa et al. Reference Püssa, Liira and Peterson2005; Kardakov et al. Reference Kardakov, Kiviste and Peterson2009). Because snow depth depends on local weather conditions, the accuracy of winter imagery classification varies between regions (Wang et al. Reference Wang, Skidmore, Toxopeus and Liu2009; Wolter et al. Reference Wolter, Townsend, Sturtevant and Kingdon2008), making it difficult to generalize.
Our overarching goal was to evaluate both selective logging and clearcuts in protected and unprotected forests in Western Siberia, a region with a relatively high logging pressure within Russia. Our specific objectives were to compare rates of selective logging and clearcut, to quantify the improvement in mapping accuracy due to the use of summer and winter satellite images and to compare forest disturbance rates within and outside of five protected areas (sanctuaries) in Western Siberia.
METHODS
Study area
Our study area is located within Altai Krai, an administrative region in Western Siberia, Russia, in the foothills of the Altai Mountains (Fig. 1) and covers 30 312 km2. The timber harvesting industry is very important for the local economy, resulting in a high logging pressure (Popriaduhin, Reference Popriaduhin2014). The area is a part of the Priobsky Plateau and is located east of the Ob River. Elevation ranges from 250 to 260 m and the mean temperature is 26–28°C in summer and –20 to –24°C in winter. About 120 days are frost-free. Forest types include temperate deciduous and coniferous forests (Silanteva Reference Silanteva2008). Our study area includes forests on the Ob riverbanks and terraces, including part of the ribbon forests, which are up to 300 km long, are interspersed with non-forest areas and occur naturally due to the underlying geomorphology. The tree species composition is dominated by Scots pine (Pinus sylvestris), aspen (Populus tremula) and birch (Betula pendula). Subdominant species include several types of willows (Salix alba, S. triandra, S. viminalis, S. dasyclados and S. caprea).
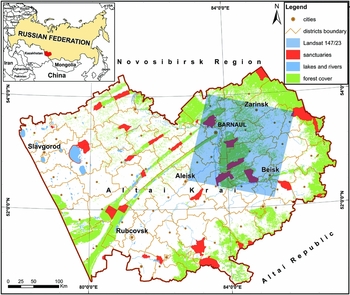
Figure 1 Study area in Altai Krai, Western Siberia, with Landsat footprint path 147/row 23.
Most of the forest has regrown following extensive logging after World War II (Shershnev Reference Shershnev2006). Pine is the most commercially valuable tree species (Paramonov 1997), but most of the pine outside of protected areas has been harvested. Recently, the forests within our study area were classified as ‘protective forests’, a category in Russian forest legislation that implies that a forest provides protection for local ecosystems. A protective forest might or might not be within protected areas (Forest Code of Russian Federation 2006) and commercial clearcuts are banned, although clearcuts to develop infrastructure (e.g. pipelines, electricity lines and fire lines) and sanitary clearcuts to limit the spread of insect and tree pathogen outbreaks are allowed (Forest Management Plan of Altai Krai 2008).
There are five zakaznik (sanctuaries; IUCN category IV) in the study area and they were established to protect game species. In 2007–2008, however, their purpose was extended to protecting the entire ecosystem, including non-game animal and plant species (Regulations of Sanctuaries of Altai Krai 2007, 2008). As such, we focused on forest disturbance in 2009–2013, during the period after these changes. The Bobrovsky Sanctuary (established in 1972) 254 km2, the Kislukhinsky Sanctuary (1976) 362 km2, the Bolsherechensky Sanctuary (1973) 332 km2, the Obskoy Sanctuary (1963) 421 km2 and the Sokolovsky Sanctuary (1993) 403 km². In all sanctuaries, forest is the main land cover (61–96% of the area). The management guidelines within the sanctuary regulations do not explicitly ban timber harvest, and selective logging is allowed; however, the regulations stipulate that nature conservation is the main goal, and they prohibit “any activity that destroys wildlife habitat” (Regulations of Sanctuaries of Altai Krai 2007, 2008).
Data
We acquired six cloud-free Landsat images (L5 Thematic Mapper/L8 Operational Land Manager; path 147, row 23) for the years 2007–2014 from the archives of the United States Geological Survey (Table 1). Landsat Level 1 Terrain data with systematic, radiometric and geometric corrections were used. We obtained forest inventory data from the federal state Roslesinforg enterprise in Novosibirsk (Russia), which were available as vector data and included information about dominant tree species and tree age in each forest compartment and forest stand in our study area (Fig. 2). We also used the forest inventory data as an additional reference in order to validate our training data, because the boundaries of stands and harvest areas usually coincide (i.e. selective logging is conducted throughout the entirety of a given stand). For training and accuracy assessments, we collected ground truth data on selective logging during field surveys in Kislukhinsky Sanctuary and Obskoy Sanctuary in June 2013.
Table 1 List of Landsat imagery used in our analysis. All are from path 147, row 23. TM = Thematic Mapper; OLI = Operational Land Imager.


Figure 2 The neighbourhood of the Bobrovsky Sanctuary (from the east side). Forest inventory data with forest compartments (left) and Landsat 5 Thematic Mapper image (band combination 5, 4 and 3) of the same territory (right). Each individual colour patch indicates a forest stand (i.e. a forest patch with a similar age and tree composition). The forest is mixed with a majority of Scots pine (Pinus sylvestris), but also birch (Betula pendula) and aspen (Populus trémula) trees.
Forest disturbance mapping
To detect forest disturbance, we first generated a map of forest and non-forest areas. We collected 3061 training points in forest areas and 1302 training points in non-forest areas based on visual interpretation of Landsat imagery. We applied the Interactive Data Language-based tool imageRF (Waske et al. Reference Waske, Van der Linden, Oldenburg, Jakimow, Rabe and Hostert2012), which implements a random forest classifier (Breiman Reference Breiman2001) based on two summer images (23 July 2007 and 30 September 2009; see Table 1). The image from 2007 was not used for any further analysis.
In further analysis, we focused on forest areas only. We classified forested area as: (1) stable forest (not disturbed during study period); (2) selectively logged (defined as partial removal of the tree canopy where trees are harvested in no particular spatial pattern); (3) stripcuts (see definition below); and (4) clearcuts. For stable forest, we used the same training data as for forest area mapping in the first step of our analysis, but we excluded disturbed pixels (i.e. those logged between the two image dates). For the selectively logged class, we used 286 training points that we manually collected at the logged plots in the Kislukhinsky and Obskoy Sanctuaries during field work in the summer of 2013. Strip cutting is a type of selective logging in which sections that are 250–300 m long (approximately the length of the forest stand) and 25 m wide are completely harvested to foster natural regeneration of light-demanding species via seed dispersal from the remaining trees or by planting (Regulations on Timber Harvesting 2011). Because the resolution of Landsat imagery (30 m) is roughly equal to the width of the stripcuts, mixed pixels were common. Therefore, we classified stripcuts separately from clearcuts and selective logging. For stripcuts, we identified 459 training points. Clearcuts may include some burned areas, but since forests fires were rare during our study period, we did not separately classify burned areas. We identified 435 training points for clearcuts.
To explore how the classification accuracy varied with the number of images used and their dates, we conducted our classifications with various sets of images (Table 2). For the classifications themselves, we applied a random forest classification algorithm (Breiman Reference Breiman2001). To quantify the accuracy of the models for different image sets, we conducted the fast accuracy assessments as implemented in imageSVM (van der Linden et al. Reference Van der Linden, Wirth, Leitao, Rabe, Suess, Okujeni and Hostert2010), which is a cross-validation of the training data.
Table 2 Cross-validated accuracy assessment based on the training data only.

Accuracy of forest disturbance mapping
To quantify the accuracy of the classifications, we conducted accuracy assessments based on independent data for our best classification (image set 5; see ‘Results’ section), which we used for further analysis. From the classified map, we randomly selected 50 points from each class. Then, we looked at each point using three types of data: high-resolution imagery (Google Earth), medium-resolution imagery (Landsat) and forest inventory data, which provide information about forest species and age. Based on all three sources, we manually assigned each random point. To estimate accuracy, we calculated producer and user accuracies. Producer accuracy shows how well a certain area can be classified (omission error) and user accuracy shows the probability that a pixel on the map represents the category on the ground (commission error). Finally, we calculated the area-adjusted accuracy of each class and the overall accuracy of the map (Olofsson et al. Reference Olofsson, Foody, Stehman and Woodcock2013; Olofsson et al. Reference Olofsson, Foody, Herold, Stehman, Woodcock and Wulder2014).
RESULTS
Forest disturbance mapping
We mapped 6205 km2 of forest, for which we obtained highly accurate forest disturbance maps. The overall area-adjusted accuracy was 97.5% (Table 3) for the best image set (image set 5; Table 2).
Table 3 Area-adjusted accuracy, based on independent ground truth data, for image set 5, which included images from 22 March 2009, 30 September 2009, 1 November 2009, 25 September 2013 and 16 February 2014; overall accuracy was 97.5%. CI = confidence interval.

The total map-based area of all forest disturbances from 2009 to 2013 was 132 km2, or 2.13% of the forested area (0.53% annual rate). We mapped selective logging on 68.1 km2, constituting 51.63% of the total forest disturbance in our study area. Stripcuts encompassed 58.9 km2 or 44.68% of all forest disturbances (Fig. 3); clearcuts encompassed only 4.8 km2 or 3.69% of total disturbances. Thus, selective logging and stripcuts were by far the dominant types of timber harvesting in the study area.

Figure 3 An example of stripcuts where 30-m wide strips of forest alternate with intact forest in order to stimulate regeneration of the forest through seed dispersion. The forest disturbance rate inside of the Bolsherechensky Sanctuary (zoomed in and outlined in yellow) was as big as that outside of the protected area.
Image selection effect
The accuracies of forest disturbance maps varied substantially depending on the date and number of satellite images used (Table 2). The fast accuracy assessment (i.e. our estimate of classification model performance) of two summer images (image set 1) resulted in an overall accuracy of 96.08%, with a producer's accuracy for selective logging of only 63.1%. However, for the second image set, which included winter images only, the accuracy of the selective logging class was much greater (89.8% of user accuracy and 83.5% of producer accuracy).
Assessing summer and winter images in a single data set (image set 3) further improved the overall accuracy (98.4%), as well as the accuracy of the selective logging class (96.4% of user accuracy and 85.3% producer accuracy). Including a larger number of summer and winter images (image sets 4 and 5) resulted in even higher overall accuracies (99.5% and 99.8%, respectively).
Map-based forest disturbance rate within and outside protected areas compare different methods of remote sensing to find the best one for the study area
We detected all types of forest disturbance both inside and outside the protected areas in our study region. In 2009, forests covered 1473 km2 within the protected areas, comprising 24.3% of all forest in our study area (6205 km2; Table 4). By 2013, 37.4 km2 of the protected forests had been disturbed, equivalent to an overall forest disturbance rate of 2.54%, or 0.66% annually. In unprotected forest areas, the total disturbance rate was 1.99% (i.e. 0.50%/year; 94.5 km2) over the same period for accuracy of disturbance estimates (see Table 3). In general, the forest disturbance rates within protected areas were higher than outside, but there were considerable differences between the five sanctuaries (Table 4). The highest forest disturbance rate occurred in Obskoy Sanctuary, where 5.61% of the forest area was disturbed from 2009 to 2013 (Table 4). The smallest areas of disturbance occurred in the Bobrovsky and Sokolovsky Sanctuaries, where only 1.57% and 1.58% of the forest area was disturbed, respectively.
Table 4 Forest disturbance within and outside of protected areas (sanctuaries) in Altai Krai, Western Siberia, 2009–2013; map-based area.

Most of the forest disturbances within protected areas were due to selective logging and stripcuts (Table 4), whereas most clearcuts occurred outside of the protected areas. Specifically, 57.8% (21.6 km2) of all forest disturbances within protected areas was selective logging (1.47% of the protected forests from 2009 to 2013; 0.37% annual rate), 40.6% was stripcut (15.2 km2; 1.03% of the protected forests from 2009 to 2013; 0.21% annually) and only 1.6% was clearcut (0.04% of the protected forest from 2009 to 2013; 0.01% annually). The area of selective logging within protected areas was 21.6 km2, equivalent to 31.7% of the total area of selective logging in our study area. Selective logging was most widespread in Obskoy Sanctuary, where 12.2 km2 of forest was affected, representing more than half of the total selective logging that we detected in protected areas during the study period. The second most common type of disturbance in protected areas was stripcutting, which had been conducted on 15.2 km2, representing 25.8% of all stripcuts in the study area. Among all protected areas, Bolsherechensky Sanctuary was the most disturbed by stripcuts, with 5.6 km2 affected, representing 36.6% of the total stripcuts within protected areas (Fig. 3). The area of clearcuts within protected areas was 0.6 km2, equivalent to 12.7% of the total area of clearcuts in our study area. Among the protected areas, most of the clearcuts were in Obskoy Sanctuary (0.4 km2 affected), representing 62.9% of all clearcuts of protected forest.
DISCUSSION
To our knowledge, our study is the first to map selective logging in temperate forests in Eurasia with such high overall accuracy. We found that the dominant cause of forest disturbance was selective logging. Surprisingly, we also found that forest disturbance rates were substantially higher inside than outside of protected areas, a pattern that seems not to have been reported before from studies of protected area effectiveness.
In our analysis, adding winter satellite images to the Landsat data image set substantially improved the accuracy of our forest disturbance map. In most prior studies, forest disturbance has been mapped with images from the growing season only (e.g. Healey et al. Reference Healey, Cohen, Zhiqiang and Krankina2005; Masek et al. Reference Masek, Huang, Wolfe, Cohen, Hall, Kutler and Nelson2008; Potapov et al. Reference Potapov, Turubanova, Zhuravleva, Hansen, Yaroshenko and Manisha2012). Our study demonstrates that winter imagery can be very useful for mapping different forest types and can improve forest type classifications. Furthermore, forest clearcuts in temperate forests can be more easily detected in winter due to the high radiance contrast in the image data (Püssa et al. Reference Püssa, Liira and Peterson2005; Liira et al. Reference Liira, Püssa and Peterson2006; Kardakov et al. Reference Kardakov, Kiviste and Peterson2009). For example, Pinder et al. (Reference Pinder, Rea and Funsch1999) successfully mapped pine forest with winter images, and Stueve et al. (Reference Stueve, Housman, Zimmerman, Nelson, Webb, Perry, Chastain, Gormanson, Huang, Healey and Cohen2011) improved overall accuracy from 86.3% to 91.2% when including winter imagery in order to map mixed forests of the Great Lake basin. In China, bamboo understory in panda habitats is difficult to map with summer images alone, because both understory and canopy provide similar radiance in summertime, but winter images improved the classification accuracy up to 89% (Wang et al. Reference Wang, Skidmore, Toxopeus and Liu2009). Similarly, the normalized difference vegetation index derived from winter imagery was the most reliable tool for discriminating evergreen and deciduous understory in ruffed grouse habitats in the Appalachian mountains (Tirpak & Giuliano Reference Tirpak and Giuliano2010). Winter images also improved mapping of boreal forests in Canada (Wolter et al. Reference Wolter, Townsend, Sturtevant and Kingdon2008) and temperate forests in Russia (Baumann et al. Reference Baumann, Ozdogan, Kuemmerle, Wendland, Esipova and Radeloff2012). However, to our knowledge, our study is the first to test the value of winter Landsat imagery for mapping selective logging. Interestingly, our classification of winter images alone resulted in higher accuracies than solely classifying summer images, although best results were achieved by combining both summer and winter images. Our results suggest that Landsat imagery is suitable for the classification of selective logging in coniferous forests, especially when multi-season imagery is available.
Our analysis revealed an annual forest disturbance rate of about 0.53%. However, by also mapping selective logging and stripcuts, we found a larger overall proportion of forest disturbance in our study area, which is not detected by classifications that are focused only on clearcuts. In general, the high rates of forest disturbance in our study region were not expected.
In contrast to their stated goal, the sanctuaries in our study area did not provide full protection of the forest (Regulations of Sanctuaries of Altai Krai 2007, 2008), but rather experienced an increased probability of logging. The protection status of Russian sanctuaries (IUCN category IV) allows for some timber harvest (Forest Code of Russian Federation 2006), which means that the legality of selective logging within these sanctuaries depends on the regulations of each protected area. However, the amount of timber that is removed often exceeds what is allowed according to the logging permit (i.e. loggers remove a higher canopy percentage, conduct logging on larger areas or remove the best trees while leaving only decaying and dead trees) (Kabanets Reference Kabanets2013). However, the sanctuary regulations typically do not specify the exact number or size of trees or timber volume that can be removed, but only state in general terms that any timber harvesting that is harmful for wildlife habitat is prohibited (Regulations of Sanctuaries of Altai Krai 2007, 2008). As a result, current regulations provide loopholes that allow the logging of substantial areas (Kuemmerle et al. Reference Kuemmerle, Chaskovskyy, Knorn, Radeloff, Kruhlov, Keeton and Hostert2009), thereby degrading wildlife habitats and threatening wildlife species that depend on the elements, structure or landscape patterns that are characteristic of unharvested forests.
Our study makes a new contribution by measuring both clearcut and selective logging in order to estimate total harvest within protected areas. By comparing harvest within with harvest outside of protected areas, the erosion of natural forest integrity due to harvest becomes apparent. We have demonstrated how a free satellite resource can be used to monitor anthropogenic forest disturbance, and thus facilitate transparency with regards to how local regulation of harvest adheres to the intent of the legislation that is designed to maintain natural forest integrity.
We suggest that the reasons for the high rate of logging within the protected areas are that Scots pine is one of the major tree species in these forests (Forest Management Plan of Altai Krai 2008), the high market price of pine timber during the study period provided strong incentives to harvest it (Paramonov 1997) and the sanctuaries are among the last places where large areas of Scots pine can still be found. Pine forests along the Ob River were largely logged in the 1950s–1960s, before the designation of protected areas in the 1970s slowed down forest harvesting, at least within sanctuaries. Today, there is a high pressure to log valuable trees in protected areas, because other timber sources are depleted (Greenpeace, 2014). However, pine forests are an important component of the region's biodiversity and provide a habitat that safeguards the persistence of many species. For example, these protected forests are habitats for the globally vulnerable greater spotted eagle (Aquila clanga) and white-tailed eagle (Haliaeetus albicilla) (Red List of Altai Krai 2006; IUCN 2015), as well as for many other species. Our analysis suggests that stricter enforcement and less ambiguously worded legislation are needed to ensure sustainable forest use.
CONCLUSION
The spatial and temporal patterns of forest disturbance in Altai Krai, Western Siberia, from 2009 to 2013 indicate forest disturbance rates that are higher than in other Russian regions, and that selective logging and stripcuts were the dominant types of disturbance. Winter Landsat imagery added substantially to the accuracy of forest disturbance maps. Forest disturbance rates within protected areas exceeded those outside protected areas, suggesting that the protected forest ecosystems are under high logging pressure. Institutions that are responsible for maintaining the unique biodiversity of Western Siberian forests should consider the degree to which selective logging may be eroding ecosystem integrity. Our findings highlight that once forest resources outside of protected areas have been depleted, the pressure to log within them is likely to increase.
ACKNOWLEDGEMENTS
We acknowledge the support of D. Helmers in our analysis and M. Baumann for fruitful discussions and advice.
FINANCIAL SUPPORT
This work was funded by the Trust for Mutual Understanding (grant number MSN183221) and the NASA Land Use and Land Cover Change programme (grant number NNX15AD93G).