Introduction
The field of developmental psychopathology has had an enormous impact on our understanding both of development broadly and the development of psychopathology. What once was an intersection between fields, is now a field of study itself. The original goals of developmental psychopathology included bringing a more interdisciplinary approach to understanding child psychiatric disorders and focusing on a developmental systems approach to defining, conceptualizing, and studying the development of risk and resilience across the life span (Cicchetti & Rogosch, Reference Cicchetti and Rogosch1996; Cicchetti, Reference Cicchetti1984; Cicchetti & Toth, Reference Cicchetti and Toth2009; Sameroff, Reference Sameroff1995, Reference Sameroff, Cicchetti and Cohen2009, Reference Sameroff2000; Sroufe, Reference Sroufe2013). This intersection provided an array of critical theories and new approaches that reshaped the way child psychopathology is studied. Development and Psychopathology has been central to the emergence of the field. Thus, in Dante Cicchetti’s last issue as Editor of the Journal, we are honored to reflect on future directions of the field.
As developmental psychopathology has become well established, major progress in developmental neuroimaging has created another new and rapidly developing field. Developmental neuroscience is also an intersection of fields that include neuroscience, developmental science, and often, clinical science and psychiatry. The tools and methods of developmental neuroscience can offer a lot to developmental psychopathology by embedding the brain as a mechanism and central feature in models of development (Hyde, Reference Hyde2015). This approach can merge clinical and psychiatric neuroscience approaches with ecological neuroscience (Hyde et al., Reference Hyde, Gard, Tomlinson, Burt, Mitchell and Monk2020) and within models from developmental psychopathology. However, although these two fields have a lot to offer each other, they need better integration. Developmental neuroscience could benefit from adoption of models from developmental psychopathology, while developmental psychopathology could benefit from continued integration of neuroscience within empirical and theoretical models of development.
Thus, to support the integration of developmental psychopathology and neuroscience, the goals of this paper are: First, to describe major principles of developmental psychopathology and how each principle is being, or can be, applied in developmental neuroscience (Table 1). Second, to highlight how developmental neuroscience can inform the future of developmental psychopathology. Third, we discuss challenges to this integration along with policy and treatment implications.
Table 1. Principles of developmental psychopathology and example applications to developmental neuroscience
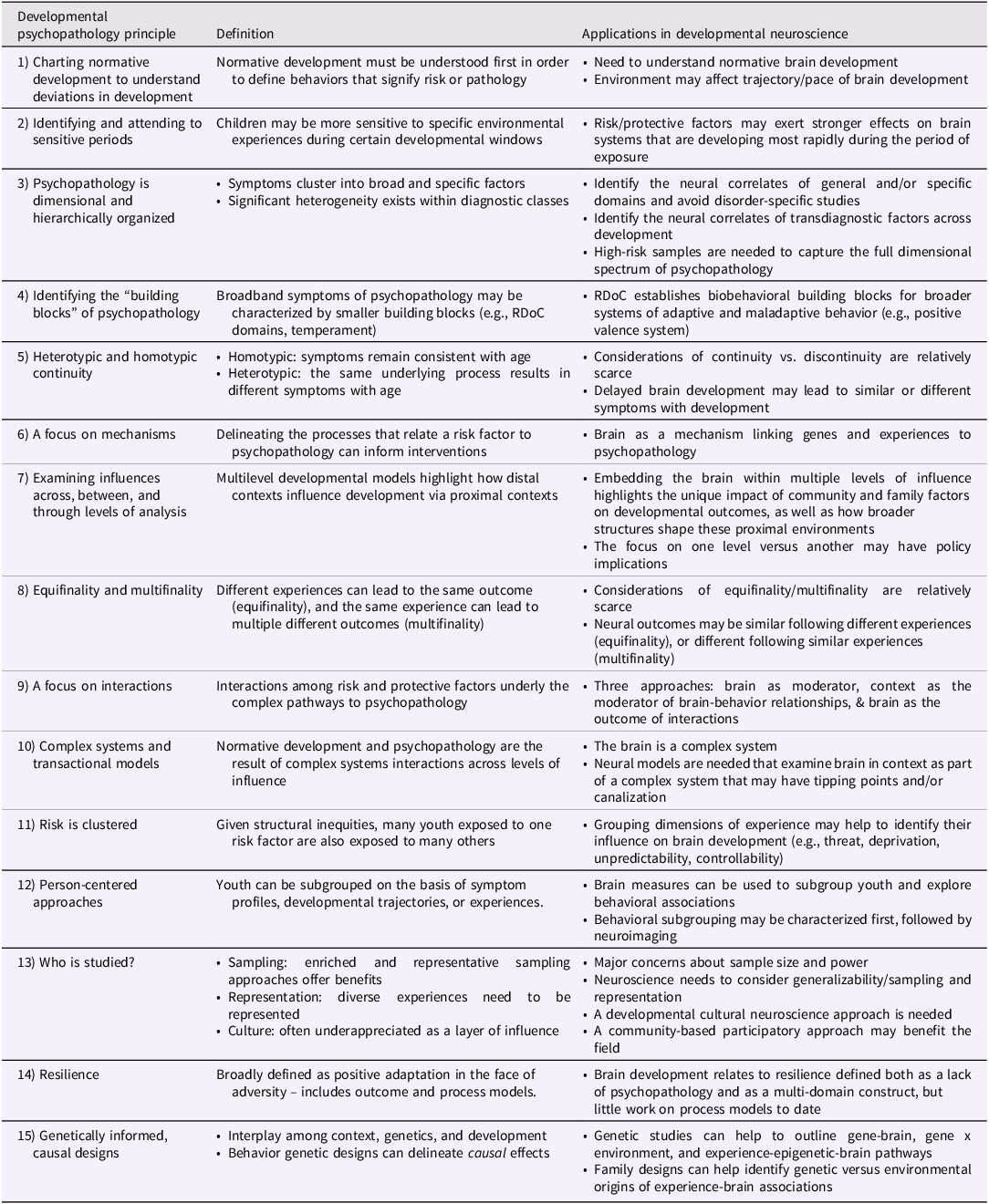
Principles of developmental psychopathology and integration with developmental neuroscience
Charting normative development to understand deviations in development
A major initial thrust of developmental psychopathology was that we must understand normative development to define behaviors or developmental pathways that signify risk. For example, identifying that some aggression is common in toddlerhood, but not adulthood, highlights that an incident of biting may not be concerning for a 1-year-old, but would be for a 20-year-old. This notion prompts the need for longitudinal studies to chart normative, within-person changes with age. Broadly, this principle has shifted the view of child development and psychopathology to understand that it is the trajectory and timing of symptoms, traits, and behaviors that matter, resulting in conceptualizations that psychiatric symptoms and categories should not be static, but rather depend on developmental stage.
One example of this approach is the development of antisocial behavior. Through prodigious theory and quantitative advances, research has revealed distinct trajectories of antisocial behavior including an early onset subtype that is especially chronic and an adolescent onset subtype that may be more “normative” with a greater chance of desistence. This research has informed intervention by focusing more intensive approaches to preventing early onset subtypes (Moffitt, Reference Moffitt2018).
Applications to developmental neuroscience
Although clinical developmental neuroscience examines how the brain relates to psychopathology, we must first understand normative brain development. Charting brain development is difficult because of limited longitudinal datasets, which suggest different conclusions about brain development than cross-sectional studies. For example, cross-sectional work suggests linear, whereas longitudinal work suggests curvilinear, declines in cortical volume with age (Giedd et al., Reference Giedd, Blumenthal, Jeffries, Castellanos, Liu, Zijdenbos, Paus, Evans and Rapoport1999; Jernigan et al., Reference Jernigan, Trauner, Hesselink and Tallal1991). Similarly, cross-sectional work suggests that prefrontal-amygdala connectivity, which is critical for emotion regulation, switches from positive to negative during adolescence (Gee, Humphreys, et al., Reference Gee, Humphreys, Flannery, Goff, Telzer, Shapiro, Hare, Bookheimer and Tottenham2013). Conversely, recent longitudinal work has found no consistent maturational changes in prefrontal-amygdala connectivity (Bloom et al., Reference Bloom, VanTieghem, Gabard‐Durnam, Gee, Flannery, Caldera, Goff, Telzer, Humphreys, Fareri, Shapiro, Algharazi, Bolger, Aly and Tottenham2022). However, this emerging longitudinal work is still small (N = ∼100) with few scans (1–3 MRI scans). Therefore, our understanding of normative brain development is still limited, illustrating the need for longitudinal data.
Developmental neuroscience has also related environmental risk factors to differences in brain structure and function (Johnson et al., Reference Johnson, Riis and Noble2016; McLaughlin et al., Reference McLaughlin, Sheridan and Lambert2014), but the meaning of these differences is not always clear. Based on life history theory suggesting that adversity accelerates child development, promoting “adult-like” functioning earlier to maximize survival (Ellis & Del Giudice, Reference Ellis and Del Giudice2019), one key theory is that adverse experiences may affect the pace of brain development (Callaghan & Tottenham, Reference Callaghan and Tottenham2016). Results are mixed about whether adversity may accelerate or delay brain development. For example, some work suggests that caregiving-related adversity is linked to more positive fronto-amygdala connectivity (Gard et al., Reference Gard, Hein, Mitchell, Brooks-Gunn, McLanahan, Monk and Hyde2022), which may reflect delayed maturation, whereas other work finds this adversity to be related to negative connectivity (Gee, Gabard-Durnam, et al., Reference Gee, Gabard-Durnam, Flannery, Goff, Humphreys, Telzer, Hare, Bookheimer and Tottenham2013), which could represent accelerated development. Studies relating neighborhood disadvantage to network connectivity also report similarly conflicting results (Michael et al., Reference Michael, Tillem, Sripada, Burt, Klump and Hyde2023; Tooley et al., Reference Tooley, Mackey, Ciric, Ruparel, Moore, Gur, Gur, Satterthwaite and Bassett2020). The few longitudinal studies in this area also yield conflicting findings: Abuse and neglect have been related to accelerated network development (Rakesh et al., Reference Rakesh, Allen and Whittle2023), whereas neighborhood disadvantage has been linked to delayed structural development (Whittle et al., Reference Whittle, Vijayakumar, Simmons, Dennison, Schwartz, Pantelis, Sheeber, Byrne and Allen2017). These conflicting findings emphasize the need for longitudinal studies to first characterize normative trajectories of brain development, and then identify how context modulates this trajectory.
Identifying and attending to sensitive periods
A key concept in developmental psychopathology is that there are periods during which children may be more sensitive to certain environmental inputs. The first evidence for sensitive periods came from animal models showing critical periods in the brain for the development of vision (e.g., Wiesel & Hubel, Reference Wiesel and Hubel1963). This work spawned the desire to identify sensitive periods during which specific experiences have stronger effects, which could inform the timing of more effective interventions.
Applications to developmental neuroscience
Interest in sensitive periods is growing in developmental neuroscience and suggests that experiences may exert more potent effects on the brain systems that are developing most rapidly during the period of exposure. For example, subcortical regions develop rapidly early in life, whereas cortical regions continue to develop across adolescence (Lupien et al., Reference Lupien, McEwen, Gunnar and Heim2009). Consistent with this trajectory, retrospectively reported sexual abuse during early childhood was related to subcortical volume in young adulthood, whereas sexual abuse during adolescence was related to cortical volume in young adulthood (Andersen et al., Reference Andersen, Tomada, Vincow, Valente, Polcari and Teicher2008). Similar findings are reported in prospective studies measuring adversity (neighborhood disadvantage, harsh parenting) longitudinally across development, and brain function during adolescence (Gard et al., Reference Gard, Maxwell, Shaw, Mitchell, Brooks‐Gunn, McLanahan, Forbes, Monk and Hyde2021, Reference Gard, Shaw, Forbes and Hyde2022). This work extends to protective factors which can identify neurodevelopmental windows of opportunity. For example, in a prospective longitudinal study, maternal support during preschool was uniquely linked to subcortical (caudate) volume, whereas maternal support during elementary years was uniquely linked to cortical (insular) volume (Luby et al., Reference Luby, Tillman and Barch2019). Though promising, this work is still relatively new and most studies measure context longitudinally, but the brain cross-sectionally. To really delineate sensitive periods, we need both experience and brain probed at multiple time points.
Psychopathology is dimensional and hierarchically organized
Early work in developmental psychopathology identified that symptoms cluster into two domains: internalizing and externalizing (Achenbach, Reference Achenbach1966). Recent work revealed a potential general “p factor,” as well as other confirmed broadband (e.g., internalizing, externalizing) factors that organize psychiatric symptoms (Caspi et al., Reference Caspi, Houts, Belsky, Goldman-Mellor, Harrington, Israel and Poulton2014; Lahey et al., Reference Lahey, Applegate, Hakes, Zald, Hariri and Rathouz2012). This hierarchical model of the structure of psychopathology has been codified by the HiTOP consortium, which contrasts traditional classification systems (e.g., DSM-5) by delineating shared features of commonly comorbid diagnostic categories (e.g., anxiety, depression; Kotov et al., Reference Kotov, Krueger, Watson, Cicero, Conway, DeYoung, Eaton, Forbes, Hallquist, Latzman, Mullins-Sweatt, Ruggero, Simms, Waldman, Waszczuk and Wright2021; Krueger & Markon, Reference Krueger and Markon2006). Moreover, these models, along with other quantitative work (Krueger & Markon, Reference Krueger and Markon2011; Plomin et al., Reference Plomin, Haworth and Davis2009), emphasize that symptoms of most common mental disorders are dimensional, not categorical. This work has supported and extended models in developmental psychopathology demonstrating that dimensions of development span from normative to maladaptive. Collectively, this literature highlights the need for work in normative samples, enriched samples, and samples recruited via exposure, rather than case-control approaches used more typically in psychiatry, which miss the dimensionality of symptoms across the population.
In parallel, while meta-factors of psychopathology are organized hierarchically, there is also tremendous heterogeneity within distinct diagnostic classes. For example, in the diagnosis of Conduct Disorder, there are now two subtyping approaches (early versus late onset, presence versus absence of limited prosocial emotions), that help delineate groups of youth with different etiologies, trajectories, and outcomes (American Psychiatric Association, 2013; Frick et al., Reference Frick, Ray, Thornton and Kahn2014; Moffitt, Reference Moffitt2018). Beyond subgroups, calls for personalized models of psychopathology argue that psychopathology is organized dimensionally and hierarchically, and contains substantial heterogeneity at the individual level, necessitating approaches that merge population-level accounts with person-level models (Wright & Woods, Reference Wright and Woods2020).
Applications to developmental neuroscience
This complex conceptualization of psychopathology and how it develops across the lifespan, has an array of implications for developmental neuroscience. First, methodologically, this work highlights potential limitations of clinical neuroscience that mainly compares brain structure and function between those with and without a specific categorical disorder. Probing the neural correlates of a specific diagnosis is not likely to have major implications for our understanding of psychopathology, nor its prevention and treatment. Rather, work connecting brain structure and function dimensionally to broadband factors (e.g., p factor, internalizing/externalizing factors), while also examining links to specific syndromes and symptoms, can explain the neural patterns that increase risk for broad versus narrow phenotypes (Hyde, Reference Hyde2015). For example, a combination of biometric, quantitative, and EEG research has helped identity that the externalizing factor is related to disinhibition, as captured by the P300 in EEG studies (Iacono et al., Reference Iacono, Malone and McGue2008). In parallel, studies have elucidated why some youth with disinhibition primarily show ADHD symptoms, whereas others are oppositional or engage in substance use (Iacono et al., Reference Iacono, Malone and McGue2008).
Identifying the “building blocks” of psychopathology
In addition to hierarchical and dimensional models, theory and empirical work have attempted to identify the building blocks of broadband symptoms of psychopathology (e.g., cognitive, affective, social processes). The most widely articulated of these models is the Research Domain Criteria (RDoC) (Insel et al., Reference Insel, Cuthbert, Garvey, Heinssen, Pine, Quinn, Sanislow and Wang2010). This initiative has outlined levels from genes and molecules to cells and circuits up to constructs including positive and negative valence, cognitive, social, and sensorimotor systems. This approach aims to address the issues of comorbidity in traditional diagnostic systems by characterizing the underlying neurobiobehavioral processes that give rise to specific symptoms or transdiagnostic constructs (Figure 1). Thus, whereas HiTOP takes a top-down structural approach by organizing symptoms, RDoC takes a bottom-up approach to identify clusters of biobehavioral processes that interact to give rise to symptoms. When combined (Michelini et al., Reference Michelini, Palumbo, DeYoung, Latzman and Kotov2021), these models can specify how distinct biobehavioral processes can lead to broad hierarchical dimensions of psychopathology (e.g., for an elegant developmental psychopathology model of externalizing see Beauchaine & McNulty, Reference Beauchaine and McNulty2013).

Figure 1. Multilevel Biobehavioral Model of Psychopathology.
Applications to developmental neuroscience
As some of these models (e.g., RDoC) are rooted in neuroscience, developmental neuroscience has been making strong progress in identifying the building blocks of psychopathology and its transdiagnostic factors (e.g., irritability, anhedonia). For example, though irritability has been related to an array of clinical phenotypes (e.g., oppositional defiant disorder, depression, mania), developmental neuroscience has offered insight into consistent neural correlates of irritability, such as altered reward and threat processing in prefrontal and subcortical regions (Brotman et al., Reference Brotman, Kircanski and Leibenluft2017). Beauchaine and Hinshaw (Reference Beauchaine and Hinshaw2020) describe that an important future direction will be to take a “neural systems approach” emphasizing the RDoC building blocks underlying such transdiagnostic factors, rather than a “disorder-first approach.” Within this framework, variability in the brain may map more directly to narrower and homogenous building blocks, rather than directly onto complex, overlapping, and heterogeneous clinical diagnostic constructs (Ofrat & Krueger, Reference Ofrat and Krueger2012; Plomin et al., Reference Plomin, Haworth and Davis2009). One day, we may think more of various clinical diagnoses in terms of their building blocks (e.g., low reward, high emotionality), which will explain their overlapping and hierarchical structure, as well as why certain neural, genetic, and experiential variables map on to general vs. specific psychopathology outcomes (e.g., Dillon et al., Reference Dillon, Rosso, Pechtel, Killgore, Rauch and Pizzagalli2013).
Indeed, the RDoC framework has already begun to reveal the potential brain systems supporting many of the constructs and subconstructs within each system (e.g., aspects of the amygdala have been identified at the circuit level for the acute threat/fear subconstruct). However, while the RDoC approach has great potential, there is still little developmental RDoC research, and until recently (Pacheco et al., Reference Pacheco, Garvey, Sarampote, Cohen, Murphy and Friedman-Hill2022; Sanislow et al., Reference Sanislow, Morris, Cuthbert and Pacheco2022), both development and the environment were not explicitly integrated into the RDoC matrix. Fortunately, recent research at the intersection of developmental psychopathology and developmental neuroscience has begun to articulate candidate environmental dimensions, which may cluster like RDoC domains. For example, the developmental model of adversity and psychopathology has posited dimensions of adversity that are clustered into threat and deprivation domains (McLaughlin et al., Reference McLaughlin, Sheridan and Lambert2014), with recent models also positing a dimension of unpredictability (Cohodes et al., Reference Cohodes, Kitt, Baskin-Sommers and Gee2021). These types of approaches can bridge RDoC to the environment by articulating patterns of clustering among experiences that link to RDoC domains, which will enrich our understanding of psychopathology as we delineate how these constructs unfold across development (Sanislow et al., Reference Sanislow, Morris, Cuthbert and Pacheco2022).
Heterotypic and homotypic continuity
One challenge to understanding typical and atypical development is that the same behavior has different meanings, underlying causes, and outcomes at different ages (Hyde, Reference Hyde2015). A temper tantrum at 2 is normative, may reflect typical development, and result in minor consequences (e.g., a time-out). A temper tantrum at 15 could have different underlying causes or could be caused by the same process that is now non-normative at this age (e.g., emotion dysregulation). It is critical to consider which behaviors, and at which ages, we expect homotypic continuity (the same behavior or trait is consistent across age) versus heterotypic continuity (the same underlying process results in different phenotypes across age). For example, different antisocial behaviors may represent age-specific presentations of the same underlying psychopathology (Figure 2): difficult temperament in early childhood, ADHD and oppositional defiant disorder in middle childhood, conduct disorder in adolescence, and substance use and antisocial personality disorders in adulthood (Beauchaine & McNulty, Reference Beauchaine and McNulty2013). These distinct behaviors reflect the same underlying vulnerability (e.g., impulsivity, disinhibition), which could stem from a consistent underlying cause that yields different behaviors across age.
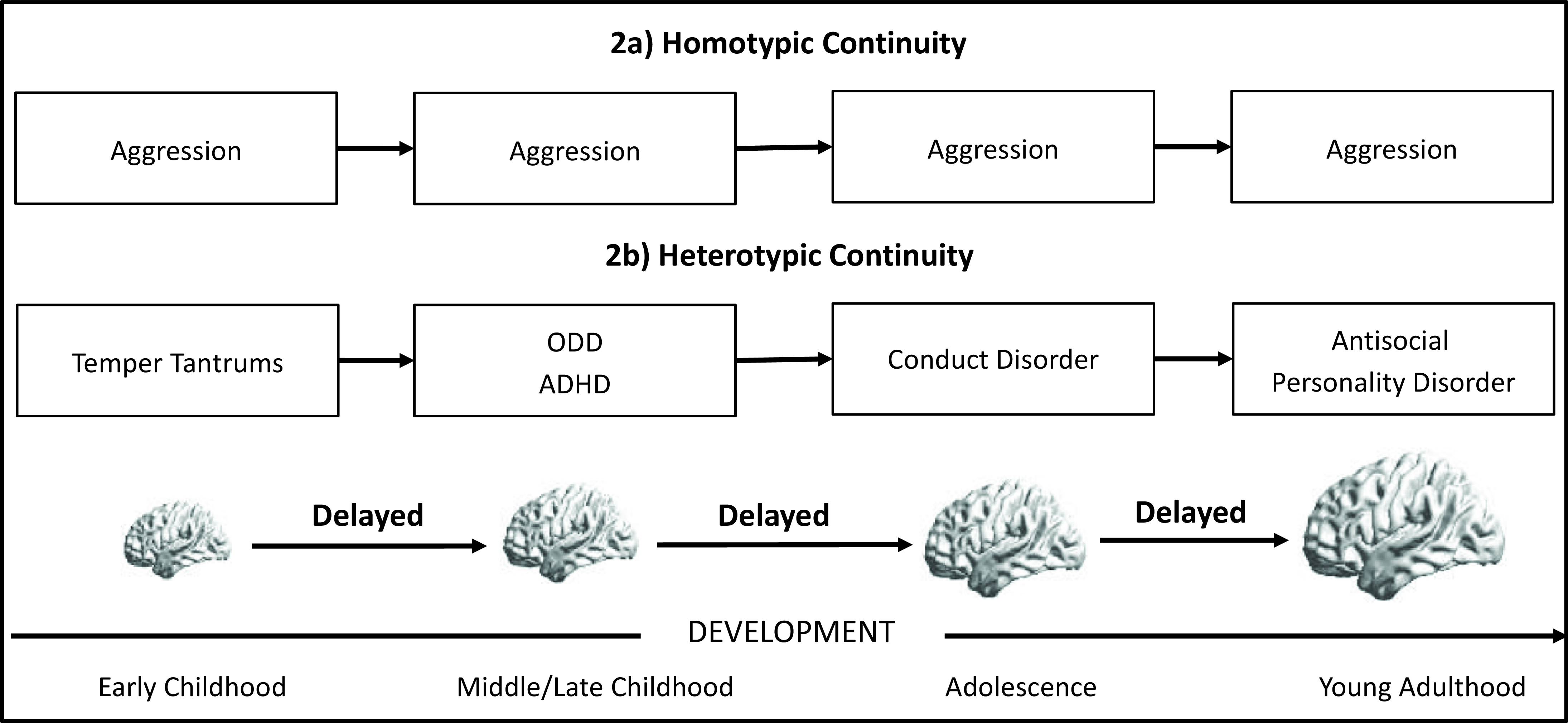
Figure 2. Developmental Continuity.
Applications to developmental neuroscience
Applying these principles to developmental neuroscience raises intriguing questions about the nature of continuity in complex behaviors (Figure 2). The same neural phenotype (e.g., high limbic reactivity) may yield persistent anxiety (homotypic) or anxiety in childhood but aggression in adolescence (heterotypic). Moreover, specific neural phenotypes may not represent a static indicator of pathology because brain function shifts with development, and with these shifts, may still reflect continuity in the same underlying process. For example, delayed brain development is linked to ADHD in childhood (Shaw et al., Reference Shaw, Eckstrand, Sharp, Blumenthal, Lerch, Greenstein, Clasen, Evans, Giedd and Rapoport2007) and a history of conduct problems in young adulthood (Sanford et al., Reference Sanford, Ge, Antoniades, Modabbernia, Haas, Whalley, Galea, Popescu, Cole and Frangou2022). Thus, delayed maturation of specific brain systems could reflect an underlying vulnerability that presents differently with age both at the neural and behavioral level.
Another possibility is that brain development represents an endophenotype of continuity. That is, the underlying continuity could be static genetic vulnerability, which could then give rise to different neural and behavioral phenotypes over time. For example, depression has been related to affective systems during preschool (Gaffrey et al., Reference Gaffrey, Luby, Belden, Hirshberg, Volsch and Barch2011), accompanied by reward systems in adolescence (Chahal et al., Reference Chahal, Gotlib and Guyer2020) and cognitive systems in adulthood (Kerestes et al., Reference Kerestes, Davey, Stephanou, Whittle and Harrison2014). Synthesizing across these studies suggests that symptoms and neural correlates may shift with age, with potential “continuity” arising at a different (e.g., genetic) level. Ultimately, this discussion highlights the challenge of understanding heterotypic continuity, as we need to better understand at what level there is continuity versus change. Addressing this question will require longitudinal, multilevel designs to identify whether the brain represents a continuous marker of underlying vulnerability to, or dynamically changes to reflect shifts in, expressions of psychopathology across development.
A focus on mechanisms
Much research in developmental psychopathology has focused on mechanisms – that is, why does a risk factor relate to a specific form of psychopathology? Ecological theories (e.g., the family stress model) emphasize how distal risk shapes development via proximal risk factors and mechanisms. A focus on mechanisms is critical for informing intervention by identifying mechanisms for change (Hyde, Reference Hyde2015). For example, research in both internalizing (Abramson et al., Reference Abramson, Seligman and Teasdale1978) and externalizing disorders (Dodge, Reference Dodge1993) has emphasized the mechanistic role of cognitions in the development of psychopathology. This basic work then shaped important treatment approaches for depression and conduct problems targeting maladaptive cognitions (Beck, Reference Beck1976; Conduct Problems Prevention Research Group, Reference Bierman2002).
Applications to developmental neuroscience
Developmental neuroscience offers exciting avenues to delineate how the brain represents a mechanism that confers risk or promotes resilience following different experiences (or to link gene x environment interactions to psychopathology; Hyde et al., Reference Hyde, Swartz, Waller, Hariri and Cicchetti2014; Hyde, Reference Hyde2015). For example, in examining the brain as a mediator (Figure 3), greater neighborhood disadvantage was linked to worse response inhibition via prefrontal activation during a cognitive control task (Tomlinson et al., Reference Tomlinson, Burt, Waller, Jonides, Miller, Gearhardt, Peltier, Klump, Lumeng and Hyde2020). As another example, greater household instability in childhood predicted depressive symptoms in young adulthood via more efficient neural information flow during adolescence (Hardi, Goetschius, Tillem, et al., Reference Hardi, Goetschius, Tillem, McLoyd, Brooks-Gunn, Boone, Lopez-Duran, Mitchell, Hyde and Monk2023).

Figure 3. Theoretical Models of Biology X Environment X Mental Health.
Although these examples highlight the utility of the brain as mediator approach, there are challenges. First, to really test a mechanistic mediation model, longitudinal measurement of predictors, mediators, and outcomes are needed. Second, the described studies are the exception, rather than the norm. Many studies in developmental neuroscience study adversity-brain associations without explicitly relating brain alterations to behavioral outcomes. This approach is problematic because without directly anchoring brain to behavior, we risk speculating that brain changes reflect the toxic effects of adversity, even though they could actually reflect adaptation (Gee, Reference Gee2021). For example, stress-related changes in cortico-limbic connectivity may confer resilience against internalizing symptoms (Brieant et al., Reference Brieant, Sisk and Gee2021).
Examining influences across, between, and through levels of analysis
Multilevel developmental models are a core feature of developmental psychopathology (Figure 1). Early work by Bronfenbrenner emphasized that development is the result of multiple levels of influence from distal (e.g., culture, social structures) to proximal contexts (e.g., the family), and that more distal influences often influence development via more proximal contexts (Bronfenbrenner & Ceci, Reference Bronfenbrenner and Ceci1994). This work has also given rise to cascade models (e.g., Dodge et al., Reference Dodge, Malone, Lansford, Miller, Pettit and Bates2009), which articulate how risk at one stage (or in one level), can lead to later risk across development and across different levels of influence.
The Family Stress Model (Conger & Donnellan, Reference Conger and Donnellan2007) is a prominent model that articulates some of these levels and how distal contexts influence development. In this case, economic hardship impacts youth by creating instability and stress for the parent, which in turn, undermines the parents’ emotional resources, which impacts family relationships (parent-parent, parent-child), leading to less optimal parenting, which in turn, increases risk for maladjustment. This model, along with a similar family investment model (Conger & Donnellan, Reference Conger and Donnellan2007), has been widely supported and helps to articulate how broad policies can influence the child mechanistically via family process (Masarik & Conger, Reference Masarik and Conger2017).
Applications to developmental neuroscience
Outside of cumulative risk approaches (see below), developmental neuroscience is only just starting to examine the brain embedded within multiple levels of influence (see theory from ecological neuroscience: Hyde et al., Reference Hyde, Gard, Tomlinson, Burt, Mitchell and Monk2020). For example, one study assessed the unique prediction of neighborhood (poverty) versus parent (family income, maternal depression) versus parenting (harsh parenting) influences on amygdala reactivity longitudinally in an at-risk cohort (Gard et al., Reference Gard, Shaw, Forbes and Hyde2017). Similarly, Ip et al. (Reference Ip, Sisk, Horien, Conley, Rapuano, Rosenberg, Greene, Scheinost, Constable, Casey, Baskin-Sommers and Gee2022) explored multilevel influences on the relationship between resting-state functional connectivity and internalizing symptoms across two levels of socioeconomic disadvantage: household resources (e.g., family material hardship) and neighborhood disadvantage (e.g., area deprivation). However, these are relatively rare examples and did not embed the brain within more complex multilevel models. Ultimately, more work is needed that embeds the developing brain within a multilevel model of influence. At the very least, a recent focus on broader structural factors (e.g., neighborhood disadvantage, structural racism; Barch, Reference Barch2022; Dumornay et al., Reference Dumornay, Lebois, Ressler and Harnett2023; Tomlinson et al., Reference Tomlinson, Burt, Waller, Jonides, Miller, Gearhardt, Peltier, Klump, Lumeng and Hyde2020) demonstrates increasing appreciation that more distal factors influence brain development. Looking forward, a shift in where the field focuses (e.g., structural vs. family factors) and an appreciation for how risk filters across levels, is important in terms of the policy implications that will follow from this work (Hyde et al., Reference Hyde, Gard, Tomlinson, Burt, Mitchell and Monk2020). If most work focuses on family-level factors (e.g., family poverty, parenting), then it may drive intervention and policy towards families, placing the onus on them. Alternatively, if studies highlight how broader structural factors influence these more proximal factors (e.g., how societal structures at the legal or community level may indirectly influence developing brain systems through their impact on parenting practices), it may drive policy and intervention towards broader structural changes.
Equifinality and multifinality
Since its inception, developmental psychopathology has emphasized that risk and resilience can reflect both equifinality – when different experiences lead to the same outcome – and multifinality – when the same experience leads to different outcomes (Cicchetti & Rogosch, Reference Cicchetti and Rogosch1996). For example, child maltreatment can lead to multiple forms of psychopathology, demonstrating multifinality (Baldwin et al., Reference Baldwin, Wang, Karwatowska, Schoeler, Tsaligopoulou, Munafò and Pingault2023). In contrast, both harsh parenting and deviant peer influences can both lead to antisocial behavior, demonstrating equifinality (Dishion & Patterson, Reference Dishion, Patterson, Cicchetti and Cohen2006). These concepts illustrate that many risk factors are not specific to one outcome, and that there are likely multiple distinct etiological pathways to any outcome. They also highlight that pathways to psychopathology are complex and probabilistic: the development of psychopathology is the product of interacting risk and protective factors across time.
Applications to developmental neuroscience
Equifinality in developmental neuroscience research has been examined in several ways. First, studies have considered equifinal pathways to the brain as the outcome. For example, in one study both neighborhood disadvantage and harsh parenting at age 2 were associated with amygdala reactivity to emotional faces in young adulthood (Gard, Shaw, et al., Reference Gard, Waller, Shaw, Forbes, Hariri and Hyde2017). Second, studies examining the brain as the predictor have found that multiple different neural phenotypes are associated with the same outcome. For example, greater connectivity between salience, default mode, and cognitive control networks were each related to higher hyperactivity/impulsivity symptoms among at-risk children (Jones et al., Reference Jones, Monaghan, Leyland-Craggs, Astle and Team2023).
Similar patterns have been explored with multifinality in outcomes. Considering the brain as the outcome, adversity has been associated with alterations in cortico-limbic connectivity in some studies, but not others (McLaughlin et al., Reference McLaughlin, Weissman and Bitrán2019). Considering the brain as the predictor, cortico-limbic circuitry has been implicated in transdiagnostic psychopathology in youth (Dugré et al., Reference Dugré, Eickhoff and Potvin2022). Overall, the same environmental insults may relate to different neural outcomes, and the same neural profiles may lead to different phenotypic outcomes, each illustrating multifinality.
These examples demonstrate equifinality and multifinality when considering the brain as the outcome or as the predictor. However, these concepts tend not to be widely discussed, nor be included in core theory in developmental neuroscience. Neuroimaging studies should test these concepts more explicitly to characterize how and when environmental exposures become neurobiologically embedded, as well as how and why specific patterns of brain organization confer risk versus resilience.
A focus on interactions
With rich theory in developmental psychopathology indicating complex pathways to psychopathology, there has never been a focus on single “causes,” but rather interactions of risk and protective factors. Examining interactions among these factors can identify who is at risk, in which contexts risk matters, and when those risk factors may be most potent. This approach has translational value, as it identifies who should be targeted for prevention/intervention (e.g., children with temperamental risk who live in a risky neighborhood) and which protective factors blunt risk (e.g., high parental monitoring) and thus can be the focus of intervention (e.g., increase parental monitoring).
One application of this interaction framework has been an appreciation of person-context fit and interactions. For example, early diathesis-stress models articulated that some individuals may have a diathesis (risk) for psychopathology, that is activated during stress. More recent models, like biological sensitivity to context (Ellis & Boyce, Reference Ellis and Boyce2008) and differential susceptibility (Belsky & Pluess, Reference Belsky and Pluess2009), posit that some individuals may be particularly sensitive to specific contexts for better or worse. They may flourish more in positive environments and have worse outcomes in negative environments. These theories have identified characteristics like temperament, genes, and brain structure and function, as potential markers of susceptibility, making them ripe for investigation in developmental neuroscience.
Applications to developmental neuroscience
Emerging work in developmental neuroscience points towards significant interactions between the developing brain and environmental stressors, with three main models underlying this work (Figure 3). First is work that examines brain × environment interactions (brain as the moderator) (Guyer, Reference Guyer2020). For example, Mexican-origin adolescents with larger hippocampal volume showed greater depressive symptoms when exposed to community crime, but fewer depression symptoms when they reported family connectedness (Schriber et al., Reference Schriber, Anbari, Robins, Conger, Hastings and Guyer2017). In work on differential susceptibility, greater socioeconomic resources at age 20 predicted less antisocial behavior and greater income at age 22 for young men with high, but not low, amygdala reactivity (Gard et al., Reference Gard, Shaw, Forbes and Hyde2017). These examples reveal how brain structure and function can identify individuals who may be more susceptible to specific risk and protective factors.
Second are models identifying how the environment moderates links between brain and behavior (context as the moderator of brain-behavior relationships). For example, threat-related amygdala reactivity was linked to anxiety in middle-aged adults, but only in those who reported low social support (Hyde et al., Reference Hyde, Gorka, Manuck and Hariri2011). In a sample of low-income urban adolescents, Hardi, Goetschius, McLoyd, et al., (2023) found that exposure to economic adversity during the COVID-19 pandemic moderated the association between neural reactivity to faces and symptoms of anxiety. These studies suggest that brain-behavior relationships may be contingent on socioecological context. Third is work examining whether risk and protective factors interact to predict brain structure and function (brain as the outcome of interactions). For example, in a sample of adolescent twins from low-income neighborhoods, exposure to community violence was related to amygdala reactivity to threat, but this association was diminished in youth with warm and involved parents (Suarez et al., Reference Suarez, Burt, Gard, Klump and Hyde2024).
In sum, there is emerging work in developmental neuroscience examining an array of different types of interactions, suggesting that complex and probabilistic interactive pathways are more realistic than simple main effect models. However, statistical power is an issue, as interactions require large sample sizes for adequate power (McClelland & Judd, Reference McClelland and Judd1993). Moreover, to really map these interactions, the predictors and outcomes must be represented in the data across the range of the potential values (e.g., from poverty to high income; from depression to satisfaction with life) to characterize whether interactions represent diathesis-stress or differential susceptibility, and whether the conditional mechanisms are specific to the full or select ranges of the data. Few current neuroimaging studies are large enough to reliably explore these interactions, and many large-scale studies may under-represent some environments which are key to these questions (e.g., those with the lowest incomes are underrepresented in national studies; Gard, Hyde, et al., Reference Gard, Hyde, Heeringa, West and Mitchell2023).
Complex systems and transactional models
Developmental psychopathology has had a long tradition of emphasizing complex systems and transactional models. For example, Sameroff’s seminal work on the transactional model (Sameroff, Reference Sameroff2009) and on developmental systems in developmental psychopathology (Sameroff, Reference Sameroff, Cicchetti and Cohen1995, Reference Sameroff2000) highlight the complex and ongoing transactions between the child and environment, as well as the ways in which these systems are mutually defining. This work, along with recent work on complex biological systems (Marshall, Reference Marshall2013) and complexity theory (Kauffman, Reference Kauffman1996), highlight how human development is the result of complex systems interactions across levels (Bronfenbrenner & Morris, Reference Bronfenbrenner, Morris, Lerner and Damon2007). Though these appealing models likely represent the true course of development (and psychopathology), the challenge is measuring and modeling these complex transactions within complex systems. For example, Patterson’s coercion theory and Dishion’s work on deviant peer influences, are good examples of transactional models of the development of psychopathology (Dishion & Patterson, Reference Dishion, Patterson, Cicchetti and Cohen2006). In both cases, the child and parent or peer shape each other’s behavior across multiple micro-interactions, which, in turn, change behaviors at larger time scales and into enduring patterns of behavior. However, even these models focus mostly on two actors and on a relatively small universe of variables and timescales. Thus, a challenge for the field of developmental psychopathology will be to better articulate and measure these multi-time scales and multilevel models that dynamically unfold over time. The brain is a key component of these models and is, itself, a complex system (Marshall, Reference Marshall2013).
Applications to developmental neuroscience
There has been little empirical work embedding the brain within these complex models of influence. However, theoretical work is beginning to articulate the ways in which neuroscience can be integrated into complex developmental psychopathology systems (Hyde et al., Reference Hyde, Swartz, Waller, Hariri and Cicchetti2014; Hyde, Reference Hyde2015). For example, Wiggins and Monk (Reference Wiggins and Monk2013) outline a translational neuroscience framework for investigating socioemotional functioning across development which integrates Sameroff’s transactional framework to hypothesize that links between brain function, genetic activity, behavior, and environmental conditions exhibit bidirectional effects on one another. Looking ahead, a basic direction forward for developmental neuroscience is to conceptualize the brain as embedded within a complex system of influences (Figure 4). This “brain in context” approach requires acknowledging that the brain is shaping the environment, while being shaped by the environment (and genetic background) dynamically and across levels (Viding et al., Reference Viding, McCrory, Baskin-Sommers, DeBrito and Frick2023). This approach also requires modeling brain interactions with multiple levels of influence (while also modeling the brain as a complex, dynamic system), prompting the need for quantitative models that incorporate multiple levels in a transactional system that reaches from cells to brain circuits to symptoms.

Figure 4. Transactional Models.
Another intriguing approach to consider when studying developmental psychopathology using neuroscience, is that complex systems are probabilistic and often contain “tipping points” (Kauffman, Reference Kauffman1996; Moore, Reference Moore2018). These could be points where risk overwhelms protection, or where behavior goes from being dynamic to more stable. Some of this theory highlights potential canalization (Gottlieb, Reference Gottlieb1991) in which development will mostly proceed in a certain direction even with minor perturbations, unless there are major shifts to new defined directions. In this sense, we may see the brain as an organ designed to be responsive to the environment and culture more broadly (Frankenhuis et al., Reference Frankenhuis, Panchanathan and Nettle2016; Varnum & Kitayama, Reference Varnum and Kitayama2017), but there are certain tipping points when this plasticity may be lost or require a greater input to shift to a new developmental trajectory.
Risk is clustered
Work in developmental psychopathology has highlighted that risk is clustered within individuals. Risk is not evenly distributed across the population; rather, many youth exposed to one risk factor are also exposed to others. This clustering comes from an array of factors, but one of the most obvious involves systemic factors (e.g., structural racism, neighborhood inequality) that cluster some families spatially in areas where youth are exposed to a greater array of risks because of underinvestment in the area (which leads to under-resourced schools, low-quality housing, exposure to community violence, etc).
One prominent way developmental psychopathologists have addressed this clustering of risk involves cumulative risk models. These models posit that accumulation of risk (i.e., exposure to a greater number of risk factors) is more important than a single, specific risk factor alone (Sameroff et al., Reference Sameroff, Seifer, Zax and Barocas1987; Trentacosta et al., Reference Trentacosta, Hyde, Goodlett and Shaw2013). This work means that studying a single adversity in isolation is missing the point that youth who experience one adversity are also experiencing others.
Applications to developmental neuroscience
While developmental neuroscience is just starting to appreciate certain themes from developmental psychopathology, it has been leading the charge in describing clustered risk. Recent theories parse dimensions of adversity that may uniquely shape brain development (McLaughlin et al., Reference McLaughlin, Sheridan and Lambert2014), suggesting distinct pathways to psychopathology. Threat has been posited to influence affective brain systems, while deprivation has been posited to influence cognitive brain systems. These predictions have been supported by a systematic review (McLaughlin et al., Reference McLaughlin, Weissman and Bitrán2019) (though see Machlin et al., Reference Machlin, Egger, Stein, Navarro, Carpenter, Goel, Patel, Copeland and Sheridan2023). However, detractors of this theory also argue that adversity dimensions often overlap and are not as distinct as they seem (Smith & Pollak, Reference Smith and Pollak2021). For example, neglect is also likely to be experienced as threatening.
Building on this work, other dimensions of stress exposure have also been posited to uniquely influence brain development, such as unpredictability and controllability (Cohodes et al., Reference Cohodes, Kitt, Baskin-Sommers and Gee2021). These dimensional models are influential and specify how we can group cumulative risk into biologically relevant input that may uniquely influence child development (similar to “environtypes” described in RDoC). However, we need more research in this area and developmental neuroscience may benefit from considering the clustered and overlapping nature of positive environments and their effect on brain development. For example, an analysis in the ABCD study revealed links among positive ecologies (e.g., social support, perinatal wellbeing) and brain structure and cognition (Gonzalez et al., Reference Gonzalez, Palmer, Uban, Jernigan, Thompson and Sowell2020).
Finally, it is also critical to consider how risk can cluster within individuals due to person-context interactions that generate cascades. Specifically, youth with the highest genetic risk for psychopathology are more likely to live in environments with multiple risk factors due to gene-environment correlation (Jaffee & Price, Reference Jaffee and Price2007). For example, children inheriting genes that impact brain function and impulsivity are more likely to have parents with genes related to impulsivity, who may model this behavior, and who live in risky neighborhoods, placing children with the riskiest genetic loading in the riskiest environments (Baskin-Sommers et al., Reference Baskin-Sommers, Viding, Barber, Ruiz, Paskewitz and Hyde2024). The context is then likely to reinforce whatever underlying biological risk is present, leading to further developmental cascades, a challenge for interventions.
Person-centered approaches
The acknowledgement that dimensions of symptoms only capture population-level phenomena (while ignoring heterogeneity) has led to an additional focus in developmental psychopathology on ways of identifying subgroups of individuals who may share symptom profiles, developmental trajectories, or experiences of risk or resilience. One example of research using a person-centered approach has been studies on the development of antisocial behavior. Using group-based trajectory modeling, groups of youth have been identified with early onset versus late onset antisocial behavior. These approaches have helped to identify different etiologies for each group (Moffitt, Reference Moffitt2018), which has informed preventative interventions. These person-centered approaches have been strengthened by quantitative advances that allow for identifying clusters of individuals through a variety of methods focusing on data over time (e.g., group-based trajectory modeling) or across measures (e.g., latent profile analysis).
Applications to developmental neuroscience
Person-centered approaches in developmental neuroscience have been used to help define more homogeneous subgroups using data-driven approaches on neural data such as brain volume and functional connectivity time series. For example, Buthmann and colleagues (Reference Buthmann, Miller, Uy, Coury, Jo and Gotlib2023) used longitudinal k-means clustering with measures of hippocampal brain volume and emotional problems to identify groups of youth with distinct brain-behavior trajectories in the face of early life stress. Relatedly, subgrouping-group iterative multiple model estimation has been used to estimate distinct functional connectivity profiles that were associated with differing psychological symptom trajectories in adolescents (Hardi et al., Reference Hardi, Goetschius, McLoyd, Lopez‐Duran, Mitchell, Hyde, Beltz and Monk2023). Beyond applying these methods to neural data, researchers can use person-centered methods to identify subgroups of youth based on their symptoms or behavioral trajectories and then explore the neural correlates of these subgroups. For example, Hyde et al. (Reference Hyde, Shaw, Murray, Gard, Hariri and Forbes2016) identified early versus late starting groups of youth using longitudinal data on antisocial behavior and then examined whether amygdala reactivity to emotional faces differed between these person-centered, data defined groups.
Who is studied?
Samples and sampling
Developmental psychopathology work marked a shift away from case-control approaches popular in child psychiatry. This shift has led to more diversity in approaches with some researchers studying these issues in relatively healthy community samples, some in samples defined by a specific exposure (e.g., recruited from child protective services), and many in high-risk or enriched samples. Each type of sample brings strengths and weaknesses. High-risk samples have been particularly useful in developmental psychopathology since they can yield dimensional outcomes and can be sampled to be representative, while also having enough enrichment of an exposure or risk factor to yield substantial numbers of participants with clinically meaningful symptoms and outcomes.
Applications to developmental neuroscience
As developmental neuroscience is a relatively new field in which sample size can be challenging due to the costs of neuroimaging, most work in this field has been with convenience samples. More recently the field has begun to contend with two core issues in the area of sampling: power and generalizability. Marek et al. (Reference Marek, Tervo-Clemmens, Calabro, Montez, Kay, Hatoum, Donohue, Foran, Miller, Hendrickson, Malone, Kandala, Feczko, Miranda-Dominguez, Graham, Earl, Perrone, Cordova, Doyle and Dosenbach2022) examined multiple large neuroimaging data sets and concluded that brain-phenotype associations may be smaller than expected in past work, resulting in inflated and irreproducible associations. As a result, they called for future work with sample sizes in the thousands. However, the authors highlight some exceptions, noting that certain forms of imaging (e.g., functional vs. structural) and certain brain-behavior associations with larger effect sizes may be well powered in smaller samples. Approaches that increase power by increasing the reliability of neuroimaging and using approaches such as hold-out samples to test optimized model fit, or pursuing models that focus on within-person variation may also decrease sample size demands (e.g., Spisak et al., Reference Spisak, Bingel and Wager2023). Moreover, work highlighting the need for thousands of participants has not considered sampling itself – that is, enriched samples may provide more power by adding participants with more data on higher ends of psychopathology dimensions while representative samples may help avoid replication crises. Additionally, the quality of measurement will impact power in brain-behavior analyses, as very large studies inherently have shallower (and thus more noisy) measurement.
Beyond how many individuals are included, it is important to consider whether results generalize to the broader population (Falk et al., Reference Falk, Hyde, Mitchell, Faul, Gonzalez, Heitzeg, Keating, Langa, Martz, Maslowsky, Morrison, Noll, Patrick, Pfeffer, Reuter-Lorenz, Thomason, Davis-Kean, Monk and Schulenberg2013). Convenience samples, even very large ones, pose a challenge to generalizability. One example moving towards greater generalizability is the ABCD study, which has recruited youth from diverse geographic regions across 21 sites (DeJoseph et al., Reference DeJoseph, Herzberg, Sifre, Berry and Thomas2022). However, a study by Gard et al. (Reference Gard, C. and T.2023) found that discrepancies in SES emerged between the recruited ABCD sample and the sample of youth with usable neuroimaging data. This work highlights the need for weighting data, such as post-survey adjustments. This type of weighting have been applied to a non-probability neuroimaging sample, revealing that weighting (versus not) can dramatically influence the conclusions made with the data (LeWinn et al., Reference LeWinn, Sheridan, Keyes, Hamilton and McLaughlin2017). ABCD is an important example of both the promise and perils in this regard: ABCD offers a very large sample with a moderate depth of measurement, which helps to raise statistical power. Moreover, the sample is not fully probability based, but can be weighted for better generalization, though it still may have poor coverage of youth with high adversity exposure and/or high symptom levels (e.g., those with externalizing are underrepresented) (Cosgrove et al., Reference Cosgrove, McDermott, White, Mosconi, Thompson, Paulus, Cardenas-Iniguez and Aupperle2022).
One promising direction from developmental psychopathology has been the focus on recruiting high-risk samples (Sameroff, Reference Sameroff2000). Limited neuroimaging work has utilized large high-risk samples, though some notable examples exist: For example, the nation-wide Future Families and Child Wellbeing Study, which recruited ∼ 5,000 children and oversampled for children born to unwed mothers (Reichman et al., Reference Reichman, Teitler, Garfinkel and McLanahan2001) has an add-on study, the Study of Adolescent to Adult NeuroDevelopment, which engaged a subset of these families for neuroimaging, thus providing neuroimaging in a relatively disadvantaged, though population-based sample (Hein et al., Reference Hein, Goetschius, McLoyd, Brooks-Gunn, McLanahan, Mitchell, Lopez-Duran, Hyde and Monk2020; for another example see the Philadelphia Neurodevelopmental Cohort; Satterthwaite et al., Reference Satterthwaite, Connolly, Ruparel, Calkins, Jackson, Elliott, Roalf, Hopson, Prabhakaran, Behr, Qiu, Mentch, Chiavacci, Sleiman, Gur, Hakonarson and Gur2016).
Representation
Much of the early work in developmental psychopathology sought out youth and families who were exposed to greater levels of adversity, often through community partnerships (e.g., see Cicchetti and other’s work with Mount Hope Family Center). This approach diverged, not only from case-control designs in child psychiatry, but also from the focus on White and upper middle-class participants that dominated developmental psychology (McLoyd & Randolph, Reference McLoyd and Randolph1985). Thus, in some ways research in developmental psychopathology led to greater representation of youth and families with identities and experiences that had been underrepresented in psychiatry (though the field has not been free of problematic approaches nor has it represented all groups). At the same time, a critique of this approach is that though work in this area has better represented underrepresented identities (e.g., families of color, low-income families), it has often focused mostly on risk and negative outcomes, without enough attention to resilience and promotive factors (McLoyd & Randolph, Reference McLoyd and Randolph1985).
Applications to developmental neuroscience
Though a trend towards representativeness is important, it is also important to consider representation. There are likely multiple reasons for lack of representation, including the pragmatics of much neuroimaging research needing to be near larger universities and medical centers for access to scanning, as well as broader factors like the willingness of marginalized participants to engage in studies involving neuroimaging given the mistreatment of participants of color in biomedical research (Leve et al., Reference Leve, Kanamori, Humphreys, Jaffee, Nusslock, Oro and Hyde2024). However, we believe one barrier is also interest from the imaging community and ability to build ties to the community to engage in this work. Community-researcher partnerships, focus groups, and advisory boards are efforts needed for moving the field toward building community trust and finding more effective ways to represent people’s lived experiences in research (Gard, Mueller, et al., Reference Gard, C. and T.2023). Current examples of community-based participatory research have also highlighted the importance of outlining positionality and power dynamics in community-engaged developmental neuroscience (La Scala et al., Reference La Scala, Mullins, Firat and Michalska2023). Further, it is important for the field to diversify who is doing the research, as that will inevitably shift what questions are being asked as well (Roberts et al., Reference Roberts, Bareket-Shavit, Dollins, Goldie and Mortenson2020).
Culture
Scholars in the field have offered important perspectives on how developmental psychopathology has, can, and should integrate culture more into theory and empirical studies of the development of psychopathology (Causadias, Reference Causadias2013; García Coll et al., Reference García Coll, Akerman and Cicchetti2000). These critiques have articulated that, though an examination of multiple levels of analysis has been an important hallmark of the field, the culture level often has received less attention than other levels (e.g., the family or biological levels) (Causadias, Reference Causadias2013). Often research in this area has examined culture in non-developmental terms, as if it is a fixed property of an individual or community or something only minorities or foreigners possess (Causadias, Reference Causadias2013). The issue is that “culture” is many things from materials to ideas to community practices, and impacts development at every stage and through multiple contexts (Causadias, Reference Causadias2013).
Applications to developmental neuroscience
Despite decades of research demonstrating that the environment a child is exposed to can “get under the skin” by influencing brain development, developmental neuroscience has largely focused on proximal environments (parenting, family income). Recent work is increasingly considering macro-level influences on brain development, such as neighborhood exposures (Hyde et al., Reference Hyde, Gard, Tomlinson, Burt, Mitchell and Monk2020). Nonetheless, the interplay among culture, brain development, and mental health is only beginning to be considered, with recent calls for greater attention to “developmental cultural neuroscience” (Qu et al., Reference Qu, Jorgensen and Telzer2021). As examples in this area, cultural influences have been examined with respect to family values. In one study, while Latinx youth displayed greater reward-related neural activity when donating money to their family, White youth displayed greater reward-related neural activity when retaining money for themselves (Telzer et al., Reference Telzer, Masten, Berkman, Lieberman and Fuligni2010). Beyond cross-group comparisons, in the same study youth who identified with their family to a greater extent exhibited greater reward-related neural activity when contributing money to their family. These findings highlight the type of work that is needed to probe how culture shapes neurobiological and behavioral processes relevant for psychopathology.
Resilience
The fields of developmental psychopathology and resilience have always been tightly intertwined (Cicchetti & Toth, Reference Cicchetti and Toth2009; Masten et al., Reference Masten, Lucke, Nelson and Stallworthy2021) and highlight that focusing only on risk without identifying protective and promotive factors misses the boat in terms of understanding why so many youth exposed to adversity have positive outcomes, as well as how that information can inform interventions for those facing adversity (Masten et al., Reference Masten, Lucke, Nelson and Stallworthy2021). Moreover, a strengths-based approach is critical in identifying the many wonderful things that parents, families, communities, and cultures are doing well to support development even in the face of adversity and structural inequality (Zimmerman, Reference Zimmerman2013).
Applications to developmental neuroscience
Applying developmental neuroscience to the study of resilience is important but highlights the complexity of studying resilience. Across the evolution of resilience research, resilience was primarily defined as a manifested outcome (Masten et al., Reference Masten, Lucke, Nelson and Stallworthy2021), a definition lending itself to identifying neural correlates of those who are “resilient” (versus not). Other definitions include individuals who do not develop psychopathology (e.g., PTSD) even when exposed to risk (e.g., trauma), which leads to studying the neural correlates of those who do not show psychopathology; a focus of most neuroimaging research related to resilience (e.g., Zhang et al., Reference Zhang, Rakesh, Cropley and Whittle2023).
However, more recent accounts identify that resilience may exist in multiple domains of functioning (e.g., youth may show resilience socioemotionally, but not academically; Miller-Graff, Reference Miller-Graff2022), suggesting that research is needed on the neural correlates of multiple dimensions of resilience (Bezek et al., Reference Bezek, Tillem, Suarez, Burt, Vazquez, Michael, Sripada, Klump and Hydein press; Burt et al., Reference Burt, Whelan, Conrod, Banaschewski, Barker, Bokde, Bromberg, Büchel, Fauth‐Bühler, Flor, Galinowski, Gallinat, Gowland, Heinz, Ittermann, Mann, Nees, Papadopoulos‐Orfanos, Paus and Garavan2016). In parellel, there is growing recognition of resilience as a process (i.e., generative resilience), which considers the unique set of resources an individual accesses (e.g., support across the family, community, etc.) and/or actions an individual or community takes (e.g., coping, community involvement) that support adaptive functioning (Miller-Graff, Reference Miller-Graff2022). These process models push forward the question of how the developing brain may contribute not only to youth’s behavioral outcomes, but also to their multisystemic interactions supporting resilience as a process. For example, we may examine how protective factors buffer the impacts of adversity on the brain or moderate brain-behavior relations. Regardless of the model, one major challenge is to understand whether the brain correlates being measured are an outcome, producer, or byproduct of resilience. Further, more work should explore longitudinal trajectories of resilience (defined as a multidimensional outcome and a process) and integrate brain structure and function into transactional models of resilience.
Genetically informed and causal designs
While not an explicit thrust of developmental psychopathology, many scholars in the field have emphasized the complex interplay of genes and the environment and published on these approaches in the Journal. Recent special issues of the Development and Psychopathology have covered related topics, such as genetically informed designs to understand familial transmission of psychopathology (Volume 34, Issue 5, 2022), epigenetics (Volume 28, Issue 4, 2016), and genetic moderation of intervention efficacy (Volume 27, Issue 1, 205). Emerging work across these areas is exploring how polygenic scores shape the development of psychopathology (Speyer et al., Reference Speyer, Neaves, Hall, Hemani, Lombardo, Murray, Auyeung and Luciano2022) or how epigenetic processes are influenced by experience and modulate expressions of psychopathology (Curley & Champagne, Reference Curley and Champagne2016). Finally, this work is also highlighting the potential for gene-environment correlation and the need for genetically informed and/or causal models, such as (quasi) experiments (e.g., Hyde & Dotterer, Reference Hyde and Dotterer2022). Though much work in developmental psychopathology is observational, complimentary work addressing gene-environment correlation via genetically informed designs has identified environments that are having true “environmental” influences on outcomes (e.g., Burt, Reference Burt2022).
Applications to developmental neuroscience
Neurogenetic studies are increasingly probing the dynamic interplay among context, genes, and neurobiology (Hyde, Reference Hyde2015). Molecular genetic studies have linked psychopathology-related polygenic scores to neural correlates in youth (Fernandez-Cabello et al., Reference Fernandez-Cabello, Alnæs, van der Meer, Dahl, Holm, Kjelkenes, Maximov, Norbom, Pedersen, Voldsbekk, Andreassen and Westlye2022), while epigenetic factors have been linked to brain structure and function in youth (Wheater et al., Reference Wheater, Stoye, Cox, Wardlaw, Drake, Bastin and Boardman2020) and may offer a mechanism relating adversity to brain outcomes (e.g., Wrigglesworth et al., Reference Wrigglesworth, Ryan, Vijayakumar and Whittle2019). However, the role of development has rarely been considered in molecular and epigenetic studies (Hyde, Reference Hyde2015).
In addition, twin/family designs are beginning to investigate which aspects of brain development, and its association with behavior, are genetic versus environmental in origin (Brouwer et al., Reference Brouwer, Schutte, Janssen, Boomsma, Hulshoff Pol and Schnack2021; Wallace et al., Reference Wallace, Lee, Prom-Wormley, Medland, Lenroot, Clasen, Schmitt, Neale and Giedd2010).Twin/family designs are promising because most studies relating environmental risk to neural outcomes have been purely observational, and thus adversity-brain associations could reflect gene-environment correlations. Behavior genetic designs can test whether the association between an experience and brain outcome is indeed environmental in origin (e.g., de Manzano & Ullén, Reference de Manzano and Ullén2018). At the same time, some experiences under study (e.g., household/neighborhood poverty) do not differ between twins and thus may benefit from other causal designs including experiments and quasi-experiments. For example, the baby’s first years study is using cash transfers to test whether changes in family income may have causal effects on the developing brain (Noble et al., Reference Noble, Magnuson, Gennetian, Duncan, Yoshikawa, Fox and Halpern-Meekin2021), which has clear policy and intervention implications.
Innovations in developmental neuroscience and how they can inform developmental psychopathology
Though much of our goal has been to highlight how developmental psychopathology can inform the emerging field of developmental neuroscience, developmental neuroscience also has much to offer developmental psychopathology. Developmental neuroscience offers the obvious addition of increasingly advanced conceptualizations and measurement of the brain as it fits into developmental psychopathology models. Beyond simply the addition of “the brain,” there are multiple approaches in developmental neuroscience that are important directions for developmental psychopathology:
A hallmark of developmental neuroscience is the ability to leverage animal models to inform how environmental experiences that can be manipulated in animals, but not ethically in humans, influence brain and behavior in youth. For example, neuroscientists have experimentally manipulated caregiving behaviors in rodent models to characterize how adversity modulates brain and emotional development (Callaghan & Tottenham, Reference Callaghan and Tottenham2016). Moreover, since sensitive periods are tied to underlying brain systems and genetic underpinnings, developmental neuroscience can use experimental animal models to interrogate timing-dependent effects of experience on development (Knudsen, Reference Knudsen2004). Further, developmental neuroscience can characterize computational neural mechanisms of behavior that are harder to uncover in behavioral studies. For example, reduced segregation between cognitive control and default mode networks is linked to worse cognitive performance in high-SES, but better cognitive performance in low-SES, youth, suggesting that different individuals may apply unique neurobehavioral strategies for cognition – a finding that can help tailor intervention programs (Ellwood-Lowe et al., Reference Ellwood-Lowe, Whitfield-Gabrieli and Bunge2021).
Despite these advances, developmental neuroscience contends with unique challenges. First, MRI research is costly and located where magnets (and biophysics support) exist, which influences who can participate and who is trained in neuroimaging. Second, biomedical research has disproportionately excluded and maltreated underrepresented communities (Leve et al., Reference Leve, Kanamori, Humphreys, Jaffee, Nusslock, Oro and Hyde2024), which requires thoughtful action to build community engagement and trust in future research (a time-consuming and challenging process that takes novel training and knowledge). Third, neuroimaging is a relatively young field with frequent changes to methods (e.g., scan sequences, analytic pipelines), which can be difficult to address in longitudinal studies. Fourth, not everyone can be scanned or continue to be scanned (e.g., braces, bullet fragments), which impacts representation. Finally, the logistics of neuroimaging (time, cost) introduce barriers to scanning on short (and long) timescales, despite its utility for capturing dynamic brain-experience-psychopathology associations.
Future directions in a neuroscience-informed developmental psychopathology: policy and treatment
The dialog between developmental psychopathology and developmental neuroscience has motivated policy changes to facilitate wellbeing in youth: First, work documenting the pernicious effects of poverty on brain development (Johnson et al., Reference Johnson, Riis and Noble2016), and the neurobiological effects of interventions that reduce poverty (Noble et al., Reference Noble, Magnuson, Gennetian, Duncan, Yoshikawa, Fox and Halpern-Meekin2021), can motivate changes in systemic inequities that concentrate disadvantage to marginalized communities. Second, as adversity powerfully influences stress neurobiology, developmental neuroscientists have provided recommendations for migration-related policy, such as ending deportation and supporting migrant children (Kribakaran et al., Reference Kribakaran, Cohodes and Gee2023). Third, the neurobiological sequelae of parent-child separation and social isolation have been used to advocate for eliminating money bail and solitary confinement in the juvenile justice system, placing “healthy development as a human right” (Casey et al., Reference Casey, Taylor-Thompson, Rubien-Thomas, Robbins and Baskin-Sommers2020). Finally, the slow pace of brain development has been used to advocate for banning juvenile transfer to adult courts (Casey et al., Reference Casey, Taylor-Thompson, Rubien-Thomas, Robbins and Baskin-Sommers2020), and was used by the US Supreme Court to limit capital punishment or life without parole in adolescents convicted of serious crimes (Steinberg, Reference Steinberg2013). These examples demonstrate how the combination of these fields can have, and is having, important impacts on the lives of youth and families.
The integration of developmental neuroscience with developmental psychopathology is also a powerful future direction for informing prevention and treatment. From a basic science perspective, neuroimaging offers a tool for focusing in on the brain mechanisms potentially underlying the “active ingredients” of therapy approaches. For example, developmental neuroscience has helped to identify the neural mechanisms underlying the effectiveness of an early parenting intervention (e.g., Valadez et al., Reference Valadez, Tottenham, Tabachnick and Dozier2020). In doing so, developmental neuroscience can contribute a sophisticated understanding of the brain systems underlying memory, cognition, and executive functioning, which may help to delineate the building blocks underlying symptom remission in various interventions (e.g., Becker et al., Reference Becker, Beltz, Himle, Abelson, Block, Taylor and Fitzgerald2023).
In addition to informing behavioral interventions, developmental neuroscience is poised to make unique contributions to brain-based interventions as well. Growing evidence supports the efficacy of repetitive transcranial magnetic stimulation as a relatively noninvasive treatment for a variety of psychopathologies (Bejenaru & Malhi, Reference Bejenaru and Malhi2022). Though more invasive, deep brain stimulation has also shown efficacy for reducing treatment-resistant self-injurious behaviors in autism spectrum disorders (Razmkon et al., Reference Razmkon, Maghsoodzadeh and Abdollahifard2022). Lastly, EEG theta/beta ratio neurofeedback training has been studied as an intervention for ADHD (Enriquez-Geppert et al., Reference Enriquez-Geppert, Smit, Pimenta and Arns2019). Though there have been critiques of neurofeedback training (McGough, Reference McGough2022), brain-based interventions remain a notable goal for mobilizing neuroscience advances in the treatment clinic.
Conclusions
The field of developmental psychopathology has come a long way since the inception of the journal Development and Psychopathology. The field of developmental neuroscience is now emerging with new tools and new models for development. Through a greater integration, models from developmental psychopathology can strengthen developmental neuroscience and developmental neuroscience can better inform the role of the brain in developmental psychopathology models. In general, though there are certainly perils, with a more community-based approach, developmental neuroscience, informed by developmental psychopathology, is poised to contribute to progress in our understanding of development and psychopathology, as well as its translation to policy, prevention, and intervention (Leve et al., Reference Leve, Kanamori, Humphreys, Jaffee, Nusslock, Oro and Hyde2024).
Acknowledgements
We appreciate the opportunity to contribute to the final issue of Developmental Psychopathology edited by Dante Cicchetti. Dante has been an incredible inspiration, mentor, and friend to so many, including the first author (LWH). We are grateful for his impact on the field and on our work. Thank you, Dante!
Author contribution
Jessica L. Bezek and Cleanthis Michael contributed equally to this manuscript.
Funding statement
None.
Competing interests
None.