Nomenclature
- GBT
-
Gradient-Boosted Trees
- CMAPSS
-
Commercial Modular Aero-Propulsion System Simulation
- CV
-
Cross-Validation
- DdM
-
Data-Driven Model
- DT
-
Decision Tree
- HI
-
Health Index
- GBR
-
Gradient Boosting Regressor
- LightGBM
-
Light Gradient Boosting Machine
- LSTM
-
Long Short-Term Memory
- MAE
-
Mean Absolute Error
- ML
-
Machine Learning
- MPD
-
Maintenance Planning Document
- MRO
-
Maintenance, Repair and Overhaul
- MSE
-
Mean Squared Error
- PdM
-
Predictive Maintenance
- PHM
-
Prognostics and Health Management
- RF
-
Random Forest
- RMSE
-
Root Mean Square Error
- RUL
-
Remaining Useful Life
- SVM
-
Support Vector Machine
- SVR
-
Support Vector Regression
1. Introduction
Leadership within airline organizations grapple with an array of optimization dilemmas within the fiercely competitive aviation sector. Among these complexities lies the maintenance regimen, a critical determinant of an airline’s financial performance [Reference Baohui, Chunhui and Yaohua4]. Aircraft maintenance encompasses multifaceted considerations for optimization, encompassing the provision of maintenance equipment, aviation materials, and the management of human resources. The harmonization of these elements is essential to ensuring the provision of cost-effective and secure transportation services by airlines.
The ongoing discourse regarding the interplay between profitability and safety within the aviation industry has been a subject of enduring deliberation spanning numerous years. This dialogue transcends the purview of airline executives, extending to encompass regulatory authorities tasked with safeguarding the integrity of airline operations and ensuring enduring safety standards in air transportation [Reference Karaburun, Arık Hatipoğlu and Konar10]. In tandem with financial analysts, governmental regulators exhibit a pronounced interest in elucidating the intricate dynamics between an airline’s fiscal viability and its safety protocols [Reference Noronha and Singal17]. The optimization of this delicate balance between profitability and safety necessitates the meticulous execution of maintenance plans within the realm of aviation maintenance, repair and overhaul (MRO).
The majority of airlines rely on technical documentation such as Maintenance Planning Documents (MPDs) to ensure the continued airworthiness of their aircraft fleet, typically disseminated by aircraft manufacturers [Reference Maulana, Starr and Ompusunggu16]. Nonetheless, the statistical methodologies predominantly employed often base their calculations on population or fleet-wide data. An inherent limitation of this method lies in its susceptibility to uncertainty, thereby permitting the occurrence of unforeseen component failures before their projected maintenance intervals, as depicted in Fig. 1. In the aviation industry, fleet-wide data analysis is routinely used to assess the remaining useful life of components. By analyzing large datasets collected from multiple aircraft, maintenance teams can identify patterns and trends that help predict when a component is likely to fail. This approach allows for maximizing the use of serviceable life while minimizing the risk of unexpected failures.
Statistical reliability is based on the collection and analysis of failure, removal, and repair rates of systems or components, often referred to as “events.” Event rates are typically normalized per 1,000 flight hours or 100 flight cycles to facilitate analysis. However, it is crucial to ensure the accuracy of the input data, as incorrect data can lead to misleading results. As described in the reference text, improper use of statistical methods, such as including non-representative data points, can result in erroneous conclusions [Reference Kinnison and Siddiqui12].
For instance, an airline may incorrectly include months without data collection in their statistical analysis, leading to an invalid mean failure rate and alert level. Proper statistical analysis should only consider valid data points, and historical reliability data can be useful when statistical reliability is not applicable due to insufficient data points. This uncertainty is exacerbated by variations in operational conditions and individual component performance, which are not always accurately reflected in fleet-wide data. Conversely, there exists a pronounced risk of premature component replacement, whereby serviceable parts are removed preemptively prior to their actual failure, thus resulting in an opportunity cost incurred due to the under-utilization of the component’s remaining serviceable lifespan in Fig. 2. The premature replacement of components can lead to increased maintenance costs and unnecessary waste of resources, further straining airline budgets. By adopting more precise predictive maintenance techniques that account for individual component conditions and real-time data, airlines can mitigate these risks and optimize their maintenance schedules, ultimately improving profitability.

Figure 1. Traditional maintenance.

Figure 2. Predictive maintenance.
Numerous established maintenance methodologies encompass reactive maintenance, preventive maintenance, and predictive maintenance. Reactive maintenance involves undertaking maintenance interventions solely in response to system failures. Conversely, preventive maintenance involves both the scheduled maintenance inspections and the preemptive replacement of system components prior to the onset of any discernible failure. While preventive maintenance aids in sustaining operational continuity, its cost-effectiveness may be questioned due to the requirement for replacing potentially costly components and allocating human resources or time resources before any fault manifestation [Reference Chao, Kulkarni, Goebel and Fink7].
In the realm of commercial enterprises, the meticulous balance between operational risk and expenditure is paramount to ensuring the enduring provision of services to clientele. Predictive Maintenance (PdM) emerges as a promising solution, affording firms the capacity to foresee equipment malfunctions and schedule maintenance activities judiciously. By integrating predictive maintenance methodologies, enterprises managing complex systems can tactically orchestrate maintenance endeavors, thereby mitigating the incidence of unplanned downtime and enhancing the efficiency of component utilization. Consequently, this strategic approach facilitates savings in time, labor, financial resources, and facilitates a reduction in carbon emissions [Reference Zelaya28].
While there are multiple backup systems for communication or radar onboard to ensure airworthiness, having a backup for the aircraft engine is not feasible. The engine represents a crucial component of the aircraft that must be maintained to ensure airworthiness and safety. In this paper, we deal with mainly Machine Learning (ML) algorithms, and a similarity-based approach was used for predicting the Remaining Useful Life (RUL) of the turbofan engine. A performance comparison of ML algorithms is also carried out.
1.1 Related work
Over the last decade, there has been a proliferation of scholarly literature investigating the prediction of RUL for aircraft engines. The primary objective of these studies is to achieve precise estimations of RUL, aiming to mitigate maintenance expenditures while preserving operational reliability. This objective is realized through the formulation of RUL prediction models, which leverage the analysis of degradation patterns gleaned from various condition monitoring sensors. Such models play a pivotal role in devising tailored maintenance strategies, thereby reducing instances of unplanned downtime and enhancing the longevity of machinery, particularly in safety-critical contexts.
The expeditious detection of anomalies and timely provision of warnings regarding potential failures constitute essential measures for optimizing system utilization. In the estimation of RUL, three principal categories of prognostic methodologies are commonly employed: physical model-based approaches, data-driven approaches, and hybrid approaches [Reference An, Kim and Choi2]. These methodologies assume critical significance in the diligent monitoring of the health and operational efficacy of aircraft engines.
The model-based approach involves a comprehensive understanding of the mechanical structure of the machinery, utilizing mathematical equations to articulate and predict sub-sequent behaviors. However, addressing complex systems necessitates the formulation of numerous assumptions to facilitate the solution of these equations [Reference Bektas5]. Consequently, predictive accuracy often suffers due to the inherent assumptions inherent in grappling with intricate problems.
In contrast, the data-driven approach offers a diverse array of statistical and ML techniques tailored to address the designated issue [Reference Bektas, Marshall and Jones6]. These methodologies endeavor to uncover correlations between input variables and target outcomes, thereby simplifying the prediction of RUL compared to the model-based approach. Unlike its model-based counterpart, the data-driven approach does not necessitate an exhaustive comprehension of the intricate mechanical architectures or operational processes of the machinery.
A significant proportion of prognostics research within the aviation domain has relied upon data sourced from the NASA repository. The most recent release of datasets pertaining to the Commercial Modular Aero-Propulsion System Simulation (CMAPSS) for aircraft engines was made publicly available in 2021 [Reference Chao, Kulkarni, Goebel and Fink7]. These updated datasets signify an advancement over their antecedents published in 2008 [Reference Saxena, Goebel, Simon and Eklund20]. While it is acknowledged that data derived from laboratory experiments and simulations may not entirely replicate real-world flight conditions [Reference Verhulst, Judt, Lawson, Chung, Al-Tayawe and Ward27], the newly released CMAPSS datasets enhance fidelity by incorporating real flight conditions and enriching the degradation model with operational history [Reference Chao, Kulkarni, Goebel and Fink7].
Several extant studies in the literature employ ML techniques to forecast the RUL for the C-MAPSS dataset. One such study conducted by Mathew et al. [Reference Mathew, Toby, Singh, Rao and Kumar15] outlines a methodology centered on supervised ML for RUL prediction in turbofan engines [Reference Mathew, Toby, Singh, Rao and Kumar15].
Singh et al. conducted an additional experiment employing gradient-boosted trees (GBT) and the stacking ensemble method. This study is significant in assessing the efficacy of hybrid prediction methodologies for the CMAPSS dataset. Rather than relying on a singular model and averaging techniques, the authors endeavored to mitigate variance and enhance predictive robustness through the proposed methodology [Reference Singh, Kumar and Dwivedi24].
Fei et al. introduced the utilization of the Light Gradient Boosting Machine (LightGBM) for RUL prediction. Their findings indicate that LightGBM demonstrates notable performance when applied to C-MAPSS data characterized by high-dimensional inputs, while also possessing interpretability. Moreover, to enhance the capture of degradation information, the authors incorporated the time window of raw data and the runtime of the turbofan engine as inputs to their proposed method following normalization. By integrating data manipulation techniques such as combining window size and normalization methods, the effectiveness of the proposed methodology was augmented [Reference Fei, Gao, Lu and Xiang9].
Xin et al.’s study assumes significance in evaluating the efficacy of tree-based methodologies within the existing literature concerning RUL prediction for the C-MAPSS dataset. While the authors discuss the performance of various models and approaches in their paper, they propose a straightforward and robust method for estimating RUL based on random forest regression. Notably, their feature selection approach stands apart from conventional methodologies found in the literature. Additionally, the utilization of Lasso regression for feature selection is elucidated for readers’ comprehension [Reference Mathew, Toby, Singh, Rao and Kumar15].
In the realm of Prognostics and Health Management (PHM) applications, sequential data, such as pressure, temperature, and time-series data, represents a prevalent format of input data. While leveraging Long Short-Term Memory (LSTM), Convolutional Neural Network (CNN), or Recurrent Neural Network (RNN) for training time-series data has yielded commendable results in existing literature, these methodologies are noted for their time-consuming nature and the associated expenses in predictive scenarios [Reference Fei, Gao, Lu and Xiang9]. Consequently, researchers have redirected their focus toward single ML methods or ensemble methods for PHM applications. This study endeavors to introduce novel approaches by employing ensemble methods for RUL prediction, in contrast to the prevalent deep learning methodologies, and aims to assess the performance of the proposed model in comparison to existing benchmarks within the literature.
1.2 Aim, objectives and main contributions
This study aims to propose a methodology and algorithm for the implementation of a condition monitoring and prognostics system for aircraft engines. By leveraging the most recent NASA CMAPSS datasets as both training and test sets, the projected RULs are verified for accuracy. These RUL estimations subsequently inform critical maintenance decisions, encompassing intervention strategies, planning, scheduling, and parts preparation, in line with current practices such as MSG-3 and future advancements like MSG-4 [Reference Orel22]. To fulfill these objectives, the paper is structured as follows:
-
• Examination of the current state-of-the-art in prognostics technology.
-
• Development of condition monitoring and prognostics algorithms aimed at optimizing aircraft engine maintenance.
The primary focus of this paper is to address these challenges by proposing alternative techniques that bridge the gap between research advancements and industry demands, while simultaneously maintaining a robust RUL prediction performance. Key contributions of this study include:
-
• Introducing an alternative prognostics technique to complement existing data-driven approaches utilizing the latest CMAPSS dataset, with a particular emphasis on transparency and uncertainty quantification.
-
• Conducting an in-depth analysis of CMAPSS dataset 02 and comparing prediction performance against previously published research findings.
1.3 Paper organization
This manuscript is structured into five main sections: Introduction, methodology, results, discussion, and conclusion and future work. The introductory segment serves to expound upon the rationale behind the research and contextualizes it within the existing literature, delineating the aims, objectives, and prognostic methodologies pertinent to aircraft maintenance. The Methodology section, constituting the second component of this paper, provides a comprehensive discussion encompassing dataset description, preprocessing techniques employed, as well as elucidation of the training, prediction, and evaluation processes. The subsequent section delves into the presentation of outcomes and findings, entailing a meticulous examination and comparative analysis versus findings reported in antecedent studies. Lastly, the concluding section encapsulates the primary findings, synthesizes key insights, and proposes potential avenues for future research enhancement.
2. Methodology
2.1 Overview
The methodology employed in this study is structured into nine distinct phases, each delineating key tasks and corresponding outputs. The overarching research methodology is visually depicted in Fig. 3, elucidating the sequential progression of each process, which is subsequently expounded upon in the ensuing sections. It is noteworthy that the algorithms are developed utilizing Python Jupyter Notebook on Google Colab, leveraging the latest packages for Data Acquisition, Feature Selection, Engine Prognostics, Parameter Tuning, and Performance Tests.
2.2 Dataset
Initially introduced as the PHM Challenge by Saxena and Goebel in 2008, the CMAPSS dataset has emerged as a widely recognized resource within the field of prognostics [Reference Saxena, Goebel, Simon and Eklund20,21]. This dataset, as described by Saxena et al., provides simulated run-to-failure trajectories of a fleet comprising large turbofan engines, thereby serving as a valuable tool for research and development endeavors in prognostics and health management [Reference Saxena, Goebel, Simon and Eklund20]. In this study, the FD002 train and test sub-datasets from the CMAPSS dataset were utilized for the purpose of training ML models and predicting RUL for test data. Specifically, the train FD002 sub-dataset comprises 260 engines and 53,759 trajectories, while the test FD002 sub-dataset encompasses 259 engines and 33,991 trajectories. As seen in Table 1 the train FD002 sub-dataset comprises 260 engines and 53,759 trajectories, while the test FD002 sub-dataset encompasses 259 engines and 33,991 trajectories.
Table 1. FD002 sub-dataset


Figure 3. Research methodology overview.
2.3 Preprocessing data
Preprocessing constitutes a fundamental phase in the realm of data science. Raw data typically arrive in an unrefined state, necessitating exploration and potential cleansing or manipulation to optimize model training. Additionally, selecting an appropriate model structure is imperative to derive meaningful outcomes post-training. This section delves into the methodologies encompassing the preparation of raw sensor data leading up to model training, elucidating the sequential processes involved therein.
2.4 Z-score normalization
Normalization constitutes a fundamental preprocessing stage pivotal for both scaling and mapping, thereby enhancing the accuracy of prediction and forecasting [Reference Patro and Sahu18]. This technique involves the transformation of data from its original range to a new one, thereby mitigating the impact of significant variations among diverse prediction and forecasting methods [Reference Fei, Gao, Lu and Xiang9]. In the domain of predictive analytics, characterized by a multitude of approaches, normalization serves as an integrative mechanism, fostering cohesion among disparate models and facilitating a more comprehensive analysis of the data. Its importance lies in its capacity to establish a standardized framework, ensuring that predictions and forecasts maintain consistency and comparability across various methodologies.
Z-score normalization, also known as standardization, represents a statistical and ML method employed for scaling and normalizing features within a dataset. The primary objective of Z-score normalization is to alter the distribution of data such that it possesses a mean of 0 and a standard deviation of 1. This process of standardization guarantees that each feature is transformed to a uniform scale, thereby streamlining analysis and comparison. Such standardization proves particularly advantageous when dataset features exhibit disparities in units or scales. Overall, standardization assumes a pivotal role in rendering data into a consistent scale, thereby facilitating more meaningful interpretations and evaluations across different features.
The research applied z-score normalization to the sensor values within the FD002 dataset for the purposes of model training and data observation. This normalization procedure was undertaken to facilitate the analysis of sensor data, thereby aiding in the identification of pertinent sensors. Figure 4 illustrates the normalized raw sensor data for trajectories, providing a representative sample for observation. Through this normalization process, it becomes feasible to discern trends within the sensor data. Notably, as depicted in Fig. 4, sensors exhibiting irregular trends are deemed unsuitable for model training to prevent any adverse impact on model performance.

Figure 4. Normalized sensor data.
Monotonicity characterizes the underlying positive or negative trend of a parameter, an essential feature for prognostic parameters. Generally, it is assumed that systems do not undergo self-healing, which would be indicated by a non-monotonic parameter. This assumption holds for mechanical components or systems combining electronic and mechanical components. However, this assumption does not apply to some components, such as batteries, which may experience self-repair during short periods of nonuse [Reference Coble and Hines8].
We employed this assumption to select sensors that create more consistent degeneration patterns compared to those with erratic behaviors. Specifically, sensors identified as sensor 10, sensor 12, sensor 13, sensor 14, sensor 17, and sensor 18 are excluded from model training due to their erratic behavior. It is pertinent to note that empty sensor values were disregarded in the determination of excluded sensors. Their erratic behavior could undermine the model’s reliability and accuracy. Specifically, their non-monotonic nature introduces noise, masking the true underlying trends necessary for accurate prognostics.
2.5 Feature extraction and selection
Feature extraction and selection play a crucial role in enhancing the effectiveness of model training. Given the limited range of normalized sensor data, the trends in sensor data were examined using prognostic indicators. As illustrated in Fig. 4, the normalized data revealed that sensors with valuable information could be identified based on monotonicity and trendability behaviors, categorizing them as either useful or useless sensor data.
Following a visual inspection of the sensor data indicators in Fig. 4, sensors 6, 7, 8, 11, 15, 16, 19, 21, 24, and 25 were identified as useful sensors due to their demonstrated trendability. However, sensors 11, 16, 24, and 25 require transformation as they exhibit negative directional trends.
2.6 Data transform
Sensors exhibiting a negative trend direction were rectified to align with positive trends through a reversal process. This entailed subtracting each value within the sensor data from the maximum value present in the respective column, augmented by one. This reversal procedure aims to harmonize the data with the underlying assumptions or anticipations of the ML model, thereby fostering the generation of more interpretable results. As seen in Fig. 5, sensors 11, 16, 24 and 25 directions were reversed.

Figure 5. Useful sensors.
2.7 Health index creation
The multi-dimensional sensor readings, as well as the features extracted from the raw sensor data, are first fused to produce a single Health Indicator (HI). This process, known as performance assessment, starts with sensor selection based on observations in each operating regime [Reference Wang, Yu, Siegel and Lee30].
A few sensors have single or multiple discrete values, from which it is difficult to trace system degradation. Most sensors with continuous values exhibit a monotonic trend during the units’ lifetime. However, some sensors show inconsistent end-of-life trends among different units in the training dataset, as shown in Fig. 4. This inconsistency might indicate different failure modes of the system.
It might be possible to classify the units by failure modes based on these sensors and then use different prediction models. However, this approach encounters two challenges. First, the end-of-life readings of these sensors spread over a large range, making it hard to quantify the failure modes without extra information. Second, the failure modes might not be clearly identifiable, if at all, in the early stages of a unit’s life, contributing little to RUL estimation when only early history is available.
Therefore, only those continuous-value sensors with a consistent trend, as shown in Fig. 4, are selected for HI and RUL prediction. Some sensors do not show a clear trend due to high noise or low sensitivity to degradation. Including these sensors in the analysis may reduce the accuracy of the prediction.
In this section, health index (HI) curves were constructed for individual engines, utilizing clustered sensor data normalized and reversed in trend, categorized by engine number. The HI for each engine was derived through the averaging of pertinent preprocessed sensor data. Figure 6 depicts the HI curve for engine 1.

Figure 6. Health index creation.
2.8 Power law fitting
As illustrated in Fig. 6, the presence of noise within the HI data remains apparent, potentially undermining the efficacy of ML model training. To mitigate the adverse impact of noise on model training, a second-degree power law transformation was implemented for curve fitting. Following this procedure, a one-dimensional representation of the HI was attained, facilitating the straightforward observation of engine degradation. The power law fitting operation constituted the final preprocessing step prior to model training. A sample of the fitted curve is depicted in Fig. 7, and the resulting fitted data served as the target dataset for model training.

Figure 7. Power law curve fitting applied to health index.
2.9 Classification with ML models
In this paper, we opted to utilize basic versions of various models, eschewing extensive hyperparameter optimization typically seen in the literature. Specifically, only the K-fold cross-validation method features adjustable parameters, namely n-split and random-state, which are specified for clarity to aid readers. Rather than solely focusing on hyperparameter tuning to bolster prediction accuracy, we supplemented our basic models with similarity-based approaches to augment predictive efficacy. Consequently, we selected seven models commonly employed in regression tasks. Furthermore, Moreover, we employed k-fold cross-validation for the random forest regressor model, as it exhibited superior performance compared to other models.
The dataset splitting process is integral in the development of ML models. In this context, the provided code snippet illustrates the utilization of the train-test-split function. The dataset, comprised of useful sensors (x) and HI data (y), is partitioned into training and testing subsets. Notably, the test-size parameter is set to 0.3, signifying that 30% of the data is earmarked for testing, while the remaining 70% is allocated for training the model. To ensure reproducibility, the random-state parameter is fixed at 0, thereby maintaining consistency across different executions. Given that we are working with time series data and regression analysis, maintaining the temporal order of data is crucial for the validity of our models. Additionally, the shuffle parameter is deliberately set to False, indicating that the data points will not be randomly rearranged prior to partitioning. This controlled division facilitates robust evaluation of the model’s performance on unseen data during testing, while upholding a stable training environment. Furthermore, the regimes already come from different domains and are inherently mixed, reducing the necessity for further shuffling.
2.10 Linear regression
Linear regression is a statistical technique utilized to ascertain the association between a dependent variable and one or more independent variables [Reference Marill14]. This relationship is expressed by Equation (1), where the dependent variable, denoted as y, is a function of an independent variable, x, and a coefficient b representing the slope characterizing the linear dependence between x and y, along with the constant
$\varepsilon$
. In our context, x pertains to the raw data extracted from the engine’s useful sensor readings, whereas y signifies the value obtained through a second-degree power law fitting.

Figure 8 displays the training prediction performance for the split test data from train data engine values. The MSE of the training set is 0.07 for the linear regression model.

Figure 8. True and predicted values for the linear regression model.
2.11 Support vector regression
Support Vector Machine (SVM) for regression, often denoted as Support Vector Regression (SVR), is a statistical approach aimed at establishing relationships between a dependent variable and independent variables [Reference Kavitha, Varuna and Ramya11]. One notable distinction of SVR lies in its utilization of support vectors, which endeavor to encompass as many training points as feasible, thereby creating an optimized margin. SVMs delineate this optimal hyperplane with support vectors. The sparse solution and superior generalization capabilities of SVMs render them well-suited for adaptation to regression tasks [Reference Awad and Khanna3]. Following the training of the SVM model, predictions for new dependent values are generated based on optimized metrics established during the training phase. SVM models demonstrate proficiency in addressing problems characterized by noisy data. Figure 9 displays the training prediction performance for the split test data from train data engine values. The MSE of the training set is 0.40 for the support vector regression model.

Figure 9. True and predicted values for the support vector regression model.
2.12 Decision tree regressor
A Decision Tree (DT) serves as a statistical method employed to delineate relationships between dependent and independent variables. In contrast to SVM, which endeavor to ascertain a hyperplane for classification tasks, decision trees adopt a hierarchical tree structure. In the context of regression analysis, these structures are denoted as regression trees (Freund and Mason). The dataset undergoes recursive partitioning by the decision tree, utilizing threshold conditions optimized to maximize information gain for classification tasks or minimize variance for regression analysis. The final outcome is determined at the terminal nodes of the tree hierarchy.
Figure 10 showcases the training prediction performance for the test data split from the training dataset’s engine values. MSE observed for the training set amounts to 0.07 for the decision tree regressor model.

Figure 10. True and predicted values for the decision tree regression model.
2.13 Random forest regressor
Random Forest (RF) represents an ensemble learning technique renowned for its efficacy in both classification and regression endeavors. Introduced by Leo Breiman in 2001 [Reference Segal23], RF stands out for its remarkable performance in minimizing prediction errors across benchmark datasets.
Functioning as a tree-based method, RFs share similarities with DT models, yet their principal divergence lies in their ensemble nature. The RF model partitions the dataset into subsets through random sampling with replacement and constructs individual decision tree hierarchies for each subset. During the training phase, these hierarchical structures are crafted under optimized conditions.
For regression tasks, the RF model aggregates the outputs of the individual decision trees to derive the final prediction. This ensemble strategy contributes to enhanced predictive accuracy and serves as a preventive measure against overfitting. Upon encountering new data points, predictions are generated by leveraging the hierarchical structure of the trained RF model.
Figure 11 illustrates the training prediction performance for the test data partitioned from the training dataset’s engine values. The MSE observed for the training set amounts to 0.03 for the RF regressor model.

Figure 11. True and predicted values for the random forest regression model.
Functioning as a tree-based method, RFs share similarities with DT models, yet their principal divergence lies in their ensemble nature. The RF model partitions the dataset into subsets through random sampling with replacement and constructs individual decision tree hierarchies for each subset. During the training phase, these hierarchical structures are crafted under optimized conditions.
For regression tasks, the RF model aggregates the outputs of the individual decision trees to derive the final prediction. This ensemble strategy contributes to enhanced predictive accuracy and serves as a preventive measure against overfitting. Upon encountering new data points, predictions are generated by leveraging the hierarchical structure of the trained RF model. Figure 11 illustrates the training prediction performance for the test data partitioned from the training dataset’s engine values. The MSE observed for the training set amounts to 0.03 for the RF regressor model.
2.14 Random forest regressor with K-fold cross validation
The random forest regressor exhibited superior performance during the training phase, prompting our endeavor to refine it further through hyperparameter tuning. Given the sensitivity of ML models to such adjustments and their consequential impact on predictive efficacy [Reference Al-Abdaly, Al-Taai, Imran and Ibrahim1].
In this study, we elected to employ k-fold cross-validation (CV), a widely embraced technique for model validation, to ensure optimal data partitioning during the training process. K-fold cross-validation stands as one of the most prevalent forms of cross-validation in ML. While there exists no rigid guideline for determining the value of k, a commonly adopted standard in practical ML is k = 10 (or 5). Employing either five or ten folds yields a more accurate estimation with reduced bias [Reference Rodriguez, Perez and Lozano19]. Consequently, we set the value of k to 5 in our experiment during the training of the RF model.
Figure 12 illustrates the training prediction performance of the RF model integrated with k-fold cross-validation. The MSE observed for the training set remains at 0.03 for the RF regressor model.
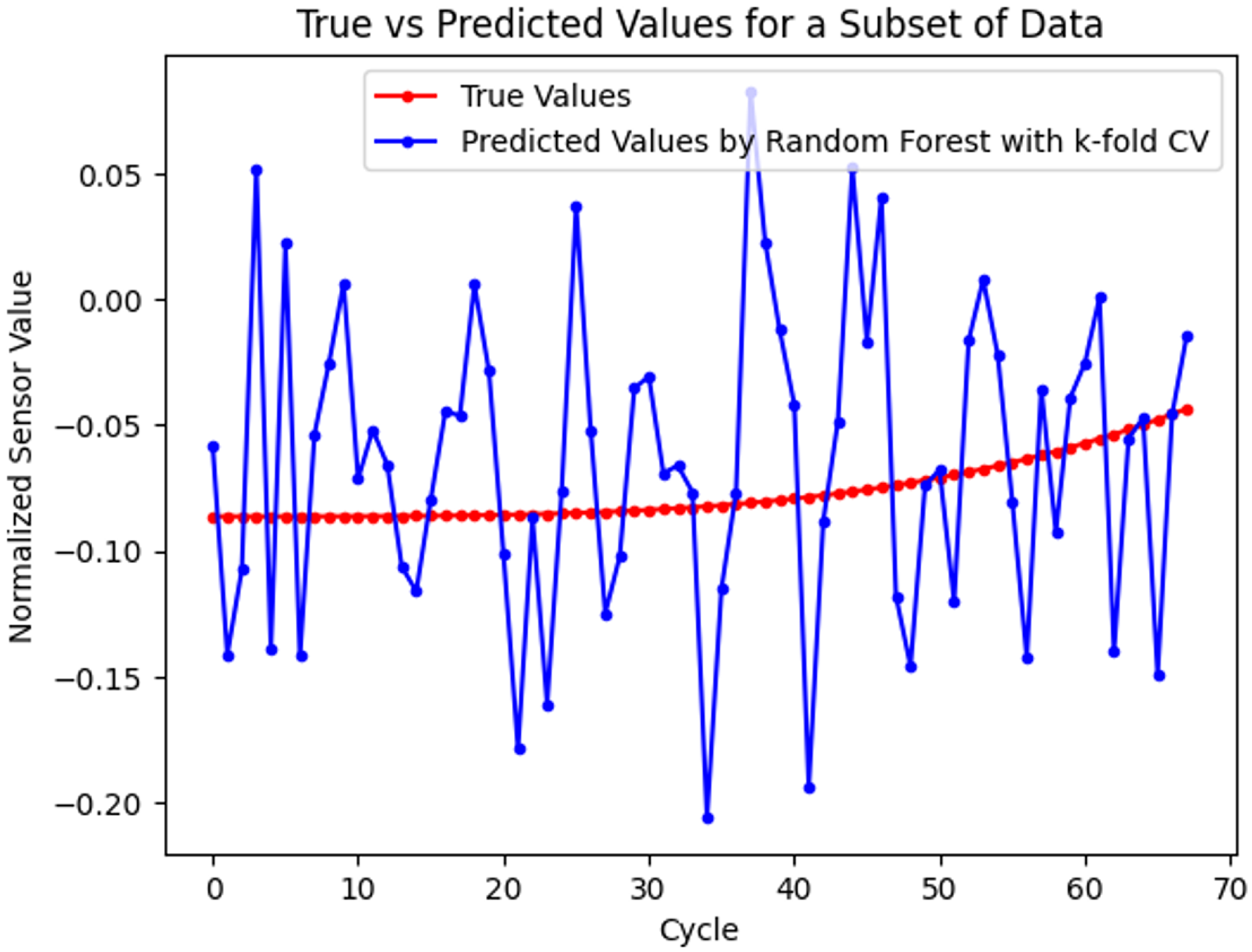
Figure 12. True and predicted values for the random forest regression with K-fold cross validation.
2.15 Gradient boosting regressor
The Gradient Boosting Regressor (GBR) serves as another statistical model utilized for delineating relationships between dependent and independent variables. Its notable significance lies in its capacity to learn from weak learners. The GBR algorithm initiates prediction at the median of the dataset for each observation, and subsequently computes residuals by determining the differences between the target and the initial prediction. As the process iterates through multiple leaves, each subsequent leaf endeavors to capture and rectify the errors (residuals) incurred by its predecessors. The learning rate parameter governs the contribution of each weak learner to the overall model [Reference Zhang and Haghani29].
In this experiment, two preprocessing techniques were applied to enhance training efficiency and reduce computational expenses prior to GBR model training, in contrast to other models. Firstly, feature standardization was performed, followed by the application of truncated singular value decomposition for dimensionality reduction. Subsequently, the model was fitted.
Figure 13 illustrates the training prediction performance for the split test data derived from the training dataset’s engine values. The MSE observed for the training set stands at 0.10 for the GBR model.

Figure 13. True and predicted values for the gradient boosting model.
2.16 K-nearest neighbors regressor
K-Nearest Neighbors (KNN) represents a versatile and intuitive algorithm employed in statistical modeling to establish relationships between dependent and independent variables. At its core, KNN operates on the principle of making predictions based on the majority class or average of the k-nearest data points in the feature space. Notably, this algorithm is non-parametric, signifying that it does not rely on assumptions regarding the underlying data distribution. Instead, it leverages the proximity of data points in the feature space to make predictions.
Figure 14 illustrates the training prediction performance for the split test data derived from the training dataset’s engine values. The MSE observed for the training set amounts to 0.07 for the KNN model.

Figure 14. True and predicted values for the K-nearest neighbors regressor model.
2.17 Ensemble model
The predictions of the ensemble model were generated by averaging all the individual model predictions for the related engines. While prediction performances varied among individual predictions, the overall model prediction performance was considered. It’s important to note that no specific training step was conducted for the ensemble model, and as such, its training performance is not discussed in this section. The performance of the ensemble model will be addressed in the Results section.
2.18 Estimation
In this section, both the training and test datasets comprising useful raw sensor data were subjected to estimation. The training data underwent re-estimation to enhance the accuracy of HI calculation. Initially, HI was computed for model training purposes; however, post-model training, the models were utilized for HI re-estimation instead of manual calculation. This automated approach significantly reduced the expense associated with creating HI manually. Additionally, while we provide a smooth curve, it is important to note that such smoothness would not be achievable in a real-world scenario. The absence of noise in our estimations is intentional to avoid potential errors that could arise from numerous sources.
For our study, we utilized the NASA Commercial Modular Aero-Propulsion System Simulation (C-MAPSS) datasets for both testing and training. The raw sensor data from these datasets served as the feature input, while the fitted values were used as the target data. This choice was made to ensure that our model could accurately learn the underlying patterns in the data without being influenced by noise.
2.19 Similarity-based approach and RUL prediction
The estimated HI values for both the training and test datasets were clustered based on the engine ID, resulting in unique trajectories for each engine. Figure 15 shows the estimated HI values for the training dataset as a solid black line and the test dataset as a dashed gray line. To align the test trajectories with the training data, the test engine trajectories were overlaid on the estimated training data. As the test engine trajectories traverse the estimated training data, the Euclidean distances between the test trajectory values and the corresponding training values were measured. These distances are visually represented by the red vertical lines connecting points on the test trajectory to the closest points on the training trajectory. For each test engine, the top five closest distances were identified using this approach, employing all models. In Fig. 15, points a and a’, b and b’ are annotated, with a and b representing points on the training trajectory and a’ and b’ representing the corresponding points on the test trajectory.

Figure 15. Similarity methodology.
Concurrently, the maximum length of the trajectory was designated as the RUL for each engine in the training data. These RUL values served as reference points for predicting the RUL for the test engines. Upon identifying the best-matched training data with the test data, RUL was calculated using the following formula:

This process was repeated five times for each engine, and the average of the resultant sum values was considered as the RUL for the engine. Figure 16 illustrates the best-matched value on the training data and the RUL for test engine 1. The same method was applied to each test engine dataset.

Figure 16. Similarity-based prediction.
3. Results
The C-MAPSS dataset has 4 sub-datasets, but we applied training and evaluation for the FD002 dataset in this paper since FD002 has data in all regimes. Specifically, the training FD002 sub-dataset comprises 260 engines and 53,759 trajectories, while the test FD002 sub-dataset encompasses 259 engines and 33,991 trajectories. Seven algorithms were tested by using the test FD002 sub-dataset and applied the same similarity-based approach for each model’s outputs.
The root mean square error (RMSE) and mean absolute error (MAE) metrics were used to evaluate model performances. RMSE measures the square root of the average squared differences between predicted and actual values of RUL. On the other hand, MAE measures the average absolute differences between predicted values and actual RUL. The objective was to identify the algorithm with the lowest RMSE and MAE values. Although net RUL cannot be directly predicted, minimizing the gap between actual RUL and predicted RUL can be achieved by selecting the best-performing algorithm. Based on our experiments, the ensemble model, demonstrated the least prediction error. Algorithm’s metric scores are represented in Table 2.
In this paper, we not only compared the algorithms with each other but also assessed their performance against models presented in the existing literature. Table 3 provides a comparison of the performance of our algorithms and the relevant models from the literature.
Table 2. Algorithm’s metric scores

*Unit: operational cycles – each data capture is a snapshot of a cycle ([21]. “Turbofan Engine Degradation Simulation).
Table 3. Comparison between the results from our study and [Reference Mathew, Toby, Singh, Rao and Kumar15]

*Unit: operational cycles – each data capture is a snapshot of a cycle ([21].“Turbofan Engine Degradation Simulation).
By incorporating initial conditions, we enable a comparative analysis between our study and the referenced work by Mathew et al. [Reference Mathew, Toby, Singh, Rao and Kumar15]. In the compared experiment, the authors used RUL cycles as the target label and raw sensor data to train the model. Although this approach provides good performance for LSTM models, traditional ML models did not have sufficient ability to learn and predict effectively. Additionally, sensor selection was not evident in the related paper, which negatively impacted model training.
In contrast, our paper defines the degradation pattern of the engine and includes sensor selection to enhance model learning. By creating degradation patterns, trained models can compare these patterns with training baseline samples, allowing a similarity-based approach to be applied to our predictions. This methodology provides a better definition of degradation than models trained on raw sensor data.
Although we used common ML models, our preprocessing methods allowed us to define degradation more accurately. As we cannot predict exact RUL, applying an average of the best-matched training samples reduces error risk. These fundamental differences contribute to the superior performance of our models. This allows us to assess the efficacy of our similarity-based approach in conjunction with algorithms that consider initial conditions. Through this comparison, we can discern any enhancements achieved by our methodology over existing approaches.
4. Discussion
Different models were employed to estimate the HI for the same dataset, while a uniform similarity-based approach was utilized to predict RUL. Consequently, each model’s performance was discerned by this method on identical data. The primary objective was to minimize the discrepancy between the actual RUL and the predicted RUL. Although MAE was calculated, both our study and other authors in the literature for this dataset typically favored the RMSE metric over MAE. This preference is attributed to the aim of reducing the occurrence of larger outliers in predictions, as RMSE provides a more comprehensive evaluation of model performance. The RF model yielded the lowest RMSE among all models, with a value of 26.88, as illustrated in Table 3. Although the ensemble model shows the best results in our study, we compared the performance of common models with the study by Ref. [Reference Mathew, Toby, Singh, Rao and Kumar15]. In that comparison, the Random Forest regressor achieved the best performance. Since Mathew et al.’s study did not utilize an ensemble model, we highlighted the Random Forest as having the best score among the common models.
Different models exhibit varying strengths depending on the trajectory length of the samples. Some models perform better with long trajectories, while others excel with short trajectories. By combining these models in an ensemble, we leverage their individual strengths, leading to improved overall prediction performance. The ensemble model achieves superior performance by averaging the predictions of individual models. This approach mitigates the weaknesses of any single model and harnesses the strengths of multiple models, resulting in lower RMSE and MAE errors.
5. Conclusion and future work
Predictive maintenance holds significant appeal for companies seeking to mitigate unforeseen operational disruptions. The RUL enables proactive planning of maintenance requirements for turbofan engines. This proactive approach empowers airline operators or MRO companies to schedule maintenance activities preemptively, thereby averting potential errors and optimizing resource management, including human resources, equipment, and cash flow. By implementing predictive maintenance strategies, companies not only minimize wasted time and resources but also enhance operational security.
In our experiment, we evaluated the performance of ML algorithms augmented by a similarity-based approach. Moving forward, we aim to delve deeper into optimizing hyperparameters for ML models. Additionally, we intend to investigate prediction errors and explore strategies for enhancing the effectiveness of the similarity-based approach. For instance, we may consider manipulating engine length data to refine the application of the similarity-based approach. For instance, we could exclude the initial 50 trajectory data points if an engine has more than 150 trajectory data points, subsequently applying the similarity-based approach to the remaining 100 trajectory data points. While 150 is provided as a sample threshold value, the determination of the optimal threshold value could be informed by observing the models’ most significant errors.
Data availability statement
All data used during the study are available from the corresponding author by request.