Resting energy expenditure (REE) is the largest component of daily energy expenditure and represents >70 % of total energy expenditure in people with a sedentary lifestyle( Reference Ravussin, Lillioja and Anderson 1 ). Accurate prediction of REE in obese individuals is needed to improve individual clinical evaluation in order to establish adequate dietary intake goals for effective weight management( Reference Heymsfield, Harp and Reitman 2 ). The most accurate procedures for measuring REE include indirect calorimetry, which is considered the gold standard method. However, their use is limited in clinical practice due to equipment costs, the need of qualified and trained personnel and time constraints. Several predictive equations have been developed and are frequently used as major alternative methods to prescribe dietary needs( Reference Schoeller 3 , Reference Frankenfield, Coleman and Alam 4 ).
The validity of REE predictive equations in obese individuals is still debatable, considering that the choice of an inaccurate equation might be one of the reasons explaining the low efficacy of weight-loss treatments( Reference Frankenfield, Roth-Yousey and Compher 5 ). Previous studies have shown that the validity of an energy prediction equation may depend on several factors such as ethnicity, age, body composition and nutritional status of the population( Reference Weijs 6 , Reference Ruiz, Ortega and Rodríguez 7 ). The level of obesity is an important factor in determining the accuracy of a predictive equation, but this level varies among studies. Therefore, validation of predictive equations should be performed in specific groups of obese subjects. Evidence exists that weight loss leads to a reduction in REE, and the validity of these equations could be different before and after an energy-restricted diet intervention( Reference Ruiz, Ortega and Rodríguez 7 ). On the other hand, the possible variation in the validity of REE equations when weight loss is achieved through programmes combining diet and exercise remains to be elucidated. Thus, a better understanding of the accuracy of REE predictive equations in obese individuals before and after an interdisciplinary therapy may help patients in weight management.
In the present study, we selected REE predictive equations on the basis of feasibility for clinical use and compared the estimated v. the measured REE in Brazilian obese, non-morbid, pre-menopausal women. The aims of this study were as follows: (1) to investigate the validity of these REE predictive equations before and after a long-term interdisciplinary therapy, and (2) to identify which REE predictive equation is the best alternative to indirect calorimetry before and after a long-term interdisciplinary therapy in Brazilian obese women.
Methods
Study population
Data were derived from measures of participants enrolled in a long-term, interdisciplinary therapy programme by the Obesity Study Group of Federal University of São Paulo, Santos, SP, Brazil. A total of forty obese women were enrolled and subjected to the therapy. They were evaluated at baseline and after the therapy. Inclusion criteria for participating were as follows: aged 30–50 years and BMI between 30 and 39·9 kg/m2. Non-inclusion criteria were musculoskeletal limitations preventing the practise of physical exercise, presence of metabolic or endocrine diseases, identified genetic diseases, pregnancy, previous drug utilisation and excessive chronic alcohol consumption (more than 1 dose/d for women or 2 doses/d for men). This study was conducted according to the guidelines laid down in the Declaration of Helsinki, and all procedures involving human subjects were approved by the Institutional Ethical Committee (no. 722.247). This study was also registered at the Clinical trial.gov as NCT02573688. Written informed consent was obtained from all subjects.
Long-term interdisciplinary therapy
The interdisciplinary lifestyle modification therapy consisted of nutritional counselling, physical exercise, and psychological and physiotherapy support during the course of 1 year. The main purpose of this therapy was to enhance the quality of life of the participants by promoting an active lifestyle, healthy nutritional habits and weight loss.
Nutritional programme
The nutritional intervention consisted of group meetings for dietetics lessons and prescription of individual diets. The participants had dietetics lessons once a week, which provided information related to improving food consumption and nutritional quality. For prescribing diet, energy intake was set at levels recommended by the dietary reference intakes( 8 ) using equations for obese subjects with low levels of physical activity of the same age and sex following a balanced diet in accordance with the adapted food pyramid( Reference Philippi, Teresa and Cruz 9 ). Foods were distributed into eight groups according to basic nutrient contribution to the diet (cereals, fruits, vegetables, beans, milk, meat, fat and sugar). The distribution of macronutrients was as follows: fat (20–30 %), carbohydrate (45–60 %) and protein (10–20 %)( 8 ).
Physical exercise programme
The intervention focused on improving activity habits and body composition, leading to an increase in energy expenditure and, consequently, to a negative energy balance. Participants followed an aerobic plus resistance training (AT+RT) three times a week, under the supervision of an exercise physiologist. Each session included 30 min of AT and 30 min of RT, totalling 180 min/week. The exercises included walking, running, aerobic gymnastics, muscular endurance exercises, recreational activities, dances and others. The exercise programme was based on guidelines from the American College of Sports Medicine, which recommend >150 min/week of exercise with an energy consumption rate of 5021–7531 kJ/week (1200–1800 kcal/week) for effective weight loss( Reference Donnelly, Blair and Jakicic 10 ).
Psychological programme
Participants were followed-up weekly, and if necessary individual psychological therapy was recommended. Once a week, they participated in a group session of 60-min duration. The aim of these sessions was to discuss psychological aspects commonly associated with obesity, such as depression, anxiety, stress, family problems, body image, eating disorders and others. The idea was to help the participants face difficulties and to facilitate behaviour modification and lifestyle improvement.
Physiotherapy programme
Physiotherapy interventions aim at increasing functional capacity. Participants were followed-up once a week. Each session was split into 20 min of theoretical discussions and 40 min of practical activities. Information on health issues of the musculoskeletal system was discussed during theoretical discussions. The main topics were ergonomic adaptations, posture for daily activities, risk of falls in obese individuals and musculoskeletal adaptations related to obesity. Besides, functional exercises were proposed to improve static and dynamic balance, postural stability and flexibility.
Anthropometric measures and body composition
Anthropometric measures were obtained with subjects wearing light clothing and no shoes. Body mass was determined using a Toledo balance (Sanny®, model BL-2097PP; Sanny Inc.), with a maximum capacity of 200 kg and a calibration of 0·01 kg. Height was measured to the nearest 0·5 cm using a wall-mounted stadiometer (Sanny®, model ES-2030; Sanny Inc.). After obtaining the data, BMI was calculated as the ratio of weight:height squared (kg/m2).
Body composition was assessed by dual-energy X-ray absorptiometry (Lunar Prodigy Advance; GE Medical Systems) for determination of fat mass (kg), percentage body fat and fat-free mass (FFM) (% and kg). The procedure was performed in a specialised clinic by an imaging specialist.
Indirect calorimetry
REE was measured by indirect calorimetry. Indirect calorimetry was performed using a ventilated hood system (Fitmate®; Cosmed). Fitmate is an equipment that measures REE by VO2. REE values are expressed in kJ/d (kcal/d). Participants fasted for ≥4 h and were asked not to perform any intense physical activity 24 h before the measurements( Reference Weijs 6 ). The participants were in the supine position and awake during the procedure. The measurements took 15 min to be completed. The first 5 min were disregarded and were only important for the participant to adapt to the test conditions. This way, we were safe to measure the REE in the steady state condition.
Resting energy expenditure predictive equations
Predictive equations were obtained by screening previous publications. Inclusion criteria were as follows: (i) equations based on body weight, height, age, sex and/or FFM and fat mass (FM) and (ii) equations developed for adults. Exclusion criteria were as follows: (i) equations derived only for elderly populations, patients or athletes; (ii) small (n<20 %) proportion of overweight subjects; (iii) small sample size (n<50); and (iv) specific ethnic groups or insufficient information. According to these criteria, we included a total of twelve REE equations (Table 1).
Table 1 Resting energy expenditure (REE) predictive equations
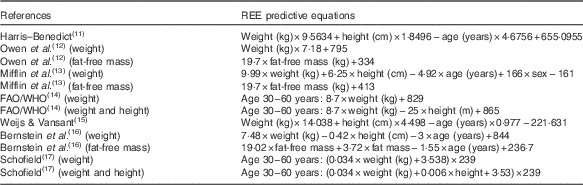
Statistical analysis
Sample size was calculated on the basis of REE data from a previous weight-loss programme including obese women( Reference Ruiz, Ortega and Rodríguez 7 ). According to this study, a reduction of 10 % in REE measured by indirect calorimetry is expected after a weight loss programme. Assuming an α error of 0·05 and a power of 80 %, a minimum of thirty-two individuals would be sufficient to assess the agreement between REE predictive equations and measured REE in our study.
Data were checked for normality of distribution using the Shapiro–Wilk test. Parametric variables are presented as means and standard deviations. We conducted paired Student’s t tests to analyse differences in changes in body weight, BMI, FM and FFM after the interdisciplinary therapy.
The accuracy of the predictive equations at individual and population levels was calculated. The bias (mean percentage differences between REE estimations by predictive equations and measured REE values by calorimetry) was considered a measure of accuracy at group levels. The percentage of women who had a REE predicted within ±10 % of the REE measured was considered a measure of accuracy at the individual level. A prediction between 90 and 110 % of the REE measured was considered an accurate prediction, a prediction <90 % of the REE measured was classified as an under-prediction and a prediction >110 % of the REE measured was classified as an over-prediction. The root mean sum of squared errors (RMSE) was used to indicate how well the model predicted in our data set.
Intraclass correlation coefficient (ICC), paired Student’s t test and Bland–Altman plot analysis for comparing values of estimated and measured REE were used to determine the reliability of REE predictive equations. Measured and predicted REE were compared by paired Student’s t test to analyse significant differences. The agreement between REE predicted equations and measured REE was graphically examined by plotting the difference between the predicted and the measured REE against their mean values, with the 95 % limits of agreement (mean difference ±1·96 sd of the difference)( Reference Bland and Altman 18 ). The reproducibility of REE predictive equations was evaluated by the ICC with its 95 % CI.
Data were analysed using SPSS version 23.0; ICC and Bland–Altman plot values were analysed using MedCalc software (version 15.10). A P value of <0·05 was considered to be significant.
Results
In this study sample of forty obese women, the mean age was 41 (sd 6) years, and the mean height was 164 (sd 5) cm. Participants achieved significant improvements in body weight, BMI, FM and FFM after the interdisciplinary therapy (Table 2).
Table 2 Clinical data of individuals before and after the interdisciplinary therapy (Mean values and standard deviations)

* P<0·001, ** P<0·05 (mean value after therapy was significantly different from that of baseline).
Table 3 shows (before and after the interdisciplinary therapy) mean and standard deviation values of measured REE and estimated REE with selected predictive equations, percentage bias, RMSE (kJ/d (kcal/d)) and ICC. At baseline, we observed a significant bias in half of the REE predictive equations (P<0·05). The highest bias observed corresponded to the equation reported by Bernstein et al. when including FFM and FM (−25·5 %, P<0·001). Equations that showed non-significant REE overestimation or underestimation were the Harris–Benedict equation, the Mifflin et al. equation when using weight, FAO/WHO equations and the Schofield equation when using only weight and weight and height together. The lowest bias observed corresponded to the two equations reported by Schofield (−0·1 %, P=0·46).
Table 3 Evaluation of resting energy expenditure (REE) predictive equations in Brazilian obese women before and after an interdisciplinary therapy based on bias, root mean sum of squared errors (RMSE) and intraclass correlation coefficient (ICC)

W, weight; WH, weight and height; FFM, fat-free mass.
* P<0·05, ** P<0·001 (comparison of REE predicted and REE measured).
After interdisciplinary therapy, the equation reported by Weijs & Vansant was the one with the lowest bias (+2·3 %, P=0·724), with RMSE of 1234 kJ/d (295 kcal/d). The highest bias observed corresponded to the equation reported by Bernstein et al. when including FFM and FM (−31·1 %, P<0·001), with RMSE of 2358 kJ/d (564 kcal/d).
The ICC varied from 0·15 (95 % CI –0·08, 0·40) to 0·37 (95 % CI 0·08, 0·60) at baseline and from 0·09 (95 % CI –0·07, 0·31) to 0·40 (95 % CI 0·10, 0·63) after therapy. The highest ICC observed corresponded to the equation reported by Weijs & Vansant at both time points (Table 3).
The Bland–Altman plots for the difference between predicted and measured REE against the mean obtained using the Harris–Benedict and FAO/WHO equations are reported in Fig. 1 and 2. They present the best agreement compared with the other equations tested, with their mean differences lying within ±10 % of the REE measured. However, an inverse association between the average and the difference between predicted and measured REE was observed. The plots illustrate that both equations tend to overestimate measured REE values at lower means and tend to underestimate at higher means. The limits of agreement ranged from −2920·0 to 2674·4 kJ/d (−697·9 to 639·2 kcal/d). On the other hand, the Bernstein et al. predictive equation (when using FFM and FM) showed the worst agreement between measured and predicted REE values (Fig. 3). This predictive equation showed a stronger bias and revealed a tendency for underestimating measured REE values by 4184 kJ/d (1000 kcal/d).

Fig. 1 Bland–Altman plots of differences in resting energy expenditure (REE), measured using indirect calorimetry and calculated using the Harris–Benedict predictive equation in Brazilian obese women before and after an interdisciplinary therapy. , Mean difference between predicted and measured REE.
, 95 % limits of agreement (mean difference ±1·96 sd of the difference).

Fig. 2 Bland–Altman plots of differences in resting energy expenditure (REE) measured using indirect calorimetry and calculated using the FAO/WHO predictive equation in Brazilian obese women before and after an interdisciplinary therapy. , Mean difference between predicted and measured REE.
, 95 % limits of agreement (mean difference ±1·96 sd of the difference). WH, weight and height.

Fig. 3 Bland–Altman plots of differences in resting energy expenditure (REE) measured using indirect calorimetry and calculated using the Bernstein et al. predictive equation in Brazilian obese women before and after an interdisciplinary therapy. , Mean difference between predicted and measured REE.
, 95 % limits of agreement (mean difference ±1·96 sd of the difference). FFM, fat-free mass.
The equations of Harris–Benedict and FAO/WHO (when using weight and height) were the only ones that did not change significantly from the REE measured at baseline and after therapy. The Harris–Benedict equation provided 40–47·5 % accurate predictions before and after therapy, respectively (Fig. 4). The FAO/WHO equation provided 47·5 % accurate predictions, 32·5 % under-predictions and 20 % over-predictions after therapy (Fig. 5).

Fig. 4 Percentage of accurate (), under- (
) and over-predictions (
) for Harris–Benedict resting energy predictive equation in Brazilian obese women before and after an interdisciplinary therapy.

Fig. 5 Percentage of accurate (), under- (
) and over-predictions (
) for FAO/WHO resting energy predictive equation in Brazilian obese women before and after an interdisciplinary therapy.
Considering baseline and after therapy values separately, the equation reported by Mifflin et al. (when using weight) showed the best evaluation before therapy with a bias of −0·96 %, RMSE of 1339 kJ/d (320 kcal/d), ICC of 0·36 and 32·5 % accurate predictions (Fig. 6). After therapy, the best equation was the one reported by Weijs & Vansant (bias=+2·25 %, RMSE of 1234 kJ/d (295 kcal/d), ICC of 0·4 and 47·5 % accurate predictions).

Fig. 6 Percentage of accurate predictions for resting energy predictive equations in Brazilian obese women before and after an interdisciplinary therapy. W, weight; FFM, fat-free mass; WH, weight and height; , baseline;
, after therapy.
Discussion
This study shows a wide range of differences between predicted and measured REE in Brazilian obese women before and after an interdisciplinary therapy. The Harris–Benedict, Mifflin et al. (when using weight), FAO/WHO and Schofield equations showed mean differences between measured and predicted REE<5 % before therapy. After therapy, the Harris–Benedict, FAO/WHO and Weijs & Vansant equations achieved similar levels of accuracy. The Harris–Benedict and FAO/WHO (when using weight and height) equations were the only ones that did not show statistically significant differences when compared with indirect calorimetry before and after the interdisciplinary therapy. These results suggest that these two equations are the most suitable equations to estimate REE before and after a weight loss programme in obese (BMI 30–39·9 kg/m2), pre-menopausal women compared with the other published equations considered in this study. On the other hand, Bland–Altman plots did not show good agreement between these equations and indirect calorimetry. At the individual level, accuracy did not reach 50 %, considering all predictive equations. The Harris–Benedict equation provided 40 and 47·5 % accurate predictions before and after the interdisciplinary therapy, respectively, whereas the FAO/WHO equation provided 35 and 47·5 % accurate predictions. The average weight loss percentage in this study was around 6 %, which matches well with the current clinical recommendation for the treatment of obese individuals (≥5–10 % of initial weight)( Reference Wirth, Wabitsch and Hauner 19 ). These findings are clinically relevant and suggest that the best equation to estimate REE in obese women depends on whether the individual has participated or not in a weight-loss programme.
Energy intake restriction is one of the most commonly used interventions for the treatment of obesity( Reference Wirth, Wabitsch and Hauner 19 ). To reduce body weight, the energy requirements are calculated using REE predictive equations. There is no consensus about the most accurate REE predictive equation to be used for obese individuals. Although some studies support using the Mifflin equation, especially for European-American females, males and extremely obese females, other studies positively suggest the FAO/WHO or Owen equations as the best choices( Reference Weijs 6 , Reference Ruiz, Ortega and Rodríguez 7 , Reference Das, Saltzman and McCrory 20 ). Moreover, the Harris–Benedict equation has been found to be acceptable in individuals with a broad weight range and in extremely obese individuals( Reference Das, Saltzman and McCrory 20 , Reference Muller, Bosy-Westphal and Klaus 21 ). Such discordance is explained, for example, by the subject group composition, ethnicity, intrinsic variability among the methods or statistics used. Moreover, previous studies have noted that the error in the prediction of REE to be more likely in obese than in non-obese individuals( Reference Weijs and Vansant 15 , Reference Frankenfield, Rowe and Smith 22 ).
Recently, studies have been performed to find the best REE predictive equation before and after a weight-loss programme( Reference Ruiz, Ortega and Rodríguez 7 , Reference Siervo, Boschi and Falconi 23 ). The metabolic adaptation of REE caused by a energy intake-restriction period( Reference Jimenez Jaime, Leiva Balich and Barrera Acevedo 24 ) can affect the validity of equations derived from data of individuals with a stable energy balance( Reference Martin, Heilbronn and de Jonge 25 ). This disagreement can explain the observed variability in the accuracy of REE predictive equations before and after the interdisciplinary therapy in our study. This finding is in accordance with previous studies, which have shown that after losing at least 5 % of body weight we cannot use with accuracy the same equation used originally( Reference Ruiz, Ortega and Rodríguez 7 , Reference Siervo, Boschi and Falconi 23 ). On the other hand, the use of different equations in each situation is not as relevant in clinical practice as the use of a single equation to suit reliably situations both before and after weight loss. For this reason, we tried to evaluate the responsiveness of equations before and after therapy when compared with indirect calorimetry. On the basis of this, we were able to choose the best alternative to predict the REE in the target population.
Our study demonstrated that the Harris–Benedict and FAO/WHO equations did not show statistically significant differences when compared with indirect calorimetry before and after the interdisciplinary therapy. Furthermore, these equations had differences between predicted and measured values <5 %, the smallest RMSE and the highest accuracy level compared with the other equations in both periods of time evaluated. The FAO/WHO/United Nations University (UNU) equations were developed using a database of 7173 individuals, of which 47 % were Italian subjects( Reference FAO/WHO/UNU 14 ). A survey of the most recent studies suggests that in most cases the current FAO equations overestimate REE in many populations( Reference Henry 26 ). On the other hand, validation studies show that the FAO weight and height equation is the most accurate( Reference Weijs 6 ), which is in agreement with our results. An explanation for this could be that this equation, derived from a similar ethnic population as Brazil, is based on a population mainly of European descendent, especially Italians.
The Harris–Benedict equation is one of the most commonly used equations in clinical practice and, as it is the oldest, has undergone the most extensive validation( Reference Harris and Benedict 11 ). Weijs et al.( Reference Weijs and Vansant 15 ) examined the validity of REE predictive equations in 536 normal weight to morbidly obese Belgian women and showed that neither the Harris–Benedict nor the Mifflin equation is a reliable tool to predict REE across a wide variety of body weight (BMI 18·5–50 kg/m2). However, they noticed that the accuracy of the Harris–Benedict and the Mifflin equations was fairly low when considering a BMI range of 30–40 kg/m2. This could explain the relatively low accuracy observed in our study.
The Mifflin et al. ( Reference Mifflin, Jeor and Hill 13 ) equation was developed with a large sample of obese subjects. Several studies proposed this equation as the most valid to estimate REE in overweight and obese subjects aged 19–69 years (78 % accurate predictions)( Reference Ruiz, Ortega and Rodríguez 7 , Reference Weijs and Vansant 15 ). Ruiz et al. in a validation study conducted in seventy-eight obese women aged 19–49 years observed that 74 % were within the range of agreement (±10 % of measured REE) before a 12-week, energy-restricted diet intervention. However, this level of accuracy could not be reached after the diet intervention (24 % of accuracy)( Reference Ruiz, Ortega and Rodríguez 7 ). In our study, this equation provided 32·5 and 40 % accurate predictions before and after therapy, respectively. After interdisciplinary therapy, the Mifflin equation showed statistically significant differences compared with indirect calorimetry and could not be used with accuracy to predict REE in this population.
Regarding individual accuracy level, our study showed a low level of accurate predictions of the evaluated equations (<50 %). In addition, Bland–Altman plots illustrated a strong systematic association between the mean and the difference, which means predictive equations constantly underestimate or overestimate REE compared with indirect calorimetry. The REE values ranged up to 4519 kJ/d (1080 kcal/d). Considering that an energy deficit of 2092–2510 kJ/d (500–600 kcal/d) is recommended for weight reduction( Reference Wirth, Wabitsch and Hauner 19 ), the choice of a predictive equation that overestimates the REE could make the dietary therapy ineffective. Thus, it should be desirable to critically re-assess REE data and to generate regional and more homogeneous REE databases, mainly regarding level of obesity and stability of energy balance.
In agreement with other studies, we noted that the inclusion of body composition (FFM and/or FM) into the equations did not improve the accuracy of REE prediction( Reference Weijs 6 , Reference Ruiz, Ortega and Rodríguez 7 , Reference Weijs and Vansant 15 ). This is a relevant finding because equations based on anthropometric parameters (weight and height) are more feasible in clinical practice than are body composition-based equations.
Our study has limitations, which need to be taken into account in the interpretation of these findings. First, we did not measure sex hormone levels to ensure that women were at the same phase of the menstrual cycle at baseline and after the interdisciplinary therapy. Second, a large sample size is needed to better confirm the findings. Third, there were different percentages of weight loss after interdisciplinary therapy that could affect the accuracy variability.
In conclusion, this study showed that there is a wide variation in the accuracy of REE predictive equations before and after weight loss in non-morbid, obese women. The accuracy of REE predictive equations should be adequate to promote the efficacy of dietary counselling and obesity treatment. Our findings indicated that in the absence of a gold standard method the best equations to estimate REE in non-morbid obese, pre-menopausal women are the equations reported by Harris–Benedict and FAO/WHO/UNU when using weight and height. However, these predictive equations should be used with caution for obese women, as from Bland–Altman analysis the limits of agreement showed underestimation or overestimation around 2510 kJ/d (600 kcal/d). Future studies in obese populations are needed to further investigate the validity of REE predictive equations, to critically re-assess REE data and to generate regional and more homogeneous REE databases.
Acknowledgements
The authors thank the volunteers and investigating staff. The authors thank Professor Dr Victor Zuniga Dourado for his expert assistance in performing this study.
This work was supported by the CAPES-Reuni, FAPESP (2011/51723-7, 2015/00953-3) and CNPQ (471108/2011-1).
V. F. S. P. wrote the manuscript, performed the statistical analyses and performed the nutritional interventions. D. A. C. and L. C. Y. advised the group and designed the research protocol of the study. J. C. C. carried out the DXA in individuals. R. B. S., A. d. S. M., J. P. N. F., M. A. N. and S. G. A.-S. carried out the interdisciplinary interventions and collected data. Each author has read and approved the content of the submitted manuscript.
The authors declare that there are no conflicts of interest.