Introduction
There is a growing interest in, and emphasis on, ageing in both the marketing literature and business practice. Many aspects of ageing attract attention (e.g. Goldberg, Reference Goldberg2009; Yoon et al., Reference Yoon, Cole and Lee2009; Moschis, Reference Moschis2012; Berg, Reference Berg2015; Host et al., Reference Host, McMahon, Walton and Charlton2016; Gentry and Mittelstaedt, Reference Gentry and Mittelstaedt2017; Kłopocka, Reference Kłopocka2017). There are, though, relatively few publications focused on ageing and consumer behaviour (e.g. Cole et al., Reference Cole, Laurent, Drolet, Ebert, Gutchess, Lambert-Pandraud, Mullet, Norton and Peters2008), despite authors emphasising its significance and the need for further research (Fang et al., Reference Fang, Wen, George and Prybutok2016; Zniva and Weitzl, Reference Zniva and Weitzl2016; Kuoppamäki et al., Reference Kuoppamäki, Taipale and Wilska2017; Van Droogenbroeck and Van Hove, Reference Van Droogenbroeck and Van Hove2017; Lee and Lyu, Reference Lee and Lyu2019).
The internet and e-commerce play an increasing role in society and the economy, altering consumer behaviours. Older generations are generally understood to use such technologies less and in more-restricted ways than younger generations. This is, however, under-researched and variations within the older generations have not been considered.
Our study enhances understanding of the underdeveloped theme of ageing consumers and e-commerce activities. Our overall research question is: what is the impact of age and individual and household characteristics on the online behaviour of older consumers? Given the rise of e-commerce and the increased expectation from many businesses (and other organisations) that all consumers and society are digitally and technology enabled, this topic is important. Whilst not explicitly the focus of this study, the relevance of this work has increased in the context of digital access and digital poverty during the COVID-19 pandemic.
Our paper consists of six sections. This Introduction is followed by the Literature Review, contextualising the research and emphasising the gaps in knowledge that we address. The Methods section presents and justifies the approach to our research. The Results and Discussion section provides the findings and analysis of the research. Conclusions and Practical Implications demonstrate the contribution of our research and the added value for business and marketing practice and theory. Finally, we provide a Limitations and Future Research section.
Literature review
Ageing processes – new research necessary
Whilst we understand more about the dynamics of ageing processes (Mortimer and Shanahan, Reference Mortimer and Shanahan2007; George and Ferraro, Reference George and Ferraro2015), more depth and detail are needed about the lifestyle and behavioural consequences of ageing and their interactions with everyday activities (Fowler et al., Reference Fowler, Gentry and Reisenwitz2015). More rigorous and holistic ageing studies (providing more data on wider aspects) can help fill this gap in knowledge. Qualitative, in-depth and individual-oriented approaches can provide insights (Eriksson and Kovalainen, Reference Eriksson and Kovalainen2015), as can quantitative approaches providing generalisations (Antwi and Hamza, Reference Antwi and Hamza2015). Cross-sectional studies in the ageing context, including mixed-method approaches (Rahman and Areni, Reference Rahman and Areni2016), might provide benefits. A longitudinal investigation, however, would provide perspectives on trends over time and causal relationships, especially if connected to administrative datasets (Banks et al., Reference Banks, Nazroo and Steptoe2012). The goal is an approach that would enable research to focus on the multi-dimensional aspects of the ageing process, providing a fuller picture of its complexity and consequences.
The approach outlined in the previous paragraph is neither simple nor quick. Healthy Ageing in Scotland (HAGIS) was designed to achieve these broad and long-term goals (the details of HAGIS are found in the Methods section). We have access to the consumer behaviour component of the pilot phase of this long-term data collection project and it is that data that we use in this analysis to fulfil our research need. This gives us a baseline snapshot of ageing consumers and e-commerce activities.
This topic is important for at least two reasons. First, businesses rely on consumers and as demographics shift to an older population, so businesses need to be aware of, and respond to, their needs, wants and capabilities. Secondly, these capabilities have, and continue to, change, very notably in the field of technology. Businesses have adapted rapidly to changing technologies, most notably the internet, and are expecting consumers to make the same adaptations. However, the desire and capability to use aspects of these technologies in e-commerce is not well understood. Individuals who grow up embedded in the technology often have a different capacity and reaction to that technology to those who have to learn its use in later life. Research, thus, has consumer and business implications as well as contributing to the understanding of ageing and society.
The meaning and consequences of ageing for business
Older consumers’ capabilities and activities are important for business. Ageing affects consumer behaviour, including decision making (Cole et al., Reference Cole, Laurent, Drolet, Ebert, Gutchess, Lambert-Pandraud, Mullet, Norton and Peters2008; Goldberg, Reference Goldberg2009; Yoon et al., Reference Yoon, Cole and Lee2009), cognition and persuasion (Selwyn et al., Reference Selwyn, Gorard, Furlong and Madden2003; Yoon et al., Reference Yoon, Laurent, Fung, Gonzalez, Gutchess, Hedden, Lambert-Pandraud, Mather, Park, Peters and Skurnik2005; Wilson, Reference Wilson2018), product evaluation attributes (Wei et al., Reference Wei, Donthu and Bernhardt2013), e-marketing (Taylor and Strutton, Reference Taylor and Strutton2010), brand awareness (Lambert-Pandraud et al., Reference Lambert-Pandraud, Laurent, Mullet and Yoon2017), advertising (McKay-Nesbitt et al., Reference McKay-Nesbitt, Manchanda, Smith and Huhmann2011), reactions to the market (Silvera et al., Reference Silvera, Meyer and Laufer2012), sensitivity to ethical aspects (Cowart and Darke, Reference Cowart and Darke2014) and luxury products purchase (Amatulli et al., Reference Amatulli, Guido and Nataraajan2015). As the number of older consumers grows, so do the implications for a variety of sectors, including finance and banking (e.g. Rytelewska and Kłopocka, Reference Rytelewska and Kłopocka2010; Milner and Rosenstreich, Reference Milner and Rosenstreich2013; Harris et al., Reference Harris, Cox, Musgrove and Ernstberger2016), tourism (e.g. Eby and Molnar, Reference Eby and Molnar2002; Borges et al., Reference Tiago MTPM, Couto, Tiago and Dias Faria2016), food (e.g. Silvera et al., Reference Silvera, Meyer and Laufer2012) and retail (e.g. Lange and Velamuri, Reference Lange and Velamuri2014; Van Droogenbroeck and Van Hove, Reference Van Droogenbroeck and Van Hove2017; Bae et al., Reference Bae, Jo, Han and Lee2018; Rybaczewska and Sparks, Reference Rybaczewska and Sparks2020). Many marketing practices and activities are designed by people far younger than the target group for these activities. This can lead to tensions and discrepancies between the desired and received message, and practitioners and older consumers (Thompson and Thompson, Reference Thompson and Thompson2009). The consequences can be lower sales for businesses, unmet demands of older consumers, and dissatisfaction with the products and services on the market (Cameron et al., Reference Cameron, Richardson and Siameja2016). Businesses need a better understanding of older consumers’ behaviours, including their online and e-commerce behaviour.
E-commerce from the perspective of consumers’ ageing
Technology usage and awareness are increasing in the United Kingdom (UK) (internet sales were at 20 per cent of all retail sales in early 2020 and went up to 33% during the COVID-19 pandemic), making it the world's third largest e-commerce market and the most advanced e-commerce market in Europe (Falk and Hagsten, Reference Falk and Hagsten2015; Butcher, Reference Butcher2020; Office for National Statistics, 2020; Ogonowski, Reference Ogonowski2020; Statista, Reference Statista2020). Studies concerning e-commerce and ageing are limited (Zniva and Weitzl, Reference Zniva and Weitzl2016), however, with a consistent emphasis on the need for further investigation (e.g. Tatnall and Lepa, Reference Tatnall and Lepa2003; Kim and Forsythe, Reference Kim and Forsythe2010). Despite the widely accepted belief that age is a strong negative predictor of internet engagement (Sourbati, Reference Sourbati2009; Haight et al., Reference Haight, Quan-Haase and Corbett2014), its influence in the specific context of e-commerce is underexplored. Gaps in ageing e-commerce knowledge include how goods and services are accessed (e.g. physical shop or internet) and the suitability of the system and the offer. Age needs to be taken into consideration for all marketing and retailing activities (Groeppel-Klein et al., Reference Groeppel-Klein, Helfgen, Spilski and Schreiber2017). The gaps in knowledge around technology use by older consumers for e-commerce purposes and variations likely over age due to experience with technology lead us to our first research question:
• RQ1: How does the age of older consumers affect their e-commerce behaviour?
To address this research question, we considered types of activities connected with e-commerce and the particular skills required/helpful around the online purchase decision-making process. Our access to data was structured around the HAGIS data collection (see the Methods section). Consequently, we disaggregated the first research question to four sub-questions concerning various technology and consumer behaviours: sending/receiving emails (RQ1a), finding information about goods/services via the internet (RQ1b), online shopping (RQ1c) and online financial transactions, i.e. banking, paying bills, etc. (RQ1d). These components are derived from the most commonly identified aspects of e-commerce behaviour by the respondents and they encompass the key areas of technology/online activities (Figure 1).
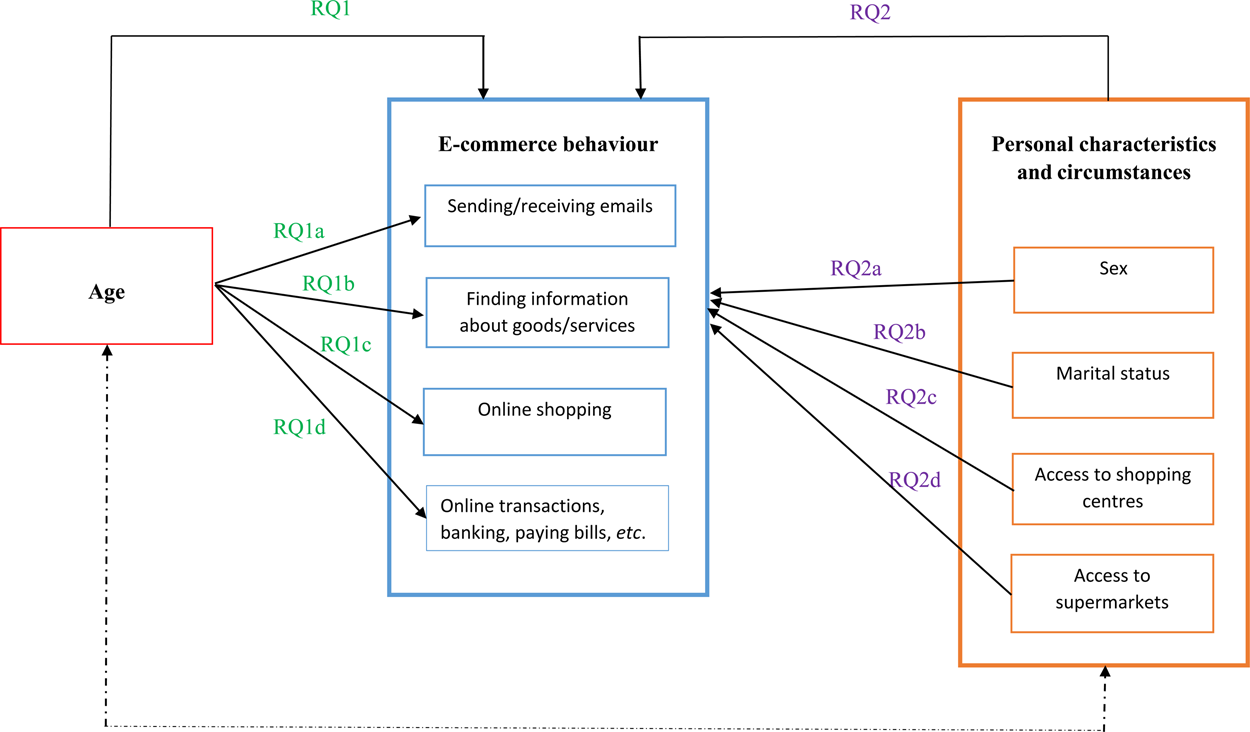
Figure 1. Conceptual model.
Note: RQ: research question.
Age and lifestyle/circumstances
Whilst physical age may alter behaviours, it is also likely that personal (including household) circumstances and lifestyle will be as important (Yoon et al., Reference Yoon, Cole and Lee2009; Zniva and Weitzl, Reference Zniva and Weitzl2016). These encompass information sources (Moschis et al., Reference Moschis, Mathur and Smith1993), overall cognitive abilities (Yoon et al., Reference Yoon, Laurent, Fung, Gonzalez, Gutchess, Hedden, Lambert-Pandraud, Mather, Park, Peters and Skurnik2005; Kohlbacher and Chéron, Reference Kohlbacher and Chéron2012), behaviour (Hopkins et al., Reference Hopkins, Roster and Wood2006), attitude (Burnett, Reference Burnett1996) and values (Moschis and Ong, Reference Moschis and Ong2011; Loroz and Helgeson, Reference Loroz and Helgeson2013). Focusing on the heterogeneity of age cohorts and following suggestions by Moschis et al. (Reference Moschis, Mathur and Smith1993), Yoon et al. (Reference Yoon, Laurent, Fung, Gonzalez, Gutchess, Hedden, Lambert-Pandraud, Mather, Park, Peters and Skurnik2005) and Zniva and Weitzl (Reference Zniva and Weitzl2016) on the importance of personal characteristics and circumstances of individuals, we developed our second research question:
• RQ2: How do individual and household characteristics and circumstances influence the e-commerce behaviour of older consumers?
Personal characteristics such as sex and marital status of the respondents are expected to influence behaviours, including technology usage. The other aspect available to us in the HAGIS data is access to supermarkets and shopping centres. It is likely that those with better local access will have less need to use e-commerce activities, particularly in the case of shopping centres. This measure, however, can only be a proxy for household access and location. Consequently, the second research question (RQ2) is disaggregated to four sub-questions separating individual and household characteristics and circumstances: sex (RQ2a), marital status (RQ2b), access to shopping centres (RQ2c) and access to supermarkets (RQ2d), as shown in Figure 1.
Methods
We investigate ageing consumers and their e-commerce activities within the UK – the world's third largest e-commerce market. More specifically, we concentrate on Scottish data – HAGIS – the first longitudinal research on ageing in Scotland. HAGIS is intended to build a longitudinal sample, linked to administrative data (Douglas et al., Reference Douglas, Rutherford and Bell2018). A pilot wave has been developed and operationalised. HAGIS uses an innovative sample frame designed to produce a random sample of eligible households (i.e. older respondents – here 50+ years old) across mainland Scotland (Douglas et al., Reference Douglas, Rutherford and Bell2018). The sample design is based on a proportionally stratified and clustered sampling approach with a subsequent phase of screening by the National Records of Scotland. This produces a representative sample of households with the potential to tie these to administrative records and to ‘follow’ them over a long period of time.
The HAGIS main questionnaire was administered as part of a household interview. All participants who responded to the main interview were also asked to complete a self-completion questionnaire, designed by the HAGIS team. A total of 1,054 main interviews were completed in the pilot wave and 67 per cent of respondents completed the self-completion questionnaire; 88 per cent of all interview respondents agreed to link their survey to at least one source of administrative data, developing the longitudinal base for future work. Data were sought on a wide variety of subjects, of which e-commerce was one (HAGIS, 2017). This paper is based on both questionnaires from this pilot wave; subsequent development of HAGIS will build on this over time. This pre-defined dataset can be considered a limitation as it was not designed and collected for our specific purpose. Nonetheless we believe it has value in answering our research questions. The limitations are considered further at the end of the paper.
Data concerning the demographics of the respondents (age, sex and marital status) are provided by the HAGIS main questionnaire. A very small number of respondents were recruited in the final age band (90 and over). In order to remain true to the HAGIS data collection they have been retained in the analysis. The self-completion questionnaire provides information on specific aspects of computer, internet and e-commerce activities, namely sending/receiving emails, finding information about goods and services via the internet, and online shopping/buying goods and services. These three aspects are recognised as steps of growing confidence in e-commerce activities, i.e. using the most common function, searching the internet and then purchasing online. There is a logic in considering this sequence as a ladder comprising separable components.
To answer the research questions, we have chosen to conduct predictive analysis addressing the probability of specific e-commerce behaviour of older consumers (i.e. sending/receiving emails, finding information about goods/services via the internet, online shopping and online financial transactions) contextualised within the individual and household characteristics. Since our dependent variables are dichotomous (binary), logistic regression models are applied (following the approach of Akinci et al., Reference Akinci, Kaynak, Atilgan and Aksoy2007 and Peng et al., Reference Peng, Lee and Ingersoll2002). We acknowledge that our approach is not the only one possible in this context, but given the specific characteristics of the dataset and our desire to establish correlations, we believe logistic regression is the most effective method at this stage (alternatives would be used when future waves have been generated). We created four logistic regression models with the identified dependent variables: sending/receiving emails (Table 1), finding information about goods and services via the internet (Table 2), online shopping/buying goods and services (Table 3) and online financial transactions, i.e. banking, paying bills, etc. (Table 4). The reference group for the logistic regression models is composed of married women aged 55–59, having very easy access to a shopping centre and to a medium or large supermarket.
Table 1. The results of estimation of the logit model for sending/receiving emails

Note: Ref.: reference group.
Source: Authors’ own upon Healthy Ageing in Scotland data.
Significance levels: *p < 0.05, **p < 0.01, ***p < 0.005.
Table 2. The results of estimation of the logit model for finding information about goods and services via the internet

Note: Ref.: reference group.
Source: Authors’ own upon Healthy Ageing in Scotland data.
Significance levels: * p < 0.05, *** p < 0.005.
Table 3. The results of estimation of the logit model for online shopping/buying goods or services

Note: Ref.: reference group.
Source: Authors’ own upon Healthy Ageing in Scotland data.
Significance levels:* p < 0.05, **p < 0.01, ***p < 0.005.
Table 4. The results of estimation of the logit model for online banking, paying bills, etc.

Note: Ref.: reference group.
Source: Authors’ own upon Healthy Ageing in Scotland data.
Significance levels: *p < 0.05, **p < 0.01, ***p < 0.005.
Results and discussion
E-commerce activities – descriptive analysis
We begin with a description of the internet usage by older consumers. Figure 2 shows that within the age cohorts the biggest group of every day internet or email users appears amongst respondents aged below 60 (over 87%). Then it drops rapidly by age 75–79 (when it equals less than half of the highest value, i.e. 42%). If we concentrate on the device on which respondents access the internet, the most commonly used devices include smartphone (35.8%), tablet computer (24.2%) and television (14.2%) both overall and within the majority of age cohorts. Desktop computers and laptop computers are not the preferred devices (a laptop is popular only among those aged 70–74, 80–84 and 85–89 years old, additionally these last two age cohorts use desktop computers as well). The three most often chosen options for accessing the internet (Figure 3) are at home (43.2%), on the move (28.9%) and in another place, e.g. library (12.2%). The most frequent household situation is living with a spouse (50.4%) before living as a single person (43.2%). This is age related, with the former describing the situation of the majority of respondents prior to age 75, and the latter for cohorts over 75.

Figure 2. Descriptive statistics on average usage of the internet or email and the device on which respondents access the internet by age cohort.
Note: TV: television.
Source: Healthy Ageing in Scotland data.

Figure 3. Descriptive statistics of the place where the respondents use the internet and household size by age cohort.
Source: Healthy Ageing in Scotland data.
Figure 4 shows that sending/receiving emails is the most popular online activity amongst respondents aged 60–64 (50.7%) but drops rapidly by age 75–79 (31.5%). Finding information about goods and services was reported most often by respondents aged 65–69 (50.0%) but again decreases by age 75–79 (27.9%). Figure 4 also provides information about shopping/buying goods and services on the internet. This was most frequent in the 50–54 age group (44.2%), reducing at ages 70–74 (33.6%) and 75–79 (26.1%). Online banking is practised by a decreasing proportion of respondents in every older age cohort, with the only exception being the 65–69 age cohort which is more familiar with this activity (30.6%) than the two younger age cohorts (27.9 and 27.4%, respectively).

Figure 4. Descriptive statistics on shopping/buying goods and services, online banking, paying bills, etc., sending/receiving emails, finding information about goods and services by age cohort.
Source: Healthy Ageing in Scotland data.
Age and other individual/household characteristics/circumstances – logistic regression models
Sending/receiving emails
Analysis of age influence on the first online activity (Table 1) showed that respondents aged 75 and over are less likely to send/receive emails than those aged 55–59. The logit model did not produce any statistically significant results for younger cohorts. Consequently, it can be suggested that a behavioural ‘breaking point’ is the age of 75 (not earlier) and people aged 75–79 are 45 per cent less likely to use email than respondents aged 55–59. Further investigation of sending/receiving emails (Table 1) did not provide statistically significant results in terms of sex and access to supermarket (with only one exception: respondents not accessing the supermarket are less likely to use email). Therefore, components of the second research question (RQ2a and RQ2d) in this context do not reveal any influence. Concurrently, a difficulty in accessing shopping centres makes respondents more likely to communicate via email in comparison with those having very easy access to shopping centres (RQ2c). In terms of the marital status of respondents (RQ2b), widowed, single and divorced persons are less likely (57, 41 and 51%, respectively) to use email than married ones (in all three cases statistically significant logistic regression results were obtained).
Finding information about goods/services
We investigated the online searching information about goods and services by consumers aged 50 and over (Table 2). No statistically significant results were obtained in terms of sex (RQ2a) and access to supermarkets (RQ2d). If the difficulty of accessing a shopping centre is considerable, people are more likely to use the internet to find information about goods and services (with the exception of those who are unable to go to shopping centres) than those having very easy access to such amenities (RQ2c). Marital status provides statistically significant results. The probability that widowed, living with partner, single and divorced persons would search online for information about goods and services is lower (60, 53.2, 44.1 and 48.8%, respectively) in comparison with those being married (RQ2b). Age cohort analysis (RQ1b) showed that the statistically significant results are achieved for age 75–79 and older cohorts. The probability that those aged 75–79 would use the internet to find information about goods and services is 49 per cent lower in comparison with those aged 55–59 years old and decreases for older cohorts.
Online shopping/buying goods and services
Shopping/buying goods and services, as the key aspect of e-commerce, was the dependent variable of the third logit model (Table 3). Analysis did not provide any statistically significant results concerning sex (RQ2a) and access to supermarkets (RQ2d). People having either easy or quite difficult access to shopping centres are more likely to use the internet for shopping/buying goods or services than those having very easy access to shopping centres (RQ2c). Marital status (RQ2b) again provided statistically significant results for widowed, single and divorced persons (i.e. probability that widowed persons would shop online is 49 per cent lower in comparison with those being married). Analysis of age cohorts (RQ1c) showed that the statistically significant results are achieved for age 75–79 and older cohorts. The probability that those aged 75–79 would shop online is 41 per cent lower in comparison with those being 55–59 years old and decreases for older cohorts.
Online banking, paying bills, etc.
The fourth logit model addressed online financial transactions (banking, paying bills, etc.) as the dependent variable (Table 4). Age cohort analysis (RQ1d) showed that the statistically significant results were obtained for age cohorts 80–84 and 85–89, both being less likely to use online banking etc. than those being 55–59. At the same time, respondents belonging to the 50–54 age cohort are more likely to use online banking etc. than the 55–59 age cohort. The model brought no statistically significant results for sex (RQ2a) and access to supermarkets (RQ2d), with the exception for respondents with easy access to supermarkets being 52 per cent less probable to do online financial transactions than those with very easy access to supermarkets. Access to a shopping centre did provide statistically significant results showing that the respondents with the easy, quite difficult and very difficult access to a shopping centre are all far more (five or three times) likely to use online banking, paying bills, etc. than those with very easy access to a shopping centre. Widowed and single persons (RQ2b) are less likely (55 and 58%, respectively) to conduct online financial transactions than married ones (in both cases statistically significant logistic regression results were obtained).
The research provides reasons to treat older cohorts of consumers heterogeneously since it confirms the role of personal and household characteristics/circumstances (Moschis et al., Reference Moschis, Mathur and Smith1993; Yoon et al., Reference Yoon, Laurent, Fung, Gonzalez, Gutchess, Hedden, Lambert-Pandraud, Mather, Park, Peters and Skurnik2005). Our results support previous research that age influences the e-commerce activities of consumers, irrespective of the country or sector of the market (Kuoppamäki et al., Reference Kuoppamäki, Taipale and Wilska2017; Van Droogenbroeck and Van Hove, Reference Van Droogenbroeck and Van Hove2017). Marital status analysis provided statistically significant results in all three analysed e-commerce-related aspects. It can be explicitly stated that in comparison with married people, those being widowed, single or divorced are less likely to use email, shop online and search for information about goods and services via the internet (RQ2b). Sex, though, does not change the likelihood of occurrence of any of the analysed activities (RQ2a).
Conclusions and practical implications
Age associations and defining what age is old
An important confirmatory conclusion from the study is that age affects e-commerce behaviour (e.g. Sourbati, Reference Sourbati2009). Whilst many might say this was known, there is little detailed evidence of this in prior research. Answering the first research question, we suggest a breaking/turning point at the age of 75 years. Zniva and Weitzl (Reference Zniva and Weitzl2016) showed that the majority of publications defined age as old at a critical value of 55 years and older or 65 years and older. Chronological age is not as good a predictor of technology use as may have been thought, and is likely to be both dynamic and variable. HAGIS data provide reasons to claim that the boundary in terms of e-commerce-related aspects is rather later at 75 and older. This corresponds with a recognition that behaviour is different than it was a decade or two ago. Generalising, people aged 75+ nowadays are more active than those of the same age ten years ago (Mortimer and Shanahan, Reference Mortimer and Shanahan2007; George and Ferraro, Reference George and Ferraro2015), have potentially had more exposure to technology, and will certainly have greater access to those with technology expertise and use. Assumptions among (business) practitioners that the breaking point is when people are 60 or 65 and/or begin retirement can be misleading. Growing e-commerce usage and popularity is a relatively new phenomenon, so consumers aged 75 and older were not familiar with it during their professional career, in contrast with younger (i.e. below 75) cohorts. Such explanations could lead to a further age increase in this breaking point (which would happen later and later in human life) as technology becomes more and more embedded in society and economy. This is important for the delivery of services and for business activities focused on older individuals and consumers.
Individual and household (area) characteristics/circumstances and e-commerce
In terms of personal characteristics and circumstances, sex did not provide any statistically significant results, i.e. e-commerce use was not sex-specific. From a business perspective, sex differentiation among target groups can be connected exclusively with the offer specificity and character, instead of the form of communication (online versus offline).
Marital status, however, did produce statistically significant results, with married respondents more active/engaged online. This needs further investigation, but does potentially suggest an issue about isolation and social inclusion for those single, widowed or divorced, and possibly for those moving into divorced or widowed stages at later life. There are likely to be multiple issues arising at this point, including digital access.
Whilst supermarket access does not affect the probability of online searching for information, shopping centre access does provide statistically significant results. Supermarkets may be perceived as places where obtaining information is not encouraged or required (or the setting is more known or regular) while shopping centres play a role of spaces where wider interactions happen, so obtaining information is stimulated (e.g. cinemas in shopping centres). The product mix and shopping mission will also play a role. It might also be a consequence of the habits of the consumers (when they are used to online shopping they do it, irrespective of the supermarket location). Further investigation would be needed to verify this.
Business and marketing implications
Our findings highlight the general importance of exploring e-commerce and ageing. Our results correspond with the literature showing that age matters for retailing and marketing activities (e.g. Gentry and Mittelstaedt, Reference Gentry and Mittelstaedt2017). We contribute new findings to this by emphasising a behavioural breaking point at 75 years old (and not earlier as others often assume). This has significant business and marketing implications. Academics have assumed ‘older consumers’ can be as ‘young’ as 55; practitioners have sometimes tended to ignore markets beyond this age. We find that these older age cohorts are technology enabled and have demands that marketers and businesses need to accommodate. Older consumers should be a significant part of the e-commerce target group, contrary to the common marketing practice which focuses on young consumers and underestimates older ones (Nielson and Curry, Reference Nielson and Curry1997; Tréguer, Reference Tréguer2002).
We also provide evidence to support the debate about the heterogeneous nature of the older consumers group. Analysis of wider aspects, including personal and household characteristics, shows that such criteria influence the e-commerce behaviour of the older consumers. We present results suggesting that neither sex nor access to a supermarket are decisive factors in the context of the e-commerce behaviour of older cohorts. These findings need to be considered by marketing, business and e-commerce practitioners, and they also hold implications for other service providers to older individuals.
Recent global challenges have added significance to these findings. The COVID-19 pandemic has had a significant impact on older people, most obviously in terms of mortality. The process of ‘lockdown’ has constrained ‘normal’ activities and emphasised the role of technology. The penetration of the internet on UK retail sales rose during lockdown from 20 to over 33 per cent. During lockdown, if you were unable to use technology or access the internet you suffered adverse consequences. This is true in our context of internet shopping, but also in other contexts, e.g. the online video delivery of doctor's consultations or even staying in contact with family. Understanding the age-related – and personal- and household-related – capabilities and access to e-commerce is thus a precursor to enhanced interventions, both in the e-commerce sphere but also more widely.
Limitations and future research
The main focus of the study was ageing in Scotland (via the HAGIS project). This raises issues about international comparability and generalisation. We also had to accept the pre-defined data from the HAGIS questionnaires. This has limited our scope to both generate and investigate research questions and hypotheses. We recognise this as a limitation but believe the data do have significance. Similarly, we were constrained in our age coverage of respondents. Younger respondents (below 50) are not represented; comparisons between totally different age groups (very young e.g. 18–22 versus mature e.g. 50–54 and the oldest consumers e.g. 90 and over), following e.g. Chakraborty et al. (Reference Chakraborty, Lee, Bagchi-Sen, Upadhyaya and Raghav Rao2016), was not possible here. Pilot wave characteristics resulted in limitations concerning the extreme age cohort (90 and over) connected with difficulties of reach and reliability. There is also an issue over the degree of access to the internet implied by the questionnaires. The questionnaires do not focus on access, but use. We have to assume that those who did answer the use question did have access (either directly or via support).
Further study will be enabled by the longitudinal approach of HAGIS. There is also potential for cross-country analysis within the UK (e.g. the English Longitudinal Study of Ageing versus HAGIS results). Research on the dynamics of the ageing process and reduction of the ‘digital divide’ in the field of e-commerce remains a research and business task for the future. Research could also be directed into more in-depth analysis of the individual circumstances among the age cohorts to provide a more holistic investigation, e.g. around the dimensions of isolation. Secondly, qualitative study could help verify our expected explanations and stimulate further discussion about technology adoption and usage by, and support for, older consumers.
Acknowledgements
The authors would like to acknowledge Dr Elaine Douglas and Prof. David Bell of the University of Stirling for their original work on the HAGIS survey design and data collection, and for suggesting we analyse the data generated in the area of old age and e-commerce.
Ethical standards
Ethical approval of the overall HAGIS study was given by the University of Stirling Ethics Panel. Additionally, approval for access to administrative data (Administrative Data Research Network - ADRN); and scrutiny of proportionate information governance (Public Benefits and Privacy Panel – PBPP) were obtained to approve the requirement to (1) screen the drawn household sample for eligibility against NHSCR records and (2) to link anonymised HAGIS survey responses to administrative data, where participant consent is given. As we carried out secondary data analysis for this paper, no further ethics approval was required.