Introduction
Prediction and prediction error are topics of growing interest in the field of second language (L2) acquisition studies (Bovolenta & Marsden, Reference Bovolenta and Marsden2021b). There is evidence to suggest that formulating expectations which are not met, broadly speaking, can enhance learning of new input. For instance, new words can be better learned when they are unexpected (Gambi et al., Reference Gambi, Pickering and Rabagliati2021; Stahl & Feigenson, Reference Stahl and Feigenson2017), due to a phenomenon known as one-shot declarative learning which is found in a variety of domains besides vocabulary learning (De Loof et al., Reference De Loof, Ergo, Naert, Janssens, Talsma, Van Opstal and Verguts2018; Greve et al., Reference Greve, Cooper, Kaula, Anderson and Henson2017). Another potential mechanism by which unmet expectations can enhance learning, this time specific to language, is implicit error-based learning (Chang et al., Reference Chang, Dell and Bock2006). This mechanism, which forms the theoretical background for the current study, posits a unified mechanism for language processing and learning that is driven by prediction error. The hypothesis is that learners are constantly formulating expectations about upcoming linguistic input based on their knowledge of the statistical distribution of the language, and when those expectations are not met, they revise their expectations accordingly (in a manner proportional to the magnitude of the prediction error), which amounts to learning.
Computational models implementing implicit error-based learning can reproduce behavioral findings from both first language (L1) acquisition (Hirsh-Pasek & Golinkoff, Reference Hirsh-Pasek, Golinkoff, McDaniel, McKee and Cairns1996; Naigles, Reference Naigles1990) and processing, specifically structural priming in adults (Chang et al., Reference Chang, Dell and Bock2006). Additional behavioral evidence in favor of implicit error-based learning accounts comes from inverse frequency priming: the finding that syntactic priming effects are stronger when the structure to be primed is encountered in an unexpected context, normally a verb that is not frequently used with that structure (Bernolet & Hartsuiker, Reference Bernolet and Hartsuiker2010).
Inverse frequency priming has been observed in both L1 and L2 speakers (Fazekas et al., Reference Fazekas, Jessop, Pine and Rowland2020; Jackson & Hopp, Reference Jackson and Hopp2020; Montero-Melis & Jaeger, Reference Montero-Melis and Jaeger2020) which, insofar as the phenomenon can be taken as an indication of implicit error-based learning, suggests that error-based learning is operating in L2 as well as L1. However, even the L2 speakers involved in these studies already had existing L2 representations of the target structure at the time of testing. Therefore, while such findings provide valuable information on L2 processing, there is still limited empirical evidence on whether prediction error can play a role in the L2 learning process—specifically, the establishment of new representations, which is the gap addressed by our study.
The aim of the present study was to investigate whether implicit error-based learning can operate at the earliest stages of L2 learning. The behavioral phenomenon we chose to investigate is inverse frequency priming, which, if observed, would suggest that an implicit error-based learning is at play. We created an artificial language study in which we manipulated verb surprisal by varying the statistical patterns of co-occurrence between specific lexical verbs and syntactic constructions. Our research question was whether experiencing higher verb surprisal would induce inverse frequency priming effects, even at the earliest stages of exposure to a new language. Below, we describe the theoretical background and existing evidence on error-based learning with specific reference to L2 acquisition.
Prediction error in language processing and learning
When we are listening to language, we are constantly and automatically forming predictions about what is coming next (Kuperberg & Jaeger, Reference Kuperberg and Jaeger2016). Computational models of language processing (Chang et al., Reference Chang, Dell and Bock2006; Elman, Reference Elman1990) suggest that prediction mechanisms may not only be helpful for comprehension but may be implicated in language learning, too. In these models, prediction error is suggested as the link between processing and learning: when predictions are disconfirmed, the model adjusts its expectations, gradually adapting to the statistical distribution of the language. The source of prediction error in these models is operationalized as surprisal, which refers to the likelihood of a specific word given the preceding context (Hale, Reference Hale2001; Levy, Reference Levy2008). Word-by-word surprisal from these models correlates with language processing in humans, measured by reading times (Frank, Reference Frank2013; Frank & Hoeks, Reference Frank and Hoeks2019; Goodkind & Bicknell, Reference Goodkind and Bicknell2018; Monsalve et al., Reference Monsalve, Frank and Vigliocco2012; Van Schijndel & Linzen, Reference Van Schijndel, Linzen, Rogers, Rau, Zhu and Kalish2018), N400 amplitudes during EEG (Frank et al., Reference Frank, Otten, Galli and Vigliocco2013, Reference Frank, Otten, Galli and Vigliocco2015), and MEG responses (Wehbe et al., Reference Wehbe, Vaswani, Knight and Mitchell2014), suggesting that humans are sensitive to the same statistical properties of language (surprisal) which generate prediction error in computational models.
A particularly influential model of language processing and acquisition which is based on prediction error is the Dual-Path model (Chang et al., Reference Chang, Dell and Bock2006). This connectionist model is based on a recurrent neural network trained on next-word prediction. As the model encounters more sentences, it gradually improves its predictions by adjusting its weights based on the magnitude of the prediction error, i.e., the discrepancy between predicted and actual input (Chang et al., Reference Chang, Dell and Bock2006). This model can reproduce data from child language acquisition (Hirsh-Pasek & Golinkoff, Reference Hirsh-Pasek, Golinkoff, McDaniel, McKee and Cairns1996; Naigles, Reference Naigles1990) and from structural priming in adults (Chang et al., Reference Chang, Dell and Bock2006). The Dual-Path model’s ability to reproduce phenomena from L1 acquisition and processing suggests that these may be driven by prediction error: as we encounter unexpected (high-surprisal) input, we update our representations to match that input, which amounts to learning. Therefore, there is growing interest in the role that prediction error may play in first language acquisition (Fazekas et al., Reference Fazekas, Jessop, Pine and Rowland2020; Havron et al., Reference Havron, Babineau, Fiévet, Carvalho and Christophe2021, Reference Havron, de Carvalho, Fiévet and Christophe2019).
In addition to modeling, there is empirical evidence to suggest a role of prediction error as a consequence of surprisal in language learning, specifically in the development of syntactic representations. Encountering an infrequent structure (which has high surprisal) leads to stronger structural priming of that structure compared to encountering a frequent one (Bernolet & Hartsuiker, Reference Bernolet and Hartsuiker2010; Jaeger & Snider, Reference Jaeger and Snider2013; Kaan & Chun, Reference Kaan and Chun2018; Kaschak et al., Reference Kaschak, Loney and Borreggine2006), a phenomenon usually referred to as “inverse frequency priming.” Inverse frequency priming effects have been shown to last beyond immediate priming, leading to adaptation in L1 in both adults and children (Fazekas et al., Reference Fazekas, Jessop, Pine and Rowland2020; Jaeger & Snider, Reference Jaeger and Snider2013). Fazekas et al. (Reference Fazekas, Jessop, Pine and Rowland2020) investigated adaptation to the English dative alternation (direct object vs. prepositional dative construction) in an empirical study with both adults and children. They found that exposing participants to surprising dative sentences (using verbs rarely associated with the dative structure) made participants more likely to use the dative structure in a post-test.
Empirical evidence for error-based learning in L2 acquisition
Alongside L1 acquisition research, the evidence reviewed in the previous section has led to increasing interest in the role that prediction error may play in second language (L2) acquisition too (Bovolenta & Marsden, Reference Bovolenta and Marsden2021b; Kaan & Grüter, Reference Kaan and Grüter2021; Phillips & Ehrenhofer, Reference Phillips and Ehrenhofer2015). Crucially, inverse probability priming and adaptation effects have also been observed in L2 speakers (Kaan & Chun, Reference Kaan and Chun2018; Montero-Melis & Jaeger, Reference Montero-Melis and Jaeger2020) suggesting that error-based learning mechanisms may be active during L2 acquisition. Priming effects in L2 learners can be affected by the statistical distribution of relevant structures in the learners’ L1, especially at lower proficiency levels (Jackson & Ruf, Reference Jackson and Ruf2017; Montero-Melis & Jaeger, Reference Montero-Melis and Jaeger2020). In Montero-Melis & Jaeger (Reference Montero-Melis and Jaeger2020), L2 Spanish (L1 Swedish) speakers were exposed to descriptions of motion events that varied in how they were encoded (by path or manner). For low-proficiency speakers, adaptation was strongest for encoding that was rarer in their L1 Swedish, but as proficiency increased, learners progressively aligned with L1 Spanish speakers, that is with stronger adaptation to the type of encoding that is rarer in Spanish than in Swedish. Therefore, it seems that low-proficiency learners can exhibit inverse frequency priming based on the statistical distribution of the relevant structure in their L1 and gradually become sensitive to L2 statistics as their proficiency increases. However, while these findings provide evidence of a shift in the strength of established L2 representations, they do not provide direct evidence for a role of prediction error in the development of new syntactic representations. To our knowledge, no study has investigated inverse frequency priming and adaptation effects at the earliest stages of L2 acquisition.
Evidence from artificial language learning studies suggests that direct structural priming effects can operate at the very earliest stages of L2 acquisition in adults: in Weber et al. (Reference Weber, Christiansen, Indefrey and Hagoort2019), participants who were exposed to a novel artificial language began exhibiting repetition priming for syntactic structures from the second day of exposure, measured by faster read-aloud times and improved structural comprehension on a picture matching task. Therefore, it is of theoretical interest to investigate whether inverse probability effects could a) lead to enhanced priming at the earliest stages of L2 acquisition and b) have lasting effects on newly developed representations, promoting the establishment of structural knowledge. To our knowledge, this question has not been investigated before. If we observe that inverse probability priming and adaptation can affect the development of new structural representations, it could suggest that error-based learning mechanisms can operate at the initial stages of L2 learning in adults.
Previous empirical studies on priming, including inverse frequency priming, have usually relied on the distribution statistics of competing syntactic structures, such as the alternation between the propositional dative and direct object dative constructions in English (Fazekas et al., Reference Fazekas, Jessop, Pine and Rowland2020; Jaeger & Snider, Reference Jaeger and Snider2013; Kaschak et al., Reference Kaschak, Kutta and Jones2011). However, for ab initio learners, one might ask what the source of prediction error would be. On the one hand, evidence suggests that priming effects in low-proficiency L2 learners are affected by the statistics of related constructions in their L1 (Montero-Melis & Jaeger, Reference Montero-Melis and Jaeger2020; Weber et al., Reference Weber, Christiansen, Indefrey and Hagoort2019). On the other hand, the distribution of the L2 input can inform learners’ expectations even at the earliest stages of learning. For instance, artificial language learning research on the acquisition of verb selectional restrictions has shown that the presence of a class of alternating verbs (i.e., verbs that can occur with different syntactic structures) in an artificial language can affect the acquisition of other verbs, generating weaker selectional restrictions for non-alternating verbs learned in alternating context relative to those learned in a fully non-alternating one (Wonnacott et al., Reference Wonnacott, Newport and Tanenhaus2008). Relatedly, formal accounts of generalization in the development of linguistic rules, including syntactic alternation (Yang & Montrul, Reference Yang and Montrul2017), suggest that the extent to which learners generalize new rules depends on the ratio between the total number of items in a category (e.g., verbs), and the number of instances from that category that do and do not conform to the rule (e.g., verbs that can alternate between competing syntactic structures versus those that cannot). Until a threshold for generalizing a rule is crossed, learning remains item-specific. Therefore, the distribution of a rule in the input can shape rule learning to be item-specific, creating a potential source of prediction error. In the current investigation, we used the alternation between the active and passive structure in an artificial language as a case study. We manipulated surprisal values for verbs in specific syntactic contexts by only exposing participants to non-alternating verbs during initial learning, which would generate strong expectations for verbs to be structure-specific—providing the opportunity for prediction error when these expectations would later be violated.
The current study
The aim of this study was to test whether manipulating input surprisal could aid the acquisition of new L2 syntactic structures. The specific mechanism we investigated was inverse frequency priming and adaptation, which we assumed to be an instance of implicit error-based learning (Chang et al., Reference Chang, Dell and Bock2006). We hypothesized that if inverse frequency effects can occur at the earliest stages of developing L2 syntactic representations, we should see immediate and delayed priming effects for high-surprisal verb-structures as manifested by higher accuracy in structural comprehension (Weber et al., Reference Weber, Christiansen, Indefrey and Hagoort2019), as well as grammaticality judgments. To address our research question, we conducted a pre-registered study, in which participants learned an artificial language over the course of three days.
The language and training paradigm we used were built on a previous language learning study, which investigated the effect of prediction error at the event level (Bovolenta & Marsden, Reference Bovolenta and Marsden2021a). In that study, participants learned an artificial language with an active and a passive structure (Yorwegian). Learning took place in a cross-situational learning paradigm where participants heard sentences and had to select their correct interpretation from two pictures presented on screen. Cross-situational learning is uninstructed and exposes learners to the language under conditions of uncertainty, in a way that reflects, to some extent, naturalistic language learning (Rebuschat et al., Reference Rebuschat, Monaghan and Schoetensack2021; Walker et al., Reference Walker, Monaghan, Schoetensack and Rebuschat2020; Yu & Smith, Reference Yu and Smith2007). Bovolenta & Marsden (Reference Bovolenta and Marsden2021a) aimed to generate prediction and prediction error by manipulating feedback to participants’ answers, whereby the feedback either aligned with or violated expectations. In the current experiment, we changed Bovolenta & Marsden’s paradigm to study the effect of verb surprisal on priming by manipulating the statistical distribution of verbs in the language (instead of manipulating the syntactic structure used in feedback).
Training on the first day established expectations for specific co-occurrence patterns between individual verbs and structures, which were then violated on the second day for the surprisal group, but not for the control group. Participants were then tested on their knowledge of the Yorwegian active and passive structures using old (already encountered) as well as new (not previously encountered) verbs to test for generalization.
Research questions and hypotheses
Our main research question was whether higher verb surprisal would lead to inverse probability priming and adaptation for newly encountered structures. We hypothesized that high-surprisal input would lead to inverse frequency priming and adaptation even at the very earliest stages of language acquisition, promoting the development of new structural representations. If higher surprisal led to priming, we would expect to see an immediate (priming) effect as well as a delayed one (adaptation). We tested for priming effects on acquisition with two kinds of auditory tests: structural comprehension (both immediate [day two] and delayed [day three]) and grammaticality judgments (delayed only).
With regard to grammaticality judgments, we also hypothesized that encountering verbs in unexpected syntactic contexts may make the surprisal group more likely to revise their expectations and accept verbs in alternative structures, compared to the control group. Therefore, we expected the surprisal group to be more accepting of verb-mismatched items (e.g., formerly—Day 1—active verbs presented on Day 2 in passive structures) in the auditory grammaticality judgment task relative to the control group.
Data availability
All materials, data, and analysis code for the experiments in this article can be found at https://doi.org/10.17605/OSF.IO/EU4AV and on the IRIS database (https://www.iris-database.org/).
Method
The predictions, sampling plan, and statistical analysis for this study were pre-registered online (https://doi.org/10.17605/OSF.IO/Q9KRZ).
Power analysis
To calculate sample size, we ran a power analysis based on the findings of a previous study carried out using the same paradigm, though with different statistical distributions on Day 1 (Appendix S1). That study had shown group differences in a test of structural comprehension at the end (Day 3), with higher accuracy on passive structures for the surprisal group, but these differences were not statistically significant. We calculated Bayes’ factors for the difference between means in this structural comprehension test using a Bayes’ factor online calculator (Dienes, Reference Dienesn.d., Reference Dienes2014). The results showed that the observed difference had a Bayes’ factor of 1 (inconclusive), meaning that it did not provide strong evidence either in favor of or against our hypothesis. Given the trends we observed, we considered whether the manipulation we used may not have been sufficiently strong: evidence suggests that adaptation effects can be quite subtle and that studies examining these effects require large numbers of participants in order to reach acceptable statistical power (Prasad & Linzen, Reference Prasad and Linzen2021).
The R script for the power analysis is available from the OSF repository for this study (https://doi.org/10.17605/OSF.IO/EU4AV). We simulated an average Surprisal - Control difference of 8% on passive sentences and -2% on active sentences. We tested for an interaction between group and structure using a GLMER with random intercepts for subjects and items. The results showed that increasing power by using a larger sample size would be impractical: a sample size of 144 would be required to achieve .80 power. Therefore, we opted instead to increase the number of testing items (k). Our simulation showed that if we tripled the number of items used in the structural comprehension tests, a sample of 84 participants would achieve .97 power to observe a significant interaction of the size observed in our preliminary experiment.
Participants
84 native speakers of English (68 females, M AGE = 33, SD = 6.31, range 18–45) were recruited via the online research platform Prolific (https://www.prolific.co/) and completed the study over the course of three consecutive days, receiving a compensation of £12. The study was given ethics approval by the Ethics Committee in the Department of York at the University of York. Participants all reported living in the United Kingdom at the time of taking part in the study, and all had English as their first and home language. All had to be 18 or over. 13 out 84 reported being university students. None of the participants reported having any knowledge of Scandinavian languages. On the first day of the study, participants were randomly assigned to either the surprisal or control group.
Stimuli
Participants were trained in an artificial language (Yorwegian), consisting of four nouns (glim, blom, prag, meeb—man, woman, boy, girl), twelve verbs (flug-, loom-, gram-, pod-, zal-, shen-, norg-, klig-, jeel-, lemb-, gond-, and vang-—to call, chase, greet, interview, pay, photograph, scare, threaten, dismiss, serve, kick, tease), one determiner (lu - the) and one preposition (ka - by), following the stimuli used by Bovolenta & Marsden (Reference Bovolenta and Marsden2021a). The specific word-meaning pairs, within the noun and verb categories, were randomly assigned for every participant. All sentences were SVO, but there were two possible syntactic structures, differentiated by verbal inflection and use of the preposition ka. These were the active structure (e.g., Lu meeb flugat lu prag, meaning, for example, “The girl greets the boy”) and the passive (e.g., Lu prag fluges ka lu meeb, “The boy is greeted by the girl”). The two structures are modeled on the active and passive structure found in Norwegian (as well as other Scandinavian languages).Footnote 1 The rationale for using these structures is that while the active/passive alternation is familiar to L1 English speakers, the Norwegian passive structure is formed in a different way to the English one (by verb inflection instead of a BE auxiliary + participle). This choice ensured that the passive structure in the study could not be learned simply by directly transferring the L1 English structure wholesale.
Sentence stimuli were accompanied by the set of 288 black and white photographs used by Bovolenta and Marsden (Reference Bovolenta and Marsden2021a), which those authors had adapted from materials created by Segaert and colleagues (Menenti et al., Reference Menenti, Gierhan, Segaert and Hagoort2011; Segaert et al., Reference Segaert, Menenti, Weber, Petersson and Hagoort2012). The photographs depicted transitive scenes involving the twelve verbs and four nouns of Yorwegian. Each action (e.g., call) was played out in twelve different agent-patient combinations (man call woman, woman call man, man call boy, etc.), and there were two versions of each combination, enacted by different pairs of actors.
In the learning blocks on Day 1 and 2 (including the target structure test trials on Day 2), participants were exposed to eight verbs. These verbs could only occur with one of the structures (single-structure verbs): four verbs always appeared in the active, the other four always in the passive. Four more verbs were then introduced in the structure testing blocks at the end of Day 2 and 3, and in the grammaticality judgment task. These latter four verbs could occur equally frequently with either structure (alternating verbs). Because participants had not been exposed to them during training, the four alternating verbs used in the tests served as a test of how well participants could generalize their structural knowledge to new instances.
Procedure
Participants took part in the study online over the course of three consecutive days. The average total duration of the study was ∼75 min, with each of the three sessions taking approximately 25 min. On Day 1, Day 2, and Day 3, participants performed an auditory cross-situational learning task (Figure 1), which included both learning trials and structural comprehension test trials. On Day 3, participants also did an auditory grammaticality judgment task and filled in a debriefing questionnaire. All tasks were created using JavaScript library PsychoJS, based on PsychoPy (Peirce et al., Reference Peirce, Gray, Simpson, MacAskill, Höchenberger, Sogo, Kastman and Lindeløv2019). All experimental scripts were hosted and run online through the platform Pavlovia (https://pavlovia.org/). Surveys (to gather data on participants’ language background and awareness of Yorwegian rules at the end of the experiment) were administered using Qualtrics (www.qualtrics.com).

Figure 1. Summary of experimental procedure.
Cross-situational learning task
Participants received no explicit instruction on either the grammar rules or vocabulary of Yorwegian. Participants heard individual sentences in Yorwegian, while two pictures (a target picture and a distractor picture) appeared on screen side by side. Their task was to select the picture that corresponded to the sentence they just heard (the target) by pressing the left or right arrow on their keyboard. Thus initially, responses would be based on guessing, but participants would then gradually gather more evidence to allow them to make more informed choices. There were two types of trials: learning trials and structure test trials (Figure 2).

Figure 2. Example of a learning trial and structure test trial used in the cross-situational learning task. Participants hear a sentence (written version here for display only) and must choose the correct picture by pressing the arrow keys on their keyboard.
In learning trials, the agent, patient, and verb depicted in the distractor picture were selected by the software at random, with the only constraint being that the distractor verb could not be the same as the target verb (to avoid the possibility of participants seeing two pictures depicting the same scene, only enacted by different actors). These trials were designed to expose participants to the language, including co-occurrence patterns between verbs and structures, in a semi-naturalistic way.
In structure test trials, the same nouns and verb were depicted in both target and distractor picture, but with reversed agent and patient roles (e.g., if the target picture depicted The girl interviews the man, the distractor would depict The man interviews the girl). These trials tested whether participants could assign the correct interpretation to each structure (active and passive). The position of agent and patient characters inside the pictures (left/right) was randomized, as was the position of target and distractor pictures on screen (left/right).
Design of trials, blocks, and sessions in the cross-sectional learning task. On Day 1, all participants followed the exact same protocol, with 176 learning trials (11 blocks of 16), evenly split between active and passive sentences. The training items were created from a set of eight “single-structure” verbs, which only ever occurred in one of the two structures (four in the active, four in the passive; Table 1). Learning trials were followed by a structure test block also using single-structure verbs (16 items). At this stage, participants were not given any feedback on their answers, in either the learning or structure test trials.
Table 1. Distribution of verbs used in the cross-situational learning task

On Day 2, participants did 96 learning trials (six blocks of 16). Eight of the trials in each block of 16 were followed by feedback (after the participants made their choice, the correct picture was again displayed in the center of the screen, and the sentence was played again) and then by a structure test trial. Half of the trials that were followed by feedback (i.e., four per block) were normal learning trials, and each structure test trial that followed them simply tested participants’ structural knowledge (“neutral structure test” trials). The other half of the learning trials with feedback (i.e., four per block) was where the surprisal manipulation was implemented: for the surprisal group, these trials used a single-structure verb with the opposite structure (e.g., a formerly [Day 1] “active-only” verb would now be presented in a passive sentence). The corresponding trials in the control group used the appropriately consistent structure (e.g., a formerly [Day 1] “active-only” verb was presented in an active sentence, consistent with the Day 1 learning phase). The structure test trials that followed these manipulated trials (“critical structure test” trials) were aimed at testing immediate priming effects. There were four neutral and four critical structure test trials in each block, for a total 24 neutral and 24 critical trials over the course of the Day 2 session. After the learning phase, participants did a structure comprehension test using novel alternating verbs, which consisted of 48 items (split into three blocks of 16).
On Day 3, a second structure comprehension test with the same alternating verbs as used on Day 2 was administered, also of 48 items over three blocks of 16.
Grammaticality judgment task
After the cross-situational learning task on Day 3, participants did an auditory grammaticality judgment task (a widely used technique—see Plonsky et al., Reference Plonsky, Marsden, Crowther, Gass and Spinner2020) with Yorwegian sentences. They were instructed to listen to each sentence and indicate whether it was a correct sentence in the language they had been learning. After each sentence was played, the words CORRECT and INCORRECT appeared side by side on screen, and participants had to press either the left or right arrow on their keyboard to give a response. Responses were untimed and the next sentence was heard only after participants gave a response. Participants heard a total of 96 sentences, of which 48 were grammatical and 48 ungrammatical. Sentences were evenly distributed between verb types (alternating and single-structure) and structures (active and passive). Ungrammatical active sentences contained the active verbal inflection incorrectly followed by the preposition ka, while ungrammatical passive sentences contained the passive verbal inflection but no preposition (Table 2). While this operationalization of grammaticality and ungrammaticality was arbitrary (as, for example, an active verbal inflection followed by the preposition ka could be labeled as “ungrammatical passive”), the critical distinction was that the structures were “ungrammatical”—albeit in different ways—relative to the language that participants had been exposed to.
Table 2. Sample items from the grammaticality judgment tasks

Debriefing questionnaire
At the end of Day 3, participants filled in a language background and debriefing questionnaire. The first part of the questionnaire included questions on the participants’ educational and language background, including the amount of formal grammar instruction received in the L1, whether participants could speak any foreign languages, and the amount of instruction received in any foreign languages spoken. The second part included specific questions on the experiment itself, aimed at probing participants’ awareness of the structures and of the functional distinction between them (“Did you notice that a new type of sentence was introduced on Day 2 (yesterday’s session)?”, and if Yes, “What were the two types of sentences you learned, and what do you think the difference was between them?”).
Statistical analysis
We analyzed data with mixed-effects modeling implemented in R version 4.0.3 (R Core Team, 2021). Accuracy dataFootnote 2 from structure tests and endorsement data from the grammaticality judgment task were analyzed with generalized linear mixed-effect models (GLMER) for binomial data, using the lme4 package (Bates et al., Reference Bates, Mächler, Bolker and Walker2015).
We used dummy coding for all categorical variables. For fixed effects, the model for structure tests included group (control: 0, surprisal: 1) and structure (passive: 0, active: 1) as fixed predictors. The models for the grammaticality judgment task included group, grammaticality (grammatical, ungrammatical), and verb inflection (active, passive)Footnote 3 as predictors. Target structure tests contained only alternating verbs, whereas the grammaticality judgment task contained both single-structure and alternating verbs. Therefore, endorsement data from the grammaticality judgment task were analyzed in two separate GLMER models: The first model was on alternating verb trials only (ensuring that results could be compared with data from the structure tests, which used alternating verbs only), with group, grammaticality, and verb inflection (active vs. passive) as predictors. The second model included all trials, with verb-structure (mis)match (i.e., whether or not the verb had been used with that inflection during Day 1 training) added as predictor. We also computed d’ scores for the grammaticality judgment task (the difference between correctly accepted grammatical items and incorrectly accepted ungrammatical ones) as a measure of grammatical sensitivity independent of individual bias. We analyzed d’ scores in a multiple linear regression with group and verb inflection as predictors.
When constructing the mixed-effects models, we used the maximal random structure supported by the model, following Barr et al. (Reference Barr, Levy, Scheepers and Tily2013). For each model, we first created a formula containing the maximal fixed effect structure and the maximal random effect structure (random intercepts by subject and item, as well as random slopes for subjects and items by each of the fixed effect predictors, and their interactions). We identified the maximal random structure that would allow the model to converge using the package buildmer (Voeten, Reference Voeten2020). We then used buildmer again on the resulting formula do stepwise backward model selection using likelihood-ratio tests, eliminating fixed effect predictors one by one (starting from higher-level interactions) and only retaining them if they significantly improved model fit. All models were checked for overdispersion and none of them showed signs of being overdispersed. Any post-hoc comparisons were carried out using the emmeans package (Lenth et al., Reference Lenth, Buerkner, Herve, Love, Riebl and Singmann2021). We report the coefficients of the mixed-effects models converted to odds ratios (OR) to provide a measure of effect size, together with the statistical significance of the effects (p values), with α = .05.
In addition to the pre-registered analysis outlined above, we carried out a number of exploratory analyses, which we report together with the corresponding pre-registered analysis (specifying clearly that they are exploratory).
Results
Descriptive statistics for our participants can be found in Table 3. The groups were matched in L2 learning experience, and they did not differ in their awareness of the function of the two Yorwegian structures at the end of the study (operationalized as being able to describe the function of the structures, and/or being able to provide correct translations of sentences using the structures with novel verbs). A full summary of data from the debriefing questionnaire can be found on the OSF repository for this study.
Table 3. Descriptive summary of main data from debriefing questionnaire

* At any level and regardless of how the knowledge was acquired (question: “Do you have any knowledge of any languages in addition to English?”).
Below, we report the results of our statistical analyses. A summary of findings from pre-registered and exploratory analyses can also be found in Table 4; full model outputs can be found in Appendix S2. Error bars in all figures represent 95% confidence intervals.
Table 4. Summary of main statistically significant effects from pre-registered and exploratory analyses

* Verb-structure match: whether verb-structure pairing follows or violates Day 1 verb-structure assignments.
Cross-situational learning task: Structural comprehension
Day 1: Structure test block (single-structure verbs): Baseline structural comprehension test
The structure test at the end of Day 1 took place before the surprisal manipulation was introduced, so we expected both groups to perform similarly. However, we observed significant differences between the groups as the surprisal group showed higher accuracy (Figure 3). We observed a main effect of group (OR = 1.41, 95% CI [1.03, 1.95], p = .034), as well as one of structures (OR = 2.04, 95% CI [1.47, 2.83], p < .001), due to overall higher accuracy for active sentences. We discuss possible reasons for the unexpected differences between groups at baseline in the Discussion (Limitations section).

Figure 3. Average accuracy on Day 1 structure test block (k = 16).
Day 2: Structure test trials during learning (single-structure verbs): Immediate priming test
If high verb surprisal increased immediate priming effects (inverse probability priming), we expected to see a main effect of group in immediate priming test trials, with the surprisal group showing higher accuracy than the control group. We entered data from all target structure test trials during learning (blocks 1–6) in a GLMER model with group and structure as predictors. We observed a main effect of structure, with overall greater accuracy for active sentences (OR = 2.27, 95% CI [1.62, 3.18, p < .001) but no effects of group, meaning that the group difference observed on Day 1 was no longer present (Figure 4). We did not, therefore, observe evidence of immediate priming, nor a visible learning effect over the course of the Day 2 learning task.
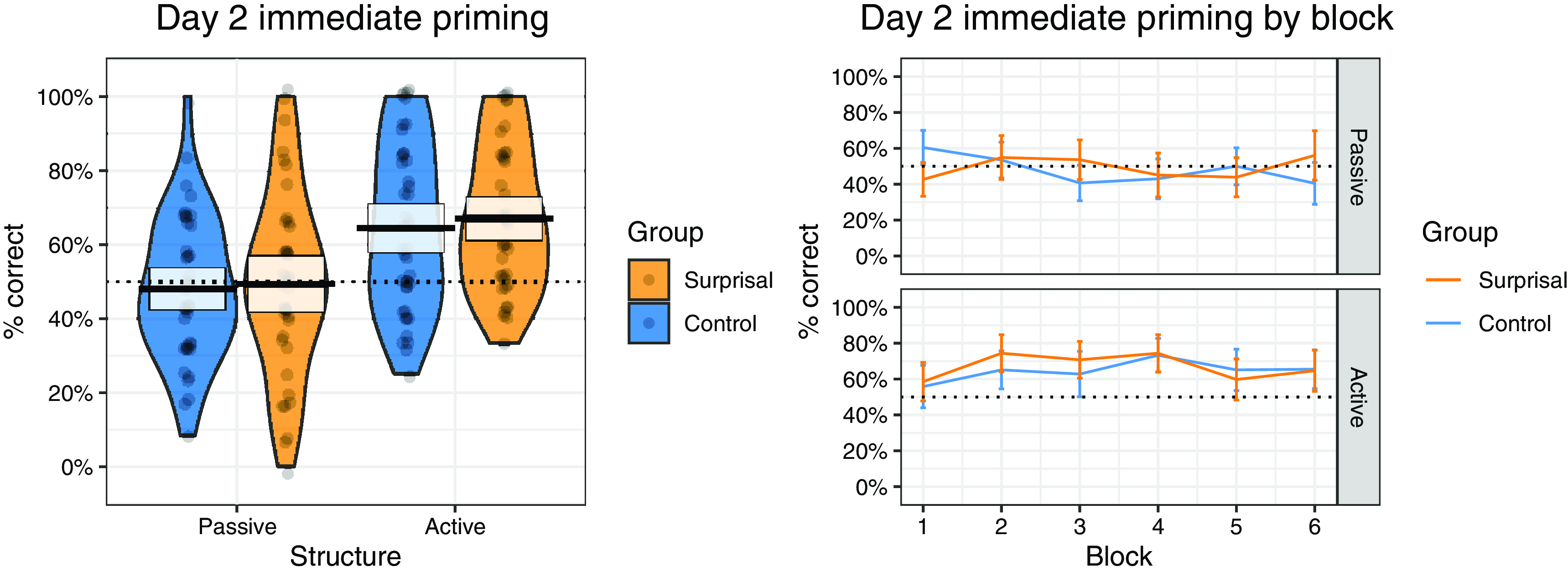
Figure 4. Mean accuracy on structure test target trials during Day 2 learning task (blocks 1–6), aggregated (left panel) and by block (right panel).
Day 2: Structure test blocks (alternating verbs): Same-day structural comprehension test
In comprehension tests following exposure, we hypothesized that if high verb surprisal contributed to adaptation to novel structures, we should see a main effect of groupFootnote 4 , with higher accuracy for the surprisal group relative to control. In the structure test blocks at the end of Day 2 (blocks 7–9), we observed an effect of structure, with higher accuracy for active sentences (OR = 5.61, 95% CI [3.30, 9.54], p < .001) but no significant main effects of group or interactions between group and structure (Figure 5).

Figure 5. Mean accuracy on Day 2 structure test blocks (blocks 7–9 of Day 2 task) and Day 3 structure test blocks.
Day 3: Structure test blocks (alternating verbs): Delayed structural comprehension test
In the delayed comprehension test on Day 3, as in the Day 2 comprehension test, we expected to see a main effect of group, with higher accuracy for the surprisal group relative to control. Although there was a visible trend towards an interaction between group and structure (Figure 5), it was not statistically significant in the pre-registered analysis, which returned only a main effect of structure (OR = 7.70, 95% CI [4.08, 14.54], p < .001).
Given the variability between groups observed on Day 1, we ran an exploratory analysis to get a more sensitive measure of the change in participants’ knowledge from Day 2 to Day 3, adding accuracy on Day 2 test trials as a covariate. The rationale for using these trials as a baseline measure is that they provide the earliest picture of participants’ structural knowledge after the chance for overnight consolidation, just prior to further exposure and the manipulation on Day 2, and it had a higher number of items (24 instead of 16) relative to the Day 1 structure test block. The lack of differences between groups in the structure test trials on Day 2 (Figure 4) suggests that they were not affected by the group manipulation, also rendering them suitable as a baseline measure.
When adding accuracy on Day 2 structure test trials as a covariate to the model, we observed significant interactions between group and structure (OR = 0.28, 95% CI [0.09, 0.87], p = .028) and between group and Day 2 accuracy (OR = 2.04, 95% CI [1.18, 3.52], p = .010)Footnote 5 . Post hoc comparisons showed that the interaction between group and structure was due to a significant difference between groups on the passive items (OR = 2.63, 95% CI [1.21, 5.69], p = .014) but not on the active items. Therefore, we observed a significant effect of the surprisal manipulation on comprehension, which affected passive items but not active items. Post hoc tests on the interaction between group and Day 2 accuracy showed that the effect of Day 2 accuracy on Day 3 accuracy was significant for both groups (surprisal: β = 1.51, 95% CI [1.12, 1.90], p < .001; Control: β = 0.80, 95% CI [0.41, 1.18], p < .001), but the effect was smaller in the Control than in the surprisal group (β = −0.71, 95% CI [−1.26, −0.17], p = .010).
Aural grammaticality judgment task: Structural knowledge and verb selectional restrictions
If high verb surprisal contributed to adaptation to the novel structures, we expected the surprisal group to show better structural knowledge relative to control. In the grammaticality judgment task, we therefore expected to see a group × grammaticality interaction: the surprisal group should be more likely to endorse grammatical sentences as grammatical, and less likely to endorse ungrammatical ones as grammatical relative to control. Analyzing endorsement of items with alternating verbs (i.e., the four alternating verbs that were introduced in Day 2)Footnote 6 , we observed significant two-way interactions between group and verb inflection (OR = 1.51, 95% CI [1.08, 2.21], p = .017), between grammaticality and group (OR = 1.77, 95% CI [1.10, 2.87], p = .02), and between grammaticality and verb inflection (OR = 0.30, 95% CI [0.21, 0.42], p < .001)Footnote 7 . Overall, the surprisal group showed higher endorsement of all item types compared to control, apart from for ungrammatical passive sentences, i.e., sentences with the passive verb inflection but n ka marker (Figure 6). This means that participants in the surprisal group were more accurate in accepting all grammatical sentences, but they were also less accurate than control in rejecting ungrammatical active ones.

Figure 6. Average endorsement in the grammaticality judgment task (all items), by sentence grammaticality.
We analyzed d’ scores (Figure 7) to assess sensitivity to grammaticality. This analysis included all items (both the four alternating and the eight structure-specific verbs), as per the pre-registration. When entering the scores in a linear regression with group and verb inflection as predictors, we observed a significant effect of group (b = 0.43, 95% CI [0.06, 0.80], p = .023), due to higher d’ scores among the surprisal group, as well as a main effect of verb inflection (b = −1.11, 95% CI [−1.48, −0.74], p < .001), due to higher discrimination accuracy for sentences in the passive inflection. The results thus show a significant effect of the surprisal manipulation on the development of structural knowledgeFootnote 8 .

Figure 7. Mean d’ scores in grammaticality judgment task by group and verb inflection.
We then analyzed endorsement for structure-specific items, to test our secondary hypothesis that the surprisal group would be more accepting of verb-mismatched items relative to the control group, as they would have adapted to be more accepting of verbs alternating between either structure to a greater extent than control (Figure 8). Following the pre-registered analysis, we added verb-structure match to the model together with group, grammaticality, and verb inflection. We found a three-way interaction between group, verb-structure match, and inflection (OR = 0.25, 95% CI [0.15, 0.40], p < .001). Post hoc comparisons showed that participants in the surprisal group were more likely than those in the control group to accept verb-mismatched items using the passive inflection (OR = 1.88, 95% CI [1.31, 2.68], p < .001) (i.e., those verbs that had only been encountered with the active structure during training, with the exception of surprisal trials), in line with our hypothesis. Participants in the surprisal group were also more likely than control to endorse verb-matched items with the active inflection, which was not predicted: OR = 2.62, 95% CI [1.86, 3.77], p < .001. Results for the passive structure suggest that experiencing prediction error during learning led participants to revise their expectations. Again, this was limited to the passive structure only, mirroring findings from the Day 3 structural comprehension test and d’ scores.

Figure 8. Breakdown of endorsement rates in grammaticality judgment task based on match between verb type and inflection (structure) used, aggregated across grammatical and ungrammatical items. Single-structure verbs are divided into “Match” (appropriate verb for that structure) and “Mismatch” (verb that had been used with the opposite structure during learning phase on Day 1).
Discussion
We had hypothesized that being exposed to high-surprisal input would generate prediction error and lead to inverse frequency priming and adaptation effects in the surprisal group relative to control. Specifically, we expected the surprisal group to show higher accuracy in both immediate and delayed tests of structural comprehension, and in a delayed grammaticality judgment task.
Our results provide partial support for our hypothesis. We did not observe any immediate priming effects, nor any effects in a structural comprehension test immediately following training on Day 2. On Day 3, we observed significant effects of surprisal on structural comprehension, although these only emerged in an exploratory analysis with Day 2 accuracy added as covariate (and not in the pre-registered analysis or an alternative analysis with Day 1 accuracy as covariate, possibly due to the unexpected between-group differences found on Day 1).
By contrast, findings from the grammaticality judgment task were more robust. We observed significant effects of surprisal on endorsement and accuracy (d’) in grammaticality judgments (which were replicated when controlling for Day 1 and Day 2 accuracy) and on the strength of verb selectional restrictions. These results indicate that the surprisal condition had promoted knowledge of grammatical structure form (i.e., the combinations of noun order, verb inflection, and preposition use characterizing the active and passive structure) and had also led learners to update their expectations for verb-structure co-occurrences. The results from structural comprehension tests and grammaticality judgments suggest that experiencing high-surprisal input increased adaptation to newly encountered structures, promoting the establishment and development of structural representations. Unexpectedly, the effects—in both structural comprehension tests and grammaticality judgment tasks—were only observed on the passive structure, even though the manipulation was applied to both structures. We discuss possible interpretations for these findings below, as well as potential limitations of the current study.
Effect of surprisal on passive structures only
In this study, we observed an effect of verb surprisal, but only on the passive structure—even though both structures underwent the surprisal manipulation. This finding was not predicted by our hypothesis. One possibility is that this finding may simply be due to a ceiling effect for active sentences. We can speculate that active sentences, being by far the more frequent structure in the participants’ native language (English), would also be easier to acquire than the passive. The Yorwegian active structure is also constructed in the same way as the English one (unlike the passive), yielding a potential L1 transfer advantage. Additionally, a preference for the active structure is not only a feature of English, but has been attested cross-linguistically in children (Estevan, Reference Estevan1985; Jakubowicz & Seguí, Reference Jakubowicz and Seguí1980; Maratsos et al., Reference Maratsos, Fox, Becker and Chalkley1985). Finally, the entities that served as subjects and objects in our study were all animate and therefore likely to be interpreted as agents during sentence processing (Hare et al., Reference Hare, Elman, Tabaczynski and McRae2009; Kim & Osterhout, Reference Kim and Osterhout2005). Therefore, participants may have defaulted to an active interpretation, leading to high accuracy for active sentences and generally low accuracy for passive sentences (while accuracy was higher in the surprisal group, it should be noted that both groups were below chance level in their comprehension of passives).
However, data from grammaticality judgments on Day 3 suggest a more complex picture: while accuracy in comprehension tests was always significantly higher for active sentences, accuracy in the grammaticality judgment task (d’ scores) was significantly lower for active sentences, in both groups. Participants in both groups were equally likely to endorse active sentences regardless of their grammaticality, suggesting that they uncritically tended to accept items that contained the active verbal inflection (-at)Footnote 9 . The effect of high-surprisal input on verb selectional restrictions, too, only seemed to apply to endorsement of passive items. Relative to the control group, participants in the surprisal group became more accepting of passive sentences containing active-only verbs (“mismatch” items in the passive condition), regardless of grammaticality, but they did not become more accepting of active sentences with passive-only verbs (“mismatch” items in the active condition). This suggests that being exposed to mismatched verbs during the surprisal phase had led participants to revise their expectations for the passive structure (becoming more accepting of previously unattested verbs appearing in this structure), but not for the active structure.
Taken together, these data suggest a striking possibility: that participants did not develop a distinct structural representation for the Yorwegian active structure, due to its closeness to the default structure in their L1. While the passive structure was different from the English passive (most notably, due to the lack of BE auxiliary), the active structure could be mapped directly onto the English active structure. Therefore, it is possible that in comprehension tests, participants simply defaulted to an active interpretation (assigning subject role to the first noun, and object role to the second noun), resulting in high accuracy for active sentences and generally low accuracy in passive ones. But in grammaticality judgment tasks, they showed no sensitivity to morphosyntactic violation in active sentences, due to missing structural representations. For the same reason, encountering active sentences with passive-only verbs did not seem to elicit prediction error on Day 2 in the surprisal group (and consequently, no revision of verb selectional restrictions was observed).
A distinct but related possibility is that the presence of the active structure in the L1 led participants to generalize it, despite limited input. If participants saw the Yorwegian active as an instance of active (similar to their L1), then they would likely base their interpretation of the structure on distributional statistics from their L1, as has been observed in previous studies on adaptation in L2 speakers (Jackson & Ruf, Reference Jackson and Ruf2017; Montero-Melis & Jaeger, Reference Montero-Melis and Jaeger2020). This hypothesis is compatible with research on the acquisition of dative alternation in English, which follows different trajectories in L1 and L2 learners (Conwell & Demuth, Reference Conwell and Demuth2007). Although double object datives are learned sooner in L1 acquisition, prepositional datives are acquired earlier by L2 learners. Although there appears to be a general preference for prepositional object datives overall among L2 learners, some evidence also suggests that a higher prevalence (proportional frequency) of prepositional datives in the learners’ L1 could contribute to earlier acquisition of the same structure in the L2 (Agirre, Reference Agirre2015; Hawkins, Reference Hawkins1987). Similarly, if participants in our study relied on the statistical distribution of the active structure in English, where the structure is highly productive, they may have been more likely to generalize the Yorwegian active structure to new verbs too, even after limited exposure. By contrast, because no English version of the Yorwegian passive exists, the Yorwegian passive could only be acquired via item-specific learning, which would be determined by its distribution in Yorwegian. Therefore, participants may have developed stronger verb selectional restrictions for the Yorwegian passive structure than for the active one, potentially experiencing greater prediction error when these restrictions would be violated.
This explanation is compatible with theoretical accounts of the acquisition and generalization of syntactic rules. According to the Sufficiency principle (Yang & Montrul, Reference Yang and Montrul2017), a rule applying to a syntactic category becomes productive (i.e., there is a shift from item-based learning to generalization to the whole category) when the number of items following that rule passes a mathematically defined threshold (the difference between total occurrences of the category and the natural logarithm of the same value). In our case, the number of items (i.e., individual verbs) observed with the Yorwegian passive structure would not be sufficient for participants to generalize the rule (i.e., to generalize the Yorwegian passive structure to new verbs). By contrast, if participants perceived Yorwegian active sentences as instances of the active structure which they were already familiar with from their L1 English, then the number of items they had witnessed with that structure would comprise not only Yorwegian active verbs, but all English verbs they had ever encountered in the active form—a sufficient number of items to generalize the Yorwegian active structure. Under this interpretation, learners would have acquired the intended verb selectional restrictions only for the passive structure, generating prediction error when these were violated, and consequently error-based learning in the surprisal group that was restricted to the passive structure.
Lack of immediate priming effects
The other unexpected finding in our study was the fact that we did not observe any immediate effects of the surprisal manipulation, and yet we observed delayed effects. We had hypothesized that, if an error-based learning mechanism such as that specified by the Dual-Path model (Chang et al., Reference Chang, Dell and Bock2006) was driving learning, we should see both immediate (priming) and delayed (adaptation) effects of prediction error. Against our predictions, however, we did not observe significantly higher accuracy on the structure test trials immediately following surprising trials, suggesting that the manipulation did not produce any immediate priming effects.
On the one hand, our results are compatible with previous findings from other studies. In their study on adaptation to alternative dative constructions (prepositional vs. direct object dative), Fazekas et al. (Reference Fazekas, Jessop, Pine and Rowland2020) observed adaptation following exposure to low-frequency verb-structure pairs, but no immediate inverse probability priming effects. They observed a numerical trend towards priming for adults, but not for children, suggesting that well-established representations may be needed for immediate priming effects to be elicited by prediction error. Our findings, too, suggest that it is possible for participants to experience adaptation without having shown immediate priming effects.
On the other hand, the reason for the lack of immediate priming effects in our study may lie in the specific measure we chose to measure priming, which was structural comprehension. In an artificial language learning study, Weber et al. (Reference Weber, Christiansen, Indefrey and Hagoort2019) observed direct priming in structural comprehension starting only from the third day of an artificial learning task, while priming on read-aloud times emerged earlier in the study. Therefore, we cannot rule out the possibility that immediate priming effects may have emerged had we used a different test. Future research should investigate this possibility, using different tests of priming in order to gain a better picture of inverse frequency priming effects and how they interact with the strength of existing representations, as well as the measures used to assess priming.
Finally, the lack of immediate priming effects may simply be indicative of the fact that the advantage enjoyed by the surprisal group was not due to implicit error-based learning, but to other mechanisms—a possibility we explore below.
Alternative mechanisms for the effect of surprisal
There are a number of mechanisms by which higher surprisal could have led to greater accuracy in the surprisal group, besides implicit error-based learning. While the aim of the current study was to study the effects of prediction error on the acquisition of structures, we did not directly measure prediction error (e.g., with an online methodology such as eye-tracking). Instead, we manipulated surprisal (statistical properties of the input) with the assumption that it would generate prediction error. Therefore, while our findings are at least partially compatible with an error-based learning mechanism, they could also be explained by other types of mechanism.
One possibility is that participants were not processing the verbs they saw during training as inflected forms, but rather as whole lexical items. This would be compatible with their experience of their L1 English, where forms with identical onsets but different endings can be distinct verbs (e.g., cont-est and cont-rast). Additionally, if participants always interpreted the first noun as the agent, the preposition ka could be interpreted as part of an active sentence, such as introducing a prepositional complement (e.g., “The boy talks to the girl”). Crucially, this would make the presence of ka something related to the idiosyncratic meaning of each verb, rather than bearing a systematic relationship with a particular verb ending which could occur with multiple verbs. Under this interpretation, the surprisal group would have subjectively experienced a wider range of verbs during training, rather than the same set of verbs in more syntactic contexts. This is compatible with the findings that participants in the surprisal group have higher acceptance of ungrammatical as well as grammatical active sentences, because they may simply perceive the ungrammatical forms as new verbs (new lexical items), with a new meaning. It is also compatible with the fact that they were more accepting of active mismatched verbs (which they had already encountered during Day 2 training). However, it would not explain why the effects were structure-specific: the surprisal group was more accepting of ungrammatical active sentences, but not ungrammatical passive ones; when breaking down endorsement by verb type, the surprisal group was more accepting of mismatch in passive sentences, but not in active ones. Therefore, while it is possible that participants learned the inflected forms as whole verbs (indeed, that would have been a necessity at the start of the training, before any patterns could begin to be abstracted), results also suggest that participants eventually developed sensitivity to the fact that different systematic patterns existed in the language. We acknowledge, however, that it is possible that the surprisal group developed a sensitivity to a lexicalized string “es+ka” being acceptable, rather than necessarily having established a (purely) morphosyntactic structure.
A second possibility is that abstraction itself was aided by the greater range of exemplars to which the surprisal group was exposed. More precisely, participants in this group heard a wider range of verbs in each syntactic context (because they heard the single-structure verbs in both kinds of structures), compared to control. There is evidence that variability improves learning in statistical learning tasks (Bulgarelli & Weiss, Reference Bulgarelli and Weiss2021; Gómez, Reference Gómez2002). Gómez (Reference Gómez2002) found that the acquisition (assessed by grammaticality judgments) of non-adjacent dependencies between syllables presented in an auditory statistical learning task benefitted from greater variability in the strings intervening between syllables. Given evidence that increased variability aids learning, it is possible that the surprisal group benefitted from exposure to a wider range of verbs in each syntactic context (due to hearing the violation trials, whereas the control group did not), and this could have helped them to isolate the abstract structures from individual lexical items.
Finally, it is also possible that prediction error was indeed the cause of the observed differences between groups on Day 3, but this prediction error was not due to implicit error-based learning, and so was not observable in the immediate structural comprehension test. Instead, one possible mechanism we may have observed is one-shot declarative learning, i.e., the phenomenon that novel associations are better remembered if they violate an established pattern (Brod et al., Reference Brod, Hasselhorn and Bunge2018; De Loof et al., Reference De Loof, Ergo, Naert, Janssens, Talsma, Van Opstal and Verguts2018; Greve et al., Reference Greve, Cooper, Kaula, Anderson and Henson2017, Reference Greve, Cooper, Tibon and Henson2019). In language acquisition, the effect of one-shot declarative learning has been investigated in the context of vocabulary learning, both in children (Gambi et al., Reference Gambi, Pickering and Rabagliati2021; Stahl & Feigenson, Reference Stahl and Feigenson2017) and adults (Gambi et al., Reference Gambi, Pickering and Rabagliati2021). While most of the evidence comes from vocabulary learning, however, we cannot discount the possibility that one-shot declarative learning may also contribute to the development of new structural knowledge, albeit indirectly. In usage-based accounts of language acquisition, structural knowledge is thought to emerge through abstraction from individual learned exemplars (Ellis et al., Reference Ellis, Römer and O’Donnell2016). Therefore, a mechanism such as one-shot declarative learning, which aids the formation of individual memories of specific instances of structure, may be hypothesized to indirectly contribute to the development of abstract structural knowledge by providing bases for generalization. To test this hypothesis, future replications of this study would need to include tests of item memory for the specific sentences heard during the training phase (see one attempt of doing this in our earlier study in Appendix S1).
Another possibility is that high-surprisal input engaged learners’ attention, leading to better learning. In Bovolenta and Marsden (Reference Bovolenta and Marsden2021a), it was hypothesized that the observed learning effects could be due to attention raising as a function of experimental design: The feedback paradigm used for surprisal participants, which involved juxtaposing active and passive structures, could have drawn their attention to the difference between structures. The present study did not involve any juxtaposition of structures, so the same explanation could not apply. However, if surprisal caused participants to experience prediction error it may still lead to global attention raising (i.e., greater attention to the task as a whole) and overall better learning. For instance, Fitneva and Christiansen (Reference Fitneva and Christiansen2011, Fitneva & Christiansen, Reference Fitneva and Christiansen2017) found that accidentally experiencing prediction error (by forming incorrect label-referent mappings at the start of a cross-situational vocabulary learning task) led to overall higher learning rates in adults. The important thing to note is that the effect applied to the whole vocabulary set, not only to the words that participants had initially assigned to the wrong referent. This observation would not be compatible with implicit error-based learning, but rather suggests that higher surprisal may have led to greater attention and better encoding of information overall. The same mechanisms could potentially have played a role in the present study.
It should be noted that both of these potential mechanisms—one-shot declarative learning and attention raising—are “global,” in the sense that they should in principle apply to all of the sentences affected by the surprisal manipulation (which were both active and passive), and would consequently be expected to boost learning of both structures. Therefore, these explanations seem at odds with our finding that effects on structural knowledge (accuracy measures) seem to emerge primarily on the passive structure. However, any of the potential reasons we explored for the lack of learning effects on the active structure (ceiling effects, L1 transfer) could of course still apply and so partially counteract any learning advantage derived from surprisal. Thus, this could account for the asymmetrical pattern of results we observed, even in the presence of a global learning boost.
Descriptive data from the debriefing questionnaire (Table 3) show that neither group was more likely than the other to develop awareness of the distinction between active and passive. Intuitively, one might expect greater global attention to lead to greater awareness of the rules; however, that may not necessarily be the case: research on implicit language learning shows that engaging learners’ attention can affect learning even in the absence of awareness, and being unable to articulate explicit rules after a short learning study does not eliminate the possibility that attentional levels were heightened during exposure (e.g., Leung & Williams, Reference Leung and Williams2006; Marsden et al., Reference Marsden, Williams and Liu2013).
Limitations
One notable limitation of our study was the difference observed between groups in the structure comprehension test at the end of Day 1, before the experimental manipulation was introduced. This difference (higher accuracy for the surprisal group on active sentences in the structural comprehension test) was no longer visible on Day 2 and went against the pattern consistently observed elsewhere in the experiment (where the difference between groups was on passives). In addition, the effect of group observed in the pre-registered analysis for the grammaticality judgment task was replicated in exploratory analyses controlling for both Day 1 and Day 2 accuracy (while the effect on structural comprehension only emerged when controlling for Day 2 accuracy). Therefore, we think it is unlikely that the learning effects we observed—especially in the grammaticality judgment task—were due to baseline differences between groups, but can be ascribed instead to the experimental manipulation on Day 2.
Nevertheless, observing a difference between groups on Day 1 was unexpected, given our random sampling. One tentative explanation for this difference may lie in the fact that it is more difficult to avoid attrition in online data collection, and attrition may induce self-selection bias in terms of which participants complete the entire study. We experienced attrition rates of roughly 30% and all attritors were eliminated from the final dataset analyzed. While most attrition was due to participants dropping out after the Day 1, a few dropped out after Day 2. If the surprisal condition on Day 2 was perceived as more difficult, it could have made a particular subset of “lower performing” surprisal group participants more likely to abandon the study after Day 2 (thus leaving more of the “higher performers” from Day 1 remaining in the dataset), relative to those in the control group. However, this is a highly speculative account, and it does not explain why the initial difference between groups disappeared on Day 2. Nevertheless, we highlight this potential challenge for multi-session online research.
Conclusion
Overall, our findings indicate an effect of surprisal on the development of abstract structural knowledge. Participants who were exposed to unexpected verb-structure combinations showed higher accuracy in comprehension of the passive in delayed tests and on grammaticality judgments in delayed tests. Therefore, even at the very earliest stages of L2 acquisition, encountering a structure in an unexpected context can promote the development of structural representations. The delayed effects we observed are compatible with error-based learning accounts of language acquisition. However, we only observed the effects of group on the passive structure, even though both structures had been affected by the experimental manipulation. We suggested potential reasons for the lack of an effect on the active structure, which include ceiling effects and L1 structural biases—further research will be needed to examine these potential reasons. Also contrary to our expectations, we did not observe any immediate priming effects, which would be predicted by an implicit error-based learning account. The lack of immediate effects could be due to the fact that such effects may depend on more mature structural representations being already established. However, it could also indicate that a different mechanism—something other than implicit error-based learning, such as a global heightened awareness in the surprisal condition—was responsible for our findings. Therefore, further research is needed to determine the precise nature of the effect generated by our experimental manipulation and shed more light on the potential role of prediction error in L2 acquisition.
Replication package
All materials, data, and analysis code for the experiments in this article can be found at https://doi.org/10.17605/OSF.IO/EU4AV and on the IRIS database (https://www.iris-database.org/).
Competing interests
The authors declare none.