Introduction
Reward-related brain activity undergoes critical developmental changes from childhood to adolescence. Brain regions involved in reward processing, such as the ventral striatum and prefrontal cortex, mature considerably from childhood to adulthood. Research indicates that adolescents demonstrate hyperactivation of the striatum and medial prefrontal cortex (mPFC) in response to feedback indicating reward receipt compared to children and adults (Casey et al., Reference Casey, Getz and Galvan2008a; Galvan, Reference Galvan2010). Adolescence is also a time period that coincides with the emergence and increased prevalence rates of various psychiatric disorders (Kessler et al., Reference Kessler, Berglund, Demler, Jin, Koretz, Merikangas, Rush, Walters and Wang2003). A growing body of research suggests that individual differences in reward processing are associated with the onset and maintenance of various psychiatric disorders, such as depression (Keren et al., Reference Keren, O’Callaghan, Vidal-Ribas, Buzzell, Brotman, Leibenluft, Pan, Meffert, Kaiser, Wolke, Pine and Stringaris2018; Luking et al., Reference Luking, Pagliaccio, Luby and Barch2016).
The reward positivity (RewP) is an event-related potential (ERP) component that can be measured in children as young as four (Belden et al., Reference Belden, Irvin, Hajcak, Kappenman, Kelly, Karlow, Luby and Barch2016) and indexes reward system activation in the context of reinforcing behaviors that lead to favorable outcomes (for review, see Proudfit, Reference Proudfit2015). The RewP is measured at frontocentral electrode sites and is elicited approximately 250 to 350 ms following reward feedback. The RewP has been posited as a promising early biomarker of depression risk (Proudfit, Reference Proudfit2015).
A number of studies have examined developmental changes in the RewP across childhood and adolescence, but the literature has yielded mixed results. For instance, some studies have found that older children have a larger RewP compared to younger children (Gibb et al., Reference Gibb, Tsypes, Israel and Owens2022; Hennefield et al., Reference Hennefield, Gilbert, Whalen, Giorio, Camacho, Kelly, Fleuchaus, Barch, Luby and Hajcak2022) and older adolescents have a larger RewP compared to younger adolescents (Burani, Mulligan, et al., Reference Burani, Mulligan, Klawohn, Luking, Nelson and Hajcak2019; Moser et al., Reference Moser, Fisher, Hicks, Zucker and Durbin2018). In contrast, other studies using adolescent samples have found no differences in ERP reward-related brain activity as a function of age (Bress et al., Reference Bress, Smith, Foti, Klein and Hajcak2012, Reference Bress, Meyer and Proudfit2015; Ethridge & Weinberg, Reference Ethridge and Weinberg2018; Kujawa et al., Reference Kujawa, Carroll, Mumper, Mukherjee, Kessel, Olino, Hajcak and Klein2018; Lukie et al., Reference Lukie, Montazer-Hojat and Holroyd2014). However, research examining changes in the RewP across adolescence have primarily relied upon cross-sectional, between-person analyses to examine developmental changes, and few studies have examined within-person changes in the RewP across adolescence.
Of the few studies that have used longitudinal, within-person study designs to examine age-related changes in the RewP, only one study has examined changes in the RewP across three assessments. Kujawa and colleagues (Kujawa et al., Reference Kujawa, Carroll, Mumper, Mukherjee, Kessel, Olino, Hajcak and Klein2018) examined developmental changes in the RewP in a sample of youth assessed across three assessments from late childhood to middle adolescence. Results indicated that the magnitude of the RewP did not significantly change from late childhood to late adolescence and remained stable across development, particularly during early to mid-adolescence. Conversely, Burani and colleages (Burani, Mulligan, et al., Reference Burani, Mulligan, Klawohn, Luking, Nelson and Hajcak2019) examined age-related changes in the RewP across two assessments and found that the RewP increased over the two year study period for younger, but not older, participants. Therefore, initial evidence suggests that the RewP increases from late childhood to early adolescence, but no studies have examined longitudinal, within-person changes in the RewP from late childhood to young adulthood, which is critical for characterizing the developmental trajectories of reward processing. Thus, the present study aims to extend Burani et al. (Reference Burani, Klawohn, Levinson, Klein, Nelson and Hajcak2019) and use the same sample to include a third wave of data collection, a broader developmental time window, and a more thorough investigation of within-person changes in the RewP across development.
Individual differences in neural reward responsiveness have been linked to the development of depression (Gold et al., Reference Gold, Waltz, Prentice, Morris and Heerey2008; Luking et al., Reference Luking, Pagliaccio, Luby and Barch2016; Whitton et al., Reference Whitton, Treadway and Pizzagalli2015). Cross-sectional research has indicated that a more blunted RewP is associated with increased depressive symptoms and major depressive disorder (MDD) in youth and adults (Bress et al., Reference Bress, Meyer and Proudfit2015; Liu et al., Reference Liu, Wang, Shang, Shen, Li, Cheung and Chan2014; Nelson et al., Reference Nelson, Ph, Perlman, Ph, Klein, Ph, Kotov, Ph, Hajcak and Ph2016). A more blunted RewP has also been associated with risk for depression in youth. Specifically, youth with a family history of depression have been observed to have a more blunted RewP (Kujawa et al., Reference Kujawa, Proudfit and Klein2014; Weinberg et al., Reference Weinberg, Liu, Hajcak and Shankman2015). Further, a more blunted RewP has been shown to prospectively predict the development of depression in youth (Bress et al., Reference Bress, Foti, Kotov, Klein and Hajcak2013; Foti, Kotov, et al., Reference Foti, Kotov and Klein2011; Kujawa et al., Reference Kujawa, Proudfit and Klein2014; Nelson et al., Reference Nelson, Ph, Perlman, Ph, Klein, Ph, Kotov, Ph, Hajcak and Ph2016). Collectively, research suggests that a blunted RewP might be a neurobiological marker of risk for the development of depression.
Anhedonia, the inability to experience pleasure, is a defining feature of major depressive disorder and may play an important role in the relationship between reduced reward-related brain activity and depression. For example, Liu et al. (Reference Liu, Wang, Shang, Shen, Li, Cheung and Chan2014) found that adults with depression exhibited a more blunted RewP compared to healthy controls. Further, among both healthy and depressed individuals, greater trait anhedonia symptoms were associated with a more blunted RewP. In addition to being a core feature of depression, anhedonia has also been conceptualized as an enduring trait reflecting low hedonic capacity and approach motivation (Loas, Reference Loas1996; Pelizza & Ferrari, Reference Pelizza and Ferrari2009). In a separate adult sample, Foti et al. (Reference Foti, Carlson, Sauder and Proudfit2014a) found that the RewP was more blunted among depressed individuals compared to healthy controls and that this effect was driven by a subgroup of individuals characterized by impaired mood reactivity to positive events. More recent research has found similar relationships between different facets of anhedonia and the RewP (Banica et al., Reference Banica, Schell, Racine and Weinberg2022). Therefore, there is initial evidence that anhedonia is an important feature of depression that might be linked with blunted reward-related brain activity. Although a number of studies have examined the RewP as a predictor of the development of psychopathology, evidence suggests that the relationship between the RewP and depression is bidirectional in nature (Mackin et al., Reference Mackin, Goldstein, Mumper, Kujawa, Kessel, Olino, Nelson, Hajcak and Klein2023). Few studies have examined predictors of developmental trajectories of reward-related brain activity, and it is possible that childhood characteristics, such as anhedonia, that are predictive of future emotional and behavioral outcomes might also be predictive of neural trajectories during adolescence and young adulthood.
Stress is a prominent risk factor for the development of depression (Kessler, Reference Kessler1997). There is a growing consensus that the RewP, coupled with increased stress, increases risk for the development of depression. Cross-sectional and longitudinal research indicates that greater exposure to stress and stressful life events and a more blunted RewP predict greater increases in depressive symptoms among adolescents and young adults (Burani et al., Reference Burani, Brush, Shields, Klein, Nelson, Slavich and Hajcak2022; Goldstein et al., Reference Goldstein, Kessel, Kujawa, Finsaas, Davila, Hajcak and Klein2020; Pegg et al., Reference Pegg, Ethridge, Shields, Slavich, Weinberg and Kujawa2019). Evidence also suggests that blunted reward processing prospectively predicts greater life stress and future depressive symptoms among adolescents (Mackin et al., Reference Mackin, Goldstein, Mumper, Kujawa, Kessel, Olino, Nelson, Hajcak and Klein2023).
Early life stress has also been shown to impact the development of reward-related brain activity (Novick et al., Reference Novick, Levandowski, Laumann, Philip, Price and Tyrka2018; Pechtel & Pizzagalli, Reference Pechtel and Pizzagalli2010). However, there is limited evidence regarding the role of stress in the development of the RewP across adolescence. Suor and colleagues (Suor et al., Reference Suor, Granros, Calentino, Luan Phan and Burkhouse2021) found that, in 9 to 16-year-old youth, greater childhood adversity was associated with a more blunted concurrent RewP among youth with mothers with a history of depression compared to youth with mothers with no history of depression. Moreover, Burani et al. (Reference Burani, Brush, Shields, Klein, Nelson, Slavich and Hajcak2022) found that, in 8- to 14-year-old girls, cumulative life stress predicted a more blunted change in the RewP two years later. Collectively, these studies suggest that stress exposure early in childhood and adolescence are related to a more blunted RewP, but no study has explored whether exposure to stressful life events may impact developmental trajectories of the RewP from late childhood to early adulthood.
The present study utilized multilevel growth modeling to capture the developmental trajectories of reward-related brain activity during late childhood to early adulthood. Further, the study explored whether childhood psychosocial risk factors, including childhood anhedonia and exposure to stressful life events, predict changes in the RewP across adolescence. In a sample of 317 8- to 14-year-old girls (M age = 12.4, SD age = 1.8), participants completed self-report measures of depression symptoms and stressful life events at baseline and the doors guessing task to measure the RewP at baseline, 2-year, and 4-year follow-ups. We hypothesized that the RewP would demonstrate a within-person linear increase across adolescence and that greater childhood anhedonia and stressful life events at baseline would predict the smallest changes in the RewP across adolescence.
Method
Participants
The sample included 317 8 to 14 year-old girls who participated in the Impact of Puberty on Affect and Neural Development across Adolescence (iPANDA) project, a longitudinal investigation of trajectories of reward sensitivity and depression across adolescence. Participants were recruited via a commercial mailing list of families within a 30-mile radius of Stony Brook University with an 8–14-year-old girl and via fliers and online postings. Participants were eligible if they lived with an English-speaking biological parent and did not have any significant developmental or medical disabilities. Families were financially compensated for their participation. All tasks and procedures were approved by Stony Brook University’s Institutional Review Board.
ERP data was collected from 314 8 to 14 (M = 12.4, SD = 1.8) participants at baseline, 259 participants at the 2-year follow-up, and 192 participants at the 4-year follow-up for a total of 315 participants. One hundred and seventy-nine participants completed all three waves of EEG data collection, 86 participants completed only two waves of EEG data collection, and 50 participants completed only one wave of EEG data collection. The average time between the first and second assessment was 2.06 years (SD = 6.2 months) and between the second and third assessment was 3.38 years (SD = 11.1 months). Of the 765 EEG recordings collected across all three assessments, only 6 were excluded due to poor data quality (i.e., more than 50% of trials had unusable data). Participants with follow-up data did not differ in their baseline ERP response to gains (t = −0.52, p = 0.98) or losses (t = −0.39, p = 0.80) from participants who did not participate in at least one follow-up assessment. The ethnic/racial distribution of the current sample was 86.3% White, 6.7% Biracial, 6.3% Black, and 0.3% Native American. The medium family income of participants was $110,000. A total of 19 (6.0%) participants had CDI scores above the recommended clinical cutoff at wave 1.
Self-report measures
Child Depression Inventory (CDI)
The Child Depression Inventory (CDI) is a 27-item self-report questionnaire used to assess symptoms of depression over the past two weeks among youth ages 7–17 (Kovacs, Reference Kovacs1992). Item are rated on a 3-point scale from 0 to 2 and summed to create total and subscale scores, with higher scores indicating greater symptom severity. The CDI has been shown to have adequate psychometric properties (Saylor et al., Reference Saylor, Finch, Spirito and Bennett1984). The CDI was administered at the baseline assessment and the anhedonia subscale demonstrated excellent internal reliability (α = .93).
Adolescent Life Events Questionnaire (ALEQ)
The Adolescent Life Events Questionnaire (ALEQ) is a self-report questionnaire designed to measure a broad range of stressful life events that typically occur during adolescence, including school problems, family problems, and interpersonal relationship difficulties. Research has shown that the ALEQ has good reliability and validity (Hankin & Abramson, Reference Hankin and Abramson2002). Participants rated the frequency with which each of 57 stressful life events had occurred in the past 3 months using a 5-point rating scale from 0 (never) to 4 (always). The ALEQ was administered at the baseline assessment and the total score was calculated by summing the frequency ratings of each of the events. The internal consistency of the ALEQ was excellent (α = .93). At the baseline assessment, 248 participants completed the ALEQ; fewer participants completed the ALEQ since it was introduced as part of the study protocol after data collection had begun.
Task and procedure
Doors task
Participants completed the doors task administered using Presentation software (Neurobehavioral Systems, Inc., Albany, CA, USA) to elicit the RewP. The doors task consisted of 3 blocks of 20 trials each, separated by participant-timed breaks. On each trial, participants were asked to use the mouse to select between two identical doors presented on a computer screen and were informed that one door will result in winning money and the other will result in losing money. An equal number of gain and loss trials (30 each) were presented in a randomized order. Each trial began with a fixation cross (500 ms), followed by the image of two doors (until the participants make their selection), then another fixation cross (1,500 ms), followed by feedback (2000 ms) on their performance to indicate that the participant gained $0.50 (i.e., an upward green arrow) or lost $0.25 (i.e., a downward red arrow). Once the trial was over, another fixation cross was presented (1,500 ms) and then the prompt “Click for next round” appeared on the screen and remained until the participant responded with a button press to initiate the next trial. Participants were explicitly informed that they could win up to $15, which they could keep. All participants received $8 for completing the task.
Psychophysiological recording and data processing
Continuous EEG activity was collected while participants completed the doors task using the ActiveTwo BioSemi system (BioSemi, Amsterdam, the Netherlands). An elastic cap with 34 Ag/AgCl electrodes was placed according to the 10/20 system. Additional electrodes were placed on the mastoids as well as above and below the right eye and near the outer canthi of the left and right eyes to monitor vertical and horizontal electrooculographic activity, respectively. To improve the signal-to-noise ratio, the EEG signal was preamplified at the electrode. Data were digitized at a 24-bit resolution with a sampling rate of 1024Hz using a low-pass fifth-order sinc filter with a half-power cutoff of 204 Hz.
Data processing was performed offline with BrainVision Analyzer 2.2 (Brain Products, Gilching, Germany). Raw EEG data were re-referenced to the average of the left and right mastoids and band-pass filtered from 0.1 to 30 Hz. Eyeblink and ocular corrections were conducted using the Gratton and Coles method (Gratton et al., Reference Gratton, Coles and Donchin1983). An automatic procedure was used to identify and remove trials contaminated by artifacts. Data from individual channels were automatically rejected if a voltage step of more than 50.0μV between sample points or a voltage difference of 300.0 μV within a trial existed. In addition, data were identified as artifacts if a voltage difference of less than 0.50 μV within 100-ms intervals was present. Finally, visual inspection of the data was conducted to detect and reject any remaining artifacts. Feedback-locked epochs were extracted with a duration of 1,000 ms, including a 200 ms prior to feedback presentation and 800 ms following the feedback presentation. The 200 ms prestimulus segment served as the baseline. Feedback-locked ERPs were averaged separately for gains and losses. The RewP was quantified as the mean amplitude from 250 to 350 ms at FCz following feedback onset, and the ΔRewP was computed by subtracting gain minus loss. The average (and standard deviation) number of usable loss trials for waves 1, 2, and 3 were 29.5 (1.0), 29.8 (0.9), and 28.5 (1.8), respectively. The average (and standard deviation) number of usable gain trials for waves 1, 2, and 3 were 29.6 (0.9), 29.8 (0.9), and 29.6 (1.6), respectively.
Data analysis
Paired-samples t-tests were conducted using SPSS (version 26.0) at each time point (baseline, 2-year follow-up, 4-year follow-up) to assess for differences in mean ERP responses between gain and loss trials for the doors task. Multilevel growth modeling was used to assess change in reward processing across adolescence. Multilevel growth modeling is a widely used statistical technique that allows for the examination of inter-individual (between-person) differences in intra-individual (within-person) patterns of change across time. Multilevel growth models are composed of both fixed and random effects, where the fixed effect estimates the mean of the trajectory across all participants and the random effect estimates the variance of participant trajectories around the sample mean (Curran et al., Reference Curran, Obeidat and Losardo2010). In the present study, time was centered at age 12 and coded time as years since age 12. Age 12 was chosen as a reference point by which to assess changes in reward processing across development since it was the average age of participants at the baseline assessment.
We fit a series of nested unconditional growth models to establish the average trajectories of the ERP response to gain, ERP response to loss, and gain-loss (i.e., ΔRewP). For each dependent variable, we began with a random intercept only model (model 0). We used this model to calculate interclass correlation coefficients (ICC), reliability, and model fit statistics for later comparisons to more complex models. Following the random intercept model, we added a fixed slope effect (model 1), which represents the average rate of linear change of the dependent variable across time. Lastly, in model 2, we added a random slope effect, which represents variability in rates of linear change across time. This model also allowed us to examine the estimated covariance between the random intercept and slope.
To compare model fit across the different nested models, we conducted likelihood ratio tests (LRT). A significant LRT indicates that the more complex model improves model fit compared to the less complex model. If the LRT was significant, we then examined whether the added effect was statistically significant. The best-fitting model was the most complex model that met these criteria.
To identify predictors of the trajectory of the RewP across time, we fit conditional growth models by adding predictors into the best-fitting unconditional growth models. In two separate conditional growth models we examined whether anhedonia symptoms and stressful life events (level 2 time-invariant predictors) at baseline predicted trajectories of the RewP across adolescence. We also included age at baseline as a level 2 time-invariant predictor. All time-invariant predictors were grand mean centered. We included interactions of the slope with time-invariant predictors of interest, anhedonia symptoms and stressful life events.
All models were computed using version 1.1.28 of the lme4 (Bates et al., Reference Bates, Mächler, Bolker and Walker2015) and version 3.1.3 of the lmerTest (Kuznetsova et al., Reference Kuznetsova, Brockhoff and Christensen2017) packages for R using Restricted Maximum Likelihood Estimation. Maximum Likelihood Estimation was used for comparing the nested models to determine the best-fitting model. Satterwaite approximation method was used for the approximation of standard errors, confidence intervals, and p-values. These methods of parameter estimation allowed us to include participants who did not complete all three time points of data collection. See Supplemental Materials for correlations between study variables, descriptive statistics, reliability metrics, and results for a conditional models examining total depression symptoms predicting RewP trajectories and anhedonia and stressful life events predicting RewP trajectories.
Results
Sample demographics
Participants who completed only one timepoint of data collection were significantly older than participants with three time points of data collection, F(2, 312) = 3.25, p = .04. However, participants who completed one time point of data collection did not significantly differ in age at baseline from participants who completed two time points of data collection, and participants who completed two time points of data collection did not significantly differ in age at baseline from those who completed three time points of data collection (p > .05). Participants did not differ on any other demographic variables as a function of number of time points.
Task effects
Figure 1 displays the ERP waveforms and scalp distributions at each time point of the study and Table 1 shows the average ERP data at the baseline, 2-year, and 4-year follow-up assessments. As expected, the paired sample t-tests indicated that the ERP response to gain feedback was more positive than the ERP response to loss feedback at each time point (baseline = t(312) = 10.88, p < .001; 2-year follow up = t(257) = 12.34, p < .001; 4-year follow up = t(188) = 11.15, p < .001).
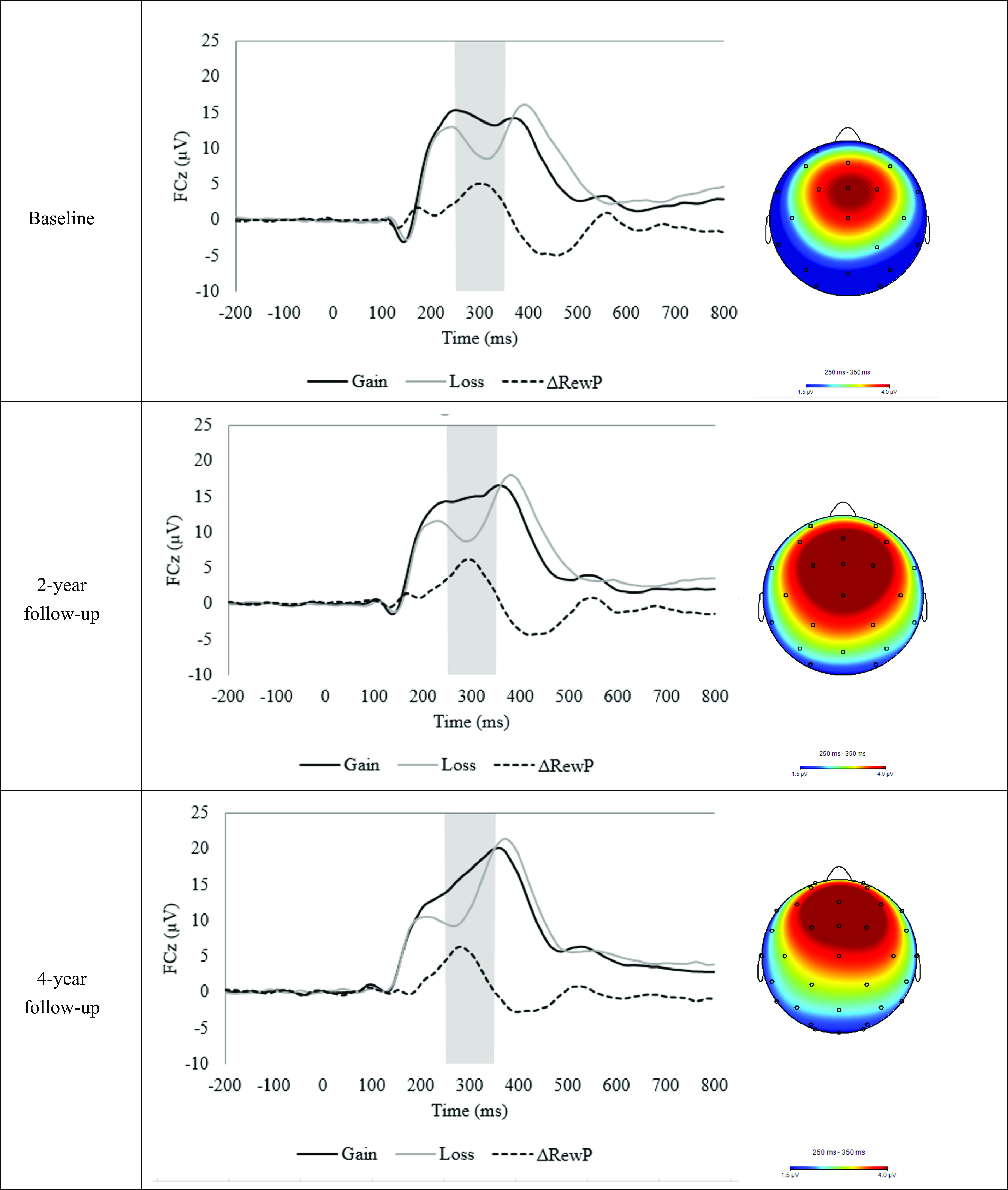
Figure 1. Event-related potential (ERP) waveforms (left) and scalp distributions (right) for the doors task at the baseline, 2-year follow-up, and 4-year follow-up assessments.
Table 1. ERP data for the doors task at baseline, 2-year follow-up, and 4-year follow-up

Note. SD = standard deviation.
Trajectories of reward sensitivity
Unconditional growth models
Table 2 shows the results of all unconditional growth models, and Fig. 2 shows the model-estimated trajectories of reward-related neural activity across adolescence using the best-fitting unconditional models. Change in the ERP response to gain is best captured by the random intercept and slope model (model 2; slope variance = 0.55; 95% CI = 0.22–1.01). The ERP response to gain model is characterized by a positive linear slope across adolescence, t(220) = 6.86, p < .001, with variability in the rate of linear change. In contrast, the best-fitting growth model for the ERP response to loss is a random intercept and fixed slope linear growth model. The ERP response to loss model is characterized by a linear increase across adolescence (positive slope effects), t(609) = 7.31, p < .001, with no variability in the rate of linear change. Lastly, analyses indicated that the ΔRewP (gain minus loss) random intercept and fixed slope model (model 1) was not a better fitting model than the random intercept only model (model 0). However, when comparing the fixed and random slope ΔRewP models, the best-fitting growth model for the ΔRewP is a random slope linear growth model characterized by a nonsignificant slope across adolescence, t(257) = 0.95, p = 0.34. Similar to the ERP response to gain growth model, the ΔRewP model is characterized by significant variability in the rate of linear change among the sample (slope variance = 0.17; 95% CI = 0.21–.67).
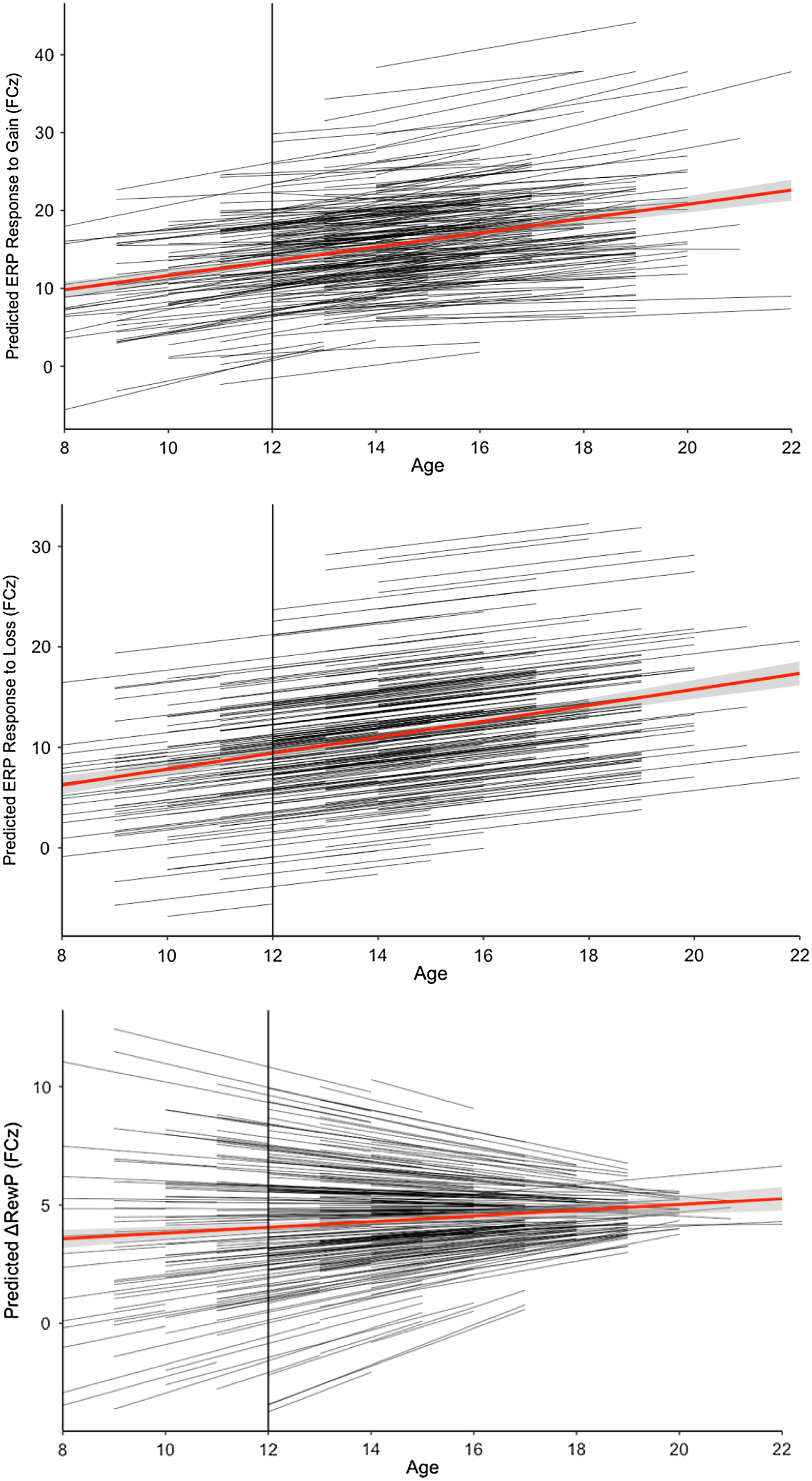
Figure 2. Model-estimated trajectories of ERP reward-related brain activity across adolescence.
Table 2. Unconditional multilevel growth models of ERP reward-related brain activity
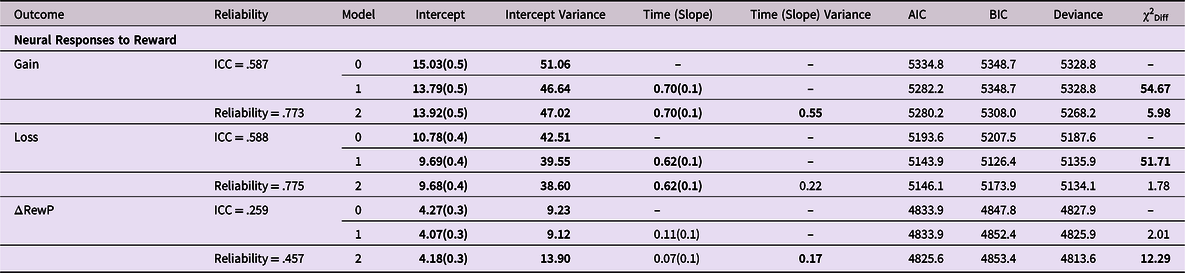
Note. Model 0 = random intercept only; Model 1 = linear growth model with a fixed slope; Model 2 = linear growth model with a random slope; χ2 Diff = likelihood ratio test comparing fit of nested models as follows: model 1 is compared to a model with the random intercept as the only predictor (results not shown), model 2 is compared to model 1; time is coded as years since age 12; Acronyms are defined as follows: AIC = Akaike Information Criteria, BIC = Bayesian Information Criteria, ICC = intraclass correlation; Significant effects are bolded and the best-fitting model for each outcome is shaded in grey.
Conditional growth models
Table 3 shows the results of the conditional growth models of baseline anhedonia symptoms and stress predicting the ΔRewP. The first conditional growth model examining whether anhedonia symptoms at baseline predicts change in the ΔRewP across adolescence controlling for age and stress at baseline revealed no significant main effects or slope effect, t(256) = 0.87, p = .39. However, variability in the slope of the ΔRewP across adolescence remained significant (slope variability = 0.15; 95% CI = 0.19–0.73) indicating that the predictors have not explained all of the variance in the slope. Results also indicated a significant CDI Anhedonia X Timepoint interaction, t(162) = −2.22, p = .03. To further probe this interaction, we estimated the simple slopes for participants with mean anhedonia scores and anhedonia scores one standard deviation above and below the sample mean. As shown in Figure 3, participants with baseline anhedonia scores one standard deviation below the mean had positive, significant linear trajectories of the ΔRewP across adolescence (estimate = 0.33, SE = 0.13, t = 2.51, p = .01). However, the trajectory of the ΔRewP across adolescence was not significantly different than zero for participants with anhedonia scores at the mean (estimate = 0.14, SE = 0.10, t = 1.48, p = .14) or one standard deviation above the mean (estimate = −0.04, SE = 0.13, t = −.35, p = .73). See Supplemental Materials for analyses demonstrating the specificity of the results to anhedonia.

Figure 3. Model-estimated trajectories of the ΔRewP for different anhedonia (top) and stressful life events (bottom) scores.
Table 3. Conditional multilevel growth models of anhedonia symptoms and stressful life events predicting the ΔRewP across adolescence

Note. SE = standard error; ALEQ = Adolescent Life Events Questionnaire; time is coded as years since age 12; significant effects are bolded.
The second conditional growth model examining whether baseline stressful life events predicts change in the ΔRewP across development showed that, after controlling for baseline age and anhedonia symptoms, the slope effect for the ΔRewP remained non-significant, t(215) = 1.43, p = .15, but the variability in the slope was significant (slope variance = 0.18; 95% CI = 0.17–0.75). Although there were no significant main effects, there was a significant ALEQ X Timepoint interaction, t(197) = −2.02, p = .044. Figure 3 shows the estimated model trajectories for participants with mean ALEQ scores and ALEQ scores one standard deviation above and below the sample mean. Change in the ΔRewP is characterized by a non-significant slope for participants who had ALEQ scores at the mean (estimate = .14, SE = .10, t = 1.43, p = .15) or one standard deviation above the mean (estimate = −.04, SE = .13, t = −0.32, p = .75), but a significant positive slope for individuals who scored one standard deviation below the mean (estimate = 0.32, SE = 0.13, t = 2.38, p = .02). See Supplemental Materials for analyses examining the independence of the relationship between anhedonia symptoms and ALEQ scores in relation to change in the ΔRewP across adolescence.
Discussion
The present study employed multilevel growth modeling to examine the developmental trajectories of ERP reward-related brain activity across adolescence. Results indicated that, across all participants, the ERP response to gain and loss demonstrated a linear increase across adolescence. In contrast, the ΔRewP (i.e., gain-loss) did not change across adolescence. However, the ΔRewP demonstrated between-person variability in rate of change across adolescence. Notably, there was significant variance in the random intercepts in all of the growth models, suggesting that there is significant between-person variability in participant ERP response to gain, loss, and the ΔRewP at age 12. Results also indicated that baseline anhedonia symptoms and stressful life events predicted within-person changes in the ΔRewP across adolescence. Specifically, individuals with low anhedonia symptoms demonstrated a positive, linear increase in the ΔRewP across adolescence, but individuals with average or high anhedonia symptoms did not show significant change in the ΔRewP across adolescence. Identical results were obtained for the conditional model using exposure to stressful life events as a predictor of change in the ΔRewP. Overall, the present study suggests there is significant between-person variability in the trajectory of the ΔRewP across adolescence, which can be partially explained by early life risk factors, such as anhedonia and stressful life experiences during childhood and early adolescence.
The present study adds novel evidence to a growing literature suggesting that adolescence is a critical period for the development of reward-related brain activity. Only two other studies have examined longitudinal, within-person changes in the ΔRewP across adolescence, which found no change in the ΔRewP (Kujawa et al., Reference Kujawa, Carroll, Mumper, Mukherjee, Kessel, Olino, Hajcak and Klein2018) and increases in the RewP among younger participants (Burani et al., Reference Burani, Klawohn, Levinson, Klein, Nelson and Hajcak2019) across adolescence. The present study extends these findings and indicates that there are within-person linear increases in the ΔRewP across adolescence—particularly in those with low risk for depression.
The present study and Burani et al. (Reference Burani, Klawohn, Levinson, Klein, Nelson and Hajcak2019) utilized the same adolescent sample, but differed in that only the latter investigation found a longitudinal increase in the ΔRewP for younger, but not older, participants. The present study included two key differences from Burani et al., including an additional third assessment of reward-related brain activity that spanned a broader developmental window (ranging from late childhood to early adulthood). Casey’s (2008) neurobiological model suggests that adolescence is characterized by different development trajectories of limbic subcortical regions and prefrontal regions, such that subcortical regions develop earlier than prefrontal regions, resulting in nonlinear changes in neurodevelopment. Consistent with this model, neuroimaging studies have found that striatal activity in response in reward increases in adolescence and decreases in adulthood, thereby providing evidence of a non-linear (quadratic) developmental pattern of reward-related brain activity (Silverman et al., Reference Silverman, Jedd and Luciana2015; Steinberg et al., Reference Steinberg, Albert, Cauffman, Banich, Graham and Woolard2008). The present study was able to assess for linear changes in reward-related brain activity—however, this occurred across a broad developmental period that could be characterized by non-linear changes in neurodevelopment. Future studies aimed at characterizing within-person trajectories of reward-related brain activity should take into consideration both the age span and number of assessments to optimize the evaluation of neurodevelopment.
The present study is the first to use multilevel growth modeling to examine within-person changes in ERP measures of reward processing from late childhood to young adulthood. The present study found that the ERP response to gain and loss increased across adolescence, but that only the ERP response to gain (not loss) showed significant variability in slope. Research studies using principal components analysis have found that the ERP response to loss is comprised of multiple overlapping components, including the P200 and the P300 (Foti, Weinberg, et al., Reference Foti, Weinberg, Dien and Hajcak2011; Proudfit, Reference Proudfit2015). However, in addition to the P200 and the P300 components, the ERP response to gain also contains a positive deflection that occurs between 250 and 350 ms following feedback (i.e., the ΔRewP). It is likely that the additional reward-related positivity that is present for gain trials (and absent for loss trials) contributed to the variability and uniformity in slope for ERP responses to gain and loss, respectively, shown across adolescence.
The present study provides novel evidence that childhood risk factors for depression predicted the developmental trajectories of the ΔRewP. Consistent with cross-sectional studies showing that anhedonia (Foti et al., Reference Foti, Carlson, Sauder and Proudfit2014b; Liu et al., Reference Liu, Wang, Shang, Shen, Li, Cheung and Chan2014) and stress exposure (Burani et al., Reference Burani, Brush, Shields, Klein, Nelson, Slavich and Hajcak2022; Suor et al., Reference Suor, Granros, Calentino, Luan Phan and Burkhouse2021) are associated with blunted reward-related brain activity, the present study found that both risk factors predicted changes in the ΔRewP across adolescence. These results are consistent with the fMRI literature, which has found that greater anhedonia symptoms and greater stress exposure are negatively associated with activation in reward circuitry, such as the ventral striatum, in clinical and non-clinical samples (Casement et al., Reference Casement, Shaw, Sitnick, Musselman and Forbes2015; Epstein et al., Reference Epstein, Pan, Kocsis, Yang, Butler, Chusid, Hochberg, Murrough, Strohmayer, Stern and Silbersweig2006; Harvey et al., Reference Harvey, Pruessner, Czechowska and Lepage2007). Further, research suggests that aberrations in reward processing may mediate relationships between early life risk factors and subsequent development of psychopathology (Hanson et al., Reference Hanson, Hariri and Williamson2015). Notably, these findings were specific to anhedonia symptoms and not overall depression symptoms (see supplemental materials for more details), suggesting that early childhood anhedonia symptoms may be an important intervention target associated with the development of aberrant reward processing patterns implicated in the etiology of depression. The exact neurobiological mechanisms that underlie associations between stress exposure and reduced reward-related brain activity are not fully understood. However, anhedonia has been proposed as a trait-like risk-factor that contributes to the liability of developing psychopathology (Hasler et al., Reference Hasler, Drevets, Manji and Charney2004). It is possible that childhood-onset anhedonia might contribute to the subsequent development of depression via aberrant reward-related neurodevelopment. Future research should examine how trajectories of reward-related brain activity and early risk factors relate to simultaneous trajectories of depression symptoms.
The present study had a number of strengths, including a longitudinal design that spanned the entirety of adolescence, three assessments of reward-related neural activity, a large sample size, and the use of multilevel growth modeling. Nevertheless, the results of the current study should be interpreted in light of several limitations. First, we used a largely homogenous sample of predominantly White, high socioeconomic status, adolescent girls, which limits the generalizability of our study findings to males and other more diverse samples. Further, the majority of sample (93%) were experiencing non-clinical depressive symptoms at baseline and future research would benefit from studying these patterns in a clinical or more risk-enhanced sample. Second, it is important to note that the ALEQ probes for stressful life events over the past three months and the CDI examines depression symptoms over the past two weeks. It is possible that participants had experiences of stressful life events and/or depression symptoms that fell outside of these time windows and may have impacted reward processing. Additionally, although the ALEQ has been found to be reliable and valid in populations of youth in 9th–12th grade (Hankin & Abramson, Reference Hankin and Abramson2002) and used in samples of this age range (Burani, Klawohn, et al., Reference Burani, Klawohn, Levinson, Klein, Nelson and Hajcak2019; Mehra et al., Reference Mehra, Hajcak and Meyer2022), we are unaware of studies where the ALEQ has been validated in children as young as nine. Third, participants were not screened for a prior history of depression and/or experiences of childhood adversity that may also have impacted the present results. Forth, although we chose age as our time variable, we did not collect measures of reward-related ERPs from all participants at every age and not all ages contained the same number of data points, which has the potential to bias our results. Therefore, it is important that researchers continue to examine longitudinal changes in the ΔRewP across critical developmental time periods. Lastly, although we were able to use growth modeling to examine trajectories of reward-related ERPs, we did not examine non-linear trajectories. Findings from neuroimaging research suggests that the ΔRewP may follow a quadratic or cubic developmental trajectory, increasing during adolescence and decreasing during adulthood (Silverman et al., Reference Silverman, Jedd and Luciana2015; Steinberg et al., Reference Steinberg, Albert, Cauffman, Banich, Graham and Woolard2008). We hope to examine non-linear developmental changes in reward-related ERPs following our fourth wave of data collection.
The ΔRewP is a promising biomarker of risk for the development of psychopathology (Proudfit, Reference Proudfit2015). However, in order for the ΔRewP to be clinically useful, it’s critical that researchers understand normative patterns of change in the ΔRewP across development and how the developmental trajectory of the ΔRewP may relate to early risk factors or combinations of risk factors. The present study demonstrates that, among a large sample of adolescent girls, ERP measures of gain and loss increase across adolescence into early adulthood, which is consistent with evidence of changes in reward-related brain function across adolescence (Casey et al., Reference Casey, Getz and Galvan2008b; Luking et al., Reference Luking, Pagliaccio, Luby and Barch2016). Moreover, early exposure to stressful life experiences and anhedonia symptoms predicted the developmental trajectory of the ΔRewP, suggesting that early risk factors impact trajectories of reward-processing, which may subsequently increase the risk for later psychopathology. Future research aimed at characterizing typical and atypical trajectories of reward-related ERPs as well as how early risk factors may impact these trajectories is essential for the translation of clinical neuroscience research to clinical settings for prevention.
Supplementary material
The supplementary material for this article can be found at https://doi.org/10.1017/S0954579424000701.
Data availability statement
Research data are not shared.
Funding statement
This work was supported by the National Institute of Mental Health R01MH097767 awarded to Drs. Brady D. Nelson and Greg Hajcak and a National Science Foundation graduate research fellowship awarded to Aline K. Szenczy.
Competing interests
The authors have no conflicts of interest to disclose.