1. INTRODUCTION
Throughout the history of artificial intelligence (AI), the legal domain has been one of most important areas of application. In particular, legal-expert systems, which are computer programs that solve legal problems by referring to statutes and old cases, have received attention as tools for solving social problems. The first legal-expert systems were developed in the US and the UK. Since then, a lot of legal-expert systems and related technologies have been developed. In Japan, research on these systems started in around 1980. The direction of research on these systems was affected by the Fifth Generation Computer Systems Project (FGCS Project), which was a national project from 1982 to 1993. As one of the key technologies of the project was logic-based AI and as logic is an appropriate tool for representing legal knowledge, legal-expert systems were a great match for FGCS technologies.
People throughout the world working on AI and law have proposed legal-reasoning models based on logic and they have succeeded in showing that typical example problems can be solved automatically. Legal-expert systems are useful in the education provided at law schools. However, the number of such systems that are used in the practical field is quite low because these systems cannot solve actual complex problems.
Recently, by using information communication technology (ICT) and machine-learning technology, novel application systems for AI and law have started to be developed. This research is not focused on legal-reasoning models and on solving legal problems logically, but rather tries to develop practical tools for legal tasks by using the technologies of legal analytics.
The objective of this paper is to introduce the research activities on AI and law from the history of legal-expert systems to current research trends in Japan. We have engaged in the application of AI technologies to law from the early stage of AI and law in Japan. From the viewpoint of AI researchers, first, we introduce several legal-expert systems in Japan and discuss what has been achieved and what has been left unsolved from the viewpoint of AI researchers. Then, we introduce the Japanese government’s policy toward ICT and law. Finally, we show several research projects for AI and law as well as international research activities.
2. RESEARCH ON LEGAL-EXPERT SYSTEMS IN JAPAN (1980s AND 1990s)
2.1 Comparison of the History of Legal-Expert Systems
Concerning the development of legal-expert systems, we compare the history of Japan and that of the US and Europe (Figure 1).
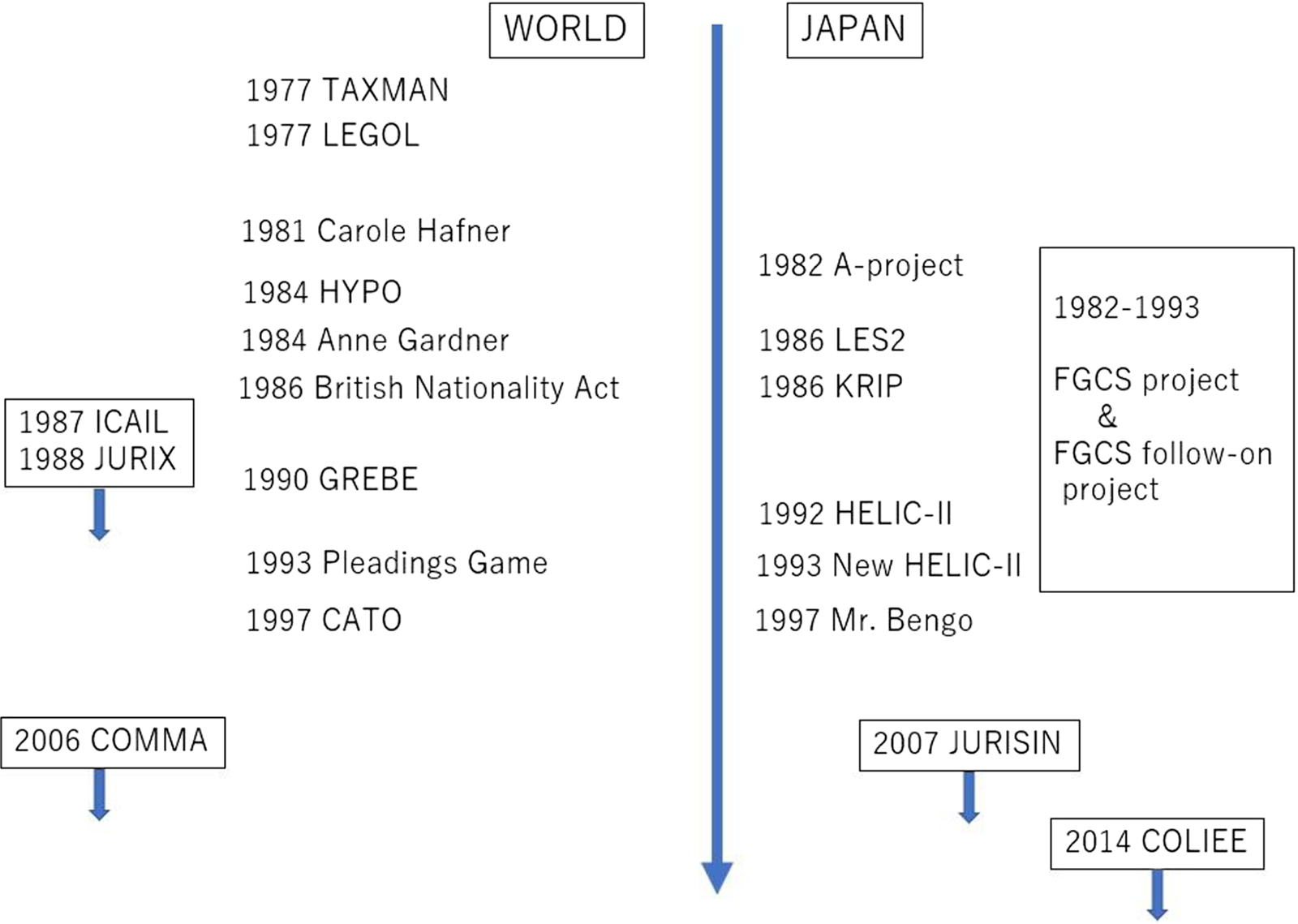
Figure 1. Comparison of the history of research of AI and law.
In the 1970s, the first legal-expert system, TAXMAN, was developed by Thorne McCarty of Rutgers University in the US.Footnote 1 TAXMAN dealt with taxation problems concerning the reorganization of a company. Ronald Stamper developed a legal-knowledge-representation language, LEGOL, and described legal knowledge by using LEGOL.Footnote 2 Since then, various legal-expert systems and related technologies such as those for legal reasoning and representing legal knowledge have been developed. For example, Marek Sergot represented the legal rule of the British National Act by using a logic-programming language, PROLOG, and showed that given legal conclusions could be validated with its theorem-proving mechanism. Kevin Ashley developed a case-based argumentation system, HYPO, for law-school education.Footnote 3
As a part of the community of AI and law researchers, the International Conference on Artificial Intelligence and Law (ICAIL) has been held every other year since 1987 and the International Conference on Legal Knowledge and Information Systems (JURIX) has been held every year since 1988.
Development on legal-expert systems was not that active in Japan because few researchers were interested in AI and law. Though several prototypes of legal-expert systems have been developed, most of them were not published in the international conference or in the international journal.
Professor Hajime Yoshino of Meijigakuin University started the A-project in 1982 and the LES-project in 1986. In the LES-project, Professor Yoshino managed a research group consisting of people involved in AI and lawyers, and developed expert systems for contract law.
Another project for legal-expert systems was established as a sub-project of the FGCS Project (1982–93). The FGCS Project was the first national AI-oriented project in the world. Under it, parallel computers, parallel AI techniques based on logic programming, and parallel AI application systems were developed. The legal-expert system was one of the applications of the project.
After these projects had finished, as AI and law researchers failed to start another big project yet wanted to keep the community going, they started the annual international workshop on juris-informatics (JURISIN) in 2007. Currently, JURISIN is known as one of the primary communities for people involved in AI and law in Asia.
Recently, not only legal-expert systems, but also systems that apply legal analytics, have started to be developed. This research is related not only to researchers of traditional legal-expert systems, but also to researchers of topics such as natural language processing (NLP) and machine learning.
To encourage this research trend, the Competition on Legal Information Extraction/Entailment (COLIEE) was started in 2014. COLIEE has been held in conjunction with JURISIN. As people involved in AI and law began to notice the usefulness of COLIEE, COLIEE 2019 was held in conjunction with ICAIL 2019.
2.2 The A-project and the LES-project (1982–1990s)
Professor Hajime Yoshino of Meiji Gakuin University is one of the pioneers of legal-expert systems in Japan. Yoshino and his group started the A-project in 1982. The purposes of the A-project were to analyze the legal rules of contract law logically and to prove the validity of the analysis by using an automated theorem prover. They represented legal rules as diagrams and, by combining the diagrams, they explained the thinking process of lawyers. Figure 2 shows an example in which conclusion F is drawn by referring to two legal rules: “if C11 or C12 are satisfied and C13 is satisfied then F becomes true” and “if C21 and C22 are satisfied then C12 is true.”

Figure 2. Representing the thinking process with diagrams.
They made a set of diagrams of a part of contract law. However, these diagrams became complex because they represented not only the original information of legal rules, but also the implicit conditions; maintaining the logical relation between complex diagrams was difficult and they failed to construct a large set of diagrams.
After the A-project, with a Grant-in-Aid for Scientific Research, Yoshino started the LES-projectFootnote 4 and most of the research results of the A-project were inherited by this project. The purpose of the LES-project was to develop a legal-expert system by using PROLOG and to propose a model of legal reasoning.
From the viewpoint of logic law, he proposed a legal-reasoning model as a back and forth between “legal justification” and “legal discovery.” “Legal justification” is logical reasoning based on deduction, which is used when legal rules are applied to facts. “Legal discovery” is an abductive reasoning that starts from the conclusion and constructs inference trees by repeatedly generating and validating hypotheses.
On the basis of this analysis, Yoshino developed two legal-expert systems: LES and LES-2. LES and LES-2 were developed by using a knowledge-representation language: Complex Predicate Formula (CPF). CPF is an extension of PROLOG with a frame structure.Footnote 5 For example, the obligation of Thomas to pay tax in April is represented as follows. In this example, “obl_1034” is an ID of a specific obligation:
PROLOG
obligation(thomas, paying, tax, April)
CPF
obligation(obl_1034, [agt:thomas, goa:paying, obj:tax, tim:April])
Yoshino and his group developed LES-2Footnote 6 by constructing a rule base for the UN Conventions on Contracts for the International Sales of Goods (CISG) by using CPF.Footnote 7 For example, the rule “if the offer becomes effective at time T1 and if the acceptance becomes effective at time T and T is after T1, then the contract is concluded at time T” is represented by the following CPF rule:
sen(‘2a’,[ ‘is_concluded’(IS_CONCLUDED,[
agt:[OFFEROR,OFFEREE],
obj:'contract’(CONTRACT,[
agt:[OFFEROR,OFFEREE],
cnt:CNT_CONTRACT,
imp:IMP_CONTRACT,
obj:OBJ_CONTRACT]),
tim:T])
<-
'become_effective'(BECOME_EFFECTIVE,[
abj:'offer'(OFFER,[
agt:OFFEROR,
cnt:CNT_CONTRACT,
goa:OFFEREE,
.. .(abbreviated). . .
]),
tim:T1])
&
become_effective(BECOME_EFFECTIVE2,[
abj:acceptance(ACCEPTANCE,[
agt:OFFEREE,
cnt:CNT_ACCEPTANCE,
goa:OFFEROR,
imp:IMP_ACCEPTANCE,
obj:offer(OFFER,[
agt:OFFEROR,
. . .(abbreviated). . .
]),
src:SRC_ACCEPTANCE,
tim:TIM_ACCEPTANCE]),
tim:’time_after'(T,[tfr:T1])])]).
To show how LES-2 functions, let us consider the following example case:
CASE
1. April 1: Anzai sent a letter to Bernard saying that
I want to buy the construction machine at the price of 10,000.
I will send the machine by May 10.
I ask you to pay the purchase fee within 10 days from the date of arrival.
I will send the machine by truck.
I will continue the offer till May 10. You are required to send the reply by May 10.
2. April 8: The offer reached Bernard.
3. April 9: Anzai called up Bernard and said he would withdraw the offer.
4. But, Bernard answered that he accepted the offer. And also he would like that the machine was sent to Bernard not by truck but by train.
If a user inputs the case facts and the following query in the form of CPF, LES-2 responds to the user with the conclusion and reasons:
QUERY
What legal rights exist on the date of May 10?
In addition to the deductive reasoning of PROLOG, CPF has the function of non-monotonic reasoning, which solves contradictions by introducing priority relations among legal rules. In the legal domain, meta-rules that decide priority among legal rules are sometimes used by lawyers. Meta-rules such as the “principle of precedence over the new law (lex posterior),” “principle of precedence over the upper law (lex superior),” and “principle of precedence over the special law (lex specialis)” are examples of meta-rules. When the judge decides on a conclusion by comparing the arguments of the plaintiff and the defendant, he or she may use reasoning based on precedents on the basis of his or her value judgement. The reasoning mechanism of LES-2 could simulate the judge’s reasoning process.
The LES-project succeeded in getting a wide range of AI researchers, such as those working on NLP, formal logic, and knowledge-base systems, to join and this project contributed substantially to the research on AI and law in Japan. However, the difficulty of developing a large-scale rule base was still unsolved.
2.3 Consultation System for Patent Law: KRIP
KRIP is a consultation system of procedures of patent law that was developed by Katsumi Nitta.Footnote 8 One of the main purposes of KRIP was to develop a knowledge-representation language for procedural laws.
In Japanese patent law, various procedures are defined, such as filing applications, amending applications, requesting examination, and publishing patent applications. Various rights concerning intellectual property are also defined in the patent law, such as the right to obtain a patent, the right to compensation for suffering damage, patent rights, and the right of injunction. For each procedure, the conditions of the agent, of the object, of the format, and of the period of time taken for the procedure are strictly defined. To describe such conditions of procedures, the frame structure and temporal notations must be described. Therefore, Nitta and his team designed a knowledge-representation language for procedural law, KRIP/L, based on PROLOG. For example, let us consider the procedure for “requesting examination.” The related rules are Section 7, Section 48-2, Section 48-3, and Section 48-4:
Section 7 (1) Minors or adult wards may not undertake procedures except through their statutory representatives;
Section 48-2 The examination of a patent application shall be initiated after the filing of a request for examination.
Section 48-3 (1) Where a patent application is filed, any person may, within 3 years from the filing date thereof, file with the Commissioner of the Patent Office a request for the examination of the said application.
Section 48-4 A person(s) filing a request for the examination of an application shall submit a written request to the Commissioner of the Patent Office stating the following:
(i) the name and domicile or residence of the demandant; and
(ii) the identification of the patent application for which the examination is requested.
KRIP/L represents this knowledge with classes and logical formulas as follows. The formula of Section 48-3 is a special form that describes temporal information:
class request_for_examination
super: procedure_before_Patent_Office
agt: X { procedural_ability_person }
obj: Y { patent_application }
dat: Z { date }
effect: {text about the effect (section48_2)}
legal_check -> procedural_ability_person(X, section7),
patent_application(Y, section48_4),
within_time_limit(X.date, Z, section48_3).
effect-> exec(Y,section48_2)
end
section7.
procedural_ability_person(X) :- not minor(X), not adult_ward(X),!.
procedural_ability_person(X) :- statutory_representative(X).
section48_2.
exec:-initiate_status(Y).
section48_3.
[patent_application(X):limit(3years)]->may request_for_examination.
section48_4.
patent_application(Y) :- demandant(X), patent_id(Y).
The following is an example case. When a user inputs the following events and a query in the form of KRIP/L, the KRIP system then checks the conditions of each event by executing the legal_check part. If the conditions are satisfied, the effect parts are then executed one by one and the query is finally answered:
CASE
1990/03/01 Mr A invented the material X.
1990/06/03 Mr A filed a patent application whose claim is X.
1991/12/10 Publication of the patent application of X
1992/01/03 Mr A amended his claim from the material X to the method of producing X.
1992/04/20 Mr A found B co-operating in selling X.
QUERY
Can Mr A prohibit B from selling X?
As knowledge representation for procedural laws, class notation is useful for representing procedures between the Patent Office and a patent applicant, similarly to using a dictionary. By separating the description of patent law as a class and logical formula, constructing knowledge became easier.
KRIP was useful for students who challenged the examination of a patent attorney. However, like most logic-based legal-expert systems, KRIP cannot make judgments under ambiguous conditions. For example, it cannot judge whether a given invention satisfies patentability, such as whether it is industrially applicable, novel, or an inventive step.
2.4 Legal-Reasoning Systems: HELIC-II, New HELIC-II
The above-mentioned FGCS Project was a national project whose main objectives were to develop a parallel computer for AI and to develop parallel AI software technologies. The parallel computer, PIM (Parallel Inference Machine), consisted of 512 CPUs and executed AI computer programs written in the parallel logic-programming language KL/1. With PIM, a lot of parallel AI technologies and AI application systems were developed. For example, parallel theorem provers, parallel DNA-sequence aligners, parallel logic circuit diagnosis systems, parallel Go game-playing agents, and parallel NLP systems were developed.
2.4.1 HELIC-II
HELIC-II, a legal-reasoning system, was one of the parallel application systems.Footnote 9 HELIC-II has an architecture with two inference engines: a rule-base reasoner (RBR) and a case-base reasoner (CBR). Legal rules are represented as a logical formula and stored in the rule base. Old cases are represented as a semantic network and stored in the case base. When a user inputs a new criminal case (a set of facts), RBR first tries to apply criminal rules to the case. However, as legal rules often include ambiguous conditions, RBR fails to apply legal rules. Then, CBR searches for similar cases from the case base and judges whether the ambiguous condition is satisfied or not.
For example, let us consider the case in which Mr A and Mr B quarrelled violently and, as Mr A threatened Mr B with a knife, Mr B shot Mr A with a gun. The related criminal rule, Article 36, is represented as follows:
not_punishable(X) :- to_protect_right(ActA), unlawful_infringement(AtcB),
against(ActA, ActB).
As the conditions “to_protect_right” and “unlawful_infringement” are ambiguous predicates, it is hard for RBR to judge these conditions as being satisfied by the given facts. Thus, CBR searches for similar cases in which these two conditions are satisfied and not satisfied. If both cases exist, RBR will generate both conclusions referring to the cases.
The architecture consists of RBR and CBR, and it has been employed in previous systems such as CABARETFootnote 10 or GREBE.Footnote 11 Usually, CBR needs a lot of time to search for similar old cases. Though HELIC-II employed a similar architecture, it calculated legal conclusions by parallel processing on PIM.
2.4.2 New HELIC-II
In 1993, as a successor to HELIC-II, New HELIC-II was developed.Footnote 12 In addition to the RBR and CBR of HELIC-II, New HELIC-II had another inference module: the non-monotonic reasoner (NMR). The role of NMR is to resolve conflict using priority between rules as above-mentioned CPF. When RBR and CBR generate possible conclusions, NMR refers to the priority rules of an agent and selects one conclusion from among them. The priority rules represent the value judgement of a person. As each person has his own priority rules, the conclusion depends on the person. For example, if Mary enters a park with a dog, the park staff may stop her from entering because entering with a dog is prohibited, but Mary may insist that this is an exceptional case because the dog is in a box. In this case, there is no definite rule for solving this conflict. If a person thinks that keeping the rule is more important, then he or she will agree to stop Mary from entering. On the contrary, if a person thinks that entering a park with a dog that is inside a box is not a problem, he or she will allow Mary to enter.
New HELIC-II was designed as an automated-argument agent: one for the plaintiff and one for the defendant. When the user inputs the initial goal and facts to a plaintiff agent, the plaintiff generates possible inference trees; then, on the basis of the value judgement of the plaintiff, the plaintiff selects the best inference tree and sends it to the defendant. The defendant agent generates possible counter-arguments (inference trees), selects the best one, and sends it to the plaintiff (Figure 3). By repeating these message exchanges, the argumentation between the plaintiff and the defendant is simulated, as a follows:
Plaintiff=> Defendant
[ cannnotEnter(mary, dog#1) <- person(mary), dog(dog#1).
person(mary).
dog(dog#1).]
% Mary should not enter the park with a dog, dog#1, because there is a rule that entering with a dog is prohibited from entering.
Defendant<=Plaintiff
[ canEnter(mary,dog#1) :- person(mary), dog(dog#1), inBox(dog#1).
person(mary).
dog(dog#1).
inBox(dog#1).]
% This rule is not applicable to a dog which is inside a box.
Plaintiff=>Defendant
[ cannotEnter(mary,dog#1) :-
oldCase([person(mary), dog(dog#1), inBox(dog#1)],
[person(ken), dog(dog#2),inCar(dog#2),
cannotEnter(ken,dog#2)]).]
% There is an old case in which a person and a dog were not allowed to enter
though the dog was inside a car.
Defendant<=Plaintiff
….
The output of New HELIC-II is not a conclusion of a given legal case, but an argumentation between the plaintiff and the defendant. This is the same as the output of HYPO. However, New HELIC-II refers to both statutes and old cases, which is different from HYPO.

Figure 3. Argumentation by New HELIC-II.
2.5 The Multimodal Legal-Argumentation System, Mr. Bengo
Though New HELIC-II could demonstrate automated argumentation between a plaintiff and a defendant, it had a problem in that there was no mechanism for controlling the argumentation. The system generates and selects a counter-argument in accordance with a predefined order and the user cannot control the strategy for making counter-arguments nor stop the argumentation until all possible arguments are generated.
To solve this problem with New HELIC-II, an extended system, Mr. Bengo, was developed after the FGCS Project.Footnote 13 To develop Mr. Bengo, a mini project team was constructed at the Electrotechnical Laboratory. The team was composed of researchers from the inference section, the image-processing section, the speech-understanding section, and the NLP section.
Mr. Bengo employed a mechanism by which a user could control the plaintiff agent or the defendant agent by voice (Figure 4). A user can ask an agent to make an argument for a given goal. Then, the agent recognizes the user’s command and shows one candidate argument. If the user likes the argument, they can ask the agent to inform the other agent of it. If the user does not like the argument, they can ask the agent to make another one. In addition to these key commands, the user may compromise by withdrawing their claim or they may stop the argumentation. A multimodal interface was developed for controlling the plaintiff.

Figure 4. Architecture of Mr. Bengo.
On a screen, three animated agents representing the plaintiff, defendant, and judge appear and the user can communicate with the plaintiff or the defendant. During argumentation, both agents recognize the situation of argumentation and their facial expressions change in accordance with the situation (Figure 5). Both agents have five kinds of facial expressions: “normal,” “smiling,” “angry,” “sad,” and “surprised.” If an agent recognizes the argumentation situation as being advantageous, it shows a smile expression and, if the situation is disadvantageous, it shows a sad one.

Figure 5. Screenshot of Mr. Bengo.
As there were few multimodal agents in the late 1990s, Mr. Bengo drew interest from people involved in AI. Mr. Bengo showed that multimodal user interfaces can be effective for argumentation. Even if an agent does not express the situation of argumentation in an obvious manner, the agent could inform the user of the situation by facial expression. However, Mr. Bengo did not have a function for observing the user’s facial expressions, voice features, and gestures, so communication between the user and the agent was limited.
3. ACTIVITIES ON ICT AND LAW IN JAPAN (2000–PRESENT)
In the previous section, we introduced examples of legal-expert systems developed in Japan from 1982 to 1997. However, they have not been applied for practical legal uses because their rule bases and case bases are small and insufficient. Therefore, they solved only typical examples and their main target users were supervisors and students of law schools. Recently, ICT has begun to be used as a convenient tool for legal practice.
In this section, we will introduce examples of ICT applications, such as legislation, litigation, and contract support. Then, we will introduce two projects for legal compliance.
3.1 Support for Litigation
With ICT, the litigation process is expected to become more effective (Figure 6). For example, e-Filing is a procedure for online suits, e-Case management is the online management of legal documents in court, and e-Court is for supporting argumentation in court. Witness interrogation with a TV conference system is one example of e-Court systems.

Figure 6. Litigation supported by ICT.
In the US, litigation databases have been made public and anyone can access court documents. In Korea, online litigation has become available and people can reserve a date for argumentation in court by using a mobile phone. In Singapore, an e-Filing system has become available. On the contrary, the Japanese government has not employed these systems proactively compared to other countries. However, in 2018, as a part of the Future Investment Plan of the Cabinet, the Japanese government decided to employ online-litigation-process systems. According to the plan, by the year 2022, litigation-support systems will be created. Then, anyone will be able to access court documents and legal documents will be analyzed in detail with techniques of text analysis.
3.2 Support for Legislation
In this section, we will introduce two legislation support systems, namely e-LAWS and e-Len, which are used practically.
3.2.1 e-LAWS
Laws are often revised according to changes in social customs or economy policy and so on. To revise a law, staff members of the Ministry of Justice make a comparable table of prior and amended article provisions, replace prior article provisions with article provisions according to the comparable table to check the validity of the table, and then make the sentences contained in amendments on the basis of the table. This process is performed manually. It takes a lot of time and sometimes causes mistakes in the amendments. In 2016, the Ministry of Internal Affairs and Communications started an e-Legislative Activity and Work Support System: e-LAWSFootnote 14 (Figure 7). e-LAWS supports staff members in making a comparable table, checking the validity of the table, and making the sentences contained in amendments. e-LAWS has a legislation database in which each legal rule is annotated with XML tags. By using XML tags, statutes are more easily revised than when using HTML tags. This legislation database is available to the public through an online portal (eGov) of the Ministry of Internal Affairs and Communications.Footnote 15 Professor Katsuhiko Toyama of Nagoya University contributed to the development of e-LAWS and he has been designing and developing a bilingual law database for the Ministry of Law. This database is expected to contribute to the internationalization of Japanese law.

Figure 7. Overview of e-LAWS.
3.2.2 e-Len
In 2016, Professor Tokuyasu Kakuta of Chuo University developed a Japanese local-government ordinance database: e-Len.Footnote 16 e-Len contains ordinances of 1,606 local governments (90% of the total number of ordinances of local governments in Japan) and they are annotated with XML tags. e-Len has functions for the cross-sectional retrieval of ordinances, classification of similar ordinances, and automatic generation of comparable tables. When a local government makes a new ordinance, by referring to similar ordinances, the time necessary to make a first version of the ordinance is decreased. In fact, e-Len is accessed by a lot of local governments, so its effectiveness has been validated. Kakuta succeeded in getting funding from CREST and he is developing a system for supporting the making of ordinances by applying machine-learning techniques to the e-Len database.
3.3 Support for Legal Practices
By employing ICT, practices in Japanese law firms are facilitated and Japanese law firms have started the following online legal services for various users, such as personal users, companies, and legal specialists:
Making contract documents by using a contract database
Managing the making of contracts and taking care to fulfil agreements
Taking care to jointly file lawsuits such as by adjusting meeting dates and summarizing opinions
Making documents for filing for intellectual property
Searching for related information from document databases
Support for due diligence and forensics
Searching for proper law firms and proper lawyers
Currently, they have not yet employed advanced AI technologies such as machine learning and text processing. However, more practical and intelligent systems will be developed soon.
4. EXAMPLES OF RESEARCH PROJECTS OF AI AND LAW IN JAPAN
4.1 Verifiable and Evolvable e-Society (2004–10)
Under the twenty-first-century COE programme of the Ministry of Education, Culture, Sports, Science and Technology, Professor Katayama of the Japan Advanced Institute of Science and Technology started a project entitled “Verifiable and Evolvable e-Society” (2004–10).Footnote 17 The objective of this project was to investigate basic technologies for realizing a stable and reliable e-Society, and researchers of AI, formal logic, computer networks, and software engineering joined this project.
The e-Society system could one day be part of our society and implemented as an information system. At the preliminary stage of the project, the project analyzed various laws, regulations, and social customs as logical models and it was thought that the e-Society model would be regarded as one of the specifications of a high-level information social system.
Figure 8 shows an overview of the project. In this figure, society is regarded as being regulated by legal rules, social customs, and so on. To construct a social infrastructure, an e-Society model is necessary. With this model, legal rules and social customs are formalized by formal logic and reasoning systems.

Figure 8. Research overview.
The main themes of the project are as follows:
1. A logical system and a formal description system capable of defining an e-Society
2. Methodologies for the definition and verification of e-Society trustworthiness requirements
3. Methodologies for e-Society verification through theorem proving, model inspection, simulation, etc.
4. e-Society modelling and evolution using the latest object technologies
5. e-Society structure and functions.
Examples of results of this project are as follows:
1. Legal engineering:
Prototype system from legal rules to logical formulas
Algorithm for detecting conflicts among legal rules
Legal databases of several ordinances and pension law
2. Formal verification and formal reasoning:
Verification technology for formal specifications
Verification of information systems with theorem-proving systems
Verification of electronic commerce protocols
3. Basic security technology:
Verification of network security
Hierarchical public key cryptography
Verification of security with agent logical model.
This project produced a lot of formal theories and developed modules of basic verification and reasoning systems, which contributed to the possible realization of e-Society. However, a model of e-Society was too complex for one project team to make into a total prototype system.
4.2 Advanced Reasoning Support for Judicial Judgments by AI (2017–Present)
With support from a Grant-in-Aid for Scientific Research (S), Professor Ken Satoh of National Institute of Informatics started a project called “Advanced Reasoning Support for Judicial Judgment by Artificial Intelligence” in 2017.Footnote 18 He focused on the fact that the intellectual tasks that judges carry out are roughly divided into the fact-finding process, the subsumption process, and the judgment process, and that, in the trial process, various complicated higher-order inferences are executed, and more accurate and prompt high-order inferences should be realized by AI.
Satoh and his research group have been developing a system that supports advanced reasoning by using the following fundamental technologies and a system that analyzes argumentation in each process (Figure 9):
1. System for supporting the fact-finding process by using evidence reasoning based on a Bayesian network
2. System for supporting the subsumption process by acquiring subsumption rules on the basis of NLP
3. System for supporting the judgment process by extending the existing Civil-Code reasoning system, PROLEG, to handle criminal cases and administrative cases
4. System for analyzing argumentation based on argumentation theory.
For the logic-programming part, they developed a language called PROLEG (PROlog-based LEGal-reasoning support system) [Satoh2010] based on PROLOG. PROLOG has a mechanism called “Negation As Failure.” This mechanism assumes that a certain requisite is false if the requisite fails to be proven. However, lawyers had difficulty in understanding the semantics of logic programming with this mechanism. Therefore, they developed PROLEG, which uses the correspondence between the default value of each requisite and the general rule/exception principle. PROLEG consists of a PROLEG rule base that represents legal rules and a PROLEG fact base that describes concrete facts in a litigation.

Figure 9. Architecture of system.
4.2.1 The PROLEG Rule Base
In the PROLEG rule base, requisites are expressed in the form of the following two expressions:
Conclusion <= Rerequisite1, Rerequisite2, …, RerequisiteN.
Exception(Conclusion, Exception).
The conclusion, requisite, and exception are each expressed in the form of: “predicate name” (argument_1, argument_2, …, argument_m). The first expression above (referred to as the “general rule”) means that a “Conclusion is satisfied if Requisite 1 and Requisite 2 and … and Requisite N are satisfied in principle.”
In addition, the second expression (called the “exception rule”) means that a “Conclusion is not satisfied if the Exception is satisfied (even if all the requisites are satisfied for the general rule).”
For example, the requisites regarding the cancellation of unauthorized subleasing (Article 612 of the Civil Code and a Supreme Court judgment, 25 September 1968) are expressed as the following rule base of PROLEG:
cancellation_due_to_sublease(Lender,Borrower,T_cancel)<=
agreement_of_lease_contract(Lender,Borrower,Object,Price1,T1,Term1),
handover_based_on_the_contract(Lender,Borrower,
lease_contract(Lender,Borrower,Object,Price1,T1,Term1)),
agreement_of_lease_contract(Borrower,ExBorrower,Object,Price2,T2,Term2),
handover_based_on_the_contract(Borrower,ExBorrower,
lease_contract(Borrower,ExBorrower,Object,Price2,T2,Term2)),
make_use_of_or_take_the_profits_of_a_leased_thing(ExBorrower),
manifestation_of_intention_of_cancellation(Lender,Borrower,T_cancel).
exception(cancellation_due_to_sublease(Lender,Borrower,T_cancel),
approval_of_sublease(Lender,Borrower,T_cancel)).
approval_of_sublease(Lender,Borrower,T_cancel) <=
date_of_approval_of_sublease(Lender,Borrower,T_accept),
before_the_day(T_accept,T_cancel).
exception(cancellation_due_to_sublease(Lender,Borrower,T_cancel),
nonabuse_of_confidence).
nonabuse_of_confidence <= fact_of_nonabuse_of_confidence(Fact).
exception(nonabuse_of_confidence,abuse_of_confidence).
abuse_of_confidence <= fact_of_abuse_of_confidence(Fact).
In the general rule in the PROLEG rule base that concludes in unauthorized sublease cancellation, Lender, Borrower, T_cancel, Object, Price1, T1, Term1, Price2, T2, and Term2 are all variables and they are instantiated by facts designated in the PROLEG fact base. There are two requisites for “agreement_of_lease_contract” in the requisites of the general rule. The first one expresses the lease agreement between the lessor and the lessee, and the second one expresses the lease agreement between the lessee and the sub-lessee.
There are two exceptions for unauthorized subleasing cancellation: “approval_of_sublease(Lender,Borrower,T_cancel)” and “nonabuse_of_confidence.” Each exception has a rule that has other requisites. Moreover, for “nonabuse_of_confidence,” there is an exception of an exception—that is, abuse_of_confidence.
4.2.2 The PROLEG Fact Base
If a requisite in a general rule does not have any rule whose conclusion is the requisite, it is called a “fact predicate” and it is defined in the PROLEG fact base to represent concrete facts in a litigation. For example, for “agreement_of_lease_contract(Lender, Borrower, Object, Price, T, Term),” the PROLEG rule base does not have a rule whose conclusion is this predicate so that it is defined in the PROLEG fact base.
“fact(XX)” means that fact XX has been asserted by either party and the judge’s degree of confidence exceeds the degree of proof. The expression “nonfact(XX)” is a formula introduced for the sake of clarity of explanation and it means that the fact is asserted by either party, but the judge’s degree of confidence does not exceed the degree of proof.
fact(agreement_of_lease_contract(plaintiff,defendant,this_real_estate,
'2_thousand_euro_per_month’,2014 year 4 month 1 day,’term_is_5years’)).
fact(handover_based_on_the_contract(plaintiff,defendant,
lease_contract(plaintiff,defendant,this_real_estate,
'2_thousand_euro_per_month’,2014 year 4 month 1 day,’term_is_5years’))).
fact(agreement_of_lease_contract(defendant,nonparty_A,this_real_estate,
permonth(800 euro),2018 year 4 month 1 day,’term_is_2years’)).
fact(handover_based_on_the_contract(defendant,nonparty_A,
lease_contract(defendant,nonparty_A,this_real_estate,
permonth(800 euro),2018 year 4 month 1 day,’term_is_2years’))).
fact(make_use_of_or_take_the_profits_of_a_leased_thing(nonparty_A)).
fact(manifestation_of_intention_of_cancellation(
plaintiff,defendant,2019 year 6 month 18 day)).
nonfact(date_of_approval_of_sublease(plaintiff,defendant,2017 year 12 month 6 day)).
fact(fact_of_nonabuse_of_confidence(short_time_usage)).
fact(fact_of_abuse_of_confidence(complaint_about_sublessee_usage)).
4.2.3 The Output of Inference Process of PROLEG
Given a query about the existence of a certain legal conclusion, the PROLEG system gives not only the existence/non-existence of the conclusion, but also the process of how the conclusion was obtained. There are two types of output methods: sentential trace and diagram trace (referred to as the “PROLEG block diagram”) (Figure 10).

Figure 10. Block diagram.
In the output of the diagrammatic trace, the conclusion is placed at the upper left and the boxes corresponding to the requisites of the general rule leading to the conclusion are connected by solid lines to the right of the conclusion. If a requisite is satisfied, “o” is shown in the bottom of the box, and, if the requisite is not satisfied, “x” is shown. When exceptions have to be considered, boxes corresponding to the exceptions connected by broken lines below are shown and the existence/non-existence of an exception is indicated by “o” or “x.” If one of the exception boxes has “o,” then the conclusion of the upper left becomes “x.”
4.3 Drafting Support of Legal Texts by AI (2017–Present)
With the support of the Japan Science and Technology Agency, Professor Tokuyasu Kakuta started a project whose aim is to support the creation of legal documents such as statutes, agreements, contracts, and so on.Footnote 19 When a local government makes a new ordinance, in many cases, a similar ordinance from another local government is referred to. By modifying this similar ordinance, a draft of a new ordinance will be produced. In the same way, when a law firm creates a contract document, the document is made by referring to other contract documents.
The objective of this project is to support these work tasks by using AI technologies and databases of ordinances and contract documents. The approach is to generate templates of legal documents from huge numbers of legal documents by using clustering techniques, to create legal documents by filling information in templates and verify the quality of the documents, and to propose a method for training local-government staff in documentation skills (Figure 11).

Figure 11. Overview of drafting support.
Kakuta has experience from developing the e-Len system, which is the above-mentioned database of ordinances of local governments. It includes about 90% of ordinances of local government and XML tags are attached to each section. Kakuta has made the e-Len system available to local governments and a lot of local governments have accessed it. Kakuta and his team noticed that the wording of ordinances whose purposes are the same is very similar because ordinances are made by referring to other ordinances. Using this fact, he has been developing an effective clustering method for legal documents based on the similarity of sentences. Similarity between sentences is used to verify the produced documents, too. If the input documents include specific sentences that have not appeared in other documents, messages to check the sentences carefully will be sent to the users.
5. INTERNATIONAL ACTIVITY OF AI AND LAW IN JAPAN
5.1 JURISIN
There are several international conferences related to AI and law in the US and Europe. For example, ICAIL and COMMA are held every other year and JURIX is held every year. As there was no such international conference in Asia, people involved in AI and law in Japan planned to have an international conference in Asia.
In 2007, with the support of the Japan Society of Artificial Intelligence (JSAI), JURISIN was started as an annual international workshop.Footnote 20 “Juris-informatics” is a new research area that studies legal issues from the perspective of informatics. The purpose of this workshop is to discuss both fundamental and practical issues among people from various backgrounds such as law, social sciences, information and intelligent technology, and logic and philosophy, including the conventional AI and law areas. The related topics cover a wide range of research areas, such as the following examples:
Models of legal reasoning
Argumentation/negotiation/argumentation agents
Formal legal knowledge base/intelligent management of legal knowledge bases
Use of informatics and AI in law
Legal issues with ubiquitous computing/multi-agent systems/the Internet
Social implications of using informatics and AI in law
NLP for legal knowledge
Verification and validation of legal knowledge systems
Online dispute resolution
Legal-document analysis
Evidential reasoning
AI applied to forensics
Any theories and technologies that are not directly related to juris-informatics but have the potential to contribute to this domain.
At the first JURISIN (2007), most of the presentations were made by Japanese researchers. However, gradually, JURISIN has become popular throughout the world. In 2018 and 2019, 37 and 39 researchers joined JURISIN, respectively, and about one-third of the presentations and participants were from foreign countries.
5.2 COLIEE
At JURISIN, various pieces of research concerning juris-informatics are presented. However, it is hard to compare the effects of these pieces of research because the research objectives are different. Therefore, in addition to an original session at JURISIN 2014, Ken Satoh and his group started COLIEE in conjunction with JURISIN.Footnote 21 The purpose of COLIEE is to promote research on AI and law by comparing the effects on the basis of the same criteria.
The current tasks consist of Tasks 1 and 2, whose target is Canadian case bases, and Tasks 3 and 4, whose target is the Japanese bar examination of statutes. When making judgments in Canada, each document of the cases referenced in a decision are annotated. Task 1 is for recognizing which cases in the case base are referred to the input case. Task 2 is for recognizing which paragraphs in an old case subsume a decision. Task 3 is for recognizing statutes that are related to an input question on the bar examination. Task 4 is for solving YES/NO questions on the bar examination. For Tasks 3 and 4, questions on the bar examination and the statutes book are given to the participants in Japanese and English. Every year, participants use various approaches, such as end-to-end learning and language-processing technologies.
COLIEE 2019 was held in conjunction with ICAIL2019 and 11 different teams participated in the case-law competition (Tasks 1 and 2). Regarding the statute-law tasks (Task 3 and 4), eight different teams participated in both tasks. For Task 1, various techniques were used, such as classification using only features extracted from the case header, random forest and k-NN classifiers, exploitation of case-structure information, deep-learning-based techniques, lexical and latent features, embedding of summary properties, and information-retrieval techniques. For Task 2, transformer-based tools such as BERT and ELMo, IR techniques, and textual-similarity features were used. For Task 3, most systems were good at retrieving relevant answers for easy questions, but it is still difficult to retrieve relevant articles for other question types. For Task 4, the overall performance of the submissions was still not sufficient to use these systems for real applications, mainly due to a lack of coverage for some classes of problems, such as anaphora resolution.
6. CONCLUSION
In this paper, we introduced the history of legal-expert systems and recent research activities on AI and law in Japan. In the early stages, legal-expert systems were developed by universities and national institutes. They solved typical problems as experimental systems, but they were not used for practical problems because it is hard to construct a rule base of all legal rules in a statute.
The Japanese Ministry of Justice was not so positive as to use computers for judicial and litigation jobs. However, because online databases of statutes and old cases have become available and because of progress made on ICT and AI technologies, the Japanese government decided to employ these technologies. And also, law firms have begun to employ these technologies to help in actual work and started new online businesses. In addition to these activities, through the JURISIN workshop and COLIEE competition, AI and law researchers in Japan will collaborate internationally to investigate more practical and higher-level legal-application systems.