Introduction
African countries striving to achieve rice self-sufficiency have proposed and implemented several interventions, particularly those aimed at narrowing yield gaps and improving producer welfare. However, little attention has been paid to interventions that would significantly improve consumer welfare. In many parts of Africa, consumers perceive the quality of rice in their local markets to be poor. Therefore, even though an increase in yield would certainly increase supply and consequently reduce prices, the reduction in prices is unlikely to proportionately increase quantity consumed in some countries owing to low price elasticities of demand such as those documented in Tollens (Reference Tollens2007), Ulimwengu and Ramadan (Reference Ulimwengu and Ramadan2009), Nzuma and Sarker (Reference Nzuma and Sarker2010), and Lazaro (Reference Lazaro2014). Instead, consumers may shift to the consumption of other foods since the market lacks rice of the desired quality. This lack of rice of the desired quality is partly due to the limited information on heterogeneity in consumer preferences that characterizes rice markets. Without this information, upgrading of Africa's rice value chains will remain an uphill task.
This study applies the hedonic pricing method, a revealed preference method (Loureiro, McCluskey, and Mittelhammer Reference Loureiro, McCluskey and Mittelhammer2003), to determine consumer preferences for rice in Benin, where rice is the second most important cereal after maize. In doing so, it accounts for market (consumer) sorting, failure of which would lead to inconsistent parameter estimates (Gustafson, Lybbert, and Sumner Reference Gustafson, Lybbert and Sumner2016). Market sorting arises from heterogeneity in consumer characteristics and preferences for quality attributes; consumers self-select into searching for certain attributes, which leads to a correlation between consumer characteristics and product attributes (Gustafson, Lybbert, and Sumner Reference Gustafson, Lybbert and Sumner2016). In other words, consumers with similar socio-demographic characteristics, constraints, and, hence, preferences are likely to make similar choices (Kuminoff, Smith, and Timmins Reference Kuminoff, Smith and Timmins2013). Danneels (Reference Danneels1996) refers to this as reverse market segmentation.Footnote 1 Therefore, the analysis essentially involves the determination of differential hedonic prices, which can then be used by marketers to build product profiles to more precisely and optimally target consumers and achieve greater market penetration. An exploratory case study by Futakuchi, Manful, and Sakurai (Reference Futakuchi, Manful, Sakurai, Wopereis, Johnson, Ahmadi, Tollens and Jalloh2013) revealed the existence of heterogeneity in consumer preferences for rice attributes in Benin.
Additionally, ensuring that quality meets consumer demand is increasingly becoming an integral part of breeding and crop improvement programs in Africa. As household incomes grow, it is expected that new breeding pipelines that include, for instance, biofortified grain will emerge. Such crop improvement programs often require huge public investments in research and development. But over the last decade, funding for national and international agricultural research has been declining, making priority setting based on rigorous impact assessment more imperative than ever before (Alene et al. Reference Alene, Abdoulaye, Rusike, Labarta, Creamer, del Rio, Ceballos and Becerra2018). Therefore, this study goes a step further to determine the likely impacts of grain quality improvement on consumer welfare.
Unlike Naseem et al. (Reference Naseem, Mhlanga, Diagne, Adegbola and Midingoyi2013) who applied a hedonic price model to Beninese consumers’ subjective perceptions of rice quality attributes, our study applies a hedonic price model to data obtained from laboratory tests of rice samples collected from different retail markets. The use of laboratory measures of quality, as in Unnevehr (Reference Unnevehr1986), Espinosa and Goodwin (Reference Espinosa and Goodwin1991), and Cuevas et al. (Reference Cuevas, Pede, McKinley, Valarde and Demont2016) rather than consumers’ and technicians’ sensory and semi-quantitative evaluation, provides comparatively reliable information on parameters relevant to the development of food products with sensory attributes desired by consumers (Singham, Birwal, and Yadav Reference Singham, Birwal and Yadav2015; Cuevas et al. Reference Cuevas, Pede, McKinley, Valarde and Demont2016). Laboratory tests on rice samples were conducted for physical and chemical characteristics associated with sensory attributes including appearance, aroma, texture, and flavor that are thought to influence consumer acceptance of rice. The attributes were then controlled for in a latent profile model to reveal the nature of hedonic prices of the underlying classes of consumers.
In the next section, we review empirical literature on the application of hedonic pricing to market sorting and to the determination of welfare impacts of quality improvement. Hedonic price functions encompass both supply and demand factors, and therefore, welfare changes accrue to both producers and consumers. However, the review of literature is restricted to consumer welfare, the focus of our study. The third section describes the economic model and the fourth section presents the data. This is followed by a discussion of the results in the fifth section. The last section summarizes and concludes the article with implications for rice breeding and upgrading of Africa's rice value chains.
Related Literature
Market (Consumer) Sorting
Economists have devoted considerable effort to studying hedonic pricing of extrinsic and intrinsic attributes of several goods such as foods, beverages, and houses. Rosen (Reference Rosen1974) intended that an empirical estimation of the hedonic price model involves estimation of the market-clearing implicit (hedonic) price function in the first stage, and the implicit marginal price function in the second stage. However, very rarely have economists been able to move beyond the first-stage estimation of the model because of theoretical and econometric challenges. The implicit marginal price function cannot provide new information without restrictions on its functional form (Brown and Rosen Reference Brown and Rosen1982). It suffers from simultaneity in that consumers with a strong preference for a given attribute will buy large quantities of products with this attribute (Bajari and Benkard Reference Bajari and Benkard2005). As such, heterogeneity in consumer preferences and consumer sorting have been found to be serious problems for conventional hedonic price estimation (Klaiber and Phaneuf Reference Klaiber and Phaneuf2009; Gustafson, Lybbert, and Sumner Reference Gustafson, Lybbert and Sumner2016), which assumes that consumers manifest homogeneous preferences (Nagler et al. Reference Nagler, Kronenberg, Kennelly, Jiang and Ma2012). To overcome these problems, a variety of techniques—some of which have been compared with the hedonic model—have been employed in the empirical literature. For instance, Klaiber and Phaneuf (Reference Klaiber and Phaneuf2009) compare estimates of hedonic and sorting models using residential sales and land-use data for east central Minnesota. Their sorting models are essentially random utility model specifications with and without observed heterogeneity and are implemented as in Bayer and Timmins (Reference Bayer and Timmins2007). The models yield similar results for some but not all variables. It is evident that the relatively high-resolution analysis achieved with sorting models comes at a cost of greater complexity in terms of data needs and theoretical assumptions. Gustafson, Lybbert, and Sumner (Reference Gustafson, Lybbert and Sumner2016) deal with consumer sorting in the American wine market by integrating consumers’ uninfluenced initial choice of wine purchase with their willingness to pay (WTP) for alternate wines in a valuation experiment. They estimate and compare two models: a typical hedonic price model (for all wines in the experiment) and a model in which WTP rather than actual wine price is the dependent variable. The WTP model is estimated in three forms: one with the same wine attributes as the hedonic model but with the addition of a dummy variable for the initially chosen wine; a model with wine attributes, a dummy for initially chosen wine and consumer characteristics; and a model with wine attributes, a dummy for initially chosen wine and a dummy for individual-specific fixed effects to account for sorting and unobserved consumer characteristics. Valuation estimates for wine attributes are found to vary significantly between the two approaches, and the model accounting for sorting and omitted consumer characteristics provides the most precise estimates of aggregate WTP for wine attributes. Bento, Li, and Roth (Reference Bento, Li and Roth2012) use the random coefficients multinomial logit model to incorporate consumer heterogeneity and avoid sorting bias in estimating U.S. consumers’ marginal WTP for future reductions in fuel costs.
While the above studies use the random utility framework to explicitly account for the parameters upon which market sorting is based, our concern is how to analyze market sorting using a hedonic pricing model when there are several unknowns, including the exact functional form of the model, the number of consumer categories, the consumer characteristics defining those categories, and the full vector of the relevant hedonic attributes. For example, regarding unknown hedonic attributes, Beninese consumers’ valuation of rice attributes may partly depend on the type of rice dish (djolof rice, white rice with soup, whatche, porridge, and dough) they desire for a given meal (breakfast, lunch, and dinner), and occasion (religious holidays and social events). This implies that some of the observed rice attributes may simply be proxy indicators of the levels of relevant attributes not directly observed by consumers and perhaps unknown to the analyst. Nagler et al. (Reference Nagler, Kronenberg, Kennelly, Jiang and Ma2012) demonstrate how a hedonic price model of market sorting can be applied to determine, for different categories of consumers, the gap between marginal WTP for a proxy attribute and marginal WTP for the attribute it represents. The authors observed the levels of the latter attribute from laboratory analysis of the products.
The present study adds to the empirical literature on market sorting by estimating a hedonic price function as a finite mixture linear regression model, which is robust for detecting differential effects when the number of consumer classes and predictors of class membership is not specified a priori, when the predictors are unreliable, and when the process that creates classes is complex, such as one involving multiple predictors that interact in multiple ways (Van Horn et al. Reference Van Horn, Jaki, Masyn, Howe, Feaster, Lamont, George and Kim2015). A similar approach has been used by Shiroya (Reference Shiroya2010) to understand household residential sorting patterns in Texas. More recently, Caudill and Mixon (Reference Caudill and Mixon2016) have applied the finite mixture model to a hedonic model of California and Washington wine prices to demonstrate its superiority over the Local Polynomial Regression Clustering (LPRC) procedure introduced by Costanigro, Mittelhammer, and McCluskey (Reference Costanigro, Mittelhammer and McCluskey2009). They find it to have better aggregate out-of-sample performance than the LPRC model.
Welfare Measurement
Conceptually, the measure of change in consumer welfare due to a change in a quality attribute is a measure of change in the consumer's utility. However, because utility is unobservable, a change in consumer welfare is often measured by a monetary measure of the consumer's own valuation of the change in their welfare. An example is the Hicksian compensating measure in the form of the maximum amount of money that the consumer is willing to pay to achieve the change, in the case of an improvement in quality, or the minimum amount that they are willing to accept to endure the change, in the case of a decline in quality (Day Reference Day2001).
Measurement of welfare effects due to changes in quality characteristics remains scarce in the empirical hedonic pricing literature. Palmquist (Reference Palmquist1988) attributes the scarcity to the complexity of analyzing welfare when hedonic price schedules are nonlinear. He proposes a technique for deriving exact measures of consumer welfare in a way that accounts for the nonparametric nature of prices. Still, the practice seems to have remained that of using implicit marginal prices as direct measures of welfare benefits since they represent the WTP for a marginal change in an attribute (see, for instance, Kim, Phipps, and Anselin Reference Kim, Phipps and Anselin2003). Harrison and Rubinfeld (Reference Harrison and Rubinfeld1978) and Chattopadhyay (Reference Chattopadhyay2002) use implicit marginal price functions (employed in the second-stage regression of Rosen's (Reference Rosen1974) procedure) to obtain estimates of household benefits from nonmarginal improvement in quality attributes in the housing market.
Hedonic estimates of marginal WTP can be used to derive partial equilibrium welfare effects (Kuminoff and Pope Reference Kuminoff and Pope2009) as demonstrated by Unnevehr (Reference Unnevehr1986) and Voon wand Edwards (Reference Voon and Edwards1991). Unnevehr (Reference Unnevehr1986) estimates hedonic prices and calculates changes in consumer surplus (CS) due to an improvement in rice grain quality in three Asian countries. The method is intuitive and, hence, provides an appropriate framework for the current study. On the contrary, Voon and Edwards (Reference Voon and Edwards1991) apply a hedonic price estimate obtained from the literature to determine the economic benefits to producers, input suppliers, marketers, and consumers of reducing backfat depth in Australian pork.
Economic Model
The hedonic price regression can be traced back to at least Court (Reference Court1938). Unlike the traditional theory of consumer demand in which goods are the direct objects of utility, the theory of hedonic pricing is based on the notion that utility is, in fact, derived, not from goods per se but from their hedonic attributes. Rosen (Reference Rosen1974) derives a model of product differentiation in a competitive market and provides an interpretation of the estimated hedonic (implicit) prices. Assume a market of differentiated products that are characterized by n attributes, z 1, …, zn. It is assumed that consumers have the same perception of the quantity of attributes in each product. Each product has an observed market price such that p(z) = p(z 1, …, zn). Rosen then derives consumption and production decisions and shows that in equilibrium, the implicit price function p(z) represents a joint envelope of a buyer's value function and seller's offer function.
Later, Ladd and Suvannunt (Reference Ladd and Suvannunt1976) derive the consumer goods characteristics model based on assumptions that specifically define the behavior of individual consumers. In this model, a product's observed market price is a weighted linear combination of the product's attributes, where each weight is the marginal implicit price of an attribute. Suppose Z 0j is the total quantity of attribute j provided by all products consumed, Zij is the quantity of attribute j provided by one unit of product i, Z 0m+i is the quantity of unique attribute m provided by product i, qi is the quantity consumed of product i, and I is income. Therefore,

The consumer maximizes

subject to

Hence,

The term in the first square brackets is the marginal rate of substitution between income (or expenditure according to equation 3) and attribute j. It is the marginal (implicit) price of attribute j. Notice that

where E is the total expenditure on all products and (δZ 0j/δqi) is the marginal yield of attribute j by product i. Thus, equation 4 implies that the observed market price of a product is equal to the sum of the marginal values of the attributes, where each attribute's marginal value is the quantity of the attribute per marginal unit of the product (i.e., yield) multiplied by the attribute's hedonic price. Equation 4 is the implicit (hedonic) price equation and can be rewritten as follows:

where the coefficients pij are the hedonic or implicit marginal prices of attributes, which can be interpreted as the marginal WTP for a change in the attributes since they encapsulate consumers’ preferences (Nerlove Reference Nerlove1995; Costanigro, McCluskey, and Mittelhammer Reference Costanigro, McCluskey and Mittelhammer2007), and u is the random error term. If an additive intercept is added to equation 6, the coefficients can be interpreted as premiums or discounts over a base price defined by the intercept.
To analyze market sorting, we apply latent class (LC) analysis to equation 5. LC analysis is a set of techniques used to model situations in which the observed data are comprised of a finite number of distinct classes (subgroups), but class membership is not directly observed (Canette Reference Canette2018). LC models permit disaggregation of a sample into existing latent classes using one or more observed dependent variables, which may be categorical or continuous.Footnote 2 In this study, we apply a finite mixture linear regression model,Footnote 3 a type of LC maximum-likelihood-based model derived from a mixture of underlying distributions, and use one dependent variable (Greene Reference Greene2008, p. 558; Costanigro, Mittelhammer, and McCluskey Reference Costanigro, Mittelhammer and McCluskey2009; Canette Reference Canette2018).
Following Greene (Reference Greene2008, p. 559), if a population consists of a mixture of two underlying normal distributions,Footnote 4 the contribution to the likelihood function for individuals or products in classes 1 and 2 is, respectively,


where yi is the outcome variable for individual or product i. Assuming that the proportion of individuals or products in class 1 is Prob(classi = 1) and those in class 2 is (1 − Prob(classi = 1)), and zi is the vector of variables (attributes) that might explain class probabilities, then the conditional density for product i is

The usual logit model can be used to parameterize the probabilities. The log-likelihood, in this case, features two random variables; the outcome variable y i and classi. For the more general case of K classes, the finite mixture model for variable y i can be specified as

where hk is the density conditioned on class k, zi is a vector of covariates, and γk is the kth parameter vector. However, even if it is impossible to conjecture the classes present in the sample, as is the case in this study, the model remains amenable to estimation as illustrated by Canette (Reference Canette2018).
Estimated hedonic prices can be used to calculate the change in CS due to a nonmarginal improvement in attributes. As in Unnevehr (Reference Unnevehr1986), we use a partial equilibrium model to this end. Improvement in a quality attribute causes a rightward shift in demand, and the gain, G, in CS per unit of the product consumed by a given class of consumers can be calculated as follows:

where $Z_{ij}^\ast$ is the new value of attribute j per unit of the product and pij is the estimated hedonic price for a given class of consumers. At the aggregate level, assuming the infinitely elastic supply of the improved product and constant production costs, the level of demand for the product after quality improvement, $q_i^\ast$
, is

where

is the elasticity of quantity consumed of the product with respect to the quantity of attribute j, and ɛ* is the own-price elasticity of demand for product i. These assumptions do not permit gain in producer surplus. The total gain in CS is

Unlike in Unnevehr (Reference Unnevehr1986), our study requires an estimate of ɛ* for each class of consumers. However, we do not have the necessary data to empirically obtain estimates of it, and turning to the literature, we find a paucity of empirical studies that have estimated ɛ* for rice in Benin. For all we know, the recent study by Codjo (Reference Codjo2019) has been the only attempt to estimate the parameter; he estimates ɛ* for three income groups (low, middle, and high) and three levels of rice quality and finds it to range between −0.91 and −2.69. Therefore, we use the average of Codjo's (Reference Codjo2019) estimates, and we conduct a sensitivity analysis for the relevant consumer classes in order to ascertain the robustness of our model's results.
Data and Estimation
In a study like this that aims to generate aggregate demand and CS based on sample data, ensuring that the sample is representative of the population is imperative. At the time of this study, a list of all rice retailers in the country and the types or brands of rice they sell was unavailable. Moreover, the cost of obtaining it and collecting samples from retailers who are dispersed throughout the country would have been prohibitive. Nonetheless, we were able to obtain rice samples representative of the rice consumed in the country's four official geopolitical and cultural regions, namely Northeast, Northwest, Central, and South. For each region, a list of all food markets was obtained, from which a random sample of markets was drawn. The number of markets randomly selected from each region was proportional to the region's share of markets in the country. Thus, a total of 33 markets were randomly selected: South (20), Central (4), Northwest (6), and Northeast (3). In each market, rice retailers and the type(s) of rice they sell were identified, and rice samples randomly collected. Only one sample per rice type or brand was obtained from each selected retailer. A total of 316 rice samples, which is adequate for our finite mixture model (Van Horn et al. Reference Van Horn, Jaki, Masyn, Howe, Feaster, Lamont, George and Kim2015), was obtained: South (176), Central (35), Northwest (64), and Northeast (41).
The distance of each market (in km) from the Cotonou port was recorded. The Northeast and Northwest of Benin are about 800–1,000 km from Cotonou port, and the populations there have a history of rice cultivation. The Northeast is the most important rice-producing region in Benin and harbors one of the country's rice sector development hubsFootnote 5—Malanville. The Central region is about 300–500 km from Cotonou port and harbors the other rice hub, Glazoue. Unlike Malanville, which is irrigated, the Glazoue hub is rainfed. The South is close (<300 km) to Cotonou port and is home to Cotonou city, the largest city in Benin, and to Porto-Novo, the country's political capital. Most of the inhabitants of this region do not have a rice cultivation history.
A detailed description of the grain quality analysis that produced data on the attributes used in the hedonic pricing model is available from the authors on request, and summary statistics of the physical (extrinsic) and chemical (cooking) attributes used in the model are presented in Table 1. Extrinsic attributes include moisture content, head rice (proportion of intact grains), length–width ratio (grain shape), chalkiness (white belly/opaque portion of a rice grain), lightness/whiteness, distance (km) of a market from Cotonou port, market type (1 = rural, 0 = urban), rice type (1 = parboiled, 0 = nonparboiled), and origin (1 = imported, 0 = local). A high moisture content of above 14 percent is undesirable as it leads to a deterioration of grain during storage (Tang et al. Reference Tang, Ndindeng, Bigoga, Traore, Silue and Futakuchi2019). The distance of a market from the port, type of market, and origin of rice are informational cues that may influence consumers’ perception of the quality of rice. For instance, the type of market may account for ease of access to market and quality information about the product, while the origin of a product may influence consumers’ perception of its quality (Elliott and Cameron Reference Elliott and Cameron1994), in addition to capturing consumers’ ethnocentric attitudes.
Table 1. Summary Statistics of Variables Used in the Analysis (n = 316)

Chemical attributes include amylose content, setback viscosity, and alkali spreading value (ASV). Amylose content determines the texture of cooked rice, whereby the greater the amylose content, the drier, the less sticky, and less tender the rice is. Setback viscosity, an indicator of the pasting quality of rice, determines the firmness of cooked rice, with higher values indicating greater firmness after cooling. The ASV is used to measure gelatinization temperature and, hence, cooking time. The higher the ASV, the lower the gelatinization temperature and, hence, the shorter the cooking time.
Results
The hedonic price finite mixture regression model was estimated in the form of a log-linear (double-log) model,Footnote 6 and Akaike Information Criterion (AIC) and Bayesian Information Criterion (BIC) were used to determine the number of distinct consumer classes present in the data. Both criteria found three classes, as shown in Table 2. We find that about 5 percent of the observations are in class 1 that pays an average rice price of $1.32/kg, while 56 percent are in class 2 with an average price of $0.89/kg, and 39 percent are in class 3 with an average price of $1.00/kg. Table 3 summarizes the results of the three-class model. In a log-linear model, coefficients on continuous regressors are elasticities, while those on discrete ones show the discontinuous effect on the regressand. Therefore, we calculate and report hedonic prices of the statistically significant attributes. The hedonic price of each continuous attribute is calculated as the product of the attribute's coefficient and the average price of rice divided by the average value of the attribute, and it reflects the change in the market price of rice for a one-unit change in the attribute. For a discrete attribute, however, the hedonic price is the effect on the price of rice of one category relative to another. It is calculated as (e β − 1), and the percentage effect is 100(e β − 1), where β denotes the coefficient (Halvorsen and Palmquist Reference Halvorsen and Palmquist1980).
Table 2. Results of Akaike Information Criterion (AIC) and Bayesian Information Criterion (BIC) for Model Selection

Table 3. Estimated Regression Results of the Finite Mixture Model

Dependent variable is the natural log of rice prices. Figures in parentheses are robust standard errors.
The smallest class (class 1) exhibits several unique characteristics: it is sensitive to all but one attribute—moisture content—and it is willing to pay price premiums for the relatively expensive imported rice and rice with chalkier grains, while discounting rice with a relatively high proportion of intact grains (head rice), lighter grains (lightness), rice that becomes firm on cooling after cooking (setback viscosity), and rice sold in markets far from the port of Cotonou. The other characteristics of this class, although not unique to it, are that it likes rice with slender grains, nonsticky rice when cooked, and shorter cooking time, and it discounts parboiled rice and rice sold in rural markets. Taken together, these characteristics point to a category of consumers that is likely to be comprised of relatively high-income consumers including foreign nationals in and around major urban centers such as Cotonou city.
Classes 2 and 3 do not care for the origin of the rice they consume, a finding that is contrary to that of Naseem et al. (Reference Naseem, Mhlanga, Diagne, Adegbola and Midingoyi2013), who concluded that rice consumers in Benin prefer imported to local rice. Consumers in the largest class (class 2) are sensitive to only five of the 12 attributes. They discount parboiled rice, rice sold in rural markets, and rice with chalky grains, but are willing to pay price premiums for head rice and amylose content. When comparing the hedonic prices in this class with those paid by consumers in the other two classes, it is quite clear that consumers in class 2 exhibit relatively weak preferences. For instance, they discount chalkiness by $0.006/kg, which is only 30 percent of the discount paid by consumers in class 3, and parboiled rice by 32 percent ($0.21/kg) of the discount paid by consumers in class 1. For class 2, gain in consumer welfare would likely come from a reduction in chalkiness, an increase in head rice, and an increase in amylose content.
Consumers in class 3 are sensitive to half of the attributes. They are willing to pay the same price premium for rice with a relatively high proportion of intact grains as consumers in class 2. They like slender grains, lighter grains, and rice that cooks fast. However, they discount rice with chalky grains and are the only class that discounts rice that does not stick together when cooked. Also notable about this class is that it is the only one that is indifferent about the type of market in which rice is sold. Based on these characteristics, this category of consumers is likely comprised of periurban and rural consumers, and it would gain from an increase in head rice and reduction in chalkiness, among other things.
To determine the consumer welfare implications of grain quality improvement, we consider improvement in two attributes whose coefficients are statistically significant and of the same sign in classes 2 and 3. The two classes together comprise 95 percent of the observations. We consider an increase in head rice by 30 percent and an equivalent reduction in chalkiness. Both attributes can be improved upon through breeding and better postharvest handling and processing. Parameters used in the partial equilibrium model of quality improvement and the results obtained are summarized in Tables 4 and 5, respectively.
Table 4. Parameters Used in the Partial Equilibrium Model of Quality Improvement
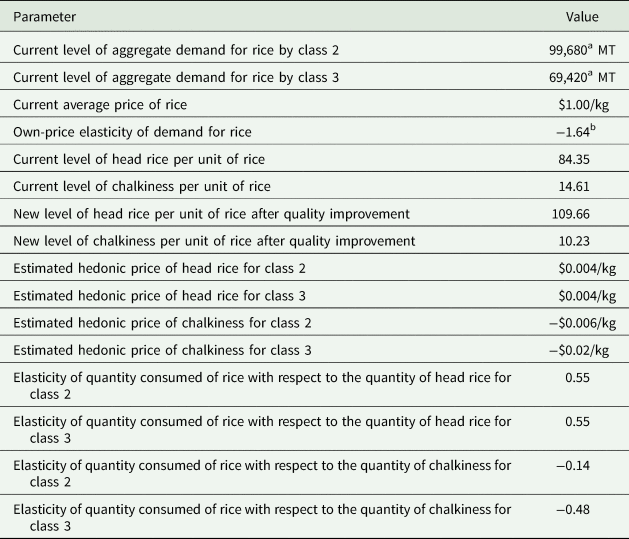
a Aggregate demand for rice in Benin was projected to reach 178,000 MT in 2018 (Republic of Benin 2011). We assume demand by a consumer class to be proportional to the share of the class in the sample.
b Source: Authors’ calculation based on Codjo's (Reference Codjo2019) estimates.
Table 5. Results of the Partial Equilibrium Model of Quality Improvement

We find that better head rice increases the demand for rice to 154,837 and 107,833 MT for classes 2 and 3, respectively. This represents a 55 percent increase in demand by each class. A reduction in chalkiness causes demand to increase by 14 and 48 percent, in classes 2 and 3, respectively. The combined annual gains in CS for the two classes are $21,852 for head rice and $10,353 for chalkiness. Since this study addresses a practical matter concerning upgrading rice value chains, it is important to consider not only the direction of change in CS but also the magnitude of the change. The gains in CS appear to be modest. A benefit–cost analysis would be informative in assessing the feasibility of investment in quality improvement. However, an attempt to determine the costs for Benin alone was met with difficulty. As has been observed in the literature (see, for instance, Pardey et al. Reference Pardey, Alston, Chan-Kang, Magalhaes and Vosti2002), the matching of research benefits to costs is a rampant problem, especially where collaborative research is involved and information on costs is not clearly documented. Rice varietal improvement programs in Africa have been led by the Africa Rice Center and the International Rice Research Institute in collaboration with National Agricultural Research Institutes such as the National Institute of Agricultural Research of Benin. The programs and their associated costs are typically spread across several regions and countries. Because of the lack of information, we were unable to calculate the relevant costs, let alone apportion them to the different activities and countries. This is an area for further research. But going by the observed internal rates of return to rice genetic improvement that range from 18 to 182 percent in West and Central Africa (Tollens et al. Reference Tollens, Demont, Sie, Diagne, Saito, Wopereis, Wopereis, Johnson, Ahmadi, Tollens and Jalloh2013), it is plausible that even in the case of Benin, the present value of benefits from grain quality improvement is highly relative to the present value of costs. Nonetheless, we are careful not to make broad-stroke recommendations for quality improvement based on the relatively small welfare impacts obtained in this study.
To examine the robustness of our results, we undertake a sensitivity analysis by varying the price elasticity of demand for rice, while holding other parameters constant. We consider 10, 20, and 30 percent increases and reductions in the price elasticity and observe corresponding changes in CS. The results are presented in Table 6. Generally, our model results are not very sensitive to changes in the price elasticity of demand. For instance, a 10 percent increase in the price elasticity increases the annual gain in CS from improvement in head rice by 2.16 percent for class 2, while a 30 percent increase would improve CS by 6.50 percent. Likewise, with respect to improvement in chalkiness for class 3, a 10 percent reduction in elasticity would reduce annual gains in CS by 1.93 percent, while a 30 percent reduction would cut gains in CS by 5.80 percent.
Table 6. Gain in Consumer Surplus (USD) for Different Price Elasticities of Demand

Summary and Conclusions
This study uses a hedonic price model to examine market sorting in the Beninese rice market and examines the effect of grain quality improvement on CS. It applies data obtained from laboratory tests of grain quality traits. Controlling for, among other things, two attributes, namely the origin and type of rice that are commonly used to characterize the country's consumer categories, the study has established that there are at least three classes of consumers in the Beninese rice market, manifesting in proportions of 5, 56, and 39 percent. Of the three classes, the smallest class pays the highest price, significantly discounts parboiled rice, and prefers imported to local rice. The second class also discounts parboiled rice but does not place a significant value on the origin of rice, whereas the third category values neither the origin nor the type of rice. Similarly, preferences for other quality attributes vary from one class to another.
Hedonic prices obtained for head rice and chalkiness are applied to a partial equilibrium model to determine the welfare implications of quality improvement for categories 2 and 3. The first important outcome from this exercise is the computed elasticities of demand for rice with respect to the quantities of the two attributes. This is especially informative because these elasticities enable breeders and private seed companies to quickly understand how consumers will respond to grain quality improvement. We then find that an increase in head rice and a reduction in chalkiness autonomously lead to modest gains in consumer welfare.
These results have two important implications for upgrading rice value chains in Benin and other African countries. First and foremost, any upgrading strategy ought to begin by recognizing potential heterogeneity in consumer preferences for rice quality attributes and that existing product categories may not fully capture the heterogeneity. As demonstrated in this study, each class of consumers has a unique hedonic price function and almost all quality attributes—head rice, grain shape, chalkiness, lightness, cooking time, and amylose content—can be targeted for product upgrading. Therefore, upgrading rice value chains requires information on the nature of demand or hedonic price function of the different categories of consumers.
Second, knowledge of the impacts of quality improvement on consumer welfare is informative for setting priorities for spending on research and development. For instance, although our estimates are generally small on economic impact, we see that an improvement in head rice leads to far greater benefits for consumers than an improvement in chalkiness. Our analysis, therefore, demonstrates a straightforward application of hedonic pricing and partial equilibrium methods to evaluating priorities for crop improvement and value chain upgrading. Currently, there are ongoing efforts by the CGIAR Consortium to develop product profiles of mega rice varieties in Africa in order to achieve certain development outcomes. The analysis used in this study can be integrated in the proposed three-stage approach to product profiling. Of course, it would be even more enlightening to consider net welfare changes by accounting for impacts on producers.
Data Availability Statement
Data used in this study are available from the corresponding author upon request.
Acknowledgments
The authors wish to thank two anonymous reviewers for their suggestions, which significantly improved this article.
Funding Statement
This work was supported by the Department of Foreign Affairs, Trade and Development of the Government of Canada under Grant No. A034968.
Conflicts of Interest
None.