1 Introduction
Multilingual speakers are able to switch from one language to the other (“code-switch”) between or within utterances. For instance, a Spanish–English speaker might produce a sentence such as “Los niños están playing in the front yard” (“The kids are playing in the front yard”). Code-switching has been studied for decades by theoretical linguists and sociolinguists (e.g., Bullock & Toribio, Reference Bullock and Toribio2009; Lipski, Reference Lipski and Paradis1978; MacSwan, Reference MacSwan2014; Muysken, Reference Muysken2000; Poplack, Reference Poplack1980) and more recently by researchers from other domains such as psycholinguistics (e.g., Dussias, Reference Dussias2003; Fernandez, Litcofsky & van Hell, Reference Fernandez, Litcofsky and van Hell2019; Guzzardo Tamargo, Kroff & Dussias, Reference Guzzardo Tamargo, Kroff and Dussias2016; Isurin, Winford & De Bot, Reference Isurin, Winford and De Bot2009; Litcofsky & Van Hell, Reference Litcofsky and Van Hell2017; Toribio, Reference Toribio2001). These studies have revealed that code-switches do not occur randomly but follow systematic patterns. For instance, as early as in the 1970s, Timm (Reference Timm1975) argued that Spanish–English bilingual speakers do not switch between the subject or object pronoun and the verb (as in “ellos play” or “they juegan”).
The aim of the current work is twofold. First, we present a novel method of researching code-switched sentence production using computational cognitive modeling. To that end, we employ the Bilingual Dual-path model (Tsoukala et al., Reference Tsoukala, Frank, Broersma, Gunzelmann, Howes and & E2017) that can produce sentences in two languages, including code-switched ones. Second, using this method, we shed light on a production phenomenon that has been observed in the Spanish–English-speaking community in the US and is known as the “auxiliary phrase asymmetry” (Dussias, Reference Dussias2003; Guzzardo Tamargo et al., Reference Guzzardo Tamargo, Kroff and Dussias2016; Lipski, Reference Lipski and Paradis1978; Pfaff, Reference Pfaff1979; Poplack, Reference Poplack1980). This asymmetry is observed in the frequency of Spanish-to-English code-switches at the participle in progressive and perfect structures. On the one hand, Spanish–English bilinguals rarely produce a code-switch between the Spanish auxiliary haber (“to have”) and the participle. On the other hand, they are likely, albeit only moderately so, to code-switch in the progressive structure between the Spanish auxiliary verb estar (“to be”) and the participle. Thus whereas Sentence 1 is attested, Sentence 2 is very infrequent and dispreferred:
1. Las personas están voting (The people are voting)
2. Las personas han voted (The people have voted)
Furthermore, a switch at the auxiliary (i.e., the first word that is switched is the auxiliary) is approximately equally likely for both structures: “Las personas are voting”, “Las personas have voted”.
The auxiliary phrase asymmetry has been found both in speech production and in reading. With respect to speech production, several quantitative analyses of Spanish–English corpora have reported code-switches at the participle in progressive sentences but none in perfect sentences (Lipski, Reference Lipski and Paradis1978; Muysken, Reference Muysken2000; Pfaff, Reference Pfaff1979; Poplack, Reference Poplack1980). Guzzardo Tamargo et al. (Reference Guzzardo Tamargo, Kroff and Dussias2016) performed a systematic analysis on two corpora: the Miami corpus (Deuchar, Davies, Herring, Couto & Carter, Reference Deuchar, Davies, Herring, Couto, Carter, Thomas and Mennen2014) that contains spontaneous conversations, and a corpus extracted by Guzzardo Tamargo et al. (Reference Guzzardo Tamargo, Kroff and Dussias2016) from entries of an online column in a Gibraltarian newspaper (“Gibraltar corpus”), which contains fictional code-switched written dialogue. As Table 1 indicates, although switches are infrequent in either structure, the asymmetry is reported in both corpora.
Table 1. Absolute and relative frequencies in the Miami and Gibraltar corpora. Values reported in Guzzardo Tamargo et al. (Reference Guzzardo Tamargo, Kroff and Dussias2016)

As Dussias (Reference Dussias2003) pointed out, because a switch within the auxiliary verb phrase is not a common phenomenon to begin with, it is difficult to obtain sufficient evidence from corpus data to establish whether the reported asymmetry is real. To resolve that, she performed an eye-tracking-while-reading study; she asked English–Spanish bilinguals to read Spanish-to-English code-switched sentences that i) were either in the progressive or perfect structure, and that ii) contained a switch either at the auxiliary (e.g., “La madre sabe que los chicos are going to the park”; English translation: “The mother knows that the children are going to the park”) or at the participle (“La madre sabe que los chicos están going to the park”). The analysis showed that for the perfect-form structures, participants took significantly longer to read switches at the participle compared to switches at the auxiliary, whereas for the progressive-form structures they did not show a significant preference for a switch position. Dussias concluded that auxiliary verb phrase switches are processed differently depending on the structure.
Guzzardo Tamargo et al. (Reference Guzzardo Tamargo, Kroff and Dussias2016) also ran an eye-tracking-while-reading study in which they confirmed the results reported in the previous study (Dussias, Reference Dussias2003). Furthermore, Giancaspro (Reference Giancaspro2015) found evidence for the auxiliary asymmetry from an acceptability judgment study. Specifically, he asked English–Spanish bilinguals to rate the grammaticality of code-switches; the participants rejected perfect participle switches and accepted progressive participle ones, thus supporting the asymmetry.
Two (non-mutually-exclusive) explanations have been proposed for this phenomenon; the “grammaticalization account” and the “exposure-based account” (Guzzardo Tamargo et al., Reference Guzzardo Tamargo, Kroff and Dussias2016). According to the grammaticalization account, the source of this asymmetry is the difference in semantic weight between the auxiliary verbs. Namely, that estar has more semantic weight and is syntactically more independent as it also functions as a linking verb (e.g., “el enfermero está cansado”; “the nurse is tired”), whereas haber is highly grammaticalized because it is almost exclusively used as an auxiliary. The verb of possession in Spanish is tener (“el enfermero tiene un libro”; “the nurse has a book”), while haber is only used as an auxiliary verb or in archaic formulations. The exposure-based account is an alternative hypothesis, which was suggested, but not attested, by Guzzardo Tamargo et al.; it states that the asymmetry emerges from community-supported practice: that is, bilingual speakers learn the asymmetry from exposure to this pattern in the community. In this study we focus on the grammaticalization account to determine whether grammaticalization is a plausible reason why the asymmetry emerged. In human bilinguals, exposure also plays a role, as experience with the language influences production patterns (e.g., MacDonald, Reference MacDonald2013).
The grammaticalization account is difficult to test experimentally with psycholinguistic methods, especially in production. Common experimental paradigms for production studies, such as shadowing (where participants repeat stimuli as quickly as possible, e.g., Lipski, Reference Lipski2019) or confederate priming (in which one of the participants is in fact a confederate with a script, who provides primes for the participant, e.g., Kootstra, Van Hell & Dijkstra, Reference Kootstra, Van Hell and Dijkstra2010), could perhaps confirm the presence of the auxiliary asymmetry. However, to test the grammaticalization account in production, which states the asymmetry is caused by the lack of semantic weight of the Spanish auxiliary verb haber, we need to know whether the asymmetry would persist if haber did have additional syntactic and semantic functions and was used more frequently, as in the case of the (main and auxiliary) English verb “to have”. It is difficult to envision a traditional technique that can test explicitly the role of semantic function of the Spanish auxiliary verb. One could potentially employ artificial language learning that mimics the acquisition and production of code-switched Spanish and English progressive- and perfect-forms. However, this would require a complex setting which would be very challenging for the participants as it would entail advanced learning of the two artificial languages.
Computational cognitive modeling, on the other hand, allows us to make modifications to the vocabularies and the language structures of the modeled languages while keeping everything else the same, thus enabling us to focus on the phenomenon of interest. In this study, we will showcase how we can use computational modeling to add and remove semantic weight from the Spanish auxiliary verb, thus investigating whether the asymmetry could be derived from the properties of Spanish and English. For that reason, we have employed the Bilingual Dual-path model (Tsoukala, Frank & Broersma, Reference Tsoukala, Frank, Broersma, Gunzelmann, Howes and & E2017), a connectionist model of bilingual sentence production that produces code-switches (see Section 3 for an explanation of the model).
The model is not exposed to any code-switched sentences; it is only trained on English sentences and Spanish sentences. Therefore, if this particular asymmetry emerges in the model it will be due to the distributional patterns of the two languages (as claimed by the grammaticalization account), in interaction with the properties of the model, and not because of exposure to the asymmetry (i.e., exposure-based account). Such a result would show that the distributional patterns are, in principle, sufficient to lead to the asymmetry.
To investigate whether the asymmetry can emerge in the model and to test the grammaticalization account, we run three sets of simulations. First, we simulate the production of participle switches for the progressive and perfect structures; we hypothesize that this simulation will produce more participle switches in the progressive structure than the perfect one, thus exhibiting the asymmetry. Second, we hypothesize that artificially adding semantic weight to the Spanish auxiliary verb will make the asymmetry disappear because it is caused by the lack of semantic weight of haber. We explicitly test this in the second set of simulations by using the Spanish main verb tener (“to have”) also as an auxiliary verb. Third, we aim to test whether the asymmetry exists due to the relatively low occurrence frequency of haber. Namely, because haber only functions as an auxiliary verb for the perfect-form sentences, it has lower frequency than the three other auxiliary verbs (be, have, estar) that are also used as independent verbs. In the third simulation we correct for this confound.
2 Method
2.1 Obtaining corpus frequencies
As the occurrence frequency of the two structures of interest could influence the asymmetry, we made sure that we used realistic relative percentages of the progressive and perfect structures for each of the two languages in the Bilingual Dual-path model. To achieve that, we ran a corpus analysis on a Spanish–English corpus. We analyzed the transcriptions of the Bangor Miami corpus (Deuchar et al., Reference Deuchar, Davies, Herring, Couto, Carter, Thomas and Mennen2014)Footnote 1 that consists of 30 hours of spontaneous and informal conversations between two or more speakers (84 speakers in total), living in Miami, Florida.
For each of the 56 conversations, we separated the sentences into English only, Spanish only, and code-switched. The corpus is predominantly English. There are 27,835 fully English sentences (61.5% of the whole corpus), 14,631 fully Spanish sentences (32.3% of the corpus), and 2,823 code-switched sentences (6.2% of the corpus).
First, we extracted i) the progressive-form sentences that contain the verb “to be” in the third singular person (“is” for English sentences and “está” for Spanish) followed by a present-tense participle, or by an adverb and a present-tense participle, and ii) the perfect-form sentences that contain the third singular person of the verb “to have” (“has” for English, “ha” for Spanish) followed by an optional adverb and a past-tense participle. From the English sentence candidates that were selected for the progressive form, we excluded the ones that were used to indicate the future form (“is gonna” and “is going to” followed by a verb, e.g., “it is going to rain”). Then, we inspected each extracted sentence manually and further excluded the ones that had been mislabeled (e.g., “disgusting” in “is disgusting” was marked as a participle, not a (participial) adjective, and is therefore not a progressive-form sentence). The results are shown in Table 2: for English, the progressive form is about twice as frequent as the perfect form, whereas for Spanish the two forms are more balanced. Furthermore, the simple form is considerably more frequent than the progressive and perfect ones for both languages.
Table 2. Absolute frequencies per language in the Miami corpus

There are enough sentences of each type in the corpus to obtain a somewhat reliable estimate of their frequencies. Therefore, these percentages will be used to generate the corresponding structures of the languages that the model learns (see Section 2.3).
2.2 Bilingual Dual-path model
Model architecture
The Bilingual Dual-path modelFootnote 2 (Figure 1; Tsoukala et al., Reference Tsoukala, Frank, Broersma, Gunzelmann, Howes and & E2017) is an extension of the Dual-path model (Chang, Reference Chang2002) of monolingual sentence productionFootnote 3. The model is called Dual-path because of its two pathways that influence sentence production: the syntactic path that learns to abstract the syntactic patterns of a language, and the semantic path that receives event semantic information and learns to map concepts onto words. It is a computational cognitive model based on the Simple Recurrent Neural Network architecture (Elman, Reference Elman1990), and it learns to produce sentences given a message to be expressed (see Section 2.3 for an explanation of messages and for an example of how a message is given and is then expressed as a sentence).

Fig. 1. The Bilingual Dual-path model generates sentences word-by-word that express a given message (see Section 2.3 for examples of messages). It is based on a Simple Recurrent Network architecture (the syntactic stream, via the ‘compress’ layers) that is augmented with a semantic stream (upper path) that contains information about concepts and their realization, thematic roles, event semantics, and the target language. The numbers in the parentheses indicate the size of each layer (e.g., 52 concept units); the sizes of the hidden and compress layers vary with each model run (see Section 2.4). The solid arrows denote connections with weights that change during training, whereas the lines between roles, realization, and concepts correspond to connections that are given as part of a message-to-be-expressed (e.g., the AGENT is connected to WOMAN in a particular message). The dotted arrow indicates that once a word is produced, it is given back as input thus contributing to the production of the next word.
We chose to work on and extend the monolingual Dual-path model because the architecture has been employed to explain a wide range of phenomena: for example, structural priming in English (Chang, Dell & Bock, Reference Chang, Dell and Bock2006) and German (Chang, Baumann, Pappert & Fitz, Reference Chang, Baumann, Pappert and Fitz2015), and cross-linguistic differences between English and Japanese (Chang, Reference Chang2009). Importantly, previous studies using the Dual-path model have focused on semantic weight effects; Chang (Reference Chang2002) simulated different types of aphasia by testing the effect of syntactic-path or semantic-path lesions on production of words with heavy vs no semantic weight (e.g., content words versus function words, heavy verbs vs light verbs).
Sentence production
As mentioned above, the model generates sentences that express a given message. To express a message, the following items are provided to the model and influence production: the to-be-expressed semantic roles (e.g., ‘AGENT’, ‘ACTION’) are connected to their concepts (e.g., ‘DOG’, ‘SWIM’) and realizations (e.g., ‘INDEF’ for an indefinite article). The relevant “event semantics” (EVENT-SEM, e.g., ‘PRESENT’, ‘PROGRESSIVE’) and “target language” (‘ENGLISH’, ‘SPANISH’) units are activated. For instance, the model learns to express the message “AGENT=DOG, DEF; ACTION=SWIM; EVENT-SEM=PRESENT, PROGRESSIVE” in English as “the dog is swimming.” and in Spanish as “el perro está nadando.”Footnote 4. The language units are included as a means to exert language control: a single language is activated in monolingual contexts and both languages are activated in bilingual contexts.
When the model is given a message, it produces a sentence one word at a time; the produced word (“output”) is considered the one with the highest output activation. Each output word is subsequently provided as input in the next time step, and it contributes to the next word production.
Correctness of produced sentences
A produced sentence is regarded as correct if it is grammatical and conveys the target meaning. In some cases, a sentence could be grammatical but incorrect. For instance, if the target sentence is “the mother is pushing a toy” it is grammatically correct to produce the sentence “the tired mother is pushing a toy”, even if the meaning is counted as incorrect because of the extra information that was expressed. The same applies to incomplete semantics (“the mother” instead of “the tired mother”); the sentence is counted as incorrect but grammatical.
Code-switching
To allow the model to produce in either language or to code-switch, when testing the model we manipulated the model's language control. Specifically, because we were interested in the Spanish-to-English switch direction, we activated the Spanish language at the beginning, before the production of the first word, so as to indicate the conversational settingFootnote 5 (intended language) and to bias towards the production of Spanish utterances. Immediately after the first word was produced, we activated both target language nodes, thus allowing the model to continue in the same language or to code-switch.
2.3 Miniature Languages
The sentences that the model learns to produce are derived from miniature versions of natural languages. As we are studying the auxiliary phrase asymmetry that is observed in Spanish–English bilinguals, we focus on Spanish and English sentence production. Hence, we generated sentences based on the relevant properties of the two languages, constrained by the corpus analysis (Section 2.1). The advantage of using artificial (miniature) languages is that we can manipulate their structural frequencies, and even grammar, which in turn allows us to isolate and study the phenomenon of interest. For instance, in the case of the auxiliary phrase asymmetry, we can change the frequency and semantic weight of the Spanish auxiliary verb haber and see whether the asymmetry persists (see Section 3 for an explanation of this process).
Bilingual lexicon
The lexicon consists of 200 lexical items (Table 3): 91 English words, 108 Spanish words, and the shared period (‘.’) which indicates the end of the sentence. The Spanish lexicon is larger because Spanish is a gendered language. For instance, the adjective ‘tall’ is either ‘alto’, if it modifies a masculine noun, or ‘alta’ for a feminine noun. We also used four common-gendered Spanish adjectives such as ‘inteligente’ (‘intelligent’) that do not change depending on the noun they modify. Note that syntactic category information (such as ‘adjective’, ‘verb’) is not given explicitly; the model learns during training that words of the same syntactic category occur in similar contexts.
Table 3. Parts of speech (POS) in bilingual lexicon (Spanish in italics)

1 Both overlap with the auxiliary verbs.
2 Nine of these have the same form as a verb; e.g., ‘walked’ is either a perfect participle or a verb.
Structures
For the two languages we used the present and past tense, and three aspects: simple, progressive, and perfect. The past tense is only used in simple aspect sentences (e.g., “the man swam”) whereas the present tense applies to all three aspects. The allowed structures for the two languages and all tenses and aspects are Subject - Verb (SV) and Subject - Verb - Object (SVO) (Table 4).
Table 4. Allowed structures for English and Spanish
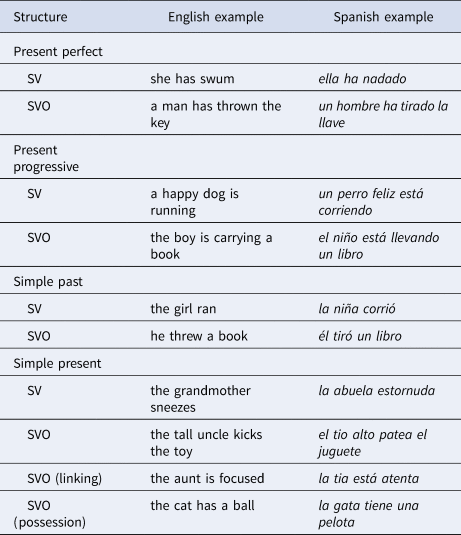
The grammatical roles can be expressed using either a Noun Phrase (NP) with definite (DEF) or indefinite (INDEF) article (e.g., ‘the woman’, ‘a woman’). Additionally, the subject can be expressed with a pronoun (PRON, e.g., ‘she’). NPs optionally contain a modifier (an adjective, e.g., ‘a tall woman’). Note that in English the adjective comes before the noun (“the intelligent woman”) whereas in Spanish the modifier comes after the noun (“la mujer inteligente”). As mentioned above, the model learns all this through the training examples and not through explicit syntactic labels.
The verbs are either intransitive (e.g., ‘swims’), transitive (‘carries’), linking (‘is’, ‘está’) or possession verb (‘has’, ‘tiene’). The two linking verbs (‘is’, ‘está’Footnote 6) and the English possession verb (‘has’) were also used as auxiliary verbs for the progressive and perfect forms respectively. As mentioned before, the Spanish perfect-form auxiliary verb is haber (‘ha’ in the 3rd person singular form), which does not function as a main verb. Following the allowed structures, each verb had four forms: simple present, simple past, present participle and past participle.
Messages
The goal of the model is to express a specified message using a grammatical sentence, such as one of the ones described above. A message is represented by (a) a target language, (b) event-semantic information, (c) pairs of thematic roles and concepts, and (d) pairs of thematic roles and realizations (pronoun, definite, indefinite) whenever applicable in the case of noun phrases.
The target languages are ENGLISH and SPANISH. The event semantics contain information regarding the aspect (SIMPLE, PROGRESSIVE, PERFECT) and tense (PRESENT, PAST), as well as the thematic roles that are used in each message.
The following simulations make use of 52 unique concepts and five thematic roles: AGENT, AGENT-MODIFIER, PATIENT, ACTION-LINKING, and ATTRIBUTE. The AGENT is only paired with animate nouns. ACTION-LINKING is a combined thematic role that can be used for all main verb types: action (e.g., ‘shows’), linking (‘is’) and possession (‘has’). ATTRIBUTE is an attribute expressed only with a linking verb.
Additionally, AGENT and PATIENT are not only connected to concepts but also to their realization: pronoun (e.g., ‘he’ for the concept MAN), and definite or indefinite article for concepts that are expressed as a noun phrase (e.g., ‘the man’ or ‘a man’ respectively).
Message-sentence pair examples
To incorporate and illustrate all the information given above (Lexicon, Structures, and Messages), here is an example of how a message is expressed as a sentence:
-
AGENT=WOMAN, INDEF
-
AGENT-MOD=TALL
-
ACTION-LINKING=CARRY
-
PATIENT=BOOK, INDEF
-
EVENT-SEM=PRESENT, PERFECT, AGENT, AGENT-MOD, PATIENT
The corresponding sentences in English and Spanish are:
a tall woman has carried a book
una mujer alta ha llevado un libro (word-by-word translation: “a woman tall has carried a book”)
If the aspect was PROGRESSIVE instead of PERFECT, the corresponding sentences would be “a tall woman is carrying a book”; “una mujer alta está llevando un libro”. Similarly, for the SIMPLE aspect, the corresponding sentences are “a tall woman carries a book”; “una mujer alta lleva un libro”.
Linking verb messages are encoded using an attribute:
-
AGENT=GIRL, DEF
-
ACTION-LINKING=BE
-
ATTRIBUTE=TIRED
-
EVENT-SEM=SIMPLE, PRESENT, AGENT, ATTRIBUTE
and expressed as “the girl is tired” or “la niña está cansada”, depending on the target language.
A message with a possession verb is encoded similarly to a message with a transitive verb:
-
AGENT=GRANDFATHER, DEF
-
AGENT-MOD=SHORT
-
ACTION-LINKING=HAS
-
PATIENT=CHAIR, INDEF
-
EVENT-SEM=SIMPLE, PRESENT, AGENT, AGENT-MOD, PATIENT
The preceding message would be expressed as “the short grandfather has a chair” and “el abuelo bajo tiene una silla”.
Note that auxiliary verbs do not have an explicit concept and are not assigned to a thematic role (e.g., for “has carried” the ACTION-LINKING is CARRY). When “have” (or “tiene”) is used as a possessive verb, as in the example above, the ACTION-LINKING is HAS which indicates that the verb has a semantic property. The Spanish auxiliary verb haber does not function as a main verb and, therefore, has no semantic weight in the model: it is never connected to ACTION-LINKING. This is in contrast to the verbs “have”, “is”, “está”, which have semantic weight (through a connection to ACTION-LINKING) when used as main verbs.
2.4 Model training
Connectionist models have trainable connection weights that are adapted during the learning process. During training, the network sees examples (targets) of messages and sentences (Section 2.3). Before training, the network produces random words (e.g., “cat cat cat”). After each word has been produced, the model receives feedback as to whether the word was correct or not, and the connections change their weights depending on the mismatch between the produced and the target word. Gradually, through exposure to the examples, the model learns to successfully express a message using the corresponding sentences.
A set of training examples always contained 2,000 message-sentence pairs. All three simulations (see Section 3) were trained for 40 epochs, where each epoch corresponds to one pass through the training set. The connection weights were updated using the backpropagation algorithm after each output word.Footnote 7
A number of random factors influence the message-to-sentence production and the overall performance of the model. In order to minimize the risk of choosing parameters that are either too specific (i.e., resulting in an effect that does not generalize) or improper (i.e., causing failure or low performance in the overall sentence production), we decided to train several networks per simulation. Specifically, for each simulation we trained 60 different networks (model runs) for 40 epochs while randomizing all free parameters per network, as explained below, except the training set size and backpropagation parameters.
The target message-sentence pairs (see Section 2.3 for examples of messages) are randomly generated before the training starts, and the sentences are constrained by the set of allowed structures (Section 2.3). For each part of speech (POS) a randomly selected lexical item (from that POS and target language) is sampled from the bilingual lexicon (Section 2.3).
As mentioned in the section on corpus analysis (Section 2.1), the simple tense occurs considerably more frequently than the progressive and perfect-form constructions. Since in the current simulations we are mainly interested in the latter two forms, to ensure the model encounters these forms sufficiently we increased their percentage in the training set by downsampling the simple form. At the same time, we made sure to keep the relative frequencies of the progressive and perfect forms intact. The percentages used to produce structures in the model are shown in Table 5.
Table 5. Structure frequencies in the model training sentences

Additionally, the percentage of English and Spanish varied slightly: the goal was to simulate balanced bilinguals, but as it is very unlikely that a human bilingual receives truly balanced input, we sampled the percentage of English using a normal distribution with a mean of 50% and a standard deviation of 8, the rest being Spanish. Importantly, the target sentences were never code-switched.
Furthermore, the network's connection weights were randomly initialized from a normal distribution centered at zero, and the (non-trainable) weights of the connections between the thematic roles and the concepts (‘concept’–‘role’ and ‘predicted role’–‘predicted concept’, see Figure 1) were integer values that were sampled for each simulation between the values of 10 and 20, whereas the unused roles and concepts are not connected. These connections are not trained. The size of the hidden layer was also random, between 90 and 110 units, and the size of the compress layer was set to roughly 77% of the size of the hidden layer.
3 Simulations
To test the grammaticalization account of the auxiliary phrase asymmetry, we ran three sets of simulations, consisting of 60 model runs each.
3.1 Simulation 1: “haber model”
In the first set of simulations, we tested whether the auxiliary phrase asymmetry can emerge only from the distributional patterns of the two languages, which would indicate that exposure to the asymmetry is not necessary to explain the phenomenon. To test that, we trained the model (“haber model”) on 2,000 sentence-message pairs using the generated examples described in Section 2.3 that contain progressive-, perfect- and simple-tense sentences. We then tested it on 700 novel messages that had Spanish as a target language. Only Spanish messages are included because we are interested in Spanish-to-English code-switches; therefore, we activated the Spanish language unit until the first word was produced, after which both languages were activated, allowing the model to continue in the same language or to code-switch. Of these 700 messages, 350 had a PROGRESSIVE aspect (e.g., “the boy is kicking a ball”) and 350 were the PERFECT-form equivalent of those sentences (“the boy has kicked a ball”). We hypothesized that the model would display the auxiliary phrase asymmetry even though the phenomenon was not present in the training data; as mentioned before, the model was not exposed to any code-switched sentences during training.
3.2 Simulation 2: “tener model”
In the second simulation we tested explicitly whether adding semantic weight to the Spanish auxiliary verb (i.e., haber) would diminish the asymmetry. This was done by taking advantage of the fact that the model's training set is generated and can therefore be manipulated. To increase the semantic weight of the Spanish auxiliary verb, we modified the Spanish main verb (tener) of the model to function both as a main verb and an auxiliary (i.e., similar to English, which uses the verb “to have” both as a main verb and as an auxiliary verb: “the boy has a dog”; “the boy has left”). More specifically, we trained the model (“tener model”) with the same training examples as in the “haber model” simulations, with the only difference that we replaced all instances of haber with tener. For instance, “el niño ha comido” (i.e., “the boy has eaten”) became “el niño tiene comido”. We kept everything else the same as in the “haber model” (the 700 test messages, initialized weights, all the layer sizes, and even the lexicon size, even though haber was no longer used, were identical), and we ran 60 networks using the modified target sentences. Because in this simulation tener is used both as an independent main verb with semantic content and as an auxiliary verb, we hypothesized that this model would not show the asymmetry.
3.3 Simulation 3: “synonym model”
Finally, we ran a third simulation to control for the frequency increase of tener which was caused by using it also as an auxiliary verb in the previous model compared to the first model. To make sure that a potential disappearance of the asymmetry in the “tener model” is not simply due to the increase of exposure of tener, we made haber and tener perfect synonyms (“synonym model”). Either could be used as a main verb or an auxiliary verb, whereas we kept the frequency of each verb the same as in the “haber model”. Once again, we also kept everything else the same as in the “haber model”. If the “synonym model” shows the asymmetry, this will indicate that the asymmetry in the “tener model” disappeared because of the increase in the auxiliary verb frequency and not because of the added semantic weight.
4 Results
All three simulations achieved a similar performance on the progressive and perfect test sentences (see Table 6). All 1000-sample bootstrapped 95% Confidence Intervals reported in this Table and the following figures were calculated over the percentages from each of the 60 model runs.
Table 6. Performance (percentage of test sentences with correct meaning) of the three models at the last epoch. The numbers in the brackets show the bootstrapped 95% Confidence Interval.

For each simulation, we ran a logistic mixed-effects regression analysis comparing the percentage of progressive and perfect switches at the last (40th) training epoch; the analyses include a by-model-run random intercept and a slope of sentence structure (coded as .5 for the perfect structure and +.5 for the progressive one), but no by-sentence random effects because test sentences differ between model runs. Comparisons between simulations were performed by including the factor simulation (dummy coded with “haber model” as the reference level) and the interaction with sentence structure; this analysis also included a random slope of simulationFootnote 8.
4.1 Simulation 1: “haber model”
Importantly, the “haber model” clearly produced the auxiliary phrase asymmetry, as hypothesized. Figure 2a shows the average percentage of Spanish-to-English participle switches over 60 model runs for correctly produced sentences per structure (progressive and perfect). The model output showed a strong preference for progressive participle switches over perfect participle switches: at the last training epoch, 2.34% of all correctly produced progressive-form sentences had a switch at the participle whereas only 0.60% of the correctly produced perfect-form sentences had a switch at the participle. The logistic mixed-effects regression analysis showed the difference to be statistically significantFootnote 9 (b = 1.13; z = 3.25; p < 0.02).

Fig. 2. Percentage of Spanish-to-English participle switches (computed over 60 network runs per simulation and over the course of network training) of the correctly produced sentences per structure in the three simulations (top: “haber model”; middle: “tener model”; bottom: “synonym model”). Shaded areas show the bootstrapped 95% Confidence Interval.
Figure 3 shows the percentages of code-switches at the auxiliary verb and at the participle for the progressive and perfect structure. A probability of a switch at the auxiliary verb was not significantly different between structures, and a participle switch for the perfect structure was the least preferred switch point. For both structures, the simulations showed a clear preference for a switch at the auxiliary position over the participle one (10.77% progressive-auxiliary switch vs 2.34% for progressive-participle switch and 9.01% perfect-auxiliary switch vs 0.60% for progressive-participle switch). However, the probability of a switch at the auxiliary was not significantly different between the progressive and perfect structures (b = 0.11; z = 0.93; p > .3).Footnote 10

Fig. 3. Percentage of code-switches at auxiliary and participle for the progressive and perfect structures in the “haber model”, computed over 60 network runs. Shaded areas show the boot-strapped 95% Confidence Interval.
4.2 Simulation 2: “tener model”
When tested on the same 700 messages, the auxiliary phrase asymmetry all but disappeared. The output of the “tener model” (that substituted the original Spanish auxiliary verb from the “haber model” for one with more semantic weight) showed at best a small (non-significant) preference for progressive participle switches over perfect participle switches (1.30% vs 0.77% in the last epoch; Figure 2b). This difference is not statistically significant (b = 0.10; z = 0.37; p > .7) and is significantly smaller than in the “haber model” (interaction between sentence structure and simulation: b = 1.05; z = 5.26; p < .0001).
4.3 Simulation 3: “synonym model”
Finally, when tested on the same 700 messages, the “synonym model”, which controlled for the frequency increase of the auxiliary verb in the “tener model”, did not show a preference for progressive over perfect participle switches either (1.13% progressive-form participle switches vs 1.03% perfect-form participle switches; Figure 2c). This difference is not statistically significant (b = 0.48; z = 1.30; p = 0.19) and is significantly smaller than in the “haber model” (b = 1.62; z = 8.75; p < .0001).
5 Discussion
All three simulations support the hypothesis that the asymmetry can be caused by the lack of semantic weight of the Spanish auxiliary verb haber “to have”. The “haber model” exhibited the auxiliary phrase asymmetry; adding semantic weight to the Spanish perfect-form auxiliary verb (“tener model”) was enough to make the asymmetry all but disappear. If the reason that the effect became significantly smaller in the “tener model” was the frequency increase of the auxiliary verb, we would have expected the “synonym model” to produce a similar pattern to the “haber model”. In the third simulation, when controlling for the increase in the frequency of the Spanish auxiliary in the second simulation, the “synonym model” did not show a preference for progressive participle switches either, thus further supporting that the lack of semantic weight of haber can cause the asymmetry.
The probability of a switch at the auxiliary verb for the progressive structure did not differ significantly from the probability of a switch at the auxiliary for the perfect structure. Furthermore, a participle switch for the perfect structure was the least preferred switch point; both patterns reflect prior experimental and corpus-based results (Guzzardo Tamargo et al., Reference Guzzardo Tamargo, Kroff and Dussias2016). However, unlike these corpus results, in the progressive structure the simulations showed a clear preference for a switch at the auxiliary position. This indicates that the model does not capture the finding that in the corpora (Table 1) there is no preference between an auxiliary and a participle switch in the progressive structure. This discrepancy between model results and corpus data could be attributed, for instance, to the limited number of structures used in the simulations.
In this study we have only focused on the grammaticalization account. We speculate, however, that, even though in bilinguals the asymmetry is likely driven by grammaticalization, the overall switching patterns are reinforced by exposure to code-switched speech in the community (as claimed by the exposure-based account discussed in the introduction). In principle, the model should be able to simulate exposure-based explanations as well, by running second-generation simulations that receive as target the code-switched sentences of the first simulations. We expect that in this scenario the amount of overall code-switching will increase. Furthermore, we hypothesize that the perfect-form participle-switch will become even less frequent over time.
Previous literature on code-switching (e.g., Pfaff, Reference Pfaff1979 and Poplack's Reference Poplack1980 Equivalent Constraint) has argued that code-switching can only occur at points where the surface word order of the two languages is the same. Similarly, grammatical constraints on code-switching from a generative framework (e.g., Functional Head Constraint, Government Constraint) likewise make broad generalizatons on which syntactic junctures code-switches cannot occur (i.e., no code-switches between an auxiliary verb and a main verb). The findings reported here, as well as in Dussias (Reference Dussias2003) and Guzzardo Tamargo et al. (Reference Guzzardo Tamargo, Kroff and Dussias2016), have shown that such constraints are not enough to explain the code-switching patterns; the auxiliary phrase asymmetry seems to exist because of differences in the semantic weight of the auxiliary verbs, despite the fact that the structures have the same syntactic patterns. Therefore, it seems that even though syntax and word order play a role in the places where code-switching can occur, they are not the only factors governing code-switching.
As is the case with every research method, using computational cognitive modeling has certain limitations. First, the languages used in the simulations are miniature, and therefore artificial, which could be seen as a disadvantage of this research method. However, using a miniature language is also an advantage as it allows us to remove any confounding factors and to focus on the phenomenon of interest. More importantly, it gives us the unique opportunity to manipulate the languages that the model learns (i.e., the lexicon and/or structures) and to investigate whether changes in the language lead to different code-switching patterns. For instance, in this paper we show that the asymmetry disappears when the Spanish auxiliary verb haber is the same as the main verb “to have” and therefore has semantic weight like its progressive-form auxiliary verb equivalent. Second, connectionist models can produce different patterns depending on the generated training sentences and the connection weights that are assigned before the learning starts. It is therefore important not to report on a single model run, nor to hand-pick free parameters that produce the results we would like to see. In this work, we showed that the result is robust by running three separate simulations using 60 networks for each while randomizing (within fixed ranges) all free parameters and the target message-sentence pairs.
The simulations’ goal was to test whether the lexical-syntactic distribution patterns of Spanish and English can lead to the auxiliary phrase asymmetry, and the simulations have provided evidence that it can indeed. We do not claim to (and did not aim to) know what mechanism drives the asymmetry in the models’ output. We speculate, however, that having a higher semantic weight, as in the case of the Spanish and English verbs “to be” (“is”, “está”), leads to more possible upcoming words. Subsequently, this leads to the activation of more output word candidates; the most activated word is less reliably the correct Spanish word, thus increasing the probability that the most activated word is the English translation. The Spanish auxiliary verb haber, on the other hand, only has one sense and a very restricted context, as it can only be followed by a participle, thus allowing for fewer options to code-switch.
6 Conclusion
We tested whether the auxiliary phrase asymmetry in Spanish–English code-switching could be derived from the properties of the two languages. The “haber model” simulated the attested asymmetry, and the “tener model” showed that this could be attributed to the fact that the Spanish auxiliary haber has only a limited, dependent syntactic function (i.e., is more grammaticalized) and is not used as frequently as the English equivalent (“have”). The follow-up model (“synonym model”) used haber and tener as synonyms, and confirmed that the lack of asymmetry in the “tener model” can be attributed to the syntactic independence of the modified auxiliary verb and not to its increased frequency compared to the “haber model”. The three simulations thus confirm that grammaticalization could be responsible for the asymmetry.
Importantly, we showed that using computational cognitive modeling we can test hypotheses that cannot be experimentally tested in humans, such as changing the function of the Spanish auxiliary verb and observing whether the auxiliary phrase asymmetry persists. We made the grammaticalization hypothesis testable in the model, and showed that indeed the difference in semantic weight between estar and haber can cause the observed phenomenon, in line with the grammaticalization account.
Acknowledgements
The work presented here was funded by the Netherlands Organisation for Scientific Research (NWO) Gravitation Grant 024.001.006 to the Language in Interaction Consortium. Part of the work was presented at the 2019 Cognitive Modeling and Computational Linguistics (CMCL) workshop (Tsoukala, Frank, van den Bosch, Valdés Kroff & Broersma, Reference Tsoukala, Frank, van den Bosch, Valdés Kroff and Broersma2019).