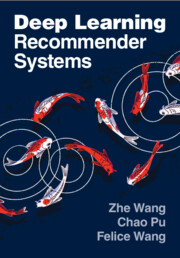
- Coming soon
- Publisher:
- Cambridge University Press
- Expected online publication date:
- May 2025
- Print publication year:
- 2025
- Online ISBN:
- 9781009447515
Recommender systems are ubiquitous in modern life and are one of the main monetization channels for Internet technology giants. This book helps graduate students, researchers and practitioners to get to grips with this cutting-edge field and build the thorough understanding and practical skills needed to progress in the area. It not only introduces the applications of deep learning and generative AI for recommendation models, but also focuses on the industry architecture of the recommender systems. The authors include a detailed discussion of the implementation solutions used by companies such as YouTube, Alibaba, Airbnb and Netflix, as well as the related machine learning framework including model serving, model training, feature storage and data stream processing.
* Views captured on Cambridge Core between #date#. This data will be updated every 24 hours.
Usage data cannot currently be displayed.