The DCE Webinar series is a programme of monthly virtual events about data-centric engineering: the use of data science methods and models for improving the reliability, resilience, safety, efficiency and usability of engineered systems. It is hosted by the Data-Centric Engineering journal (cambridge.org/dce) at Cambridge University Press – a peer-reviewed open access journal dedicated to the interface of data science and all engineering disciplines – with the support of The Alan Turing Institute and the Lloyd's Register Foundation.
The series includes talks from leading figures in academia, and those applying data-driven approaches in industry and other settings, as well as networking events for early career researchers and others new to this emerging field.
NEW SEASON! Upcoming DCE Webinars
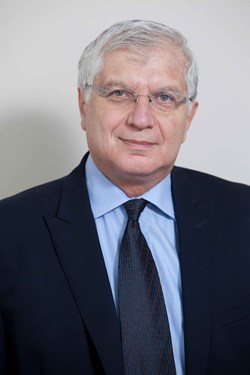
Speaker: Professor Ron Kenett, Senior Research Fellow at the Samuel Neaman Institute, Technion, Haifa, Israel, Chairman of the KPA Group, Israel, Chairman of the Data Science Society at AEAI and Research Professor at the University of Turin, Italy.
Google Scholar
Staff page
Register for the webinar
Date/Time: Wednesday, 6th December 2023, 0800 PST / 1300 EST / 1600 GMT
Title: Engineering, emulators, digital twins and performance engineering
Abstract:New developments in sensor technologies, big data and advanced analytics affect modern system engineering. In this webinar we discuss changes at a conceptual level, from nominal engineering, aiming at design optimisation, to performance engineering, aiming at adaptable monitoring diagnostic, prognostic, and prescriptive capabilities. We present the methodology of stochastic emulators using case studies and show how emulators can be used to complement finite element and computational fluid dynamic computational models, in digital twin frameworks.
Speaker biography: Professor Ron Kenett is Senior Research Fellow at the Samuel Neaman Institute, Technion, Haifa, Israel, Chairman of the KPA Group, Israel, Chairman of the Data Science Society at AEAI and Research Professor at the University of Turin, Italy. He is an applied statistician combining expertise in academic, consulting and business domains. Ron is member of the Public Advisory Council for Statistics Israel, member of the of Digital Twin Center of Excellence (DiTCE) at Ben Gurion University of the Negev, member of the INFORMS QSR advisory board and member of the advisory board of DSRC, the University of Haifa Data Science Research Center. He is Past President of the Israel Statistical Association (ISA) and of the European Network for Business and Industrial Statistics (ENBIS). He authored and co-authored over 250 papers and 18 books on data science, industrial statistics, biostatistics, healthcare, customer surveys, multivariate quality control, risk management, system and software testing, and information quality. The KPA Group, he founded in 1994, is a leading Israeli firm focused on generating insights through analytics. He was awarded the 2013 Greenfield Medal by the Royal Statistical Society, in 2018, the George Box Medal by the European Network for Business and Industrial Statistics and, in 2022, the life achievement award by the Israeli Society for Quality. He earned a BSc in Mathematics (with first class honors) from Imperial College, London University and a PhD in Mathematics from the Weizmann Institute of Science, Rehovot, Israel.
Past Webinars and Recordings
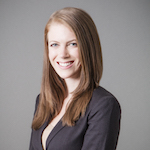
Speaker: Dr. Kelly Twomey Sanders, Sonny Astani Department of Civil and Environmental Engineering, University of Southern California.
Google Scholar
Staff page
Watch the recording here
Date/Time: Wednesday, 8th November 2023, 0800 PST / 1300 EST / 1600 GMT
Title: The Air-Conditioning Paradox: Protecting Vulnerable Populations from Extreme Heat
Abstract: Over 50% of people worldwide live in urban areas, and by 2050 this fraction is expected to increase to 70%. Meanwhile, extreme heat events are increasing in frequency and intensity, posing a human health risk for urbanites. As extreme heat is the leading cause of weather-related fatalities, research that helps identify the populations that are most vulnerable to extreme heat is urgently needed. While access to air conditioning (AC) is one of the most important adaptive technologies to protect people from heat, AC usage requires vast amounts of electricity that can exacerbate warming, both locally and globally, creating a policy paradox. We also have a very limited understanding of trends in AC access and usage, making it difficult to reach the most populations most vulnerable to extreme heat. This presentation will describe a body of work dedicated to building a more quantitative understanding of heat vulnerabilities across the Southern California region via novel methods of estimating AC penetration rates and anthropogenic heat.
Speaker biography: Dr. Kelly Twomey Sanders is an Associate Professor in the University of Southern California’s Sonny Astani Department of Civil and Environmental Engineering. Her research aims to ease tensions between human and natural systems, with particular emphasis on reducing the environmental impacts of providing energy and water, analyzing tensions between climate change adaptation and mitigation strategies, and anticipating the effects of climate change on energy systems. Sanders has been recognized in Forbes’ 30 under 30: Today’s disruptors and tomorrow’s brightest stars and MIT Technology Review’s 35 Innovators Under 35 for her contributions to the energy field. Her research and commentary have been featured in media outlets such as The New York Times, The Los Angeles Times, The Washington Post, Foreign Affairs, WIRED magazine, Forbes, Bloomberg, The Wall Street Journal and Scientific American. Sanders received her B.S. in Bioengineering from the Pennsylvania State University, as well M.S.E and Ph.D. degrees in Mechanical Engineering and Environmental Engineering from the University of Texas at Austin, respectively. She teaches classes related to energy and the environment.
Speaker: Markus Kraft, Professor of Chemical Engineering at the University of Cambridge and Director of CARES (Cambridge Centre for Advanced Research and Education in Singapore).
Date/Time: Wednesday, 11th October 2023, 0800 EDT / 1300 BST / 2200 SGT
Title: Augmenting reality with The World Avatar - automating data centric engineering
Abstract: The advent of digitalization brings forth new opportunities for data-centric engineering, leading to increased efficiency in the realms of science, engineering, and society. However, the implications of digitalization for environmental and social sustainability remain uncertain. We suggest that knowledge graph technology plays a pivotal role in addressing this challenge. In this presentation, I will introduce "The World Avatar," a dynamic knowledge graph (dKG) designed to construct a comprehensive digital replica of the world at large. I will elucidate the core concepts and principles underpinning this initiative, showcasing how it can facilitate seamless integration among various data formats and software systems, ultimately enabling automated data-centric engineering. Additionally, I will present a variety of use cases that demonstrate how The World Avatar can support cross-domain applications spanning diverse scales, from the molecular level to the global stage. These applications aim to enhance efficiency, reduce costs, diminish carbon emissions, and bolster resilience.
Speaker biography: Professor Kraft researches data-driven modelling, machine learning, and semantic web technologies and has pioneered the World Avatar – an all-encompassing knowledge graph approach to developing a dynamic world model. He co-edited the book "Intelligent Decarbonisation" which comprehensively assesses the current and future impact of digital technologies and artificial intelligence on the decarbonisation of key economic sectors. He is a Fellow of Churchill College and a Professor in the Department of Chemical Engineering and Biotechnology at the University of Cambridge. He also is the Director of the Cambridge Centre for Advanced Research and Education in Singapore (CARES), the University of Cambridge's first overseas research centre, based at CREATE
Speaker: Steve Brunton, James B. Morrison Endowed Career Development Professor in Mechanical Engineering, University of Washington
Date/Time: Wednesday, 7th December 2022, 1000 CDT / 1100 EDT / 1600 BST
Title: Machine Learning for Scientific Discovery
Speaker: Professor Ricardo Vinuesa, Department of Engineering Mechanics, KTH Royal Institute of Technology, Stockholm, Sweden
Date/Time: Wednesday, 2nd November 2022, 1000 CDT / 1100 EDT / 1600 BST
Title: Modelling and Controlling Turbulent Flows through Deep Learning
Abstract: The advent of new powerful deep neural networks (DNNs) has fostered their application in a wide range of research areas, including more recently in fluid mechanics. In this presentation, we will cover some of the fundamentals of deep learning applied to computational fluid dynamics (CFD). Furthermore, we explore the capabilities of DNNs to perform various predictions in turbulent flows: we will use convolutional neural networks (CNNs) for non-intrusive sensing, i.e. to predict the flow in a turbulent open channel based on quantities measured at the wall. We show that it is possible to obtain very good flow predictions, outperforming traditional linear models, and we showcase the potential of transfer learning between friction Reynolds numbers of 180 and 550. We also discuss other modelling methods based on autoencoders (AEs) and generative adversarial networks (GANs), and we present results of deep-reinforcement-learning-based flow control.

Speaker: Professor Stéphane Bordas, Professor of Computational Mechanics (Legato Team), Department of Engineering, University of Luxembourg
Google Scholar
Legato Team page
Twitter
Date/Time: Wednesday, 5th October 2022, 1000 CDT / 1100 EDT / 1600 BST
Title: Digital twins in mechanics and medicine
Abstract: We review recent advances in the development of digital twins for mechanics and medicine and discuss challenges ahead. We provide applications to cancer treatment and prognosis, surgical simulation, chemical vapour deposition and other engineering processes and systems.
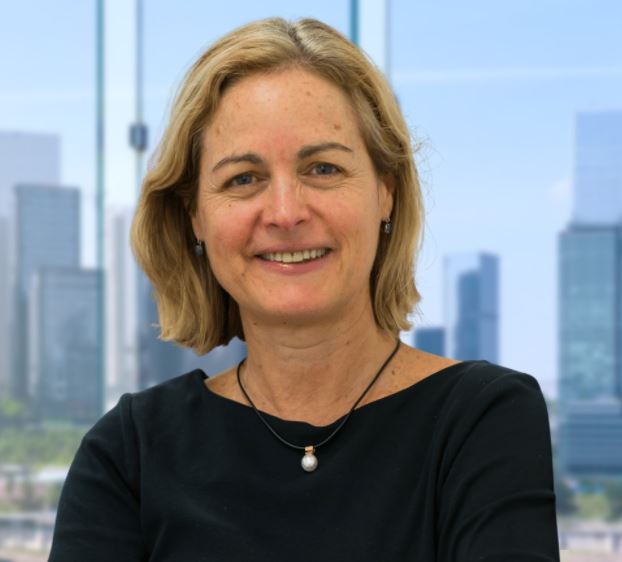
Speaker: Professor Melinda Hodkiewicz, BHP Billiton Fellow for Engineering for Remote Operations at The University of Western Australia
Date/Time: Wednesday, 7th September 2022, 0700 EDT / 1200 BST / 1900 AWST
Title: Building a Data Fit Organisation
Abstract: Evidence of use of AI-models to inform and drive day-to-day decisions about industrial assets is sparse despite significant investment in platforms, processes and developing models. While employees understand their roles in safety, cost and production, expectations for their behaviours and responsibilities towards the data they generate, manipulate and use are not clear.
This talk describes the Data Fit approach for industries involved in implementing AI to support decisions by engineers, scientists and technicians. The novelties of the Data Fit framework are a) data workflows, b) roles and behaviours for all involved (lead, enabler, consumer, composer, custodian and creator), and c) an explicit need for feedback loops. Development involved the observation of over 100 data work flows. Technical subject matter experts and their managers report that the Data Fit framework provides clarity about expectations for behaviours of all involved in the data workflows. This leads to improve process quality and enables more consistent delivery of business value through data.
Presenter: Professor Melinda Hodkiewicz is an academic working on multi-disciplinary projects to improve maintenance, asset management and safety practices though data, statistics and AI. Her early career was in operations and maintenance roles in the resources industry. Over the last decade she has been a keen observer of the progress, or lack there of, of embedding AI data workflows that routinely inform decision making about asset performance in heavy industry.
Presentation co-author: Zane Prickett is a Founder of Unearthed a large global community of innovators and SMEs solving challenges in the resources sector and CORE Skills developing skills pathway for technical experts in the resource industry. He has extensive operational experience in the international Oil & Gas industry.
About the Data Fit Organisation program
The DFO program is a collaboration between groups including Australian Government and industry co-funded Centre for Transforming Maintenance through Data Science, CORE Skills, a training organisation, and organisational psychologists at the Curtin University Future of Work Institute.

Speaker: Professor Nils Thürey, Technical University of Munich, Germany
Google Scholar
Thürey group webpage
Thürey Group on Twitter
Date/Time: Wednesday, 6th July 2022, 1000 CDT / 1100 EDT / 1600 BST
Title: Differentiable Physics Simulations for Deep Learning
Abstract: This talk focuses on the possibilities that arise from recent advances in the area of deep learning for physical simulations. In particular, it will focus on differentiable physics solvers from the larger field of differentiable programming. These solvers provide crucial information for deep learning tasks in the form of gradients, which are especially important for time-dependent processes. Also, existing numerical methods for efficient solvers can be leveraged within learning tasks. This paves the way for hybrid solvers in which traditional methods work alongside pre-trained neural network components. The resulting improvements will be illustrated with examples such as wake flows and turbulence mixing layer cases. From a machine learning perspective, regression problems with physics solvers are a highly interesting class of problems. I will conclude the talk by outlining avenues for custom learning algorithms that leverage the information from the solvers at training time.
Speaker: Professor Eleni Chatzi, Chair of Structural Mechanics and Monitoring at the Institute of Structural Engineering of the Department of Civil, Environmental and Geomatic Engineering, ETH Zürich, Switzerland
Date/Time: Wednesday 4th May 2022, 1000 CDT / 1100 EDT / 1600 BST
Title: Robust Dynamical Systems Monitoring: Learning by Modeling
Speaker: Professor Barry L. Nelson, Walter P. Murphy Professor, Department of Industrial Engineering and Management Sciences, Northwestern University, USA
Date/Time: Wednesday April 6th, 1000 CDT / 1100 EDT / 1600 BST
Title: Discrete-decision-variable Simulation Optimization in Operational Research
Abstract: “Simulation optimization” (occasionally “optimization via simulation”) is the term that operational researchers use to describe optimizing the output performance measures of a dynamic stochastic simulation with respect to some controllable design or decision variables, sometimes subject to stochastic constraints. Often the decision variables are discrete-valued, such as the number of agents to assign to each hour of the day in a call center, or the reorder points for various products in a supply chain. Discrete decision variables present a particular challenge for simulation optimization, and how the problem is attacked depends greatly on the number of feasible solutions, dimension of the decision variables, and computational cost of running the simulation.
In this talk I will describe extremes: simulating EVERY feasible solution in a statistically and computationally efficient way when the simulations are relatively cheap; and simulating a very small fraction of the feasible solutions strategically when the simulation is computationally expensive. The former draws on ideas from ranking & selection, while the latter is based on Bayesian optimization, but in each case with a new twist driven by the number of feasible solutions being large.
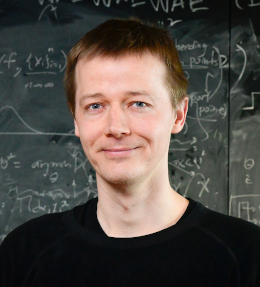
Speaker: Philipp Hennig, Chair for the Methods of Machine Learning at the Eberhard Karl University of Tübingen; Adjunct Scientist at the Max Planck Institute for Intelligent Systems, Tübingen, Germany.
Date/Time: Wednesday, 1st December 2021, 2pm UK / 9am Eastern US / 11pm Japan
Title: Probabilistic Numerics — Computation as Machine Learning
Watch the recording of this webinar
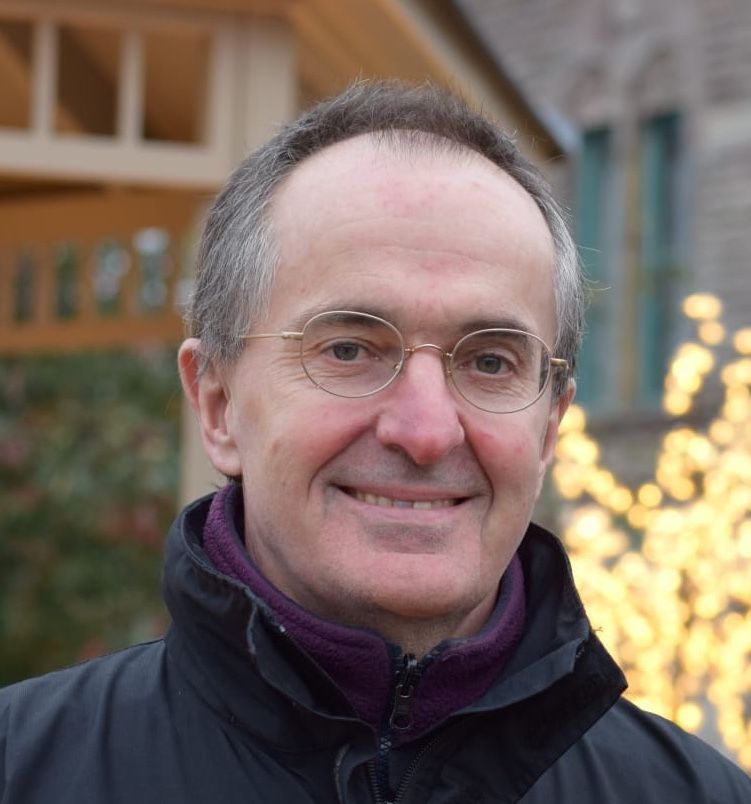
Speaker: Philip Jonathan, Statistical Modeller at Shell and Chair in Environmental Statistics and Data Science at Lancaster University, UK.
Date/Time: Wednesday, November 17th, 4pm UK
Title: Environmental decision support: rare events, monitoring and inversion, and uncertainty quantification
Watch the recording of this webinar
Abstract: Environmental data science offers the opportunity to combine theory and measurement in characterising our natural environment rationally and quantitatively, to understand its evolution, and to take decisions regarding our interactions with it wisely in the presence of uncertainty. The practical challenge is to develop and adapt ideas from statistical theory and method to address complex problems in the natural environment in a physically realistic manner, creating useful tools to quantify risk, and support real-world decision making.
Characterising rare events from observations is problematic by definition, since their rate of occurrence is low. In an environmental context, we discuss how extreme value theory is used to understand extreme ocean storms, floods, heat-waves and earthquakes. Practical application of extreme value methods numerous significant challenges, including the estimation of extreme value threshold, non-stationarity with respect to multiple covariates, and reliable characterisation of extremal dependence. These challenges become ever more acute as the numbers of responses and covariates increase. Given observational data for thousands of variables of interest in some applications, the computational complexity of extreme value analysis is enormous.
Remote sensing technologies including satellite, airborne, line-of-site and point) provide observations of the earth’s atmosphere and surface at different scales and resolutions. We discuss how these data sources are used in statistical models to detect, locate and quantify sources of natural and manmade methane and carbon dioxide emissions into the atmosphere, and to monitor atmospheric concentrations within specific domains.
Bayesian inference provides a natural framework for learning about large-scale physical systems, to quantify uncertainty in predictions regarding those systems, and to facilitate optimal decision-making. We discuss how the computational complexity of practical inference is managed by the adoption of suitable modelling frameworks and efficient numerical schemes.
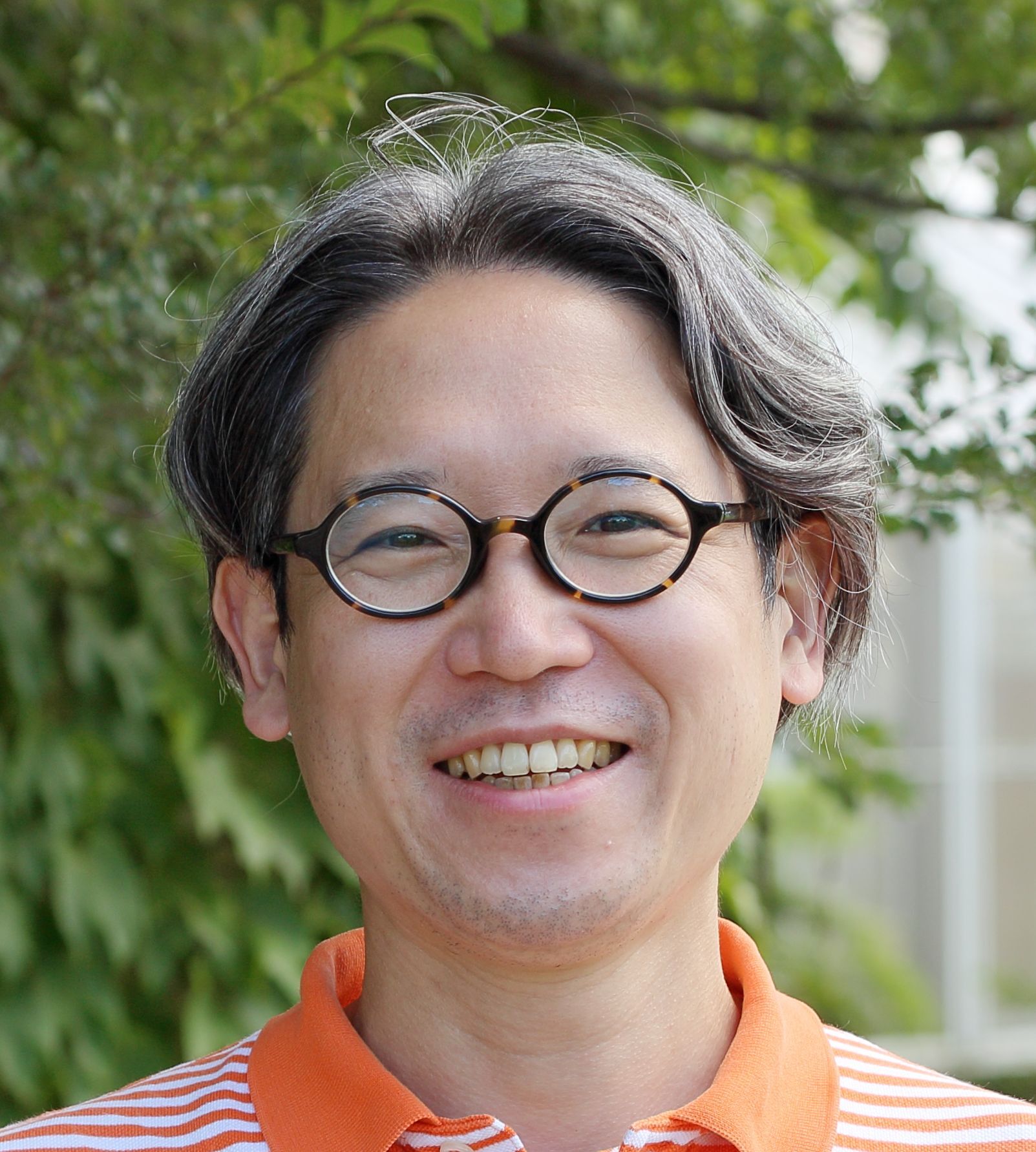
Speaker: Takemasa Miyoshi, Team Leader of the Data Assimilation Research Team at the RIKEN Center for Computational Science; Deputy Director of the RIKEN Interdisciplinary Theoretical and Mathematical Sciences Program, Wakō, Saitama, Japan
Date/Time: Wednesday 3rd November 2021, 9.45 - 11.00 am UK / 6.45pm - 8pm Japan
Title: Fusing Big Data and Big Computation in Numerical Weather Prediction
Watch the recording of this webinar
Abstract: At RIKEN, we have been exploring a fusion of big data and big computation, and now with AI techniques and machine learning (ML). The new Japan’s flagship supercomputer “Fugaku” is designed to be efficient for both double-precision big simulations and reduced-precision ML applications, aiming to play a pivotal role in creating super-smart “Society 5.0.” Our group in RIKEN has been pushing the limits of numerical weather prediction (NWP) through two orders of magnitude bigger computations using the previous Japan’s flagship “K computer”. The efforts include 100-m mesh, 30-second update “Big Data Assimilation” (BDA) fully exploiting the big data from a novel Phased Array Weather Radar. We have achieved the first-ever real-time BDA application with 500-m mesh NWP in the summer of 2020 using a supercomputer Oakforest-PACS of the University of Tokyo and Tsukuba University. In 2021, we used the new Fugaku and performed real-time 30-second update NWP during the periods of Tokyo Olympic and Paralympic games. With Fugaku, we have been exploring ideas for fusing BDA and AI. The data produced by NWP models become bigger and moving around the data to other computers for ML may not be feasible. Having a next-generation computer like Fugaku, good for both big NWP computation and ML, may bring a breakthrough toward creating a new methodology of fusing data-driven (inductive) and process-driven (deductive) approaches in meteorology. This presentation will introduce the most recent results from data assimilation and NWP experiments, followed by perspectives toward a general theory of prediction and control beyond meteorology.
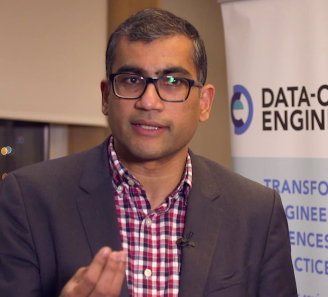
Speaker: Karthik Duraisamy, Associate Director for the Michigan Institute for Computational Discovery & Engineering, USA
Date/Time: Wednesday 6th October 2021, 4pm BST/11am EDT/12am JST
Title: Data-driven Reduced Order Models for Multi-scale, Multi-physics Systems
Watch the recording of this webinar
Abstract: This talk presents advances towards the development of effective reduced order models (ROMs) for complex multi-scale multi-physics problems. As a representative application, we consider combustion dynamics in a rocket engine, which is characterized by the coupling between heat release, hydrodynamics and acoustics. The first part of the talk is focused on improving robustness and consistency: A structure-preserving transformation of the state variables is used along with a discretely consistent least squares formulation to yield symmetrized model operators in both explicit and implicit time integration settings. The resulting reduced order model is well-conditioned and globally stable. Local stability is promoted via limiters that enforce physical realizability. The second part of the talk is focused on improving accuracy: Dimension reduction approaches based on static linear manifolds are not effective in addressing multi-scale problems with significant convection. To help mitigate this issue, we present formulations using adaptive spaces. Opportunities for further improvement are also highlighted. The last part of the talk discusses tractability : ROMs are used to enable computations of problems for which full order models are not affordable. In particular, we develop a multi-fidelity framework in which component-level ROMs are trained on small domains, and integrated to enable full-system predictions in an affordable manner. In essence, geometry-specific training is replaced by the response generated by perturbing the characteristics at the boundary of the truncated domain. This training method is shown to enhance predictive capabilities and robustness of the resulting ROMs, including conditions outside the training range.
Speaker: Julie McCann, Professor of Computer Systems and Head of the Adaptive Emergent Systems Engineering (AESE) Group, Imperial College London, UK
Date/Time: Wednesday 8th September 2021, 4pm BST/11am EDT/12am JST
Title: Does a Digital Twin have a Digital Twin?
Watch the recording of this webinar
Abstract: Since the early days of what is known today as the Internet of Things, there have been over twenty years of research. Such systems are key sources of the data that feed Digital Twins; indeed this might even be in real-time. However, when one talks to real users in the worlds of civil engineering, environment modelling, digital agriculture and the industrial IoT etc. they complain about IoT systems being flaky and completely unusable after a few years. Given that these users are designing infrastructures that are required to last potentially 10 to hundreds of years what are the implications for the field of IoT if we cannot deliver? In my talk I will discuss some of the issues we have been dealing with and put forward some of my thoughts of what we need to start looking at, as a community. Real users of networked sensor systems want smart infrastructures, my question is, will Digital Twin driven systems thinking be the answer?
Speaker: Neil Lawrence, DeepMind Professor of Machine Learning at the University of Cambridge, UK
Date/Time: Wednesday 5th May 2021, 4pm BST/11am EDT/12am JST
Title: Machine Learning and the Physical World
Watch the recording of this webinar
Abstract: Machine learning technologies have underpinned the recent revolution in artificial intelligence. But at their heart, they are simply data driven decision making algorithms. While the popular press is filled with the achievements of these algorithms in important domains such as object detection in images, machine translation and speech recognition, there are still many open questions about how these technologies might be implemented in domains where we have existing solutions but we are constantly looking for improvements. Roughly speaking, we characterise this domain as “machine learning in the physical world”. How do we design, build and deploy machine learning algorithms that are part of a decision making system that interacts with the physical world around us? In particular, machine learning is a data driven endeavour, but real world systems are physical and mechanistic. In this talk we will introduce some of the challenges for this domain and and propose some ways forward in terms of solutions.
Speaker: Gianluca Iaccarino, Professor of Mechanical Engineering and Director of the Institute for Computational and Mathematical Engineering, Stanford University, USA
Date/Time: Wednesday 2nd June 2021, 4pm BST/11am EDT/12am JST
Title: Data-Free and Data-Driven Uncertainty Quantification in Turbulent Flow Simulations
Watch a recording of this webinar
Abstract: Fluid simulations and predictions of turbulent motions play a critical role in many fluid flow scenarios but particularly when the flow is prone to separation. Despite continued advances in high-fidelity turbulent flow simulations, closure models based on the Reynolds-Averaged Navier-Stokes (RANS) equations are projected to remain in use for considerable time to come, especially when rapid responses are required or large number of conditions need to be studied. However, it is common knowledge that RANS predictions are corrupted by epistemic model-form uncertainty to a degree which is unknown a-priori. Hence, to obtain a computational framework of predictive utility, a model-form Uncertainty Quantification (UQ) framework is indispensable. UQ enables the characterization of the potential errors in the simulations and leads to prudent estimates of the impact of turbulence assumptions on the quantity of interest. Over the last several years we have developed an approach that uses a spectral decomposition of the modeled Reynolds-Stress Tensor (RST) which is the building block of all RANS closure. This strategy allows for the introduction of decoupled perturbations into the baseline intensity (kinetic energy), shape (eigenvalues), and orientation (eigenvectors) of the Reynolds stresses. Within this perturbation framework, we look for a-priori known limiting physical bounds. Since these bounds are universal, they can be used to constrain uncertainty estimates in any predictive flow scenario. Thus, even in the absence of relevant training data, we can maximize the spectral perturbations in order to obtain conservative uncertainty intervals. The approach has been proven to be useful in a variety of applications. On the other hand, any reference data (experiments or high-fidelity resolved turbulence simulations) can be used to further constrain the uncertainty estimates using commonly available data assimilation techniques. This provides a data-driven path towards UQ estimates. We will demonstrate our framework on canonical flow problems using two common data-driven approaches, random forest regression and deep neural networks. We consider a database of high-fidelity simulation data for the flow over a separated flows and compare the resulting uncertainty estimates to conventional RANS closures and available experimental data.
Speaker: George Em Karniadakis, The Charles Pitts Robinson and John Palmer Barstow Professor of Applied Mathematics and Engineering, Brown University, USA
Date/Time: Wednesday 7th April 2021, 4pm BST/11am EDT/12am JST
Title: Approximating functions, functionals, and operators using deep neural networks for diverse applications
Watch a recording of this webinar
Abstract: We will present a new approach to develop a data-driven, learning-based framework for predicting outcomes of physical systems and for discovering hidden physics from noisy data. We will introduce a deep learning approach based on neural networks (NNs) and generative adversarial networks (GANs). Unlike other approaches that rely on big data, here we “learn” from small data by exploiting the information provided by the physical conservation laws, which are used to obtain informative priors or regularize the neural networks. We will demonstrate the power of PINNs for several inverse problems, and we will demonstrate how we can use multi-fidelity modeling in monitoring ocean acidification levels in the Massachusetts Bay. We will also introduce new NNs that learn functionals and nonlinear operators from functions and corresponding responses for system identification. The universal approximation theorem of operators is suggestive of the potential of NNs in learning from scattered data any continuous operator or complex system. We first generalize the theorem to deep neural networks, and subsequently we apply it to design a new composite NN with small generalization error, the deep operator network (DeepONet), consisting of a NN for encoding the discrete input function space (branch net) and another NN for encoding the domain of the output functions (trunk net). We demonstrate that DeepONet can learn various explicit operators, e.g., integrals, Laplace transforms and fractional Laplacians, as well as implicit operators that represent deterministic and stochastic differential equations. More generally, DeepOnet can learn multiscale operators spanning across many scales and trained by diverse sources of data simultaneously.