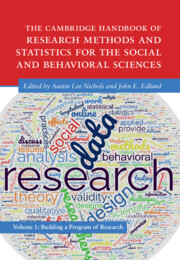
Book contents
- The Cambridge Handbook of Research Methods and Statistics for the Social and Behavioral Sciences
- Cambridge Handbooks in Psychology
- The Cambridge Handbook of Research Methods and Statistics for the Social and Behavioral Sciences
- Copyright page
- Dedication
- Contents
- Figures
- Tables
- Contributors
- Preface
- Part I From Idea to Reality: The Basics of Research
- Part II The Building Blocks of a Study
- Part III Data Collection
- 13 Cross-Sectional Studies
- 14 Quasi-Experimental Research
- 15 Non-equivalent Control Group Pretest–Posttest Design in Social and Behavioral Research
- 16 Experimental Methods
- 17 Longitudinal Research: A World to Explore
- 18 Online Research Methods
- 19 Archival Data
- 20 Qualitative Research Design
- Part IV Statistical Approaches
- Part V Tips for a Successful Research Career
- Index
- References
19 - Archival Data
from Part III - Data Collection
Published online by Cambridge University Press: 25 May 2023
- The Cambridge Handbook of Research Methods and Statistics for the Social and Behavioral Sciences
- Cambridge Handbooks in Psychology
- The Cambridge Handbook of Research Methods and Statistics for the Social and Behavioral Sciences
- Copyright page
- Dedication
- Contents
- Figures
- Tables
- Contributors
- Preface
- Part I From Idea to Reality: The Basics of Research
- Part II The Building Blocks of a Study
- Part III Data Collection
- 13 Cross-Sectional Studies
- 14 Quasi-Experimental Research
- 15 Non-equivalent Control Group Pretest–Posttest Design in Social and Behavioral Research
- 16 Experimental Methods
- 17 Longitudinal Research: A World to Explore
- 18 Online Research Methods
- 19 Archival Data
- 20 Qualitative Research Design
- Part IV Statistical Approaches
- Part V Tips for a Successful Research Career
- Index
- References
Summary
Social and behavioral researchers often draw on archival data – data collected by an entity other than the research team – to conduct scientific inquiry. Researchers typically seek to make claims about measured variables that extend beyond the measures themselves, such as interpreting a measure as representing an unobservable theoretical construct. Though researchers using archival data encounter many issues, this chapter focuses on two that have received less attention. The first concerns how researchers should justify the interpretations and uses they attach to archival measures. The second concerns how to justify generalizing findings. This chapter provides a framework to help researchers address these issues by drawing on contemporary validity theory in education and psychology as well as theory regarding causal mechanisms from philosophy and sociology. These concepts are illustrated using multiple examples from published studies.
- Type
- Chapter
- Information
- The Cambridge Handbook of Research Methods and Statistics for the Social and Behavioral SciencesVolume 1: Building a Program of Research, pp. 400 - 419Publisher: Cambridge University PressPrint publication year: 2023
References
- 1
- Cited by