Implications
Bulls with a low milk urea nitrogen concentration (MUN) breeding value (BV) would sire daughters with reduced MUN. This is expected to reduce urinary nitrogen (UN) deposition on pasture and nitrogen (N) leaching risk and reduce the amount of ammonia and nitrous oxide volatised from urine. Modelling suggests that the genetic resource available in New Zealand (NZ) could see N leaching reduced by 20% over 20 years. Low MUN cattle have higher percent true protein in their milk. Milk urea nitrogen concentration–UN relationships should be investigated in animals genetically diverse for MUN.
Introduction
Agricultural impact on the environment is attracting increased public attention internationally (Gustin, Reference Gustin2017). Deteriorating water quality in streams, rivers, lakes and groundwater poses one of NZ’s greatest environmental challenges (Gluckman, Reference Gluckman2017). Pastoral farming is a key contributor to this deterioration with N leached through the soil profile into groundwater being an element of primary interest and concern. The main source of N leached is UN, with leaching being the result of high N loading within the urine patch: Haynes and Williams (Reference Haynes and Williams1993) reported mean loading rate for dairy cows of 1000 kg N/ha while in a comprehensive review of studies addressing N cycling, Selbie et al. (Reference Selbie, Buckthought and Shepherd2015) reported loading rates from dairy cows ranged from 200 to 2000 kg N/ha with mean 613 kg N/ha. The fate of N from the urine patch where N is at such high concentration then becomes critical. From a review of published literature, Selbie et al. (Reference Selbie, Buckthought and Shepherd2015) concluded that N which reaches the ground as UN is partitioned approximately as follows: 13% to ammonia volatilisation, 2% to nitrous oxide, 20% to leaching, 26% to gross immobilisation and 41% to pasture uptake.
Dairy cattle utilise dietary N for maintenance functions, milk production and tissue growth, with surplus N being excreted via urine and faeces (Spek, Reference Spek2013). In a review of studies of forage-grazing dairy cattle, Luo and Kelliher (Reference Luo and Kelliher2010) estimated that mean daily N intake is 459 g N with 49% of this being excreted as UN. It follows that if 20% of N excreted as UN is leached (Selbie et al., Reference Selbie, Buckthought and Shepherd2015) then N leaching on a per cow basis is around 45 g/day or 16 kg/year. Clearly it is desirable to reduce N excreted as UN in order that N leaching may be reduced. Although measurement of UN output is difficult and expensive, MUN is easily measured and is linearly related to daily excretion of UN (Table 1). Urinary nitrogen arises from ammonia produced in the rumen and post-rumen being converted in the liver to urea (Spek, Reference Spek2013). This diffuses via blood (blood plasma urea (PU)) to other fluid pools in the body including milk (milk urea, MU) and, in the kidney, blood PU passively diffuses into urine and is excreted as urinary urea (Roseler et al., Reference Roseler, Ferguson, Sniffen and Herrema1993). Ciszuk and Gebregziabher (Reference Ciszuk and Gebregziabher1994) reported that the daily amount of urea excreted in urine is proportional to the concentration of urea in blood, which in turn is proportional to MU concentration. The N content of MU is 46% (MUN=MU/2.14) and MUN (mg/dl) predicts the amount of UN (g/day) excreted.
Table 1 Reported regressions between urinary nitrogen output (UN, g/cow per day) of dairy cows and milk urea nitrogen concentration (MUN, mg/dl)
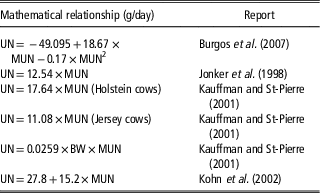
When cows are fed differing diets indoors, MUN is positively associated with UN (Table 1). Typically, the relationship is in the order of 13 to 16 g UN/day per mg MUN/dl. Average MUN is typically 12 to 16 mg/dl. Although there is considerable between-cow variation (Spek, Reference Spek2013), the data in Table 1 indicate that N excretion from a herd with mean MUN of 14 mg/dl would be about 210 g UN/cow per day, whereas a herd with a mean MUN of 10 mg/dl would excrete about 150 g UN/cow per day. For cows grazing pasture measurement of UN loading is difficult: in a NZ study, Hendricks (Reference Hendriks2016) reported that UN increases linearly with MUN at a rate of 17 g UN/day per mg MUN/dl but recommended that further work is required to determine a robust prediction equation for the MUN–UN relationship in grazing cows.
Several studies with stall-fed cows have investigated the heritability of MUN: values range from 0.13 (Bastin et al., Reference Bastin, Laloux, Gillon, Miglior, Soyeurt, Hammami, Bertozzi and Gengler2009) to 0.59 (Wood et al., Reference Wood, Boettcher, Jamrozik, Jansen and Kelton2003). Stoop et al. (Reference Stoop, Bovenhuis and van Arendonk2007), König et al. (Reference König, Chang, von Borstel, Gianola and Simianer2008), Mitchell et al. (Reference Mitchell, Rogers, Dechow, Vallimont, Cooper, Sander-Nielsen and Clay2005) and Mucha and Strandberg (Reference Mucha and Strandberg2011) all reported values between 0.14 and 0.23, whereas Migilor et al. (Reference Miglior, Sewalem, Jamrozik, Bohmanova, Lefebvre and Moore2007) reported a value of 0.38. It is therefore theoretically possible to breed cattle for lower MUN (Stoop et al., Reference Stoop, Bovenhuis and van Arendonk2007). However, for animals that are genetically lower in MUN to also exhibit lower UN excretion, they need to partition dietary N away from urine to other N pools compared to the average, unselected, animal.
Several studies have explored the potential for using MUN to predict the efficiency with which dietary N is used for milk production, but none specifically addressed whether selection for low MUN results in progeny which excrete less UN. Sebek et al. (Reference Sebek, van Riel and de Jong2007) concluded that breeding cows with low MU content did not improve the efficiency of dietary protein utilisation; nevertheless, breeding for low MU was not expected to increase costs or have consequences for milk production and composition. Vallimont et al. (Reference Vallimont, Dechow, Daubert, Dekleva, Blum, Barlieb, Liu, Varga, Heinrichs and Baumrucker2011) found that relationships between MUN and dry matter intake efficiency, net energy for lactation efficiency and CP efficiency were statistically non-significant, although genetic correlations trended negative and therefore were favourable in sign. In a meta-analysis of eight publications, Cantalapiedra-Hijar et al. (Reference Cantalapiedra-Hijar, Dewhurst, Cheng, Cabrita, Fonseca, Fouillet, Noziere and Ortigues-Marty2016) reported a significant asymptotic (negative) relationship between N use efficiency and MUN in groups of NZ Jersey (J) and Holstein-Friesian (HF) cows. Stoop et al. (Reference Stoop, Bovenhuis and van Arendonk2007) found a positive genetic relationship between MUN and per cent protein (%protein) in milk (r=0.27±0.19), and similarly Miglior et al. (Reference Miglior, Sewalem, Jamrozik, Bohmanova, Lefebvre and Moore2007) reported r=0.20.
Overall, research to date suggests MUN may be reduced through selective breeding, but it has not been demonstrated conclusively that cows with low MUN utilise dietary protein more efficiently. The opportunity to utilise the relationship between MUN and UN to decrease UN deposition through breeding has not been fully explored, possibly due to concerns that decreasing MUN may lead to reductions in milk protein yield and percentage (Miglior et al., Reference Miglior, Sewalem, Jamrozik, Bohmanova, Lefebvre and Moore2007; Stoop et al., Reference Stoop, Bovenhuis and van Arendonk2007), which might outweigh the economic benefit of decreasing UN.
However, in NZ the issue of agriculture’s influence on water quality is pressing, and cost-effective options for managing the UN load on grazed pasture are urgently required. In NZ’s pasture-based dairy systems, cattle are consuming pastures that typically have a high-protein content (DairyNZ, Reference DairyNZ2017), resulting in higher MUN compared with cows fed total mixed rations (Garcia-Muniz et al., Reference Garcia-Muniz, Lopez-Villalobos, Burke, Sandbrok and Vazquez-Pelaez2013). Based on the MUN–UN relationships described by Spek (Reference Spek2013), they can therefore be expected to excrete a high UN load. Therefore, this study investigated the phenotypic and genetic variation of MUN across the two main breeds of dairy cattle in NZ and their crosses and explored genetic relationships between MUN and other milk components. The implications of our findings are discussed with respect to prospects for breeding dairy cattle that excrete less UN, and the potential benefits of this for reducing N leaching.
Material and methods
The concentration of urea in milk was measured in milk samples from individual cows in c. 540 herds representing a cross-section of farms located throughout NZ. As part of CRV Ambreed’s herd testing service, the samples were collected at afternoon and the following morning milkings during four lactations between 2013–14 and 2016–17. Lactations generally span from July 1 year to May the next year. The 2016–17 data spanned the first 6 lactation months with the final collection date 8 December 2016. In NZ, most dairy farms are seasonal, whereby cows calve June through November (late winter-spring in Southern Hemisphere) and milk through to autumn with an average lactation length of about 270 days. Average herd size is 419 cows (New Zealand Dairy Statistics, 2015–2016). During each herd test, afternoon and morning milk was proportionally aggregated, 24-h milk volume recorded and the sample analysed for percentage of protein (%protein), percentage of fat (%fat), somatic cell content, percentage of lactose (%lactose) and percentage of MU at MilkTestNZ (Hamilton, NZ), using a FOSS MilkOscan FT+ analyser (FOSS, Hilleroed, Denmark). Protein percentage was calculated as % N ×6.38. Typically, herds were tested four times per lactation. The number of samplings measured per cow varied according to her age/number of lactations since 2013–14, and the frequency with which the herd was tested. In addition to herd test milk data, information on individual cow pedigree (compiled from parentage records submitted at an animal’s birth by the cow owner), calving date at commencement of each lactation, herd test date, cow breed and herd identification was also collated.
A repeatability animal model across test months and years and a random regression test-day model were employed to cross-verify outcomes particularly estimates of heritability (h 2) and MUN BVs. Datasets were prepared to meet each model’s requirements.
Data: repeatability animal model
All cows included in the dataset calved between 1 June and 1 November during a particular season. The contemporary group (CGp) was defined as combination of herd, season, month of herd test, age at calving where herd is the unique herd identifier; season is the calendar year in which lactation commenced; month of herd test is the calendar month of each herd test; and age at calving is age in years, grouped as 2, 3, 4, 5 to 7 and 8+. Contemporary groups of fewer than five cows or where all cows were sired by one bull, were excluded from the study. Although there was no significant effect of cow age class on MUN, it did affect other milk production traits so was included in the CGp definition for all traits analysed.
Percentage of true protein (%TPr) excluding the N contribution from MUN was calculated as:

and true protein yield was calculated by multiplying 24-h milk volume by %TPr.
Cows were predominantly HF, J or HF×J (XBd) in breed composition and were classified according to breed proportions in increments of 1/16. For cows missing pedigree at grandparent or great-grandparent generations, the proportion of known breed composition was scaled up so that total breed composition summed to 16 parts. Cows that were 16 parts HF or J were coded as purebred for within-breed analyses. For an across-breed analysis, proportions of both HF and J were fitted as covariates along with a term for heterosis. Three generations of pedigree data were used for univariate runs, and due to very long processing times, two generations of pedigree were used for bi-variate analyses. The edited data consisted of 410 510 records from 133 624 cows. New cows were added to the study each year as they commenced their first lactation at 2 years of age. Number of herd tests per cow varied from 1 to 9 across the four lactations.
Data: random regression test-day model
A multi-trait random regression test-day model was also used to analyse the data. Test-day records with MUN observations were selected using the following minimum criteria: 3 test days per cow per lactation, five cows per herd test day and five offspring per bull. This resulted in a dataset containing 152 931 test days of 37 000 cows, with a pedigree encompassing 75 500 animals.
Statistical analyses: repeatability animal model
Univariate analyses of all traits were used to estimate genetic parameters and starting values for pairwise analyses of MUN with each milk component and yield trait. These analyses were run using a repeated measures animal model in ASReml (Gilmour et al., Reference Gilmour, Gogel, Cullis and Thompson2009). The univariate model (across breeds) was:

where Y is the test-day record for MUN or the milk production traits, and HSMA the CGp definition for the combination of herd, season, month of herd-test and age at the start of lactation (grouped as 2, 3, 4, 5 to 7 and 8+). DIM is the days in milk deviated from the CGp mean for days in milk, fitted as a linear and quadratic covariate within month of herd-test. HFpr is the proportion of Holstein-Friesian; Jpr the proportion of Jersey; Het the heterosis coefficient between HF and J; ‘a’ a random animal effect; pe_within the permanent environmental effect within season; pe_across the permanent environmental effect across seasons; and e the random residual term for Y.
Heritabilities, repeatabilities, and genetic and phenotypic correlations were estimated across breed and separately within subsets of data for HF16, J16 and XBd cows. For the XBd cows, HFpr, Jpr and Het were included in the model.
The four yield traits (milk, true protein yield, fat yield and lactose yield) were scaled around month by cow age class means to reduce the heterogeneity of the CGp mean-variance ratios using:

Statistical analyses: random regression test-day model
Random regression curves were fitted using a second-order Legendre polynomial function over a period from day 5 to day 270 of lactation. The model was constructed following that of De Roos et al. (Reference De Roos, Pool, Caccamo, Azzaro, Ferguson and Licitra2005):

where y ijklmnorsupdt is the test-day record for trait t (milk, fat or protein production, or somatic cell score) of cow s on DIM d of parity p; pd it the parity × DIM class i for trait t. Parity was classified as 1, 2, 3 to 4 and ⩾5. DIM was classified in 5-day classes from DIM 5 to 270, plus a class for DIM 271 to 280 and DIM 281 to 300; psd jt the parity × year-season of calving × DIM class j for trait t. Season of calving was classified as June, July, August, September and October to November and DIM for this term was classified as DIM 5 to 50, 51 to 100, 101 to 150, 151 to 200 and 201 to 300. Pa kt is the parity × age at calving class k for trait t. Age at calving was expressed in months. Yw lt is the year × week of test class l for trait t. Het mt is the heterosis class m for trait t. Heterosis was classified as 0% to 24%, 25% to 49%, 50% to 74%, 75% to 99% and 100%, that is five classes. Rec nt is the recombination class n for trait t. Recombination was classified as 0% to 12.4%, 12.5% to 24.9%, 25% to 37.4%, 37.5% to 49% and 50%. Htd ot is herd × test date o for trait t. z dq is the order q Legendre polynomial for DIM d (Kirkpatrick et al., Reference Kirkpatrick, Lofsvold and Bulmer1990). Hc rpqt is the herd curve effect of herd × calving year r corresponding to polynomial q of parity p r and trait t, where p r =min{p,3}. Note that each herd gets a regression curve for each trait, for parity 1, 2 and ⩾3 (De Roos et al., Reference De Roos, Harbers and De Jong2002). Ag spqt is the additive genetic effect of animal s corresponding to polynomial q of parity p r and trait t. Pe spqt is the permanent environmental effect of animal corresponding to polynomial q of parity p r and trait t. ls uqt is the lactation-specific permanent environmental effect of lactation u corresponding to polynomial q of trait t. Only test-day records from lactations with parity ⩾3 were assigned to a lactation-specific permanent environmental effect. In this manner, lactations with parity ⩾3 had one common permanent environmental curve and one specific curve for each lactation (Lidauer et al., Reference Lidauer, Mantysaari, Stranden and Poso2000). Finally, e ijklmnorsupdt is the residual of the observation of y ijklmnorsupdt .
Variance component matrices were estimated for herd curve, permanent environment (pe), lactation specific pe and animal effects for six traits (milk yield, fat yield, protein yield, lactose yield, MUN and somatic cell count) in three lactation classes and three curve parameters per trait, except for the lactation-specific pe, which was only fitted for lactation class 3+. Residual error (co-) variance components were fitted for nine lactation stages in five parity classes. Parameters were estimated using the Gibbs sampler described in De Roos et al. (Reference De Roos, Harbers and De Jong2004). Burn-in chains of 10 000 iterations provided starting values for 12 chains each of 25 000 iterations. Effective chain lengths were computed from transition probabilities using Gibanal 2.9 (Van Kaam, Reference Van Kaam1998). Estimates of the variance components were calculated as posterior means of the stationary phase of the Gibbs chains. Variance components of herd curve, pe, lactation-specific pe and animal effects for 270-day lactations were obtained by:

where L is the covariance matrix of curve-parameters, I 18 a size 18 identity matrix and v a three-element vector with either the sums of Legendre-coefficients (cumulative production) or the mean of Legendre-coefficients (mean production) per day in milk from days 5 to 270. Residual error covariances were calculated per day from days 5 to 270 and summed. Breeding values of 270-day production within lactation were computed as:

where BV i is the predicted BV at the ith day of lactation. Breeding values for component traits (%fat, %protein and %lactose) were derived from the production trait BVs.
Correlations between sire milk urea nitrogen concentration breeding value and breeding values for non-milk traits
Insufficient data were available from animals in this study to allow accurate estimation of genetic correlations between MUN and several traits important to NZ dairy farmers. Instead, correlations were made between sire MUN BV and BVs for each of those traits calculated and published by New Zealand Animal Evaluation (NZAE) Limited (2017). New Zealand Animal Evaluation utilises all dairy data submitted to the NZ Dairy Industry Good Animal Data Base from licensed herd testers to calculate BVs of dairy sires for a range of traits but not MUN.
Results and discussion
Milk urea nitrogen concentration phenotypes
Phenotypic variation in MUN was evident between and within the three breeds of dairy cattle. Overall mean MUN was 14.0 mg/dl (SD 4.3 mg/dl), while the subset of cows used in the test-day model analysis had a mean of 14.1 mg/dl. Differences between cow breeds (14.4, 13.2, 13.9 mg/dl for HF, J and XBd cows, respectively, Table 2) prompted replication of analyses within breeds. This analysis revealed that MUN was positively correlated with milk and true protein yield and negatively related to true protein, fat and lactose percentage. Milk urea nitrogen concentration was positively correlated with fat yield in HF cows but this relationship was negative for J cows and near zero for XBd cows. In all cases the absolute value of the correlations was less than r=0.20. Repeatability of MUN was slightly higher within lactations than across lactations and was similar across all cow breeds.
Table 2 ASReml-derived estimates of heritability (±SE) and repeatability for milk urea nitrogen concentration (MUN, mg/dl) across all cows and within breed of cow (Holstein-Friesian (HF), Jersey (J), HF×J (XBd)) and genetic (r g ) and phenotypic (r p ) correlations of MUN with milk yield scaled (Milk Y sc), true milk protein yield scaled (True Protein Y sc), milk fat yield scaled (Fat Y sc), % true protein, % fat and % lactose

Test-day model (TDM) parameter estimates for MUN, milk, protein and fat yields using unscaled data.
1 SE not available for TDM parameter estimates.
2 Not analyzed.
Milk urea nitrogen concentration values were within the range of other reports. Milk urea nitrogen concentration is influenced by the protein content of feeds as described by Kauffman and St-Pierre (Reference Kauffman and St-Pierre2001) who reported MUN at around 6.5 and 12.5 mg/dl for diets containing 13% and 17% CP, respectively. Higher MUN values of 20.4 mg/dl were measured by Stoop et al. (Reference Stoop, Bovenhuis and van Arendonk2007) with these authors stating that this higher value was likely caused by high-protein content of the diet. Our value of 14 mg/dl is lower than the 16 mg/dl reported by Garcia-Muniz et al. (Reference Garcia-Muniz, Lopez-Villalobos, Burke, Sandbrok and Vazquez-Pelaez2013) in pasture-fed cows in NZ where diets usually contain >17% CP (DairyNZ, Reference DairyNZ2017).
Genetic parameters
Heritability of MUN from the repeatability model was 0.22 (SE 0.01) across breeds and 0.24, 0.19 and 0.19 for HF, J and XBd cows, respectively (Table 2). Estimates from the test-day model analysis resulted in a heritability for mean daily MUN of 0.28. These findings support the premise that the inheritance of MUN in NZ is similar to that elsewhere and that MUN concentration could be modified by selective breeding. The heritability of MUN (0.19 to 0.24 repeatability model, 0.28 test-day model) found in this study is within the reported range 0.13 (Bastin et al., Reference Bastin, Laloux, Gillon, Miglior, Soyeurt, Hammami, Bertozzi and Gengler2009) to 0.59 (Wood et al., Reference Wood, Boettcher, Jamrozik, Jansen and Kelton2003).
Repeatability model-derived genetic correlations between the milk traits and MUN are shown in Table 2. The genetic correlation of MUN with %TPr is of major relevance and is −0.20 (SE 0.02) across breeds and −0.16, −0.29 and −0.21 for HF, J and XBd cows, respectively. Values for genetic correlations trended similarly to the phenotypic correlations: yield traits were positive in sign (except fat yield in J and XBd cows) and composition traits were negative. Absolute values of genetic correlations tended to be larger than for the phenotypic correlations.
Should MUN become an important trait in breeding programs, it is critical to determine whether or not this would conflict with current breeding objectives. Since 1996, NZ farmers have used bulls bred using a gross efficiency breeding index (Breeding Worth (BW), NZAE Limited, 2017), which estimates economic returns per five tonnes of feed consumed. Breeding Worth incorporates BVs for lactation milk, protein and fat yields, live weight, somatic cell score, residual survival, fertility and body condition score. Correlations were calculated for sire MUN BV and BVs for each of the traits in BW (Table 3). Overall, these correlations point to a small favourable relationship between MUN and BW. Milk urea nitrogen concentration was negatively correlated with traits associated with reproduction (fertility and body condition score) but positively related to milk yield. There were small positive correlations between MUN and fat and protein yields except for MUN–fat yield in Js. In general, it appears that selection for low MUN will not conflict with genetic gain in BW.
Table 3 Correlations between breeding value (BV) milk urea nitrogen concentration and NZAE Limited-derived BVs for component traits of Breeding Worth: within breed of sire (Holstein Friesian (HF), Jersey (J), crossbred (XBd))

The negative but favourable genetic correlation between MUN and %TPr found in this study may be evidence that genotypes which differ in MUN differentially partition dietary N from urine to other pools. The negative correlation is consistent across breeds, although the relationship was stronger in J (−0.29) than HF (−0.16) genotypes. Our finding contrasts with those of Stoop et al. (Reference Stoop, Bovenhuis and van Arendonk2007) and Miglior et al. (Reference Miglior, Sewalem, Jamrozik, Bohmanova, Lefebvre and Moore2007) which reported MUN to be positively correlated with %protein, while other studies have been unable to show statistically significant favourable associations between MUN and efficiency of protein utilisation (Sebek et al., Reference Sebek, van Riel and de Jong2007; Vallimont et al., Reference Vallimont, Dechow, Daubert, Dekleva, Blum, Barlieb, Liu, Varga, Heinrichs and Baumrucker2011). However, Cantalapiedra-Hijar et al. (Reference Cantalapiedra-Hijar, Dewhurst, Cheng, Cabrita, Fonseca, Fouillet, Noziere and Ortigues-Marty2016) reported a negative asymptotic relationship between MUN and protein-use efficiency in a meta-analysis. Most experiments investigating efficiency of utilisation of dietary protein have been conducted with relatively few animals which restricted the statistical power available for detecting differences of relatively small magnitude. The genetic studies of Stoop et al. (Reference Stoop, Bovenhuis and van Arendonk2007) and Miglior et al. (Reference Miglior, Sewalem, Jamrozik, Bohmanova, Lefebvre and Moore2007) differed from our study in two respects; the number of animals phenotyped was lower and the population in each case was relatively homogeneous (Dutch Holsteins in the case of Stoop et al., Reference Stoop, Bovenhuis and van Arendonk2007 and Canadian Holsteins for Miglior et al., Reference Miglior, Sewalem, Jamrozik, Bohmanova, Lefebvre and Moore2007). Furthermore, for many decades the NZ populations of HF and J cattle have been selected for milk solids rather than milk yield and could be expected to be genetically divergent from overseas populations. These factors may partly explain why a negative genetic correlation between MUN and %TPr was found in our study, whereas others have reported a positive correlation. The fact that J cows have lower MUN (13.2 mg/dl) but higher %TPr (4.14) than HF cows (14.4 mg/dl MUN, 3.61% TPr) adds further weight to our conclusion that the genetic relationships between MUN–%protein and MUN–%TPr are negative in sign for NZ dairy cattle.
Sire breeding values
Milk urea nitrogen concentration BVs were estimated from both repeatability and test-day models. The correlation of MUN BVs generated by the two approaches for sires with phenotyped daughters was 0.94. Milk urea nitrogen concentration BVs ranged from −2.8 to +3.2 (mean 0.15). Jersey sires had lower mean MUN BV than HF sires (−0.31 v. +0.48), with similar ranges for each breed of sire (−2.8 to +2.3 for J; −2.4 to +3.2 for HF); XBd sires averaged −0.06 with range −2.2 to +3.0.
Milk urea nitrogen concentration BVs for sires were negatively correlated with their %protein BV as published by NZAE Limited (2017). The correlations between MUN BV for sires calculated in this study and %protein BV as reported by NZAE Limited (2017) were −0.15, −0.37 and −0.14 for HF, J and XBd sires, respectively. The directly calculated correlations (Table 2), together with these correlations of sire BVs strongly suggest that in NZ cattle there is a negative genetic correlation between MUN and protein content of milk, with this relationship greater (more negative) in J than in HF cattle.
Estimating reductions in urinary nitrogen loading and nitrogen leaching risk through lower milk urea nitrogen concentration genotype
The direct positive relationship between MUN and UN reported by Burgos et al. (Reference Burgos, Fadel and De Peters2007), Jonker et al. (Reference Jonker, Kohn and Erdman1998), Kauffman and St-Pierre (2001) and Kohn et al. (Reference Kohn, Kalscheur and Russek-Cohen2002) was derived from studies feeding a range of diets resulting in different N intakes. Assuming that reducing MUN through breeding has a similar effect on UN to reducing MUN through feeding, the impact on UN of reducing MUN genetically can be estimated. A bull team with a mean MUN BV of −2.4 is expected to breed offspring with a MUN phenotype 1.2 mg/dl lower than the offspring of MUN BV=0 bulls. Current HF, J and ‘NZ average’ cows have mean phenotypes for MUN of 14.4, 13.2 and 14.0 mg MUN/dl, respectively (Table 2) and their progeny sired by bulls with MUN BV −2.4 are expected to have MUN phenotypes of 13.2, 12.0 and 12.8. Substituting these MUN values in the equations of Burgos et al. (Reference Burgos, Fadel and De Peters2007), Jonker et al. (Reference Jonker, Kohn and Erdman1998), Kauffman and St-Pierre (2001) and Kohn et al. (Reference Kohn, Kalscheur and Russek-Cohen2002) result in predicted UN values shown in Table 4. The best estimate is a reduction in UN of 18 g/day in the progeny of the BV MUN −2.4 bulls compared to the progeny of BV MUN 0 bulls. Compounded, this amounts to an annual reduction in UN of 6.6 kg/cow. There are 6.5 m dairy animals farmed in NZ: 6.6 kg N reduction per animal translates to an annual reduction of 42 m kg N excreted to pastures in urine. The potential impact of these UN levels on N leaching was modelled using a whole farm system model (WFM) (Romera et al., Reference Romera, Levy, Beukes, Clark and Glassey2012; Beukes et al., Reference Beukes, Romera, Gregorini, Macdonald, Glassey and Shepherd2017).
Table 4 Milk urea nitrogen concentration (MUN) values for current generation Jersey cows, average of all cows and Holstein-Friesian cows; mean urinary nitrogen (UN) using prediction equations of Burgos et al. (Reference Burgos, Fadel and De Peters2007), Jonker et al. (Reference Jonker, Kohn and Erdman1998), Kauffman and St-Pierre (2001) and Kohn et al. (Reference Kohn, Kalscheur and Russek-Cohen2002); and MUN and predicted UN for next generation offspring of bulls with MUN breeding value −2.4 mg/dl

Briefly, the WFM simulated a typical pasture-based dairy farm (3.2 cows/ha), with a uniform silt-loam soil, and simulated for the 2013 calendar year using actual climate data. The simulation incorporated the winter of 2013, the main leaching season. The total amounts of UN deposited during each grazing event, as recorded in the event files (WFM output), were regarded as representative of the offspring of MUN BV=0 bulls (‘average’). The daily UN and N fertiliser events were extracted from WFM output and simulated with the urine patch framework that runs APSIM (Agricultural Production Systems sIMulator) simulations for all the urine patch patterns to predict N leaching per paddock (for more details of the procedure see Romera et al., Reference Romera, Levy, Beukes, Clark and Glassey2012). The predicted N leaching for the typical farm stocked with ‘average’ cows for the 2013 calendar year was 63 kg/ha per year.
It was assumed that breeding across the population using bulls with MUN BV of −2.4 will produce offspring with MUN phenotype 1.2 mg/dl lower than ‘average’. This translated into a decrease of 9% in UN for the progeny of these bulls. The WFM output in terms of the amount of UN per grazing event (kilogram) was then scaled downwards by 9% and the output ran through the leaching prediction procedure again. Urine volumes, number of urinations and, therefore, volume per urination were assumed to stay the same for the ‘low-MUN’ cows, which meant that UN concentration decreased relative to the ‘average’ group. This is an important assumption since N leaching is determined by both the amount of N deposited as UN, and the concentration of the UN. It was also assumed that low-MUN cows did not change in terms of feed intake and milk production characteristics compared to the ‘average’ group. It predicted a decline in leaching on silt loam soils of 11 kg N/ha per annum from cows sired by bulls whose BV MUN averages −2.4 mg/dl. This equates to a decrease of 17% compared to ‘today’s model farm’, where the predicted annual leaching rate is 63 kg N/ha. At 3.2 cows/ha, as modelled, this represents a per cow reduction in N leached of 3.4 kg/annum.
Clearly if the MUN–UN relationship that exists for differentially fed cattle holds for differentially bred cattle, the potential of using low MUN BV sires to reduce N leaching in future generations is attractive. However, further work is required to confirm that animals bred for lower MUN excrete less UN similar to the MUN–UN relationship reported for cattle fed differently. This requires confirmation that animals that are genetically diverse for MUN partition dietary N from urine to other pools. Use of cattle that have predicted low v. high MUN BVs for such a study may be an efficient use of resources rather than randomly selecting animals from the population.
Desirability of breed change to reduce milk urea nitrogen concentration
Jersey cows had lower MUN and therefore are predicted to excrete less UN per day. Farmers might look to change breed as a strategy to mitigate N losses. Jersey cattle are smaller and produce less milk than HFs. Using New Zealand Dairy Statistics (2015–2016) cow production and live weight values, plus the energy requirements for cow maintenance and milk production used by NZAE Limited (2017) the average NZ J cow is estimated to require 85% of the intake required by the average NZ HF. Therefore, to utilise a finite feed resource, 17% more J cows are required per ha than HFs. In terms of UN excretion per ha, a J herd is expected to be similar to a HF herd despite the J’s 9% lower per cow predicted excretion rate (Table 4).
Predictor traits of milk urea nitrogen concentration
Selection for milk characteristics in dairy cattle has historically involved progeny testing bulls to accurately predict BVs based on daughter performance and this approach was the basis of the data underpinning this study. Progeny testing is expensive and time-consuming. Blood PU can be measured in young animals of both sexes and may afford an early predictor of the sex-limited trait MU. As urea is passively transmitted across membranes, blood PU concentration closely mirrors MU concentration (Burgos et al., Reference Burgos, Fadel and De Peters2007; Spek et al., Reference Spek, Dijkstra, van den Borne and Bannink2012). Plasma urea can be measured in all animals and the possibility that PU in young bulls may effectively predict PU and MU in daughters should be explored.
Acknowledgements
The authors appreciate the contributions of the following: staff at MilkTest NZ for their diligence in supplying the MU concentration phenotypes; Tony Smart at CRV Ambreed for maintaining the database including the MU measurements; and Pierre Beukes from DairyNZ for modelling N leached from herds excreting varying loads of UN. The authors thank Joanne Deely for editing the manuscript. Contribution of Callaghan Foundation support for CRV Ambreed Research is acknowledged. This study was initiated with funding from NZ dairy farmers through DairyNZ Incorporated and the Ministry for Primary Industries through the Primary Growth Partnership.
Declaration of interest
The authors declare no conflict of interest.
Ethics statement
The data used for this research was collected via routine herd testing of dairy cows by CRV Ambreed which is a licensed herd testing company.
Software and data repository resources
Neither the data nor models were deposited in an official repository.