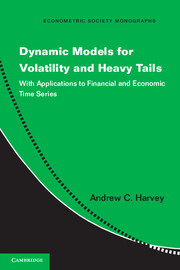
Book contents
- Frontmatter
- Contents
- Preface
- Acronyms and Abbreviations
- 1 Introduction
- 2 Statistical Distributions and Asymptotic Theory
- 3 Location
- 4 Scale
- 5 Location/Scale Models for Non-negative Variables
- 6 Dynamic Kernel Density Estimation and Time-Varying Quantiles
- 7 Multivariate Models, Correlation and Association
- 8 Conclusions and Further Directions
- A Derivation of Formulae in the Information Matrix
- B Autocorrelation Functions
- C GED Information Matrix
- D The Order of GARCH Models
- E Computer Programs
- Bibliography
- Author Index
- Subject Index
- Other titles in the series
7 - Multivariate Models, Correlation and Association
Published online by Cambridge University Press: 05 May 2013
- Frontmatter
- Contents
- Preface
- Acronyms and Abbreviations
- 1 Introduction
- 2 Statistical Distributions and Asymptotic Theory
- 3 Location
- 4 Scale
- 5 Location/Scale Models for Non-negative Variables
- 6 Dynamic Kernel Density Estimation and Time-Varying Quantiles
- 7 Multivariate Models, Correlation and Association
- 8 Conclusions and Further Directions
- A Derivation of Formulae in the Information Matrix
- B Autocorrelation Functions
- C GED Information Matrix
- D The Order of GARCH Models
- E Computer Programs
- Bibliography
- Author Index
- Subject Index
- Other titles in the series
Summary
Dynamic conditional score models extend quite naturally to deal with changing location and scale in multivariate time series. Although a full treatment requires extensive use of matrix algebra, the main practical issues concern the nature and scope of restrictions on the parameter matrices governing the dynamics. The asymptotic theory of ML estimation is straightforward in principle, though the details are not always easy to work out.
The main challenge lies in constructing and analyzing models that embody changing relationships between variables. In this case, the asymptotic theory is no longer straightforward, even for Gaussian models. Nevertheless, even when it is not possible to develop a full asymptotic theory, the structure of the information matrix, and the conditions under which it is positive definite, provides a good indication of how well different models and parameterizations might work in practice.
There are important reasons for wishing to use multivariate models in finance. Understanding and measuring the relationship between movements in different assets play a key role in designing a portfolio. The standard theory is based on the multivariate normal distribution, and it shows how to construct a portfolio that gives minimum variance for a given expected return. Unfortunately, the multivariate normal distribution is not usually suitable for this task for two reasons: asset returns are not normally distributed, and their comovements are not adequately captured by correlation coefficients. More specifically, heavy tails are a feature of marginal distributions, and the probab-ility of two asset prices exhibiting big movements in the same direction may be much higher than it would be with a bivariate normal.
- Type
- Chapter
- Information
- Dynamic Models for Volatility and Heavy TailsWith Applications to Financial and Economic Time Series, pp. 204 - 230Publisher: Cambridge University PressPrint publication year: 2013