The contribution of eating out to daily dietary intakes has been gaining increasing attention in nutrition research, possibly due to the rising concern about the role of unwise dietary choices – especially when eating out – in chronic diseases and nutrition-related disorders, including obesity and type 2 diabetes(1–Reference Duffey, Gordon-Larsen and Jacobs4). It is easier to collect information on dietary intakes at particular locations through open-ended methods like 24 h dietary recalls (24hDR) or food diaries than through FFQ, which have generally been used to assess diet–disease associations(Reference Beaton5, Reference Sempos, Liu and Ernst6). At national level, for a number of reasons mainly budgetary, national dietary surveys making use of 24hDR or food diaries often collect data for one or two records per participant(Reference Rizek and Pao7–Reference Merten, Ferrari and Bakker12). At an international level, two publications made use of a standardised data collection across ten European countries and compared patterns of food and nutrient intakes at home and when eating out based on single 24hDR(Reference Orfanos, Naska and Trichopoulos13, Reference Orfanos, Naska and Trichopoulou14).
Eating out, however, is often associated with occasional consumptions and it is not easy to conclude whether days that do not include any eating out reflect usual habits or not. It has been reported that the day-to-day variation in intakes, which is present in single or few short-term measurements, affects the estimation of habitual intake distributions and, thus, the percentages of individuals below or above a particular intake(Reference Carriquiry9, Reference WC15). In addition, the presence of within-person variability is expected to affect the magnitude of diet–disease associations, leading generally to attenuation of the effect. Therefore, a question arising is whether a single or two 24hDR per participant are adequate to describe the distribution of usual intakes when eating out and to examine associations between out-of-home eating and personal characteristics.
Since the collection of a large number of replicate measures is impractical and often not feasible, several statistical procedures have been developed and tested for estimating usual intakes and their distributions, based on short-term measurements. The purpose of these methods is to approximate the distribution of usual intakes by shrinking the distribution obtained from short-term measurements after removing the effect of day-to-day variation. Their application, however, requires at least one replicate measure, in order to estimate the within-person variance. Hoffmann et al. (Reference Hoffmann, Boeing and Dufour16) have evaluated several statistical procedures and recommended the use of the Nusser(Reference Nusser, Carriquiry and Dodd17, Reference Nusser, Fuller, Guenther, Lyberg, Collins, DeLeeuw, Dippo, Schwartz and Trewn18) and the simplified Nusser methods(Reference Hoffmann, Boeing and Dufour16), which guarantee in most cases that data are transformed to normality, allow adjustments for nuisance effects (for example, day of week, season) and can incorporate sampling weights. The authors argued that two non-consecutive 24hDR controlling for seasonal and day-of-week variation are adequate to satisfactorily estimate the distribution of usual daily intakes(Reference Hoffmann, Boeing and Dufour16).
The application of these statistical procedures is, however, limited in the case of zero intakes reflecting episodical consumption, which is more frequently related to eating out. To overcome this limitation, Nusser et al. (Reference Nusser, Fuller, Guenther, Lyberg, Collins, DeLeeuw, Dippo, Schwartz and Trewn18) proposed to make use of data from participants for whom information on quantities consumed is available (having declared themselves as consumers). Alternatively, Tooze et al. (Reference Tooze, Midthune and Dodd19) recommended the use of supplementary data on frequency of consumption collected from a short, non-quantitative FFQ as covariate information when modelling the data collected through at least two repeated short-term measurements. The rationale for using such a questionnaire is to assess the probability of consumption separately from the amount consumed and subsequently combine the two estimates to describe the distribution of usual intake. In comparison with the Nusser method, the approach of Tooze et al. (Reference Tooze, Midthune and Dodd19) is advanced as it uses information on zero consumption as an integral part of the overall estimation process.
A third method, the Multiple Source Method (MSM) has recently been suggested(Reference Haubrock, Nöthlings and Volatier20). Similarly to the method proposed by Tooze et al. (Reference Tooze, Midthune and Dodd19), the MSM makes use of at least two repeated short-term measurements (for example, two 24hDR) to estimate the within-person variability in intakes and a non-quantitative FFQ to record the probability of consuming a particular item on a randomly selected day. The method calculates usual intake as the product of the probability of consumption and the amount consumed on the reporting days. Unlike the Tooze et al. method, the MSM applies different data transformations and modelling and estimates usual intake distributions based on empirical rather than simulated data(Reference Haubrock, Nöthlings and Volatier20).
The present study aims at evaluating the effect of measurement error when using data from one or two 24hDR or the estimates derived after the application of the MSM in order to describe intakes when eating out and to assess associations between eating out and personal characteristics. The study used data collected in Potsdam, Germany and was undertaken in the context of the EU-supported HECTOR project (Eating Out: Habits, Determinants, and Recommendations for Consumers and the European Catering Sector; www.nut.uoa.gr/hector).
Materials and methods
Study participants
Between 1994 and 1998, more than 27 000 men and women from the general population of Potsdam, Germany, aged between 35 and 64 years, were recruited to participate in the German segment of the large international European Prospective Investigation into Cancer and Nutrition (EPIC) study(Reference Riboli and Kaaks21–Reference Bingham and Riboli25). Data on sociodemographic and lifestyle variables were assessed at baseline through self-administered questionnaires and computer-assisted personal interviews. During follow-up, participants were also asked to reply to a simplified, non-quantitative FFQ with low respondent burden(Reference Nöthlings, Hoffmann and Bergmann26).
In 2004, 5673 study participants were scheduled to receive the simplified FFQ. In the context of the FFQ validation study, a random sample of 460 participants (230 men and 230 women) were invited to provide two unannounced telephone-administered 24hDR on non-consecutive days within 1 year preceding the administration of the FFQ, with due attention to capture seasonal and day-of-week variation(Reference Haubrock, Nöthlings and Volatier20, Reference Nöthlings, Hoffmann and Bergmann26). A total of 393 individuals (85 %) accepted and completed both the two 24hDR and the simplified FFQ at the end of the observation year. For the purposes of the present study, twenty-seven participants with missing information in one or more of the variables of interest or with implausible values for total energy intake, i.e. < 1670 kJ or>25 100 kJ/d ( < 400 or>6000 kcal/d), were excluded. Thus analysis relied on 366 individuals, 186 men of mean age 58·4 (sd 8·1) years and 180 women of mean age 54·6 (sd 9·9) years.
Dietary data collection
The 24hDR were collected using the German version of the standardised EPIC-SOFT program developed at the International Agency for Research on Cancer (IARC) in collaboration with the Potsdam research centre(Reference Slimani, Deharveng and Charrondiere27, Reference Slimani, Ferrari and Ocké28). Information was collected on all food items consumed during the period between waking up on the day of recall and waking up on the following day (interview day). For the calculation of food, energy and nutrient intakes the German Food Code and nutrient database were used(29). The validated FFQ included questions on the frequency of consuming 102 items in the previous 12 months(Reference Haubrock, Nöthlings and Volatier20, Reference Nöthlings, Hoffmann and Bergmann26).
Assessment of eating out
For each eating (and drinking) occasion recalled in the two 24hDR, the place of consumption was also reported. Eating out was defined to include consumption of all foods and beverages at any location other than the household premises (restaurant, café, bar, friend's house, workplace, street, car/boat and other places). This definition has been previously used(Reference Orfanos, Naska and Trichopoulos13, Reference Orfanos, Naska and Trichopoulou14, Reference Vandevijvere, Lachat and Kolsteren30).
Information on the participants' eating-out occasions was only available through the 24hDR, since the FFQ collected data on the frequency of consumption overall, without distinguishing the frequency of consuming a food item at home from the frequency of consuming it when eating out.
Data on other personal characteristics
Participants further reported their age, weight, height and smoking status. For the purposes of the present study, BMI was used, calculated as weight (kg) divided by the square of height (m), relying on the self-reported anthropometric characteristics. With respect to smoking status, individuals were classified into three categories: never, former and current smokers.
Information on educational attainment and usual physical activity (PA) was collected at baseline (1994–1998) assuming that individuals' educational attainment had not changed and that they had retained their usual PA. The level of educational attainment was classified into four categories: none or primary school completed; technical/professional school completed; secondary school completed; and university degree. With respect to PA two variables were generated: (a) PA at work, based on the physical demand of the participant's profession and classified into three categories – none (including individuals who were not working or had retired at baseline) or sedentary, standing, and manual/heavy manual; and (b) PA at leisure, expressed as a score, based on information on the time spent on household and recreational activities(Reference James and Schofield31). Sex-specific tertiles of the estimated score were then used to label PA at leisure as low, moderate and intense.
Statistical methods
Daily intakes of energy overall, at home and when eating out and intakes of macronutrients and selected food groups (vegetables, fruit and cereals) when eating out were estimated for each of the 366 individuals.
The MSM operates in three steps: (a) a logistic regression model is initially applied to estimate the probability of consuming a food item, together with the corresponding model residuals. The residuals are then transformed to real numbers and between- and within-person variances are estimated. Within-person variance is removed and the shrunk residuals are back-transformed to the original scale; (b) a linear regression model is applied on the non-zero intake data and the model residuals are then transformed to normality by a two-parameter Box–Cox transformation family(Reference Hoffmann, Boeing and Dufour16). Similarly to the first step, the within-person variance estimated from the transformed residuals is subsequently removed and the derived shrunk quantities are back-transformed to the original scale; (c) as a final step, the ‘usual’ dietary intake values (and the corresponding distribution) are estimated by multiplying the quantities generated in steps (a) and (b) above(Reference Haubrock, Nöthlings and Volatier20).
When the MSM was applied to our data, in the above step (a) ‘out-of-home consumers’ were defined as those who reported any eating out in either of the two 24 hDR. The probability of usually consuming a food item out of home or not was estimated through a model in which the FFQ data were used as a covariate. In the above step (b), the FFQ-derived frequency data of a particular item were included as a model covariate in order to estimate the usual intake (amount) of this item when eating out. Finally (step c), usual intakes were calculated by multiplying the probability of out-of-home consumption with the estimated usual amounts. The values that were generated after the application of the ‘shrinkage’ MSM were used in the subsequent analyses.
The degree of agreement between estimates based on single 24hDR or (alternatively) the average of two 24hDR and those derived after the application of the MSM was assessed using the Bland–Altman method, in which the difference was plotted against the mean of the two methods compared. The dietary intake distributions were described with percentiles (5th–95th) and four moments (arithmetic mean and standard deviation, skewness and kurtosis). The descriptive results were derived from the analysis of data collected from (alternatively): (a) a single 24hDR (using the first of the two recalls), (b) the average of the two 24hDR and (c) the usual intake estimated through the application of the MSM using the 24hDR and FFQ data.
Linear regression models were fitted using as outcome the out-of-home energy intake estimated, alternatively: from the first chronologically 24hDR measurement (single 24hDR); from the average of the two 24hDR; or that estimated after the application of the MSM. Two models were run: model A including total energy intake as a covariate (continuously, per 2510 kJ/d) estimated from the single 24hDR, the average of the two 24hDR, or estimated after the application of the MSM, respectively; and model B without including total energy intake among the model covariates. In both models, sex, age (continuously), BMI (continuously) and personal characteristics (education, PA at work, PA at leisure and smoking status; all as independent variables ordered in the categories indicated above) were introduced.
Partial Spearman rank correlation coefficients between energy intake when eating out and (in turn) each one of the aforementioned personal characteristics, controlling for all the remaining ones, were estimated through converting continuous variables to ranks and leaving ordered/categorical variables unchanged. Confidence limits based on Fisher's z-transformation(Reference Fisher32) were subsequently estimated. All analyses were performed using SAS software version 9.1 (SAS Institute, Inc.).
Results
The characteristics of the 366 participants are presented in Table 1. Men were on average older than women and with a higher BMI. Nearly half of male participants had a university degree (48·9 %), but the percentage was lower for women (32·2 %). With respect to PA at work, 56 % of males and 63 % of females were either not working or had a sedentary job and approximately 30 % of either males or females reported professions of moderate PA. Half of the male participants (50 %) were former smokers and more than half of women (53·3 %) were never smokers.
Table 1 Characteristics of the 366 participants in the European Prospective Investigation into Cancer and Nutrition (EPIC)-Potsdam calibration study, Germany 2004 (Number of participants and percentages; mean values and standard deviations)
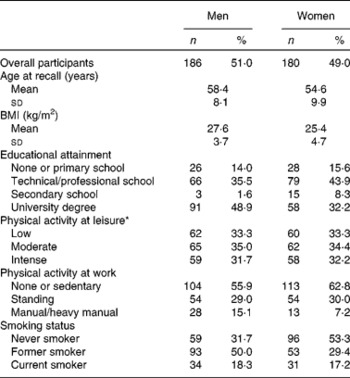
* Expressed as a score, based on information on the time spent on household and recreational activities according to James & Schofield(Reference James and Schofield31). Sex-specific tertiles of the estimated score were labelled in the three categories.
The Bland–Altman plots (Figs. 1 and 2) show the magnitude of agreement between the methods compared and spot trends within the variable (out-of-home energy intake). The mean difference (bias) between out-of-home energy intakes based on single 24hDR and those derived from the MSM was − 66·7 kJ/d (Fig. 1) and − 12·1 kJ/d between estimates based on two 24hDR and the MSM ones (Fig. 2). Both Fig. 1 and Fig. 2 indicate that for higher intakes, the application of one or two 24hDR resulted in larger estimates of out-of-home energy intake. The limits of agreement between methods (reflecting random error) are generally broad and strikingly broader in the case of the single recall in comparison with the two recalls, indicating large random variability and unveiling the limitations of short-term measurements. Fig. 1, in particular, reveals three groups of data points: (a) one group (lower left) reflecting zero daily out-of-home intakes (based on the single recall); (b) one group reflecting a slight underestimation in the lower and a more evident overestimation in the moderate intake values based on one 24hDR in comparison with the MSM (reference method). This can be appreciated by considering, for instance, that individuals with the higher/highest true out-of-home intakes may be interviewed (in the case of a single interview) for a day in which their intake was higher than their long-term average(Reference WC15); (c) lastly, one group (upper right) showing differences that increase as the intake values out-of-home increase due to heteroscedasticity. The plots suggest a restriction of the data to non-zero intakes and an appropriate transformation, in order to apply linear regression models assuming normality and homoscedasticity.
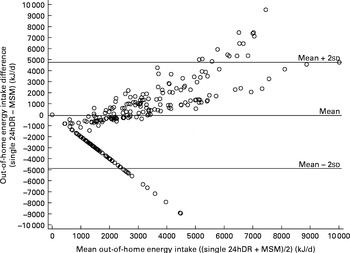
Fig. 1 Bland–Altman plot of the difference in out-of-home energy intake (kJ/d), estimated by one 24 h dietary recall (24hDR) and the Multiple Source Method (MSM) against the mean of the two methods in a sample of 366 adult participants in the European Prospective Investigation into Cancer and Nutrition (EPIC)-Potsdam calibration study, Germany 2004 (mean difference − 66·7 (sd 2407·5, 95 % limits of agreement − 4785·4, 4651·9)).
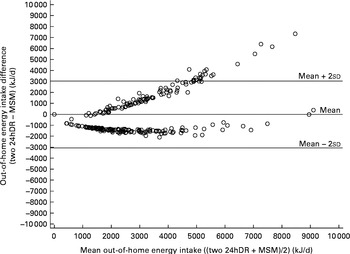
Fig. 2 Bland–Altman plot of the difference in out-of-home energy intake (kJ/d), estimated by the average of two 24 h dietary recalls (24hDR) and the Multiple Source Method (MSM) against the mean of the two methods in a sample of 366 adult participants in the European Prospective Investigation into Cancer and Nutrition (EPIC)-Potsdam calibration study, Germany 2004 (mean difference − 12·1 (sd 1526·3, 95 % limits of agreement − 3003·7, 2979·5)).
The observed energy, macronutrient and selected food (vegetables, fruits and cereals) intake distributions when eating out obtained either from a single or the average of two 24hDR are presented in Table 2, together with the corresponding usual intake distributions estimated through the application of the MSM. For energy intake only, the respective distributions overall or at home are also presented. Mean absolute values for energy, nutrient and food intakes obtained from one or two recalls were similar to the corresponding mean usual intakes. The relative differences of the mean dietary estimates based on single 24hDR from those based on the average of two recalls were generally negligible, varying from 0·3 % (vegetables) to 4·7 % (cereals). In the case of alcohol intakes, however, the mean daily out-of-home intake estimated on the basis of two recalls was nearly 30 % higher than the respective estimate from a single recall. The standard deviations decreased as the number of repeated measurements increased and this is also reflected by an increase in the lower percentiles of energy intake and a decrease in the upper ones. In the case of a single recall, in particular, the standard deviation ranged from 43 % (total energy intake) to 90 % (at-home energy intake) higher than that of the estimated usual intake. The application of the MSM resulting in a reduction of the within-person variation of the dietary data and shrinking the estimated individual intakes toward the grand mean resulted in narrower and less skewed (especially in the left tails) distributions.
Table 2 Distribution of intakes of energy (overall, at home and out of home), selected food groups (out of home) and macronutrients (out of home) by dietary assessment method in a sample of 366 adults in the European Prospective Investigation into Cancer and Nutrition (EPIC)-Potsdam calibration study, Germany 2004 (Percentiles, mean values and standard deviations, skewness and kurtosis)
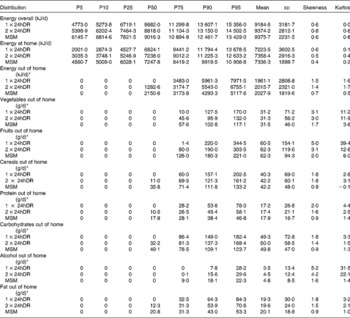
24hDR, 24 h dietary recall method; MSM, Multiple Source Method.
* Out of home is eating at places other than the household (for example, restaurant, workplace, friend's house or café).
The effect of overall energy intake and other personal characteristics on energy intake when eating out estimated from a single 24hDR, from the average of two 24hDR, or from using the MSM are presented in Table 3. When out-of-home energy intake was regressed on total energy intake (both estimated from a single recall) and other factors (model A), out-of-home energy intake was significantly positively associated with total energy intake (β = +123·5; 95 % CI +72·9,+174·2; P< 0·0001) and inversely associated with age (β = − 60·3; 95 % CI –92·3, − 28·3; P= 0·0002). When total and out-of-home energy intakes were estimated on the basis of two recalls (model A), the aforementioned associations remained, but were less attenuated and more precise. For instance, for 1 kJ/d increase in total energy intake, out-of-home energy intake increased by 158·2 kJ (95 % CI +111·1,+205·2; P< 0·0001); and, as age increased by 1 year, out-of-home energy intake decreased by 70·8 kJ (95 % CI –95·5,–46·0; P< 0·0001). In addition, a marginally significant (P = 0·045) inverse association between out-of-home energy intake and PA at work was noted. Similar were the findings when the impact of measurement error was controlled by minimising the within-person variability for both the dependent (out-of-home energy intake) and the independent (total energy intake) variables through the application of the MSM shrinkage method (model A). However, the inverse association between out-of-home energy intake and PA at work was marginally non-significant (P= 0·074), and the corresponding 95 % CI were narrower since the standard error of the estimated regression coefficients became smaller, indicating more precise estimates.
Table 3 Determinants of energy intake out of home (kJ/d) in a sample of 366 adult participants in the European Prospective Investigation into Cancer and Nutrition (EPIC)-Potsdam calibration study, Germany 2004 (Regression coefficients (β) and 95 % confidence intervals)
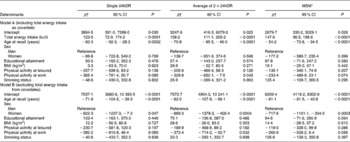
Out of home, at places other than the household (for example, restaurant, workplace, friend's house, café); 24hDR, 24 h dietary recall method; MSM, Multiple Source Method.
* In the MSM, usual intakes are estimated on the basis of the two 24hDR and information from a non-quantitative FFQ was used as covariate; non-consumers were defined as those not consuming anything out of home in any of the two 24hDR.
† Linear regression coefficients mutually adjusted for total energy intake (per 2510 kJ/d; continuous; used only in model A), age at recall (continuous), sex (men, women), educational attainment (none/primary school, technical/professional school, secondary school, university degree; ordered), BMI (continuous), physical activity at leisure (low, moderate, intense; ordered), physical activity at work (none/sedentary, standing, manual/heavy manual; ordered) and smoking status (never smoker, former smoker, current smoker; ordered).
‡ Expressed as a score, based on information on the time spent on household and recreational activities according to James & Schofield(Reference James and Schofield31). Sex-specific tertiles of the estimated score were labelled as low, moderate and intense respectively.
When total energy intake was not included in the model (model B), out-of-home energy intake estimated from a single 24hDR was again significantly inversely associated with age (β = − 71·9; 95 % CI –104·5, − 39·3; P< 0·0001). In addition, sex was found to be associated with energy intake when eating out, with women reporting on average out of home 622·3 kJ/d less than men (95 % CI –1237·3, − 7·3; P= 0·047), most likely because their average lower dietary intake was not accounted for. Both associations were strikingly more evident when two recalls were used to assess energy intake when eating out. Similarly to model A, a third statistically significant inverse association with PA at work was again detected only in the case of using the average of two 24hDR (single recall: β = − 382·2, 95 % CI − 810·8,+46·4, P= 0·080; two recalls: β = − 373·4, 95 % CI –714·2, − 32·7, P= 0·032). In both models, all significant and marginally significant associations observed after the application of the MSM had much narrower CI, indicating higher precision.
To account for zero values observed in the dependent variable (out-of home energy intake), linear regression models were subsequently applied to a subsample including individuals who reported out-of-home energy intake based on the single 24hDR (out-of-home consumers). This subsample consisted of 171 individuals, eighty-nine men and eighty-two women. The analysis on consumers only generated similar results. Nonetheless, the inverse association between out-of-home energy intake and age was no longer apparent, possibly because of the younger age of the out-of-home consumers (mean age 53·8 (sd 8·4; median 52·5) years) compared with the overall study sample. In addition, to account for the non-constant variance indicated in the Bland–Altman plots, the analysis on the 171 out-of-home consumers was repeated based on the log-transformed values of energy intakes. The aforementioned findings were again quite similar.
Table 4 presents the partial Spearman rank correlation coefficients of out-of-home energy intake with total energy intake and other personal characteristics. The findings were similar to those obtained from the estimated regression coefficients (Table 3). The magnitude of the associations of out-of-home intake with both total energy intake (r+0·27; P< 0·0001) and age (r –0·35; P< 0·0001) was stronger (higher absolute coefficients) when analysing data based on the average of the two 24hDR, rather than on the MSM as well as on a single recall.
Table 4 Correlations of energy intake out of home with each of the indicated variables in a sample of 366 adult participants in the European Prospective Investigation into Cancer and Nutrition (EPIC)-Potsdam calibration study, Germany 2004 (Partial Spearman rank correlation coefficients (r) and 95 % confidence intervals)
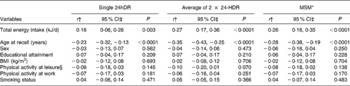
Out of home, at places other than the household (for example, restaurant, workplace, friend's house, cafeteria); 24hDR, 24 h dietary recall method; MSM, Multiple Source Method.
* In the MSM, usual intakes are estimated on the basis of the two 24hDR and information from a non-quantitative FFQ was used as covariate; non-consumers were defined as those not consuming anything out of home in any of the two 24hDR.
† Correlation coefficient between energy intake out of home and each of the corresponding indicated variables: total energy intake (continuous), age at recall (continuous), sex (men, women), educational attainment (none/primary school, technical/professional school, secondary school, university degree; ordered), BMI (continuous), physical activity at leisure (low, moderate, intense; ordered), physical activity at work (none/sedentary, standing, manual/heavy manual; ordered) and smoking status (never smoker, former smoker, current smoker; ordered).
‡ 95 % CI calculation is based on Fisher's z- transformation of the Spearman partial rank correlation coefficient.
§ Expressed as a score, based on information on the time spent on household and recreational activities according to James & Schofield(Reference James and Schofield31). Sex-specific tertiles of the estimated score were labelled as low, moderate and intense respectively.
Discussion
We have analysed data collected in a sample of 366 adult participants of the European Prospective Investigation into Cancer and Nutrition (EPIC)-Potsdam calibration study and we found that the mean daily dietary intakes when eating out estimated from one or two 24hDR were similar to the corresponding usual (‘true’) intakes estimated through the application of the MSM approach(Reference Haubrock, Nöthlings and Volatier20). Mean values were not systematically affected since between-person error implies that an overestimation for some individuals is counterbalanced by an underestimation for others. As expected, however, standard deviations decreased as the number of recalls increased from one to two, indicating that short-term dietary measurements with high within-person error, like a single 24hDR, may not be adequate to accommodate within-person variation(Reference WC15). The exaggerated standard deviations caused wider distributions with a large amount of zero intakes, even leading to zero median values when single 24hDR were analysed. Such measurement error may result in overestimating the prevalence of high or low intakes in a population and therefore identifying the percentage of individuals who are below or above a recommended intake value.
We have also relied on empirical data collected in 2004, in order to compare different estimation methods and assess the potential effect of measurement error when identifying associations of eating out with personal characteristics. Hence, results were presented to primarily highlight differences between methods and to a smaller extent describe the public health implications of our findings. Irrespective to the method used (one or two 24hDR) the daily out-of-home energy intake was found to be positively associated with the total energy intake and the associations remained when usual out-of-home energy intake estimated through the MSM was alternatively used in the models (model A). Similarly, out-of-home eating was consistently inversely associated with age. The present study population, however, is relatively old and the generalisability of this finding to younger adults (aged less than 35 years) may be questionable. A marginally significant inverse association between out-of-home energy intake estimated on the basis of two 24hDR and PA at work was observed (P= 0·045). The association remained inverse, but was marginally non-significant, when estimates of energy intake were based on a single 24hDR (P= 0·085) or when usual energy intake was estimated through the MSM (P= 0·074). The direction of associations between out-of-home energy intake and personal characteristics was similar irrespective of the method used. As expected, however, they were less precise (that is, wider CI) when estimates were based on a single recall as compared with two recalls or obtained through the MSM (with reduced within-person variance). Our findings suggest that data from a single or two 24hDR may be adequate to evaluate associations between out-of-home intake and personal characteristics.
In our analysis we used energy intake to reflect out-of-home (or overall) eating, since for energy intake the ratio of within- to between-person variance is smaller than that for macronutrients. As Beaton et al. (Reference Beaton, Milner and Corey33) have illustrated, this ratio tends to be greater than 1 for most macronutrients since the within-person variance is generally much higher than the respective between-person variance and the use of few short-term measurements has more serious consequences.
Total energy intake, one of the explanatory variables in the regression models, was also measured with error. The concurrent use of ‘imperfect’ variables as outcome and predictor into the same model or the possible confounding between the covariates (including the ‘imperfect’ variable), might unpredictably affect the associations toward any direction. To investigate this, we re-ran the models excluding total energy intake. The associations between out-of-home energy intake and age or PA at work remained and a new association between eating out and sex emerged, possibly because overall energy intake is generally lower among women than among men: women were found to acquire less energy than men when eating at places other than the household premises.
The present study made use of a variable to reflect eating out, which follows an empirical distribution dominated by zero values. To reduce the problem of inflated-zero distributions – beyond the apparent collection of data for more days – we repeated the analysis based on the non-zero values of the corresponding variable (i.e. restricting the sample to those having declared themselves as out-of-home consumers) and results were generally unaffected. The associations did not differ even when we compared the three methods after we had log-transformed the values to account for the non-constant variance and high random variability depicted in the corresponding Bland–Altman plots.
The MSM has been constructed to record the overall frequency of dietary intakes without separating the at-home from the out-of-home one. In our analysis, we assumed that the overall frequency of consuming a specific food item (recorded through a non-quantitative FFQ) also reflects the probability of usually eating this item out of home or the corresponding amount consumed. This assumption may or may not hold true in all instances and future analyses focusing on eating out could benefit from also recording the out-of-home frequency of consumption.
The points discussed so far rely on the assumption that within-person variability of reported intakes is random. Such an assumption is, however, difficult to hold as 24-HDR generally suffer from under-reporting, suggesting the presence of some systematic errors(Reference Ferrari, Slimani and Ciampi34), although focusing on a single day the magnitude of systematic errors is reduced(Reference WC15, Reference Beaton, Milner and McGuire35, Reference Dodd, Guenther and Freedman36). In the present study where the focus is on distinguishing between eating at home or out of home, systematic errors are likely to be less important. Nevertheless, these effects can be reduced by excluding under- and over-reporters(Reference Mackerras and Rutishauser10). Another key assumption when assessing random errors is that such errors must be uncorrelated among the replicate measurements within the same individual. This assumption may not hold when the sampling days are consecutive, but is less likely to be violated in the case of non-consecutive days(Reference WC15, Reference el Lozy37, Reference Kaaks, Ferrari and Ciampi38). Therefore sampling of non-consecutive days covering all days of week and seasons should be preferred(Reference Hoffmann, Boeing and Dufour16), as was done in the present study.
Short-term measurements of out-of-home intakes suffer from measurement error due to the daily variation of the individual food and nutrient consumption. The error in measurement, particularly in the independent variables (i.e. total energy intake), together with confounding are the two limitations that should receive considerable attention and should not be ignored from relevant analyses(Reference Blair, Stewart and Lubin39). The within-person variability should be minimised in order to estimate the usual out-of-home distribution. In a standard setting (random sampling of non-consecutive days from different seasons), data from a single and particularly from two 24hDR may be adequate to estimate average out-of-home intakes and investigate associations between dietary intake out of home and various individual characteristics. Still and most importantly, since within-person variability is generally expected to reduce the strength of associations it is unlikely that associations, which do not exist, appear as significant. Although data on eating out collected from a single recall may not be enough to detect significant associations due to the weakening of regression coefficients, they could provide a realistic insight of possibly true associations. The apparent abundance, however, of zero values in the corresponding variables should be treated with caution. We agree with Jurek et al. (Reference Jurek, Greenland and Maldonado40) that results should be presented in a very cautious manner, rather than promoted by unfounded judgments that biases are small or errors are in a known direction. Even a descriptive approach to presenting results may be commendable and need not detract from the scientific value of a research report(Reference Jurek, Greenland and Maldonado40, Reference Greenland, Gago-Domiguez and Castellao41).
Acknowledgements
The present study was conducted in the context of the HECTOR project entitled ‘Eating Out: Habits, Determinants, and Recommendations for Consumers and the European Catering Sector’ funded in the FP6 framework of DG-RESEARCH in the European Commission. The original study providing the data used in the present analysis was performed in accordance with the principles of the Helsinki Declaration and received ethical approval from the Ethical Committee of the State of Brandenburg, Germany. All participants provided their informed consent before enrolment. The authors are solely responsible for the contents of the paper.
The opinions expressed do not represent the opinions of the Commission and the Commission is not responsible for any use that might be made of the information included.
The MSM was developed by our German colleague Dr Kurt Hoffmann who unexpectedly passed away in 2007. All authors and colleagues thank him for his valuable research contribution.
The authors' contributions are as follows: P. O., A. N., A. T. and H. B. designed the present study. P. O. conducted the study. S. K., J. H. and P. O. performed the statistical analyses. P. O., A. N. and A. T. drafted the paper and all authors contributed in the development of the submitted manuscript. A. T. and H. B. have the primary responsibility for the manuscript's final content. A. T. was the coordinator of the HECTOR project.
The authors declare that there is no conflict of interest.