Book contents
- Frontmatter
- Contents
- Preface
- Notation Used
- Abbreviations
- 1 Introduction
- 2 Basics
- 3 Probability Distributions
- 4 Statistical Inference
- 5 Linear Regression
- 6 Neural Networks
- 7 Non-linear Optimization
- 8 Learning and Generalization
- 9 Principal Components and Canonical Correlation
- 10 Unsupervised Learning
- 11 Time Series
- 12 Classification
- 13 Kernel Methods
- 14 Decision Trees, Random Forests and Boosting
- 15 Deep Learning
- 16 Forecast Verification and Post-processing
- 17 Merging of Machine Learning and Physics
- Appendices
- References
- Index
- References
References
Published online by Cambridge University Press: 23 March 2023
- Frontmatter
- Contents
- Preface
- Notation Used
- Abbreviations
- 1 Introduction
- 2 Basics
- 3 Probability Distributions
- 4 Statistical Inference
- 5 Linear Regression
- 6 Neural Networks
- 7 Non-linear Optimization
- 8 Learning and Generalization
- 9 Principal Components and Canonical Correlation
- 10 Unsupervised Learning
- 11 Time Series
- 12 Classification
- 13 Kernel Methods
- 14 Decision Trees, Random Forests and Boosting
- 15 Deep Learning
- 16 Forecast Verification and Post-processing
- 17 Merging of Machine Learning and Physics
- Appendices
- References
- Index
- References
Summary
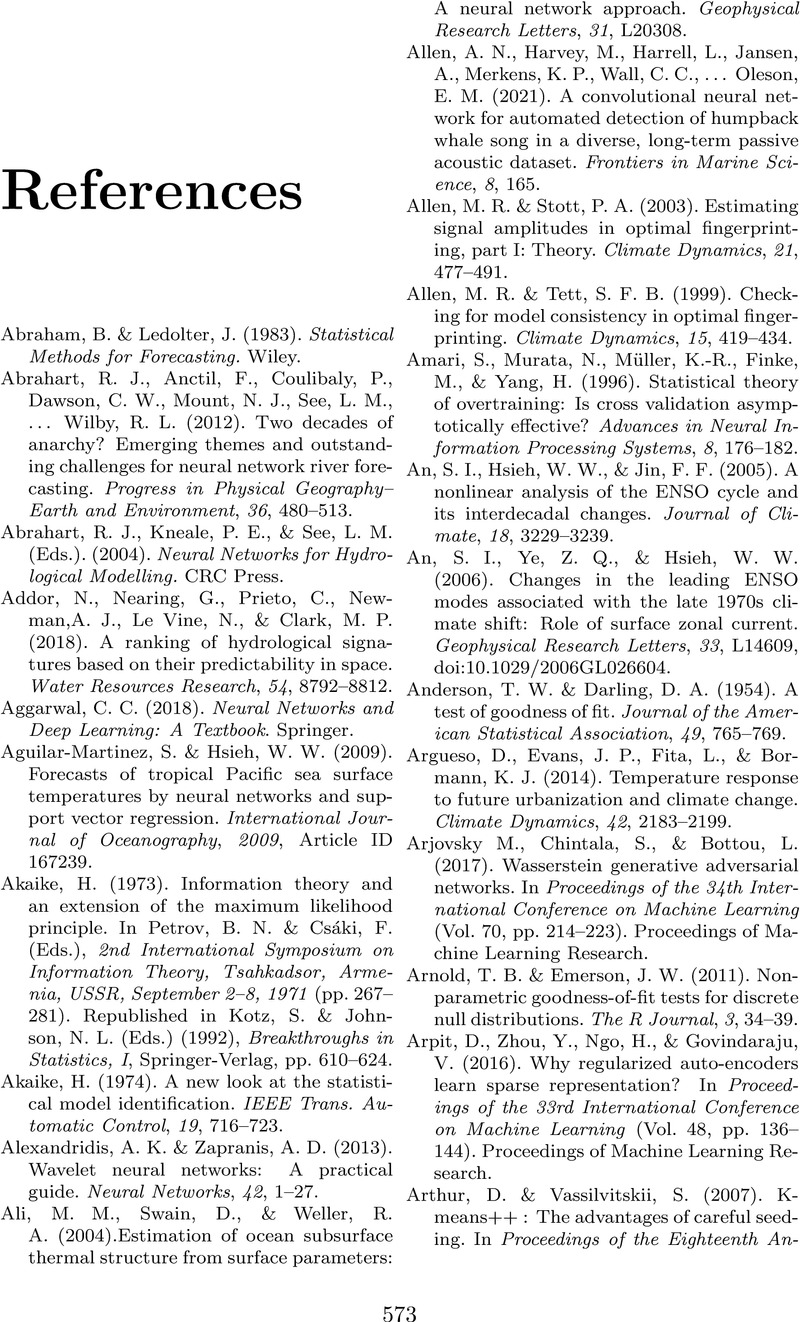
- Type
- Chapter
- Information
- Introduction to Environmental Data Science , pp. 573 - 612Publisher: Cambridge University PressPrint publication year: 2023