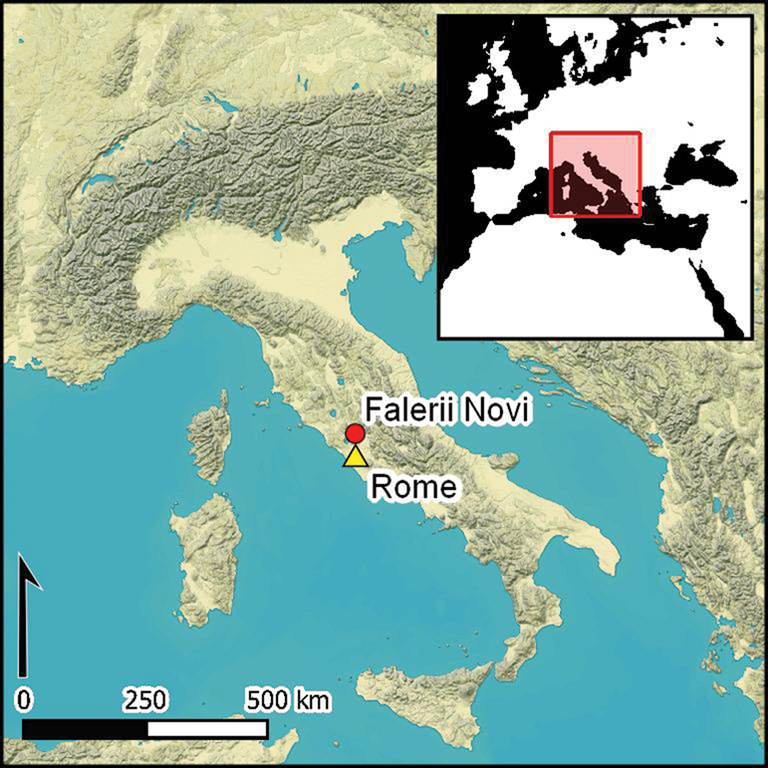
Introduction
Ancient Rome lay at the centre of a network of cities that played a pivotal role in the administration, social organisation and economy of its empire. By the first century AD, there were approximately 2000 cities across the Roman world (Scheidel et al. Reference Scheidel, Morris and Saller2007: 78) and understanding them is central to our knowledge of this period. Cities, however, are inherently large and only very small sample areas can ever be excavated. Furthermore, as many Roman cities have continued in occupation to the present day, excavations are rarely the result of rational research designs. More often, the evidence is acquired by chance, such as in developer-led excavations brought about by development threats within modern townscapes, and shaped by later phases of occupation. Consequently, we are reliant on the evidence from a small number of extensively explored Roman urban sites that have been the subject of large-scale clearance or major excavation campaigns, such as Ostia and Pompeii. These sites dominate the modern archaeological literature, but they can hardly be considered as typical cities.
Over the last 20 years, our understanding of ancient towns has been revolutionised by the use of remote-sensing techniques (Linck et al. Reference Linck, Fassbinder and Buckreuss2012; Vermeulen et al. Reference Vermeulen, Keay, Burgers and Corsi2012; Doneus et al. Reference Doneus, Verhoeven, Atzberger, Wess and Ruš2014), which have enhanced our knowledge of the overall topography of these sites, especially when combined with excavation and surface survey. For example, magnetometry (predominantly fluxgate gradiometry) has produced impressive results, mapping complete Roman towns and transforming the evidence base for Roman urban studies (see Johnson & Millett Reference Johnson and Millett2013: 4–6; Campana Reference Campana2018: 7–9). Gradiometer surveys, however, usually provide only a composite 2D image of near-surface features and, although they can provide spectacular plans of the upper layers of urban sites, they generally give only limited information about the deeper, earlier levels. Nevertheless, at some sites, such as Falerii Novi (Fabrica di Roma, Lazio, Italy), interrogation of magnetometry data has been able to generate new models for the early development of Roman towns (Keay et al. Reference Keay, Millett, Poppy, Robinson, Taylor and Terrenato2000; McCall Reference McCall2007; Millett Reference Millett, Roth and Keller2007; Hay et al. Reference Hay, Johnson, Keay and Millett2010; Wallace-Hadrill Reference Wallace-Hadrill, Eckardt and Rippon2013).
Ground-penetrating radar (GPR) is a reliable method that provides high-resolution 3D images of buried structures. It relies on the reflection of electromagnetic (radio) waves at transitions between materials of a different dielectric permittivity. The amplitudes and travel times of the reflected waves are measured, resulting in vertical radargrams or profiles. Using several parallel radargrams, horizontal slices at different depths (‘time-slices’ or ‘depth-slices’) can be created. Whereas traditionally a small, single antenna has been moved manually over the ground surface, over the last decade, multi-channel GPR arrays have been developed (Linford et al. Reference Linford, Linford and Payne2015)—usually now towed by an all-terrain vehicle. Combined with real-time positioning using a global navigation satellite system receiver or a tracking total station, GPR now allows for rapid survey of large areas, while maintaining a high sample density (Grasmueck et al. Reference Grasmueck, Weger and Horstmeyer2005).
Recent work has demonstrated the value of GPR survey on Roman urban sites (e.g. Verdonck & Taelman Reference Verdonck, Taelman, Corsi and Vermeulen2012; Jardel et al. Reference Jardel, Schmidt, Dabas, Sala, Jennings, Gaffney, Sparrow and Gaffney2017; Lockyear & Shlasko Reference Lockyear and Shlasko2017), but these projects are predominantly small scale, covering no more than a few hectares. Since 2015, however, we have deployed GPR on a much larger scale to generate high-resolution images of two complete greenfield Roman towns in Italy: Interamna Lirenas and Falerii Novi (https://www.classics.cam.ac.uk/research/projects/beneath-the-surface-of-roman-republican-cities). To explore the value of this approach, this article presents the methods and first results of this research at Falerii Novi.
Although such rapid data collection allows entire Roman cities to be mapped at an unprecedented level of detail, interpretation of these large datasets still relies largely on visual analysis and the manual digitisation of anomalies. These traditional, time-consuming interpretative methods are no longer able to exploit fully the potential of geophysical prospection, and here we propose possible ways forward. First, the combination and fusion of different geophysical datasets can result in an enhanced level of interpretation. We illustrate this with an example from Falerii Novi, in which GPR and magnetometry survey data are visualised in a single image by assigning the two datasets to different colour channels (RGB compositing). Second, computer-aided interpretation provides a means of handling large data volumes in a more efficient and objective way. We discuss one class of methods, based on mathematical morphology. Finally, we address the issue of how to publish complex, large-scale surveys that yield vast quantities of archaeological data.
Field site
Located approximately 50km to the north of Rome, Falerii Novi has a walled area of 30.5ha. The town was founded in 241 BC, following the destruction by Rome of the nearby Faliscan centre of Falerii Veteres. Occupation at Falerii Novi continued through Roman times and down to the early medieval period (sixth to seventh centuries AD). It was one of the first Roman towns to be subjected to a complete fluxgate gradiometer survey (Keay et al. Reference Keay, Millett, Poppy, Robinson, Taylor and Terrenato2000; Hay et al. Reference Hay, Johnson, Keay and Millett2010), providing a very clear plan of the entire site (Figure 1). The town plan was informed by the layout of contemporaneous colonies such as Cosa (273 BC), while integrating elements of its Faliscan predecessor (Millett Reference Millett, Roth and Keller2007). Falerii Novi was selected for the current project, as this earlier magnetometry survey provides a control for, and a complement to, the new GPR data.

Figure 1. Falerii Novi fluxgate gradiometer survey (Keay et al. Reference Keay, Millett, Poppy, Robinson, Taylor and Terrenato2000); data range −30nT (white) to +30nT (black) (aerial photograph: Google Earth; image by L. Verdonck).
Methods
Field methods, equipment and data processing
A summary of the instruments and parameters used for the data acquisition is provided in the online supplementary material (OSM). The GPR network, towed by an all-terrain vehicle, comprises 15 500MHz antennae (Figure 2a). This frequency has previously proved effective for the prospection of Roman urban sites (e.g. Verdonck & Taelman Reference Verdonck, Taelman, Corsi and Vermeulen2012). As the width of one antenna housing is ~0.25m, the arrangement of the antennae in two offset rows results in a vertical profile spacing of 0.125m (Figure 2b). In order to meet sample density requirements (Grasmueck et al. Reference Grasmueck, Weger and Horstmeyer2005; Verdonck et al. Reference Verdonck, Taelman, Vermeulen and Docter2015), a second pass was made, reducing the transect spacing to 0.0625m (Figure 2b).

Figure 2. a) GPR system used at Falerii Novi; b) antennae mounted in two rows, with the spacing between profiles at 0.125m (red lines). Two passes were made, following theoretical lines 0.0625m apart (grey lines). The second pass allowed further reduction of the transect spacing to 0.0625m (green lines). M = theoretical midpoint of the array where the global navigation satellite system or total station prism is mounted (image by L. Verdonck).
GPR performance is dependent on the electrical conductivity of the soil, which is affected by the quantity of dissolved salts, clay content and soil moisture (Daniels Reference Daniels2004). At Falerii Novi the generally dry conditions in the summer months were well suited to GPR survey, but occasional rainfall increased soil conductivity and limited GPR penetration depth. Following rain, up to seven days were needed before the ground was sufficiently dry to yield optimal data quality and maintain interpretative potential.
We followed a standard GPR data-processing workflow (see the OSM). Background removal (the subtraction of the average of all traces in a profile from each individual trace) did not entirely remove stripes in the time-slices caused by different amplitudes recorded on different network channels. These stripes were suppressed by calculating the average of the data recorded by each channel within a swath and then equalising these average values. Subsequently, migration (Verdonck et al. Reference Verdonck, Taelman, Vermeulen and Docter2015) improved lateral resolution, as illustrated in Figure 3.

Figure 3. a) GPR time-slice, between 19–20ns (0.85–0.90m); b) migration enhances the resolution so that data become more easily interpretable. Arrows indicate locations with improved resolution (e.g. a structure near 1 can be more certainly interpreted as a drain after migration) (image by L. Verdonck).
Manual mapping of anomalies
The output is represented as a series of time-slices, which map the GPR data as a series of images at successive depths below the surface (Figure 4). These time-slices can be animated to provide a 3D impression of sub-surface features (see the OSM). For a more detailed archaeological analysis and interpretation, however, we first examined each separate time-slice individually and manually mapped anomalies using GIS (ESRI ArcGIS 10) (see figures in the OSM). As a separate subsequent stage, we interpreted the anomalies in terms of architectural features, interpolating walls and identifying surfaces, while attempting to understand the features in the context of known architectural forms, such as temples and baths. Overlaying the resulting interpretative plans of features at different depths forms the basis for the discussion of the urban topography and site development below.
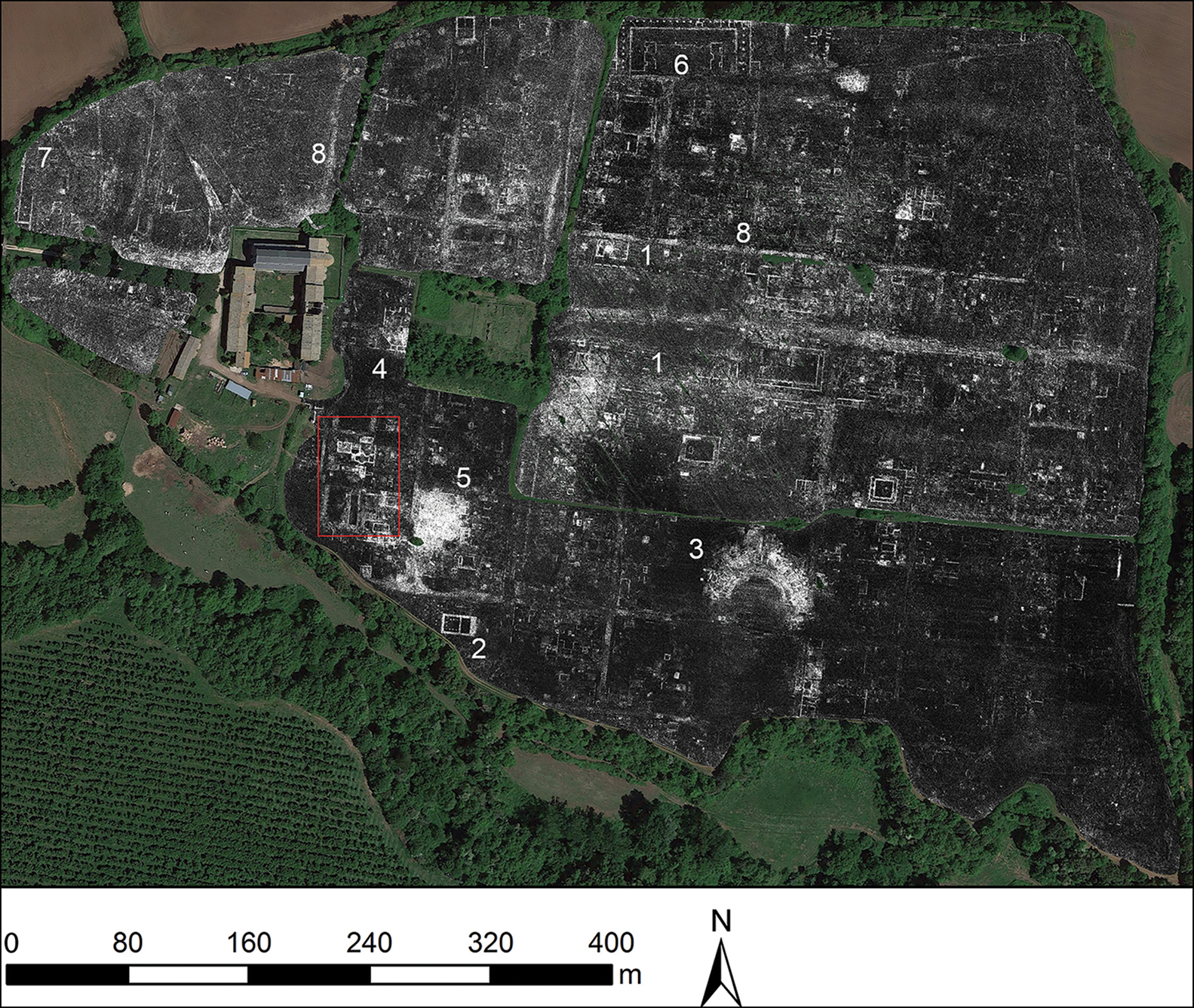
Figure 4. GPR time-slice, at an estimated depth of 0.80–0.85m. The red rectangle indicates the location of Figure 5 (aerial photograph: Google Earth; image by L. Verdonck).
Illustrating the results
Our assessment of the Falerii Novi results is at an early stage, but two conclusions are already clear. First, the high resolution of the data and the ability to distinguish features at different depths provide a much stronger foundation for understanding the town than was previously possible. Second, comparing the GPR results with those from the earlier magnetometer survey (Figures 1 & 4; Keay et al. Reference Keay, Millett, Poppy, Robinson, Taylor and Terrenato2000; Hay et al. Reference Hay, Johnson, Keay and Millett2010) shows how neither method is able to produce a complete picture of the archaeology; the forum tabernae (shop units) appear, for example, in the magnetic data, but not in the GPR survey (Figure 4.1). This underlines the need to deploy complementary prospection methods and to integrate the results.
Here, we confine discussion to an overview of the full plan, alongside a more detailed case study of one sample area. Taking the overall dataset first (Figure 4), the high resolution of the data allows us to identify many individual structural elements (e.g. columns), as demonstrated by the temple to the west of the south gate (Figure 4.2), permitting detailed architectural analysis previously only possible through excavation. The GPR data also help with a problem encountered with the magnetometer survey, whereby building rubble may mask structural detail; the GPR survey provides a clearer view. So, although the theatre is identifiable in the magnetometry survey (Figure 1), analysis of the GPR data at different depths allows the theatre's structural form to be understood in detail (Figure 4.3). Such information reveals how individual buildings developed through time. In particular, the identification in the GPR data of floor surfaces and places where stone robbing has removed walls (Figure 5.1) allows building plans to be understood more fully.

Figure 5. GPR time-slice (case study area), at an estimated depth of 0.75–0.80m (image by L. Verdonck).
GPR survey at Falerii Novi has revealed previously unrecorded public buildings, such as a temple (Figure 4.2), a macellum or market building (Figure 4.4) and a bath complex (Figure 4.5). While these buildings fall within the expected repertoire of a Roman city, some are architecturally sophisticated—more elaborate than would usually be expected in a small town. More unexpected were two very large structures adjacent to the walls. Immediately to the east of the north gate is an enclosure defined on three sides by a substantial porticus duplex (covered passageway with central row of columns) approximately 90 × 40m in size (Gros Reference Gros1996: 96), opening onto the street (Figure 4.6). A pair of structures, each with a central niche, face each other within the interior of the complex. While we know of no direct parallel to this structure, this was evidently a public monument. Its porticus is similar to that of another newly discovered structure, immediately to the north of the west gate, which defines the temenos (enclosure) of the capitolium (temple) identified during the previous magnetometry survey (Figure 4.7; Keay et al. Reference Keay, Millett, Poppy, Robinson, Taylor and Terrenato2000: 11–14).
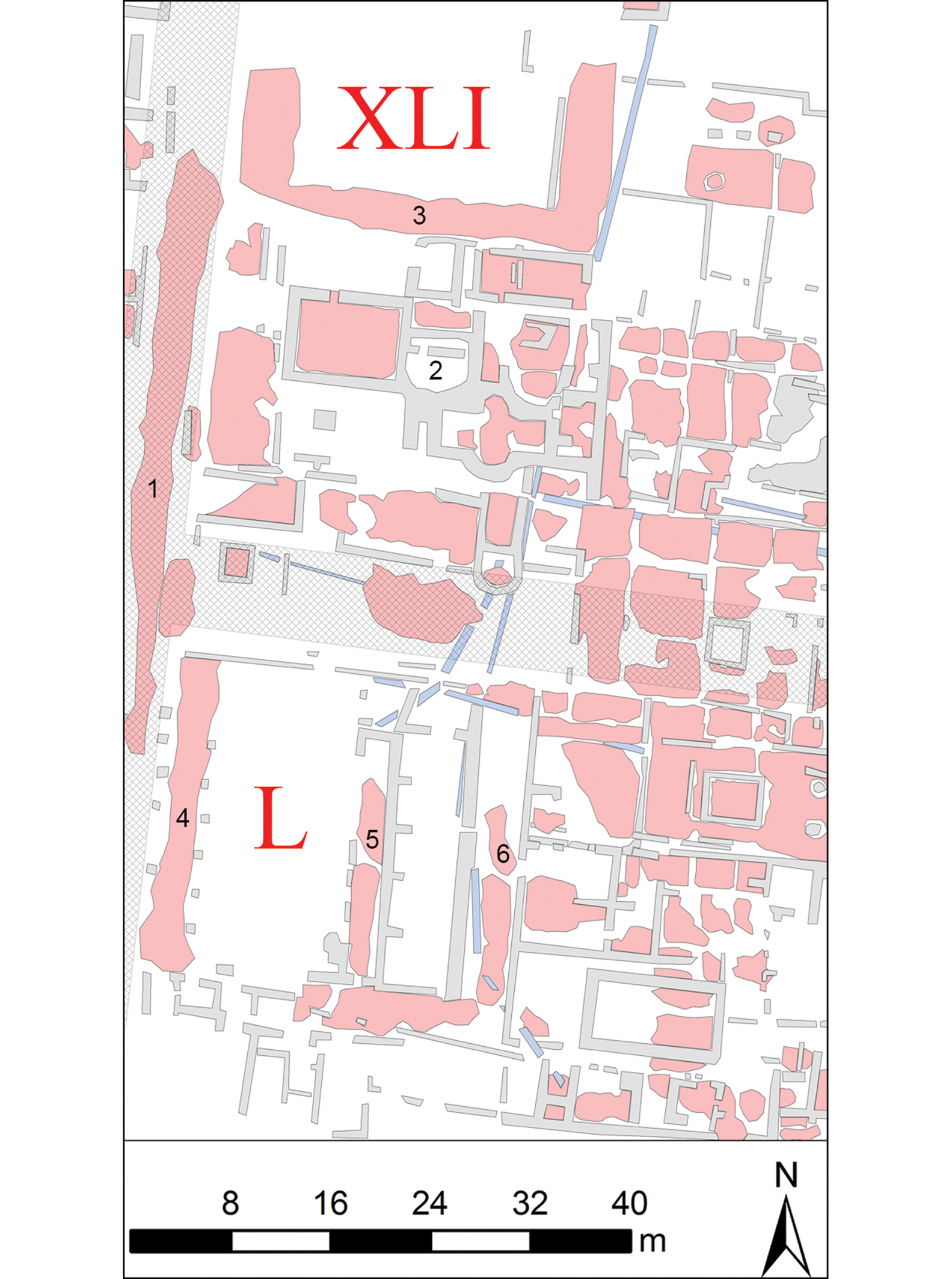
Figure 6. Interpretation of the case-study area (Figure 5) based on manual mapping of the anomalies. This summarises information from all the time-slices (illustrated in the online supplementary material), showing walls (grey), surfaces (red) and water pipes (light blue) (streets and Insula numbers from Keay et al. Reference Keay, Millett, Poppy, Robinson, Taylor and Terrenato2000) (image by A. Launaro).
As a detailed case study, we present an area in the south-west part of Falerii Novi (Figure 5). Figure 6 summarises the manual mapping of all the time-slices (illustrated individually in the OSM). This covers parts of Insulae XLI and L, bounded to the west by a north–south street that is visible in the GPR data (Figure 6.1), although it is less well defined than some other streets (e.g. Figure 4.8), suggesting the removal or loss of the paved surface. The east–west-running street separating the insulae cannot be discerned, with evidence suggesting that it may have been overbuilt.
Two or three atrium houses occupy the east of the area, all of which open onto the street that lies approximately 10m to the east. The plans of these houses are revealed by their floor surfaces, with many of the walls robbed-out. Examination of individual time-slices reveals structural remodelling over time. To the west, the area is occupied by larger structures, including a bath house in Insula XLI (Figure 6.2), with its main east–west block including a plunge bath and a series of apsidal rooms with hypocausts; another apsidal room to the south may be a later addition. A U-shaped area of flooring to the north of the bath house (Figure 6.3) may represent a portico surrounding a palaestra (exercise area). While these baths are modest in scale compared with those to the south-east (Figure 4.5), their architecture is nonetheless elaborate, perhaps suggesting that they were for public use.
To the south, in Insula L, is a very large rectangular building defined along the street to the west by a passageway with columns on either side (Figure 6.4). A second passageway along its centre is articulated with buttresses along its east face that were probably both decorative and structural (Figure 6.5), while a third passageway along the building's east side (Figure 6.6) shows no evidence for decorative elaboration. The building lies just within the town wall, towards the foot of a slope, and is connected to a series of water pipes that run beneath other buildings (Figure 6: light blue). These pipes connect with the town's aqueduct and can be traced across much of the town, running beneath the insulae, and not just along streets, as might have been expected. The architectural form and the presence of the pipes indicates that the large rectangular structure was not a cistern, but rather, an open-air natatio or pool associated with the adjacent baths, thus forming part of a substantial public bathing facility.
Problems and prospects
The results of the Falerii Novi survey show how high-quality GPR data can be collected across large areas, potentially revolutionising the study of ancient cities. The recent advances in the technology for collecting and processing the data, however, generate a series of new problems. First, is the sheer quantity of data resulting from survey at a dense sample interval. At Falerii Novi, we collected 71.7 million readings, each consisting of 400 temporal samples, equating to 28.68 billion data points—approximately 4.5GB of raw data per hectare (in the SEG-Y 32-bit single-precision floating point format). Such a dataset requires a considerable amount of time to analyse. More than 20 hours per hectare were required to produce the manual archaeological interpretations. This challenge prompted us to investigate methods of computer-aided interpretation.
The second issue relates to the combination of results from complementary geophysical prospection techniques that reveal different features, leading to the exploration of how such data can be combined for interpretation, rather than simply being juxtaposed. Finally, the question arises of how best to publish such large quantities of complex GPR data.
Image processing and fusion
The quantity and quality of the geophysical data from Falerii Novi make it ideal for assessing the utility of processing techniques for urban research. Recent work suggests that in addition to a multi-method approach (i.e. combining magnetometry, resistivity, electromagnetic induction and GPR), ‘data fusion’ enables the geophysicist to define better the position, extent, depth and physical characteristics of anomalies (Piro et al. Reference Piro, Mauriello and Cammarano2000; Kvamme Reference Kvamme2006; Bornik et al. Reference Bornik, Wallner, Hinterleitner, Verhoeven, Neubauer, Sablatnig and Wimmer2018). Various approaches to data fusion have been investigated, such as graphical integration, and operations on binary and continuous data (Kvamme Reference Kvamme2006). Using graphical overlays and colour composite images is an easy and efficient method for integrating geophysical data in a 2D environment. Two or more images are merged to generate a single composite image that contains information from all input images. Figure 7 shows a red-green-blue colour composite, where the magnetometer data (Figure 7a) are assigned to the green and blue channels, and the GPR data (Figure 7b) to the red channel. To perform the integration, both datasets were converted to a scale ranging from 0–255, and then resampled to a common sample density of 0.1 × 0.1m. The magnetometer data were also inverted so that walls are represented by high values, as is the case for the GPR data. Despite the difference in spatial resolution between the magnetometer and GPR data, the image combination (Figure 7c) allows the integration of the GPR information about a cistern (Figure 7d) with the more complete image of the house from the magnetometer data.

Figure 7. a) Extract from the fluxgate gradiometer survey, showing a house; data range −30nT (white) to +30nT (black) (Keay et al. Reference Keay, Millett, Poppy, Robinson, Taylor and Terrenato2000); b) extract from the GPR survey over the same area; c) RGB-image generated by assigning the GPR data to the red channel, and the gradiometer data to the green and blue channels. While the gradiometer survey provided a more complete image, the GPR data add high-resolution information on the peristilium, including a probable cistern, whose vault is clearly visible in the vertical GPR profile (d), the location of which is indicated by the red line in (b) (image by L. Verdonck).
Ideally, the output image should be more comprehensive than the input images and thus better suited for interpretation and further processing, while the fusion algorithm should be efficient and reliable. Image pixels are the final output of the geophysical data acquisition and processing chain. Pixel-based methods (Kvamme Reference Kvamme2006; Ogden et al. Reference Ogden, Strutt, Keay, Earl, Kay, Frischer, Crawford and Koll2010; Verdonck et al. Reference Verdonck, de Smedt, Verhegge, Persico, Piro and Linford2019) have produced significant results, resulting in a clearer depiction of structures that would otherwise be less visible in individual datasets. Nevertheless, several critical issues must be considered with such imagery. As the process seeks a targeted and combined—and to a certain extent simplified—depiction of the archaeological remains, the fused output may come at the price of some information loss (Filzwieser et al. Reference Filzwieser2018).
GIS tools are also frequently used to integrate and visualise GPR datasets. They are well suited for detailed interpretative mapping, as they support globally referenced positioning and flexible integration of field data in the form of overlays. The use of GPR data in GIS, however, remains limited to the display and overlay of horizontal 2D time-slices. Although this provides an accessible interface for visualising and interpreting the results, it does not take full advantage of the potential of 3D data. Future work using both GIS and other techniques is therefore required to explore true 3D visualisation for data analysis and interpretation. Although tests have so far been less than successful (Ogden et al. Reference Ogden, Strutt, Keay, Earl, Kay, Frischer, Crawford and Koll2010: 14), there must be future opportunity for the adaptation and application of interactive segmentation techniques. Image fusion, for instance, is frequently used in the medical sciences to improve image quality while simultaneously decreasing the amount of data and reducing information redundancy (James & Dasarathy Reference James and Dasarathy2014). Such an approach, critically deployed, would be of enormous value for archaeologists mapping ancient urban sites.
Computer-aided object detection
An important issue in processing GPR data relates to the creation of interpretative drawings. Today, this usually involves the manual definition of anomalies. This is time-consuming, raising the question of how it might be automated. When analysing GPR data from Roman urban sites, one method is to exploit the linear and orthogonal character of many anomalies, which usually represent walls or wall foundations.
This can be achieved by matching linear templates to an image (Verdonck Reference Verdonck2016): a predefined model, representative of the (linear) shape and the dimensions of the targeted wall structures, is slid across a GPR time-slice. Where the template matches the GPR image, the presence of walls can be expected. In mathematical morphology, a similar concept (the structuring element, SE) is used to probe the image, and perform image-processing operations. The size and shape of the SE makes the operation sensitive to particular objects in the image (by ‘objects’ we mean structures such as walls or floors). In mathematical morphology, two fundamental operations are erosion and dilation, which shrink and expand the objects in an image, respectively. In binary images, erosion can be used to eliminate objects smaller than the size of the SE. The larger objects are shrunk, and restored to their original size by dilating the image with the same SE. Therefore, the morphological opening (MO) operator (erosion followed by dilation) can be used to extract objects larger than the SE (Gonzalez & Woods Reference Gonzalez and Woods2002). In this way, irrelevant small objects (‘noise’) are eliminated, while the larger objects of archaeological interest, which conform to the SE, are preserved, so that the image is effectively cleaned.
A problem arises, however, when applying the MO to GPR time-slices. Using a linear SE, wall structures that are interrupted—by a doorway, for example—are not extracted, as they do not contain the complete SE. A shorter SE may detect the individual wall sections on either side of the doorway, but this also increases the risk of extracting small objects not belonging to walls (i.e. false positives). A more flexible operator is the ‘rank-max opening’ (RMO), where not only the size of the SE is defined, but also the rank—the maximum number of pixels allowed to be lacking from an image object (Soille Reference Soille2002). For linear SEs, the orientation also needs to be determined. Finally, when working with greyscale images, such as GPR time-slices, a threshold is used to obtain a binary image after applying the RMO.
We used the RMO to extract wall objects from the Falerii Novi case-study area (Figure 5). Thirty-two time-slices with a thickness of 0.05m and encompassing a total depth of 1.60m were analysed. Three parameters of the linear SE were specified. Its length was set to 4m, and its rank to 25 per cent (i.e. allowing gaps of up to 1m). Two perpendicular orientations, corresponding to the main orientations of the walls in Figure 5, were set to N6°E and N84°W. When applying these linear SEs to the horizontal GPR-slices, curved features such as the ones near numbers 1–3 (Figure 8a) were not detected. Therefore, we also applied a vertical linear SE to the vertical radargrams, with a length of 0.9m and a rank of 25 per cent. Both results were combined to give the result shown in red in Figure 8a.

Figure 8. Computer-aided object detection in the GPR data from the case-study area (Figure 5): a) the wall objects detected in each individual GPR slice and profile were combined and projected onto a 2D map (red). Detected floors are shown in green; b) 3D representation showing the same result, with the floors semi-transparent (image by L. Verdonck).
In the GPR data (Figure 5), however, it is not only the linear anomalies that contain archaeological information, but also the wider reflective areas that mostly represent floors. These were extracted by applying a median filter with a large window (up to 1 × 1m) to the time-slices. The output was thresholded to obtain a binary image. Of the resulting regions, those smaller than a predefined area were removed. The result is shown in green in Figure 8a.
This workflow was followed for each horizontal time-slice and each vertical profile. The resulting images were stacked to obtain two binary volumes, one based on the time-slices, the other on the profiles. Of these two volumes, the Boolean union was calculated, and the outcome was imported in the rendering software Autodesk 3ds Max, to create the 3D representation shown in Figure 8b. When employing this workflow, most positive GPR anomalies (i.e. reflections, indicated by the white areas in Figure 5) were detected successfully, although some anomalies interpreted as walls during manual mapping (Figure 6) were considered as floors by the computer algorithm. Of the negative GPR anomalies present in Figure 5 (weak reflections contrasting with a strongly reflecting background), some were extracted (Figure 8a, 4–5), while others remained undetected. In a different area of the site, where a bath house is located (Figure 4.5), two factors complicated the extraction of wall objects. First, the walls are on a variety of different orientations. Two regions were therefore delimited (Figure 9c, 1–2), where the extraction occurred separately using linear SEs with different orientations. Second, at deeper levels, the walls appeared barely separable from the strongly reflective areas inside the rooms (Figure 9b). This resulted in false positives when extracting wall structures from the time-slices (e.g. Figure 9c, 3–4). Further separate regions (the dashed lines in Figure 9c) were required to detect the curved walls indicated by the arrows in Figure 9a–b. Object detection was further complicated by the fact that, for the area shown in Figure 9, it was not possible to use uniform values for the length of the SEs and the thresholds (these values were determined for each time-slice separately). The process, therefore, is one of interactive rather than automatic detection: the algorithm proposes a segmentation image based on the parameter values entered by the user, who then improves the segmentation by iteratively changing the parameter values. While time-saving may currently be limited for complex datasets, growing computing power seems certain to make such interactive approaches increasingly beneficial, for example, enabling the interpretation map to change instantaneously when the user moves a slider to adjust a parameter value.
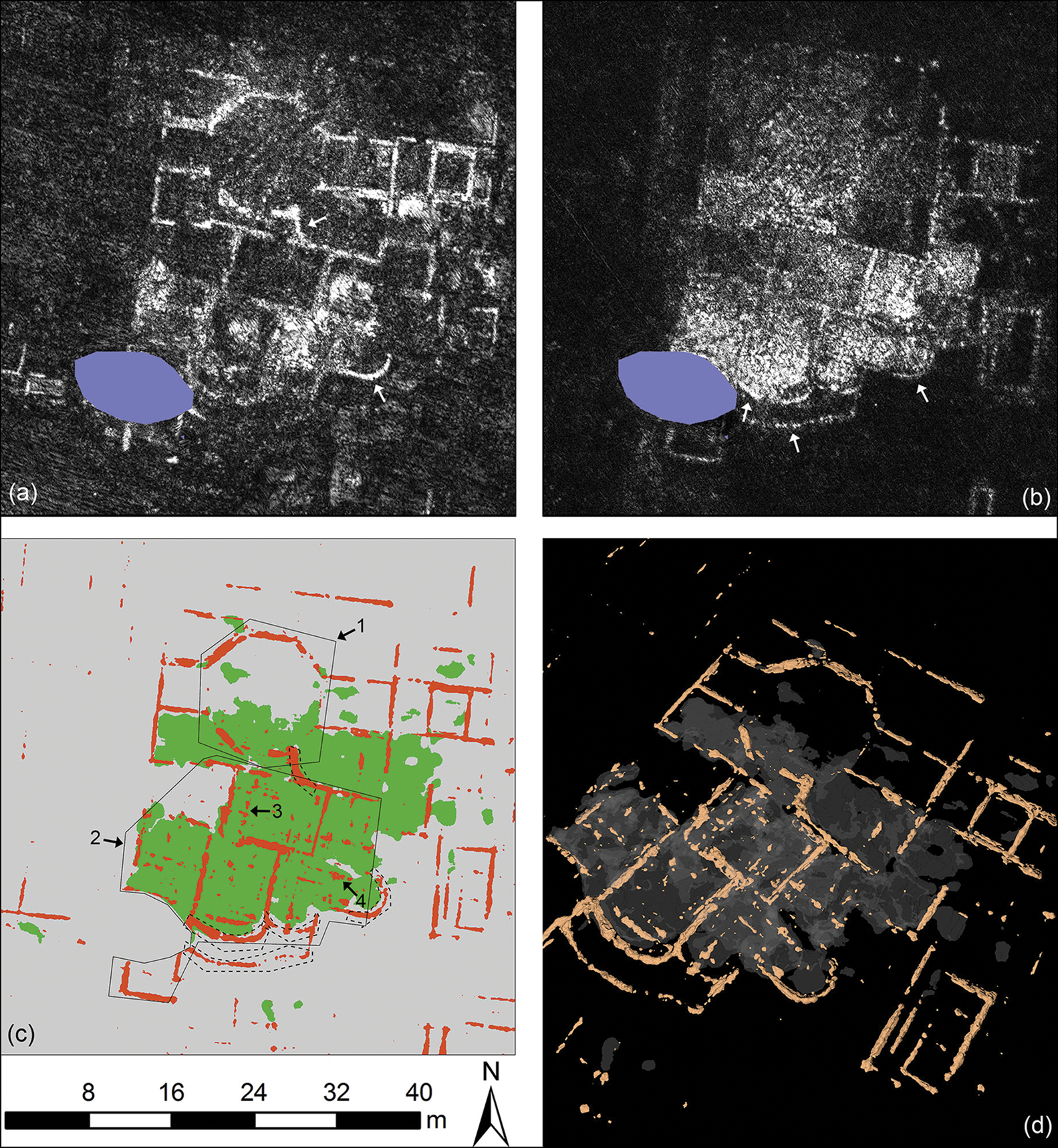
Figure 9. GPR time-slices from a bath complex, at an estimated depth of (a) 0.40–0.45m and (b) 1.30–1.35m; c) result of the computer-aided detection of walls (red) and floors (green). The solid and dashed lines indicate areas for which parameter values were set separately; d) 3D representation showing the same result (image by L. Verdonck).
Publication and archiving
A final issue concerns how best to publish such substantial datasets. Too often, major geophysical surveys are simply presented as images, usually at small-scale and without much discussion of the structures revealed. Our aim is to publish a comprehensive analysis of the architecture and history of an entire Roman town. While this will take some time to realise, we envisage that it will take the hybrid form of a print summary and an online atlas, enabling the reader to interrogate the images. In the meantime, we include OSM along with this article to enable readers to explore the evidence. Simultaneously, our data have been deposited, in accordance with the guidelines for archiving geophysical data (Schmidt & Ernenwein Reference Schmidt and Ernenwein2011), as an open-access archive with the Archaeology Data Service (Millett et al. Reference Millett, Verdonck, Leone and Launaro2019), so that they are available for other researchers to access and use. The raw and processed GPR profiles, including position information, have been converted from proprietary formats to the seismic SEG-Y rev 1 format, which can be accessed using freely available SEG-Y readers (e.g. SeiSee). The time-slices are stored as GeoTIFF files, accompanied by full metadata, including project information, field methodology, instrument settings and data treatment.
Conclusions
Together with our similar GPR survey at Interamna Lirenas, the work at Falerii Novi has significant implications for the study of Roman cities. Building on the earlier use of magnetometer surveys, it offers the possibility of investigating a series of towns as total entities, thereby moving away from a reliance on a small number of extensively explored sites and a large number of limited area excavations that provide only small samples of much larger sites. It is important to note, however, that while Falerii Novi is well suited to both magnetometry and GPR survey, other sites may not be so conducive. GPR, for example, may perform less well on conductive soils. It is therefore crucial to choose the (geophysical) prospection techniques most appropriate to the soil, geology and archaeological preservation at each site. Furthermore, although GPR survey provides both high-resolution imagery and the ability to distinguish structures at different depths, it can never provide the chronological detail or the granular evidence of a site's development accessible through excavation. Conversely, the same extent of high-resolution spatial coverage can never be achieved by excavation. The two approaches are thus complementary, and we must rethink how the two methods might better complement each other, abandoning the positivist concept of ‘ground-truthing’ to reconsider what information we require to understand entire towns, and how it could most appropriately be collected. Extrapolating from a single example (our work at Falerii Novi) is clearly hazardous, but both the magnetic and GPR surveys at Falerii Novi suggest that the greatest potential from the combination of different methods is to be found in the peripheral areas of the town. Before these surveys, we had only information from nineteenth- and twentieth-century excavations, along with an epigraphic and sculptural analysis (di Stefano Manzella Reference di Stefano Manzella1979). These limited data, together with inferences from the site's topography, comparison with other towns, and general understandings of concepts of Roman urban planning, formed the basis for speculation about Falerii Novi's layout. Our new survey results now show how Falerii conforms to such concepts (e.g. the forum's design and location), but also how it elaborates other ideas. The magnetometer survey, for instance, had revealed a sacred topography, with temples located around the town's periphery. The GPR work has greatly enhanced our understanding of this landscape, showing how other monumental buildings articulated this marginal space—particularly adjacent to the town's gates (e.g. the porticus in Figure 4.6 and the temenos in Figure 4.7). Although we are yet to understand how this sacred landscape functioned, the survey provides new insights into the variety of planning concepts underlying what are sometimes incorrectly considered to be ‘standardised’ Roman town plans. By providing a contrast with more familiar towns such as Pompeii, this work also raises important questions about the planning of Roman towns more generally.
The detailed investigation of complex sites, such as Roman cities, produces increasingly large amounts of data. Interpretation through traditional, manual methods is unfeasible for sites tens of hectares in extent. While new techniques, such as computer-aided object detection, will be indispensable, their application to archaeological geophysics is currently still in its infancy. In this article, the potential and limitations of computer-aided methods are illustrated by examining one class of algorithms, based on mathematical morphology. Further we have shown how the fusion of multiple geophysical datasets can aid in archaeological interpretation. There is little doubt that the future application of new analytical methods such as these will fundamentally change the ways in which Roman urbanisation is understood.
Acknowledgements
This research was a collaboration between the universities of Cambridge and Ghent, funded by the Arts and Humanities Research Council (AH/M006522/1). Lieven Verdonck was funded by a post-doctoral fellowship from the Fund for Scientific Research—Flanders (FWO). We are grateful for support from Soprintendenza Archeologia, Belle Arti e Paesaggio per l'Area Metropolitana di Roma, la Provincia di Viterbo e l'Etruria Meridionale.
Supplementary material
To view supplementary material for this article, please visit https://doi.org/10.15184/aqy.2020.82