Article contents
High Resolution X-Ray CT Reconstruction of Additively Manufactured Metal Parts using Generative Adversarial Network-based Domain Adaptation in AI-CT
Published online by Cambridge University Press: 30 July 2021
Abstract
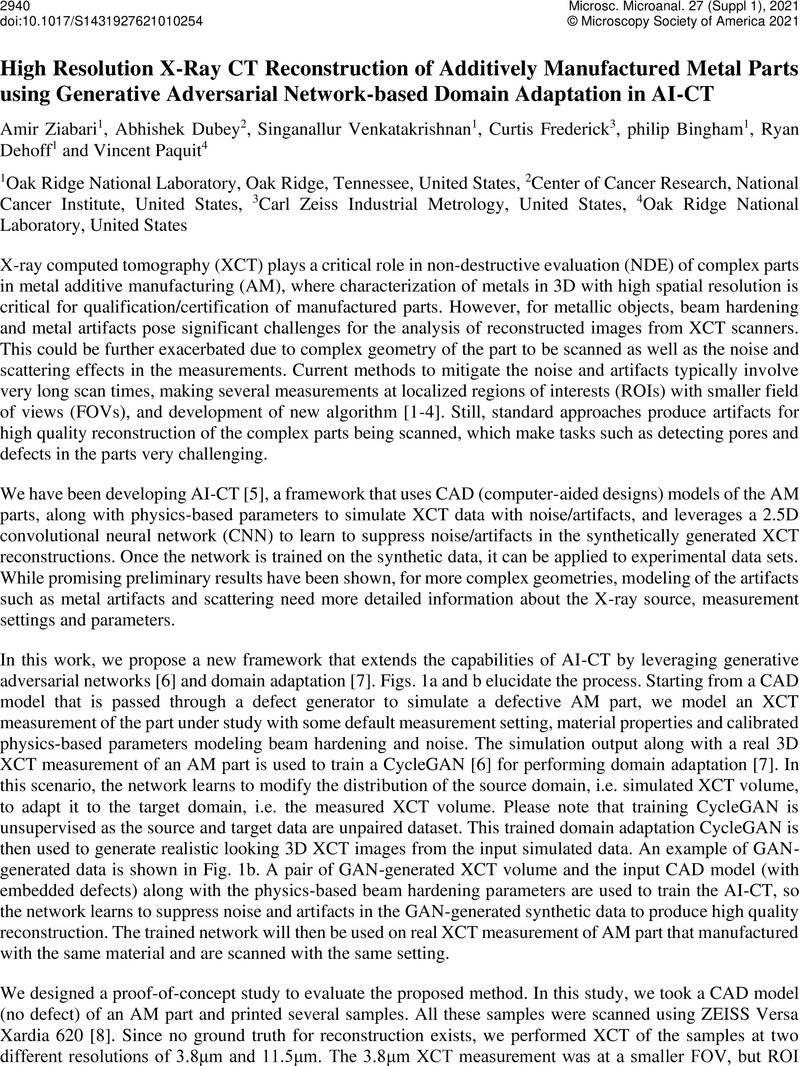
- Type
- Advanced Characterization of Components Fabricated by Additive Manufacturing
- Information
- Copyright
- Copyright © The Author(s), 2021. Published by Cambridge University Press on behalf of the Microscopy Society of America
References
- 2
- Cited by