From genetic variation to precision medicine
Published online by Cambridge University Press: 24 January 2023
Abstract
Genetics has been an important tool for discovering new aspects of biology across life. In humans, there is growing momentum behind the application of this knowledge to drive innovation in clinical care, most notably through developments in precision medicine. Nowhere has the impact of genetics on clinical practice been more striking than in the field of rare disorders. For most of these conditions, individual disease susceptibility is influenced by DNA sequence variation in a single or a small number of genes. In contrast, most common disorders are multifactorial and are caused by a complex interplay of multiple genetic, environmental and stochastic factors. The longstanding division of human disease genetics into rare and common components has obscured the continuum of human traits and echoes aspects of the century-old debate between the Mendelian and biometric views of human genetics. In this article, we discuss the differences in data and concepts between rare and common disease genetics. Opportunities to unify these two areas are noted and the importance of adopting a holistic perspective that integrates diverse genetic and environmental factors is discussed.
- Type
- Perspective
- Information
- Creative Commons
- This is an Open Access article, distributed under the terms of the Creative Commons Attribution licence (http://creativecommons.org/licenses/by/4.0), which permits unrestricted re-use, distribution and reproduction, provided the original article is properly cited.
- Copyright
- © The Author(s), 2023. Published by Cambridge University Press
References

Figure 1. Key features of forms of human disease at the monogenic and polygenic ends of the genetic architecture spectrum. Notably, although the terms monogenic and polygenic formally refer to the number of genes involved in the genetic component of a disorder, they have come to mean broader styles of genetic inheritance anchored on the distribution of variant effect sizes (concept from Loos and Yeo, 2022).
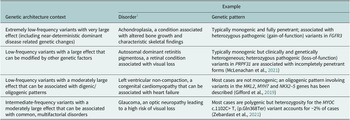
Table 1. Selected examples of genetic architecture contexts

Figure 2. Schematic outlining the distribution of variant frequencies and effect sizes for key groups of genetic changes associated with human phenotypes. The minor allele frequency spectrum for these variants ranges from extremely rare to very common. In the context of conditions related to reproductive fitness, rare causal variants generally have larger effect sizes than common changes.

Figure 3. Challenging the ‘rare disease – rare variant’ and ‘common disease – common variant’ paradigms. The rare disease – rare variant hypothesis, predicts that if a disease with a significant genetic component is rare in the population, then the underlying genetic abnormalities will also be found to be rare. In the past decade, a number of studies have challenged this paradigm and have highlighted the role of common genetic variation in rare phenotypes (e.g., Niemi et al., 2018; Michaud et al., 2022). A related hypothesis has been made for common disorders; this proposed that if a disease with a significant genetic component is common in the population, then the genetic contributors will also be common. This common disease – common variant hypothesis has dominated the field for a number of years but has now been refuted; many examples of rare genetic changes contributing substantially to special cases of common disorders have now been described (e.g., Loos and Yeo, 2022).

Figure 4. Schematic showing the joint effects of rare and common genetic variants on a disorder associated with a dosage-sensitive gene. In this hypothetical example, the presence of a rare variant results in loss-of-function of a copy of the affected gene, altering the background liability to the related disorder. This can be further modified by common variants with smaller effect sizes. In this case, the interaction between rare and common variation appears to push the individual beyond the disease threshold. It is noted that the variants may or may not interact in an additive fashion and that phase information is likely to be important.

Figure 5. Schematic showing how genetic and environmental factors interact to produce human disease phenotypes. Disease can be defined as “a state of individual homeostatic abnormality (…); an aberration of adaptation in the face of conditions which are suboptimal, not necessarily for all, but for [at least] one genetically and socially distinct individual” (Childs, 1977). Hence, disease risk (y-axis) can be plotted as a function of genotype (coloured lines) and environment (a multidimensional parameter that is shown here for visualisation purposes as one dimension at the x-axis). Some genotypes are associated with high-penetrance monogenic phenotypes (such as cystic fibrosis) and lead to disease in all environments (line A). Other diseases occur only in the case of a very specific pairing of genotype and environment; phenylketonuria falls into this group as it manifests in individuals who carry biallelic loss-of-function PAH variants but only in the context of a diet that includes phenylalanine (line B). Most diseases fall between these extremes (e.g., diabetes; line C) and arise from ‘mismatches’ between genotype and environment (modified from Benton et al., 2021).
Author comment: From genetic variation to precision medicine — R0/PR1
Comments
Further to recent correspondence with Dr Jones, Ms Beck and Professor Dominiczak, please find attached a Perspective article for consideration in your journal.
Review: From genetic variation to precision medicine — R0/PR2
Conflict of interest statement
Comments
Comments to Author: In ‘From genetic variation to precision medicine’, the authors discuss the resolution of rare and common disease research and inclusion of environmental influence in the development of Precision Medicine. The authors provide a framework for conceptualize rare and common variation in human disease. I have several comments for the authors’ consideration.
Major comments
1. Oligogenic models of disease, including digenic conditions, dual molecular diagnoses, and mutational burden are not deeply addressed here and yet it seems critical that the authors include these more directly in the discussed models of disease resulting from rare and/or common variants and gene dosage effects.
2. One advantage of family-focused studies is the ability to identify de novo variants, and this should be addressed more directly within the text.
3. On line 288, where the authors list health-focused population cohorts, H3Africa and AllofUs might also be mentioned.
4. Figure 1: the colored triangles and their intended interpretation are somewhat unclear. The first three items (genetic contribution, # variants, effect size) seem to mirror the height of the triangle — I.e. effect size on the left (monogenic) is on the taller side of the triangle suggesting a higher effect size, whereas effect size on the right (polygenic) is at the tip of the triangle suggesting a smaller contribution. However, the final two items (penetrance, environmental influence) seem to be aligned in the opposite fashion, with ‘high penetrance’ and ‘the environment is a key determinant’ both at the tip of the triangle. Some revision of the wording, or explanation of what is meant by the triangles may be helpful here to avoid misunderstanding.
5. Figure 4 is a bit tough to interpret. In part, this may be because the y-axis seems to indicate increasing disease risk as one moves further up the graph, however the area of the graph itself that is indicated as having crossed the disease threshold is the lower part of the graph (orange/red). Likewise, functional gene dosage appears to increase along the x-axis from left to right, however, the example of rare + common variants is likely meant by the authors to indicate a reduction in gene dosage.
Minor comments
6. Line 186: I believe ‘anti-PCK9’ was intended as ‘anti-PCSK9’.
7. I appreciated the table provided at the end of the manuscript, but was unable to find where it was specifically referenced within the main text.
Review: From genetic variation to precision medicine — R0/PR3
Comments
Comments to Author: In this perspective a hopeful future for genetics research in envisioned. The future of genetics in which common and rare disease genetics has a synthesis and synergism is the anticipated and hoped for goal. This is a refreshing perspective in that often the two fields of common disease GWAS studies and medical genetics are studied, treated and analyzed by different groups of individuals. Separate sessions at major conferences house these Mendelian genetics versus complex traits. However biological realities often show dramatic overlap in the genes involved in both. There is a perception of competition between groups doing these two types of research but the opportunities for synergy is where the authors emphasize. The authors point to emerging data showing that conditions like Huntington’s disease have common variant modifiers and that key components of common disease can be revealed through rare disease loci.
The authors wish to knock down some of the perceived barriers between these analyses. In the section on rare monogenic to common polygenic the argument is successfully laid out that the dichotomy between monogenic and polygenic is too simplistic and their example of eye development is an excellent one. For the rare or Mendelian traits the authors point out that many conditions labeled autosomal dominant, are in fact semi-dominant, and while this is a common sloppiness of definition in medical genetics, it does not actually point to a spectrum between rare and common. The authors don’t directly distinguish a spectrum between specific gene variants that appear to be deterministic versus those that are probabilistic. Modifiers notwithstanding an individual with enough repeats within a certain polyglutamine tract in their genome will inevitably have Huntington’s disease with certainty and this is a unique feature of some of the more rare monogenic conditions. The authors appear to be glossing over that distinction when they say
“In the near future, as whole-genome sequencing becomes the default assay, the artificial distinction between variants at the common and rare ends of the allele 202 frequency spectrum will erode and it will become easier to consider the entire 203 spectrum of genetic risk for an individual at once.”
While the field should strive to become facile at both common and rare ends of a wide spectrum, some of the differences between common probabilistic loci and ultra-rare deterministic variants is not artificial. An individual with specific mutations in FGFR3 will have achondroplasia for example. A medical geneticist might send a test in a clinic and provide a diagnosis on the basis of that molecular result, and the whole concept of a “molecular diagnosis” in those cases is somewhat dependent on how deterministic the variant is. An individual with a risk locus for diabetes or HTN may or may not ultimately have HTN so such results represent more of a challenge for a direct molecular diagnosis. As the authors point out, precision medicine results could however be part of a more wholistic risk assessment that could widely benefit population health. For that matter an individual with a very rare familial mutation in Rb or p53 has a probability but not a certainty of cancer, so there are rare variants that are not deterministic and suggest a spectrum of variant effects. In any case don’t support the argument that this distinction is entirely artificial when they also discuss phenomena such as dominance which they say is a blind spot for common approaches but essential for understanding rare variants. It might help if the authors could discuss genes that constitute intermediates between common and rare or blur the line with intermediate frequency but relatively large effect size.
In Family studies to population studies the authors lay out an argument of a resurgence in family based studies that will help the field grasp both common and rare variants. It is encouraging that the authors discuss a convergence of two distinct fields. The discussions of precision medicine and inclusive genetics offer great insights and the positive view of the future of genetics in synthesizing the perspectives and assumptions from common and rare variant studies is quite encouraging. Overall this perspective offers the reader a helpful and very positive view for the prospect of genetics to adopt more unified theories and approaches.
Recommendation: From genetic variation to precision medicine — R0/PR4
Comments
Comments to Author: Dear Dr. Sergouniotis,
The manuscript was fully evaluated by two independent peer reviewers who have raised a number of concerns which we ask you to address in a revised manuscript. We also ask you to provide a detailed point-by-point response letter, highlighting your responses to the review comments and a description of the changes you have made to the manuscript.
Decision: From genetic variation to precision medicine — R0/PR5
Comments
No accompanying comment.
Author comment: From genetic variation to precision medicine — R1/PR6
Comments
(please see the "PCM-22-0014R_response-to-reviewers" file that have been uploaded as "Supplementary Material")
Review: From genetic variation to precision medicine — R1/PR7
Conflict of interest statement
Comments
Comments to Author: The authors provide a throughful framework for how to conceptualize rare and common variation, and environmental impact, from a precision medicine lens.
The authors have responded thoroughly to reviewer feedback.
I do not have additional feedback to provide.
Review: From genetic variation to precision medicine — R1/PR8
Comments
Comments to Author: The authors have made a number of improvements and have addressed all the concerns of reviews.
Recommendation: From genetic variation to precision medicine — R1/PR9
Comments
Comments to Author: Both independent reviewers have affirmed that the authors have provided sufficient responses to their peer review comments and have addressed all concerns.
Decision: From genetic variation to precision medicine — R1/PR10
Comments
No accompanying comment.
- 5
- Cited by
Human health, well-being and behaviour are probabilistically shaped by the dynamic interplay between genetic and environmental factors. The landscape of genetic contributions to a given phenotype is referred to as its genetic architecture. This comprises the number of genetic variants that influence the phenotype; the magnitude of the variant effects; the variant frequencies in populations; and their interactions with one another and with the environment (Timpson et al., Reference Timpson, Greenwood, Soranzo, Lawson and Richards2018; Benton et al., Reference Benton, Abraham, LaBella, Abbot, Rokas and Capra2021; Visscher et al., Reference Visscher, Yengo, Cox and Wray2021).
Rare monogenic⟷common polygenic
The terms ‘monogenic’, ‘oligogenic’ or ‘polygenic’ have been classically used to describe the genetic architecture of traits and disorders (Figure 1 and Table 1). The phenotypes at the monogenic (or Mendelian) end of the spectrum are rare and driven by a small number of low-frequency variants with large effects (Figures 1 and 2). Particularly relevant to these phenotypes are the concepts of recessiveness and/or dominance (which relate to the functional link between heterozygous genetic variants and the resulting phenotype). Mendel defined these concepts specifically for discrete, discontinuous traits without intermediate forms. He and others distinguished the characteristic inheritance patterns that bear his name (in which hybrids and one original strain have identical phenotypes) from additive patterns (in which hybrids have an intermediate appearance with noticeable contribution of both alleles to the phenotype) (Zschocke et al., Reference Zschocke, Byers and Wilkie2022). It is worth noting that a significant proportion of the conditions described as dominant or recessive in the biomedical literature do not fulfil Mendel’s original criteria; many monogenic disorders, for example, exhibit semi-dominant or imperfect recessive inheritance with heterozygous carriers having a mild phenotype (Barton et al., Reference Barton, Hujoel, Mukamel, Sherman and Loh2022; Brandes et al., Reference Brandes, Weissbrod and Linial2022; Zschocke et al., Reference Zschocke, Byers and Wilkie2022).
Figure 1. Key features of forms of human disease at the monogenic and polygenic ends of the genetic architecture spectrum. Notably, although the terms monogenic and polygenic formally refer to the number of genes involved in the genetic component of a disorder, they have come to mean broader styles of genetic inheritance anchored on the distribution of variant effect sizes (concept from Loos and Yeo, Reference Loos and Yeo2022).
Table 1. Selected examples of genetic architecture contexts
1 It is noted that disease definition has an impact on the observed genetic architecture. For example, in certain disorders that are diagnosed after reproductive years (such as age-related macular degeneration [which can be associated with variants in the CFH gene] and Alzheimer’s disease [which can be associated with variants in the APOE gene]), large effect variants may lead to earlier and/or more severe clinical presentations.
Figure 2. Schematic outlining the distribution of variant frequencies and effect sizes for key groups of genetic changes associated with human phenotypes. The minor allele frequency spectrum for these variants ranges from extremely rare to very common. In the context of conditions related to reproductive fitness, rare causal variants generally have larger effect sizes than common changes.
The polygenic end of the genetic architecture spectrum includes a range of multifactorial conditions that are common and predominantly influenced by intermediate- and high-frequency variants across numerous genomic loci (each with a small effect size) (Figures 1 and 2; Claussnitzer et al., Reference Claussnitzer, Cho, Collins, Cox, Dermitzakis, Hurles, Kathiresan, Kenny, Lindgren, MacArthur, North, Plon, Rehm, Risch, Rotimi, Shendure, Soranzo and McCarthy2020). Genetic methods that can be used to study this group of conditions include genome-wide association studies (GWAS) and polygenic scores. These approaches assume additivity in the effects of genetic variants and generally have a ‘blind spot’ to phenomena like compound heterozygosity and recessiveness (Brandes et al., Reference Brandes, Weissbrod and Linial2022). Empirical and theoretical evidence support this key additivity assumption, and linear (additive) genetic models appear to provide a sufficient approximation of the underlying biological complexity for many phenotypes (Hivert et al., Reference Hivert, Sidorenko, Rohart, Goddard, Yang, Wray, Yengo and Visscher2021a,Reference Hivert, Wray and Visscherb; Brandes et al., Reference Brandes, Weissbrod and Linial2022). It is however unclear if this picture emerges because of undue focus on a relatively narrow set of traits and disorders (and/or a requirement to use additive models for the discovery of genomic loci associated with these phenotypes).
It can be argued that dichotomising phenotypic spectra into rare monogenic forms (that are mediated by low-frequency variants) and common polygenic subtypes (that are mediated by high-frequency variants) is no longer productive and, to an extent, obstructs the discovery of new aspects of biology (Figures 3 and 4). In our work specifically on human eye development, we can see the convergence of the rare and common components of genetics. We have for example found that multifactorial traits like visual function and retinal structure are associated with the same high-frequency genetic variants that play a major role in albinism, a rare recessive condition (Currant et al., Reference Currant, Hysi, Fitzgerald, Gharahkhani, Bonnemaijer, Senabouth, Hewitt, Atan, Aung, Charng, Choquet, Craig, Khaw, Klaver, Kubo, Ong, Pasquale, Reisman, Daniszewski, Powell, Pébay, Simcoe, Thiadens, van Duijn, Yazar, Jorgenson, MacGregor, Hammond, Mackey, Wiggs, Foster, Patel, Birney and Khawaja2021; Michaud et al., Reference Michaud, Lasseaux, Green, Gerrard, Plaisant, Fitzgerald, Birney, Arveiler, Black and Sergouniotis2022). We have also observed that combinations of common genetic changes in TYR, a major albinism-related gene that encodes the enzyme tyrosinase, can give rise to similar phenotypic manifestations to extremely rare loss-of-function variants in this gene. Notably, we have found evidence suggesting that the expressivity of loss-of-function alleles is altered by local and/or distal genetic interactions with other genetic changes (Michaud et al., Reference Michaud, Lasseaux, Green, Gerrard, Plaisant, Fitzgerald, Birney, Arveiler, Black and Sergouniotis2022). Similar interactions between low- and high-frequency genetic variation have been reported in a number of rare and common phenotypes including Hirschsprung disease (Tilghman et al., Reference Tilghman, Ling, Turner, Sosa, Krumm, Chatterjee, Kapoor, Coe, Nguyen, Gupta, Gabriel, Eichler, Berrios and Chakravarti2019), Huntington disease (Lee et al., Reference Lee, Huang, Orth, Gillis, Siciliano, Hong, Mysore, Lucente, Wheeler, Seong, McLean, Mills, McAllister, Lobanov, Massey, Ciosi, Landwehrmeyer, Paulsen, Dorsey, Shoulson, Sampaio, Monckton, Kwak, Holmans, Jones, MacDonald, Long and Gusella2022) and blood cell indices (Astle et al., Reference Astle, Elding, Jiang, Allen, Ruklisa, Mann, Mead, Bouman, Riveros-Mckay, Kostadima, Lambourne, Sivapalaratnam, Downes, Kundu, Bomba, Berentsen, Bradley, Daugherty, Delaneau, Freson, Garner, Grassi, Guerrero, Haimel, Janssen-Megens, Kaan, Kamat, Kim, Mandoli, Marchini, JHA, Meacham, Megy, O’Connell, Petersen, Sharifi, Sheard, Staley, Tuna, van der Ent, Walter, Wang, Wheeler, Wilder, Iotchkova, Moore, Sambrook, Stunnenberg, Di Angelantonio, Kaptoge, Kuijpers, Carrillo-de-Santa-Pau, Juan, Rico, Valencia, Chen, Ge, Vasquez, Kwan, Garrido-Martín, Watt, Yang, Guigo, Beck, Paul, Pastinen, Bujold, Bourque, Frontini, Danesh, Roberts, Ouwehand, Butterworth and Soranzo2016).
Figure 3. Challenging the ‘rare disease – rare variant’ and ‘common disease – common variant’ paradigms. The rare disease – rare variant hypothesis, predicts that if a disease with a significant genetic component is rare in the population, then the underlying genetic abnormalities will also be found to be rare. In the past decade, a number of studies have challenged this paradigm and have highlighted the role of common genetic variation in rare phenotypes (e.g., Niemi et al., Reference Niemi, Martin, Rice, Gallone, Gordon, Kelemen, McAloney, McRae, Radford, Yu, Gecz, Martin, Wright, Fitzpatrick, Firth, Hurles and Barrett2018; Michaud et al., Reference Michaud, Lasseaux, Green, Gerrard, Plaisant, Fitzgerald, Birney, Arveiler, Black and Sergouniotis2022). A related hypothesis has been made for common disorders; this proposed that if a disease with a significant genetic component is common in the population, then the genetic contributors will also be common. This common disease – common variant hypothesis has dominated the field for a number of years but has now been refuted; many examples of rare genetic changes contributing substantially to special cases of common disorders have now been described (e.g., Loos and Yeo, Reference Loos and Yeo2022).
Figure 4. Schematic showing the joint effects of rare and common genetic variants on a disorder associated with a dosage-sensitive gene. In this hypothetical example, the presence of a rare variant results in loss-of-function of a copy of the affected gene, altering the background liability to the related disorder. This can be further modified by common variants with smaller effect sizes. In this case, the interaction between rare and common variation appears to push the individual beyond the disease threshold. It is noted that the variants may or may not interact in an additive fashion and that phase information is likely to be important.
Family studies⟷population studies
The genetic architecture of traits and disorders can be studied using gene mapping approaches. During the 1980s and 1990s, efforts to map causal variants focused on rare monogenic phenotypes and mostly involved linkage studies in large pedigrees (Claussnitzer et al., Reference Claussnitzer, Cho, Collins, Cox, Dermitzakis, Hurles, Kathiresan, Kenny, Lindgren, MacArthur, North, Plon, Rehm, Risch, Rotimi, Shendure, Soranzo and McCarthy2020). In the 2000s, advances in genotyping array technologies (and the characterisation of the extensive linkage disequilibrium properties of human variation) enabled testing for associations between common phenotypes and genetic variation at a genome-wide scale. Early GWAS demonstrated the potential of these agnostic genomic surveys to highlight novel biological insights (e.g., CFH in age-related macular degeneration [Klein et al., Reference Klein, Zeiss, Chew, Tsai, Sackler, Haynes, Henning, SanGiovanni, Mane, Mayne, Bracken, Ferris, Ott, Barnstable and Hoh2005] or IL23R in inflammatory bowel disease [Duerr et al., Reference Duerr, Taylor, Brant, Rioux, Silverberg, Daly, Steinhart, Abraham, Regueiro, Griffiths, Dassopoulos, Bitton, Yang, Targan, Datta, Kistner, Schumm, Lee, Gregersen, Barmada, Rotter, Nicolae and Cho2006]), with the Wellcome Trust Case Control Consortium (https://www.wtccc.org.uk/) showing the broad applicability of these techniques (Claussnitzer et al., Reference Claussnitzer, Cho, Collins, Cox, Dermitzakis, Hurles, Kathiresan, Kenny, Lindgren, MacArthur, North, Plon, Rehm, Risch, Rotimi, Shendure, Soranzo and McCarthy2020; Crouch and Bodmer, Reference Crouch and Bodmer2020).
These successes have catalysed a shift from using family/pedigree data to studying whole populations at the genome-wide scale. More recently, however, there has been a renewed interest in conducting within-family studies (Uricchio, Reference Uricchio2020; Visscher et al., Reference Visscher, Yengo, Cox and Wray2021). These experimental designs are known to be efficient at dissecting ‘near monogenic’ phenotypes (including through the identification of de novo mutational events) but another key advantage is their ability to separate direct from indirect genetic effects. Indirect genetic effects include the influence of parental and sibling genotypes on the proband through alterations to the family environment (e.g., parents or older siblings can influence the school achievement or smoking behaviour of younger siblings) (Howe et al., Reference Howe, Evans, Hemani, Davey Smith and Davies2022). Taking these indirectly causal factors into account is particularly important for understanding phenotypes with behavioural components (Kong et al., Reference Kong, Thorleifsson, Frigge, Vilhjalmsson, Young, Thorgeirsson, Benonisdottir, Oddsson, Halldorsson, Masson, Gudbjartsson, Helgason, Bjornsdottir, Thorsteinsdottir and Stefansson2018). Overall, it is becoming increasingly evident that certain questions in human genetics are best answered using within-family studies and specially tailored experimental designs.
Genotyping arrays⟷whole-genome sequencing
For the past two decades, genotyping of individuals participating in GWAS mainly involved using DNA arrays. These assays test a large number of intermediate- and high-frequency variants but generally overlook low-frequency changes, especially if these are in low linkage disequilibrium with neighbouring variants. Notably, it is now possible and increasingly cost-effective to comprehensively assay variation across the allele frequency spectrum using whole-genome sequencing. This approach is gradually replacing genotyping arrays as the method of choice for genetic association analyses (Uffelmann et al., Reference Uffelmann, Huang, Munung, de Vries, Okada, Martin, Martin, Lappalainen and Posthuma2022; Wainschtein et al., Reference Wainschtein, Jain, Zheng, Cupples, Shadyab, McKnight, Shoemaker, Mitchell, Psaty, Kooperberg, Liu, Albert, Roden, Chasman, Darbar, Lloyd-Jones, Arnett, Regan, Boerwinkle, Rotter, O’Connell, Yanek, de Andrade, Allison, McDonald, Chung, Fornage, Chami, Smith, Ellinor, Vasan, Mathias, Loos, Rich, Lubitz, Heckbert, Redline, Guo, Chen, Laurie, Hernandez, McGarvey, Goddard, Laurie, North, Lange, Weir, Yengo, Yang and Visscher2022).
A convergence has begun between what has been two distinct fields, one focusing on families and studying rare, monogenic phenotypes and one focusing on populations and analysing common traits and disorders. Methodological challenges remain (e.g., around addressing bias due to stratification or around incorporating phase information and structural variation) but large-scale sampling of families with whole-genome sequencing data is expected to help us build a more complete picture of the role of heritable variation in human phenotypes (Wainschtein et al., Reference Wainschtein, Jain, Zheng, Cupples, Shadyab, McKnight, Shoemaker, Mitchell, Psaty, Kooperberg, Liu, Albert, Roden, Chasman, Darbar, Lloyd-Jones, Arnett, Regan, Boerwinkle, Rotter, O’Connell, Yanek, de Andrade, Allison, McDonald, Chung, Fornage, Chami, Smith, Ellinor, Vasan, Mathias, Loos, Rich, Lubitz, Heckbert, Redline, Guo, Chen, Laurie, Hernandez, McGarvey, Goddard, Laurie, North, Lange, Weir, Yengo, Yang and Visscher2022; Young AI, 2022).
Towards precision medicine
The drive behind studying the genetic architecture of human phenotypes follows a desire to explain and understand all the genetic contributions to human disorders. This knowledge directly informs the goals of medical genetics which include assisting in disease diagnostics and facilitating the identification of novel therapeutics. Furthermore, genetic studies are one of the building blocks of precision medicine which examines how an individual’s unique genetic and environmental/lifestyle characteristics come together to inform their health (Jameson and Longo, Reference Jameson and Longo2015; Ashley, Reference Ashley2016; Martschenko and Young, Reference Martschenko and Young2022). Below we provide a few examples of how genetic investigations can help us move away from ‘one-size-fits-all’ approaches to medical decisions and treatments.
First, genetic insights from gene mapping efforts can be used to obtain accurate molecular diagnoses. For many clinical presentations, there is great value in trying to refine the clinical diagnosis through genetic testing (which may involve DNA sequencing of disease-related genes, polygenic score estimation or a hybrid approach). The utility of genetic testing extends beyond rare phenotypes that are highly suggestive of a monogenic disorder (e.g., bilateral cataracts in a newborn). A notable clinical scenario is that of an individual with a common disorder (e.g., diabetes, obesity or cancer) who is found through genetic testing to carry a low-frequency genetic variant with a large effect. In a subset of cases, identifying such monogenic forms of common disorders can drive evidence-based changes in care management and result in improved outcomes (Loos and Yeo, Reference Loos and Yeo2022; Murray et al., Reference Murray, Khoury and Abul-Husn2022; Williams, Reference Williams2022). It is worth noting however that, for most patients, obtaining a genetic diagnosis does not lead to a large therapeutic change. Nonetheless, an accurate diagnosis can improve planning and remove the need for inappropriate additional investigations which can be unpleasant and costly. Furthermore, it can have a big impact on affected families by providing a sense of closure/understanding or by allowing for better advice to be given regarding future reproductive choices. Overall, the use of diagnostic genetic testing in selected clinical presentations can make a difference to the affected individual (by better planning and sometimes better care), to their family (by providing closure and helping plan for other children if desired) and to the healthcare system (better planning, more targeted management).
Second, genetic discoveries can be used to develop tests that help identify subjects who are at a high risk of developing a specific disorder. Such predictive tests have been part of the care of families affected by certain monogenic conditions for a while, with non-invasive prenatal testing being a notable application (Zhong and Chiu, Reference Zhong and Chiu2022). More recently, GWAS data have been used to create polygenic scores that aim to enhance disease risk prediction for common disorders (e.g., cardiovascular disease, glaucoma or breast cancer). The clinical utility of these tools for population-level screening will, to a large extent, depend on how they will be combined with other information including lifestyle factors, established biomarkers and/or the results of genetic tests that focus on low-frequency variant detection (Torkamani et al., Reference Torkamani, Wineinger and Topol2018; Mars et al., Reference Mars, Koskela, Ripatti, Kiiskinen, Havulinna, Lindbohm, Ahola-Olli, Kurki, Karjalainen, Palta, Neale, Daly, Salomaa, Palotie, Widén and Ripatti2020; Polygenic Risk Score Task Force of the International Common Disease Alliance, 2021; Szustakowski et al., Reference Szustakowski, Balasubramanian, Kvikstad, Khalid, Bronson, Sasson, Wong, Liu, Wade Davis, Haefliger, Katrina Loomis, Mikkilineni, Noh, Wadhawan, Bai, Hawes, Krasheninina, Ulloa, Lopez, Smith, Waring, Whelan, Tsai, Overton, Salerno, Jacob, Szalma, Runz, Hinkle, Nioi, Petrovski, Miller, Baras, Mitnaul and Reid2021; Kullo et al., Reference Kullo, Lewis, Inouye, Martin, Ripatti and Chatterjee2022).
Third, the identification of genetic variants contributing to human disease can inform therapeutic development and planning. Highly publicised examples of genotype-informed treatments include anti-PCSK9 cholesterol-lowering medications (Sabatine et al., Reference Sabatine, Giugliano, Keech, Honarpour, Wiviott, Murphy, Kuder, Wang, Liu, Wasserman, Sever and Pedersen2017; Schwartz et al., Reference Schwartz, Steg, Szarek, Bhatt, Bittner, Diaz, Edelberg, Goodman, Hanotin, Harrington, Jukema, Lecorps, Mahaffey, Moryusef, Pordy, Quintero, Roe, Sasiela, Tamby, Tricoci, White and Zeiher2018), BRAF/MEK-targeted therapy for metastatic melanoma (Vellano et al., Reference Vellano, White, Andrews, Chelvanambi, Witt, Daniele, Titus, McQuade, Conforti, Burton, Lastrapes, Ologun, Cogdill, Morad, Prieto, Lazar, Chu, Han, MAW, Helmink, Davies, Amaria, Kovacs, Woodman, Patel, Hwu, Peoples, Lee, Cooper, Zhu, Gao, Banerjee, Lau, Gershenwald, Lucci, Keung, Ross, Pala, Pagan, Segura, Liu, Borthwick, Lau, Yates, Westin, Wani, Tetzlaff, Haydu, Mahendra, Ma, Logothetis, Kulstad, Johnson, Hudgens, Feng, Federico, Long, Futreal, Arur, Tawbi, Moran, Wang, Heffernan, Marszalek and Wargo2022), triple-combination CFTR modulator therapy for cystic fibrosis (Middleton et al., Reference Middleton, Mall, Dřevínek, Lands, McKone, Polineni, Ramsey, Taylor-Cousar, Tullis, Vermeulen, Marigowda, McKee, Moskowitz, Nair, Savage, Simard, Tian, Waltz, Xuan, Rowe and Jain2019) and voretigene neparvovec intravitreal gene therapy for RPE65-related retinal dystrophy (Russell et al., Reference Russell, Bennett, Wellman, Chung, Yu, Tillman, Wittes, Pappas, Elci, McCague, Cross, Marshall, Walshire, Kehoe, Reichert, Davis, Raffini, George, Hudson, Dingfield, Zhu, Haller, Sohn, Mahajan, Pfeifer, Weckmann, Johnson, Gewaily, Drack, Stone, Wachtel, Simonelli, Leroy, Wright, High and Maguire2017). These examples highlight that gene mapping studies can not only increase our understanding of the biology of human disease but also improve our practical ability to contribute meaningfully to their treatment.
Inclusive genetics and the environment
The past 20 years have witnessed a rapid acceleration in our understanding of the genetic basis of many human disorders. With this greater understanding, it became possible to redefine disease at higher resolution and to target many disorders with precise therapies (Ashley, Reference Ashley2016).
In the near future, as whole-genome sequencing becomes the default assay, the artificial distinction between variants at the common and rare ends of the allele frequency spectrum will erode and it will become easier to consider the entire spectrum of genetic risk for an individual at once (McCarthy and Birney, Reference McCarthy and Birney2021). However, the transition from array-based to sequence-based GWAS (McMahon et al., Reference McMahon, Lewis, Buniello, Cerezo, Hall, Sollis, Parkinson, Hindorff, Harris and MacArthur2021) will require a sharper focus both on the development of appropriate methodology and on the collection of data from individuals/families with diverse ancestries.
Genetic factors are one of the many aspects to consider when studying disease risk or contemplating precision medicine approaches. Environmental factors, a reductionist label referring to a range of non-genetic parameters, heavily influence most traits and disorders. Such factors include generic external exposures (e.g., social capital, education, financial status), specific external exposure (e.g., infectious agents, chemical pollutants, radiation), and internal exposures (e.g., metabolism, hormones, physical activity) (Peters et al., Reference Peters, Nawrot and Baccarelli2021; Canali and Leonelli, Reference Canali and Leonelli2022). Another important parameter is time. Time and timing are critical to understanding how genes and environments operate together to shape probabilistically the trajectories of our lives (Figure 5). Experiences and exposures in early life for example are crucial elements of potential for success, failure, health or misfortune (Boyce et al., Reference Boyce, Sokolowski and Robinson2020).
Figure 5. Schematic showing how genetic and environmental factors interact to produce human disease phenotypes. Disease can be defined as “a state of individual homeostatic abnormality (…); an aberration of adaptation in the face of conditions which are suboptimal, not necessarily for all, but for [at least] one genetically and socially distinct individual” (Childs, Reference Childs1977). Hence, disease risk (y-axis) can be plotted as a function of genotype (coloured lines) and environment (a multidimensional parameter that is shown here for visualisation purposes as one dimension at the x-axis). Some genotypes are associated with high-penetrance monogenic phenotypes (such as cystic fibrosis) and lead to disease in all environments (line A). Other diseases occur only in the case of a very specific pairing of genotype and environment; phenylketonuria falls into this group as it manifests in individuals who carry biallelic loss-of-function PAH variants but only in the context of a diet that includes phenylalanine (line B). Most diseases fall between these extremes (e.g., diabetes; line C) and arise from ‘mismatches’ between genotype and environment (modified from Benton et al., Reference Benton, Abraham, LaBella, Abbot, Rokas and Capra2021).
Understanding the environmental contributors to specific disorders can highlight opportunities for treatment and prevention. It is known that, in certain scenaria, lifestyle changes can negate the development or progression of a disorder and may be as effective as any specific treatment; examples range from dietary interventions for rare inborn errors of metabolism such as galactosaemia to tailored lifestyle changes for chronic diseases such as hypertension and COPD (chronic obstructive pulmonary disease). To understand the role of targeted or broad interventions in various disorders and settings, the study of population-scale cohorts is required (as planned in the UK [Our Future Health], the USA [All of Us], Denmark, Iceland, Estonia, Finland and many other countries in Europe, Africa [H3Africa] and elsewhere). Additionally, there is a pressing need to improve the measurement and recording of environmental variables. Some of these factors can be imputed from the household location over a person’s lifetime and then cross-referenced to location-based environmental measures. However, many of the most important environmental parameters, such as the social environment around an individual, require individual measurement, ideally on a longitudinal basis. Here, the collaboration of geneticists with epidemiologists and sociologists will be critical, with each discipline bringing its insight into the holistic question of individual difference in phenotypes.
Ultimately, a deeper understanding of the interaction between genetic and non-genetic contributors to human disorders will allow a broader framing of disease risk, and will provide insights into how to develop optimal environments for each genetically unique individual.
Open peer review
To view the open peer review materials for this article, please visit https://doi.org/10.1017/pcm.2022.11.
Acknowledgements
We acknowledge funding from the Wellcome Trust (224643/Z/21/Z, Clinical Research Career Development Fellowship to P.I.S.; 200990/Z/16/Z, Transforming Genetic Medicine Initiative) and the UK National Institute for Health Research (NIHR) Clinical Lecturer Programme (CL-2017-06-001 to P.I.S.).
Author contributions
P.I.S. wrote the manuscript. T.F. and E.B. critically revised and approved the manuscript.
Competing interest
E.B. is a paid consultant and equity holder of Oxford Nanopore, a paid consultant to Dovetail and a non-executive director of Genomics England, a limited company wholly owned by the UK Department of Health and Social Care. All other authors declare no competing interests.