Introduction
Mood instability (MI) and sleep disturbance (SD) are clinically important features of mental illness. Although part of the diagnostic criteria for only some conditions, they are present in a wide range of mental disorders and have been regarded individually as potential transdiagnostic processes in the origin and maintenance of mental illness [Reference Broome, Saunders, Harrison and Marwaha1–Reference Harvey, Murray, Chandler and Soehner3]. MI and SD may be involved in a set of pathways that interact to produce poor mental health outcomes that transcend diagnostic boundaries [Reference Wulff, Gatti, Wettstein and Foster4,Reference Harvey5]. However, clinical evidence of the relationship between MI and SD is limited, and research has been mostly confined to small samples of specific mental disorders with limited generalizability.
We have previously demonstrated that MI and SD are closely linked and common in the general population, irrespective of mental illness [Reference McDonald, Saunders and Geddes6]. MI and SD are associated with extensive use of resources for mental health care, and their co-occurrence may be related to poorer health and social outcomes than either symptom alone [Reference McDonald, Saunders and Geddes7].
To further investigate the relationship between MI and SD as transdiagnostic features within the clinical population, this study examined data from a large sample of electronic health records (EHRs) from secondary mental health care. In the United Kingdom, access to secondary mental health care providers of specialized services such as hospitals, some psychological services, community mental health teams, and early intervention teams. Usually requires a referral from primary health care providers (such as general practitioners [GPs]). EHRs contain a wealth of clinical information from secondary services, often recorded as text. Recently, the development of novel natural language processing (NLP) approaches has enabled efficient identification of clinical information from text within EHRs, thereby permitting the secondary analysis of large quantities of data not typically feasible by manual review. We used NLP to study MI and SD in a large sample of individuals presenting to mental health services. Specifically, we sought to describe the demographic and clinical features of persons presenting with MI and/or SD and to assess the incidence rates of clinical outcomes in individuals presenting with MI and/or SD relative to persons with neither symptom.
Methods
Data source
Data were obtained from EHRs from the Oxford Health NHS Foundation Trust (OHFT) using the Clinical Research Interactive Search (CRIS; https://crisnetwork.co.uk). OHFT is the National Health Service (NHS) provider of mental health care to a catchment area of approximately 1.6 million residents in Oxfordshire, Buckinghamshire, Swindon, Wiltshire, Bath, and North East Somerset. CRIS is an anonymized database of EHRs developed at South London and Maudsley (SLaM) NHS Foundation Trust and King’s College London with the National Institute for Health Research (NIHR), and consists of structured (e.g., numbers and dates) and unstructured text (e.g., clinical notes and correspondence) data fields.
Sample
We included EHRs of all individuals aged 16–65 years who presented to the OHFT between December 31, 2007 and January 1, 2016. Study entry was the date of the patients’ first clinical progress note. Patients were followed-up until the date of their most recent clinical progress note or 31 January 2018. Based on the assumption that patients hospitalized long-term are not representative of the broader clinical psychiatric population, we excluded patients with a hospital duration longer than two standard deviations above the mean number of days in hospital for the total patient sample (mean = 19.8, SD = 120.1 days).
Data extraction
Where available, data were extracted from routinely completed structured fields. Data extraction from unstructured fields was supported by a set of NLP applications described previously [Reference Patel, Lloyd, Jackson, Ball, Shetty and Broadbent8,Reference Perera, Broadbent, Callard, Chang, Downs and Dutta9]. These applications permit efficient, automatic processing of free text clinical notes and correspondence using General Architecture for Text Engineering (GATE) [Reference Cunningham10] and TextHunter [Reference Jackson, Ball, Patel, Hayes, Dobson and Stewart11] software.
Measures
Mood instability
Three NLP applications were used extract documentation of MI, affective instability, and emotional instability, and outputs were combined into a single parameter (which we termed MI). The terms identified by the applications included frequently used combinations of mood, affect, and instability with modifier terms such as dysfunction, instability, and lability [Reference Patel, Lloyd, Jackson, Ball, Shetty and Broadbent8].
Sleep disturbance
The presence of sleep problems and insomnia were identified using two separate NLP applications. The sleep problems application searched for instances of selected modifier terms (poor, interrupt, disturb*, inadequate, no, problem*) within 0–5 words either side of the keyword (sleep). The insomnia application searched for instances of insomn* within the text. Since the NLP applications for insomnia and sleep problems were produced separately, we initially evaluated their outputs separately. However, given the overall aim of assessing SD more generally, we combined their outputs into a single SD variable for the main analysis.
Prescribed medication
The medication application was designed to extract the names and dosages of medications prescribed to the patient. The application ignores medications that might be prescribed in the future (e.g., a medication to be considered if the patient’s condition worsens). We included antidepressants, antipsychotics, mood-stabilizing antiepileptics, lithium, sedative-hypnotics, sympathomimetics, amphetamines, and sedating antihistamines, defined according to the British National Formulary (https://bnf.nice.org.uk/; Supplementary Table S1).
Diagnosis
Diagnoses, classified according to the International Classification of Disease 10th revision (ICD-10) [12] were extracted from structured fields, including primary diagnoses of organic mental disorders (F00–F09), substance use disorders (F10–F19), schizophrenia and related (F20–F29), mood disorders (F30–F39), anxiety disorders (F40–F49), behavioral syndromes (F50, F52–F59), personality disorders (F60–F69), mental retardation (F70–F79), disorders of psychological development (F80–F89), behavioral and emotional disorders with onset usually in childhood and adolescence (F90–F98), and unspecified mental disorders (F99). Given the specific interest in SD, sleep disorders (F51, G47) were initially examined separately, but sample sizes were too small for estimation.
Events
The number of contacts (events) for each patient was identified from dates of clinical notes, diary and clinic appointments, and participation in group therapies.
Hospitalization
Frequency and duration of hospitalization were extracted based on hospital admission and discharge dates recorded in structured fields.
Health of the Nation Outcome Scale
The Health of the Nation Outcome Scale (HoNOS) is a 12-item scale that measures the health and social functioning of people of working age with severe mental illness [Reference Wing, Beevor, Curtis, Park, Hadden and Burns13]. HoNOS is a routine outcome measure for the NHS used to assist with offering patients the care and interventions to meet their individual needs [14]. The total score was adjusted for missing responses to individual items (12*total score/number of responses). Assessments missing more than three responses were excluded.
Statistical analysis
We selected a random subset of 100 documents from the final sample to validate each NLP application against classifications by a human annotator (K.M.). Each feature (MI, sleep problems, insomnia, and medication) was classified as present or absent at the document-level. Manual classifications were compared with the NLP classifications, and sensitivity, and positive predictive value were calculated.
For the remaining analyses, study groups (MI-only, SD-only, MI and SD, no MI or SD) were defined based on their documentation within 1 month of first presentation to OHFT (baseline). This enabled comparability with a methodologically similar study [Reference Patel, Lloyd, Jackson, Ball, Shetty and Broadbent8] and was compatible with timings of outcomes of interest. Prevalence was calculated as proportions with 95% confidence intervals (CI) at baseline and 2-years follow-up. Demographic and clinical features within each subgroup were described using estimates of frequencies, proportions, means and standard deviations. Incidence rate ratios (IRRs) of outcomes in each study group (MI-only, SD-only, and MI and SD) versus the common referent (no MI or SD) were assessed within 2-years of presentation. The family-wise error rate was controlled using the Bonferroni–Holm procedure [Reference Holm15]. All analyses were performed in R [16].
Results
A total of 31,912 patients aged 16–65 years presented to OHFT between December 31, 2007 and January 1, 2016. We excluded 521 patients for having a hospital duration greater than 260 days (see Section “Sample”), yielding a final sample of 31,391 patients.
Validation of the NLP outputs
Estimates of sensitivity ranged between 70.3% for MI and 85.9% for the medication application. Positive predictive values ranged between 82.1% for sleep problems and 93.8% for the MI application (Table 1).
Table 1. Estimates of sensitivity and positive predictive value for each NLP classifier against manual annotation.

Abbreviations: PPV, positive predictive value; Se, sensitivity.
Demographic and clinical features of the sample at baseline and 2-years follow-up
Of the total sample, approximately 4.2% (n = 1,322) had MI-only, 20.9% (n = 6,559) had SD-only, 5.4% (n = 1,684) had MI and SD, and 69.7% (n = 21,826) had neither symptom documented at baseline.
Patients presenting with SD-only were more often male, whereas the remaining groups were more often female (Table 2). Age distributions were similar across all groups. Patients presenting with MI and SD were most often diagnosed with any psychiatric (29.9%), mood (16.3%), personality (4.6%), and schizophrenia and related (3.4%) disorders. MI-only showed the highest proportion of behavioral syndromes (1.9%), whereas SD-only had the highest proportion of anxiety disorders (5.5%). Few individuals without either symptom had any psychiatric diagnosis (11.7%).
Table 2. Descriptive features of the sample with mood instability-only, sleep disturbance-only, mood instability and sleep disturbance, and no mood instability or sleep disturbance within 1 month of presenting to OHFT (n = 31,391).

Abbreviation: HoNOS, Health of the Nation Outcome Scale adjusted total score.
– represents estimates unavailable due to small numbers.
Polypharmacy was most frequent in patients with both MI and SD, whereas patients without MI or SD were taking the fewest medications. Patients with MI and SD (24.2%) were most often hospitalized within 1 month of presentation compared with SD-only (8.5%), MI-only (6.7%), and no MI or SD (1.7%). The mean number of days spent in hospital were similar across the four groups.
There were 14,351 patients with at least 2 years of follow-up data. Age distributions were similar, but a slightly higher proportion of females than males remained in the sample. Between 51.6% and 74.5% had a diagnosis at 2-years follow-up (vs. 11.7%–29.9% at baseline). The proportion of patients prescribed multiple medications and hospitalized remained highest in MI and SD. Patients without MI or SD had the fewest hospitalizations, but spent, on average, approximately 6–14 days longer in hospital than the patients from the other groups. Few individuals in any study group received HoNOS assessments within 2 years of first presentation (Table 3).
Table 3. Descriptive features of the sample with mood instability-only, sleep disturbance-only, mood instability and sleep disturbance, and no mood instability or sleep disturbance within 2-years of presenting to OHFT (n = 14,351).
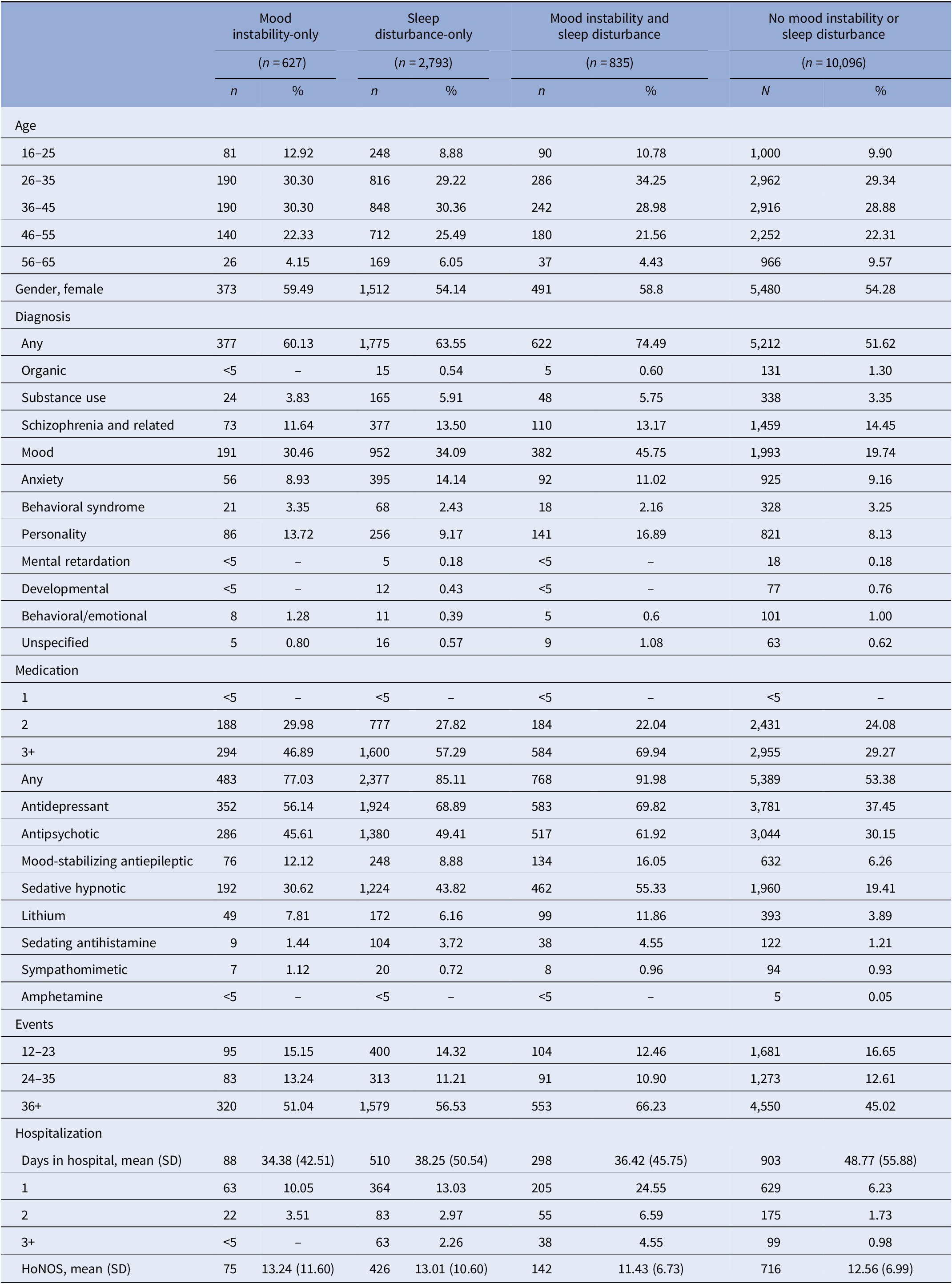
HoNOS=Health of the Nation Outcome Scale adjusted total score.
– Estimates unavailable due to small numbers.
Prevalence of mood instability and sleep disturbance
The prevalence of MI at baseline was approximately 9.6% (95% CI: 9.3–9.9), was higher in females, and decreased with age. Approximately 26.3% (95% CI: 25.8–26.8) of patients presented with SD (sleep problems or insomnia). Prevalence of SD was higher in males and increased with age, except among the oldest age group for whom prevalence was lowest. The prevalence of sleep problems and insomnia were 24.9% (95% CI: 24.4–25.4) and 4.0% (95% CI: 3.8–4.3), respectively, and showed similar trends in gender and age-specific prevalence (Table 4).
Table 4. Total, gender-specific, and age-specific prevalence estimates for mood instability, sleep problems, insomnia, and combined sleep problems and insomnia.

Incidence rates of selected clinical features
After adjusting for multiple comparisons, the IRR of any psychiatric diagnosis was significantly increased in persons with MI and SD (IRR = 1.8, 05% CI: 1.7–2.0, p < 0.0001) and SD-only (IRR = 1.4, 95% CI: 1.4–1.5, p < 0.0001), but not MI-only (IRR = 1.2, 95% CI: 1.0–1.3, p < 0.007) compared to those without MI or SD (Table 5). The IRR for substance use was strongest among those with SD-only (IRR = 1.5, 95% CI: 1.3–1.8, p < 0.001), whereas the IRR for mood disorder was strongest for those with MI and SD (IRR = 1.8, 95% CI: 1.6–2.0, p < 0.0001). SD-only was associated with significantly increased IRR of antidepressants and sedative-hypnotics. MI and SD showed significantly increased IRR for lithium, and had the highest IRR of first hospitalization within 2-years follow-up (IRR = 5.32, 95% CI: 4.7–6.1, p < 0.0001).
Table 5. Estimated incidence rate ratios of diagnoses, prescribed medications, and first hospitalization within 2 years of presenting to OHFT in mood instability-only, sleep disturbance-only, and mood instability and sleep disturbance versus no mood instability or sleep disturbance.

* Indicates significance in relation to the Bonferroni-Holm critical value.
– represents estimates unavailable due to small numbers.
Discussion
Summary of findings
This study examined clinical outcomes among patients presenting with MI and/or SD to secondary mental health services, and provides further evidence that MI and SD are commonly found in a wide range of psychiatric disorders [Reference Marwaha, Parsons, Flanagan and Broome17–Reference Roth, Jaeger, Jin, Kalsekar, Stang and Kessler19].
We observed a slightly lower prevalence of MI (9.58%) than a previous study using the MI application in data from SLaM NHS Trust (12.1%) [Reference Patel, Lloyd, Jackson, Ball, Shetty and Broadbent8]. This is likely because Patel et al. restricted their sample to affective, psychotic or personality disorders, many of which are often characterized by MI [Reference Bopp, Miklowitz, Goodwin, Stevens, Rendell and Geddes20–Reference Trull, Solhan, Tragesser, Jahng, Wood and Piasecki22], whereas our sample included all patients, irrespective of diagnosis. We found a higher prevalence of MI in females and young people, consistent with Patel et al. [Reference Patel, Lloyd, Jackson, Ball, Shetty and Broadbent8] and with patterns observed in the general population [Reference McDonald, Saunders and Geddes6].
The observed patterns in age and gender-specific prevalence of SD align with the wider literature, where increased prevalence is associated with age and female gender [Reference Arber, Bote and Meadows23,Reference Ohayon24]. However, the overall prevalence of SD observed in this study was considerably lower than expected for a clinical psychiatric populations [Reference Hombali, Seow, Yuan, Chang, Satghare and Kumar25], which is likely due to the limited range of terms identified by the SD NLP applications.
Regardless of the presence of MI and/or SD, the most frequent diagnoses were mood, anxiety, personality and schizophrenia and related disorders. Although there were some differences between the study groups, there were no clear trends in their relationships with specific diagnostic categories. This appears to support the hypothesis that MI and SD are transdiagnostic processes given their presence in nearly all classes of primary mental disorders.
The proportion of patients with a recorded diagnosis was low despite continued care; only approximately 56% of patients had a diagnosis within 2 years. The diagnosis field is not compulsory and located within a separate part of the EHR, and therefore may not be routinely filled in by health care providers. Many assessments are not conducted by doctors, and non-medical health care providers may feel less comfortable or able to give a diagnosis. An examination of records from SLaM found that only 82%–93% of patients were ever assigned a primary diagnosis [Reference Perera, Broadbent, Callard, Chang, Downs and Dutta9]. Since we restricted our study to only primary psychiatric conditions within the past 2-years, a subset of patients with other diagnoses, such as neurological conditions, were excluded.
Notably, the prevalence of sleep disorder diagnoses in this study was negligible (<0.5%), and considerably lower than reported in the wider literature [Reference Hombali, Seow, Yuan, Chang, Satghare and Kumar25]. The low frequency may reflect a common view that SD is an epiphenomenon of the primary psychiatric disorder [Reference Harvey26]. If sleep problems are viewed as secondary symptoms, then sleep disorders may be underdiagnosed in primary psychiatric patients, even when SD is identified. Nevertheless, the proportion of sedative-hypnotic prescriptions was nearly two times higher in SD-only than MI-only, which may suggest recognition of SD as an important focus of treatment. Given extensive evidence that SD may be a maintaining factor in mental illness, a shift toward better recognition of SD will provide important benefits to treatment.
Individuals with MI and SD showed very high service use. Over 85% of people with MI and SD had three or more contacts with services within their first month of presentation, and over 65% had 36 or more visits over the 2-year period, equating to approximately more 1.5 visits per month. Interestingly, while patients without MI or SD had the fewest hospitalizations, they spent, on average, 1–2 weeks longer in hospital than patients from the other three groups. There may be several possible explanations for this finding. One possible reason may be the higher proportion of personality disorders in the patients with MI and/or SD compared with those without either feature. There is concern that hospitalization may be counterproductive in personality disorders [Reference Paris27], which may account for slightly shorter hospital duration in these groups compared to people without MI or SD. Further MI and SD are modifiable, and their stabilization may lead to improvements that allow for shorter hospitalizations [Reference Sheaves, Freeman, Isham, McInerney, Nickless and Yu28].
An unexpectedly small proportion of individuals in the sample had HoNOS ratings, and the low completion rate limits the conclusions that can be drawn from the HoNOS-related findings. HoNOS is a main routine outcome measure of health and social functioning in patients with mental illness in England, as set by the NHS [Reference Holm15], and comprise part of the Mental Health Minimum Data Set (MHSDS). The absence of these data may have important implications for resource allocation to OHFT and also for the implementation of mental health care in England.
This study adds to evidence that psychiatric symptoms like MI can be identified from EHRs using NLP applications with reasonable accuracy. Previous validation of the MI applications in a sample of EHRs from patients who presented to SLaM, showed a sensitivity of approximately 91%, and positive predictive values ranging from approximately 46%–73% for mood, affective, and emotional instability [Reference Patel, Lloyd, Jackson, Ball, Shetty and Broadbent8]. In our study, the combined accuracy of these three applications showed a similar specificity (92%), but considerably higher positive predictive value (94%). The other applications for identifying sleep problems, insomnia, and prescribed medications also showed good accuracy, with sensitivity estimates ranged between 80% and 86%, and positive predictive values between 82% and 92%.
Limitations
Some limitations to this study are acknowledged. First, EHRs represent only information documented during service provision and missing data cannot be quantified. For example, it is unclear whether a missing diagnostic code reflects the absence of a diagnosis or just no record of a diagnosis within that field.
MI, SD, and prescribed medications were identified from clinical progress notes and correspondence, and therefore, reflect only information documented during routine care. Specific symptoms may not be discussed uniformly or routinely in each patient, but rather in relation to a number of other factors, such as the patient’s diagnosis or medication. For example, MI may be overlooked within disorders for which these symptoms are seen as uncommon.
Additionally, NLP applications have some degree of inaccuracy, which may have introduced bias into the prevalence and IRR estimates. Although some validation of the applications was carried out in this study, it was based on a relatively small number of manual annotations by a single human annotator due to time and resource constraints. Validation could be improved by assessing a larger sample of documents, and using more than one annotator to permit estimation of inter-rater reliability.
Further, the outputs of the NLP applications are dependent on the terms that they are intended to identify. For example, the low prevalence of SD observed in this study may reflect somewhat limited terms identified by the NLP applications for SD. The applications showed reasonably accuracy in the identification of mentions similar to “sleep problems” and “insomnia,” but they did not identify other words or phrases that could also indicate issues with sleep, such as “somnolence” or unusual behaviors during sleep.
Finally, severity of MI and SD could not be assessed from the NLP applications used in this study. Although, ideally, MI and SD are routinely assessed in care, this may not be the case, and there is little standardization between clinical assessments and limited deployment of standardized measures routinely used to assess these features.
Future directions
The results of the current study highlight important avenues for future work. Foremost, interventional research may help to better understand and improve the effectiveness of transdiagnostic treatments (both pharmacological and psychosocial treatments) of MI and SD. For example, cognitive behavioral therapy (CBT) for insomnia delivered at hospital admission has been shown to considerably reduce insomnia and marginally improve psychological well-being after 2 weeks [Reference Sheaves, Freeman, Isham, McInerney, Nickless and Yu28]. Further work could aim to produce adaptations to CBT with the aim of improving MI in addition to sleep, and for delivery outside of acute care.
Many pharmacological treatments that target mood disturbances also have secondary effects that may exacerbate or improve symptoms of SD. For example, insomnia is among one of the most frequently reported adverse events of fluoxetine, while trazodone has been found to improve sleep symptoms in patients with depression plus insomnia [Reference DeMartinis and Winokur29]. Further research is needed to clarify which treatments may be most effective in persons with both MI and SD.
Future research should also seek to clarify factors that influence clinical outcomes, such as hospitalization, in individuals with MI and SD. Early interventions targeted at modifiable risk-factors may help to reduce complications and improve quality of life in persons with mental illness.
Finally, EHRs hold a wealth of clinically relevant information and NLP applications offer a novel method to examine these data. More work is needed to refine and validate NLP methods for eliciting symptoms from EHRs. Future research may also seek to establish whether the accuracy of the NLP applications could be improved by altering thresholds for the presence or absence of each feature. This study established the presence of MI based on one or more mentions of MI within documents within the first month of presentation. Future work may seek to determine if accuracy can be improved when, for example, mentions of the feature in two or more documents are required.
Given growing evidence that MI and SD are transdiagnostic features of psychiatric disorders, and their important impact on clinical outcomes and functioning, it is important they are assessed routinely in clinical care. Regular ongoing monitoring of mood and sleep are recommended for patients with mood and anxiety disorders [Reference Yatham, Kennedy, Parikh, Schaffer, Bond and Frey30]. Brief standardized assessments of these symptoms, such as those included on the True Colors remote monitoring platform (https://oxfordhealth.truecolours.nhs.uk) may be useful tools for health care providers. Evidence-based clinical practice guidelines for the assessment and treatment of sleep disorders are available [Reference Wilson, Anderson, Baldwin, Dijk, Espie and Espie31] to improve the standardization of assessment and care for patients.
Conclusions
The findings of this study suggest that MI and SD may contribute to the high cost of mental disorders, especially when they co-occur. MI and SD are present in a range of psychiatric disorders and associated with increased use of services and prescribed medications, and significantly increased risk of hospitalization. These findings have important implications for clinical practice. One possible reason for the poor outcomes associated with MI and SD may be that they are often overlooked during care. Both MI and SD are often seen as epiphenomena of the underlying disorder. However, growing evidence that they are transdiagnostic features and involved in the origins and maintenance of mental disorders implies that routine assessment and prompt and ongoing treatment may help to improve prognosis in individuals with a wide range of mental disorders.
Acknowledgments.
We would also like to acknowledge the work and support of the Oxford CRIS Team, Tanya Smith, CRIS Manager, Adam Pill, CRIS Academic Support and Information Analyst, Suzanne Fisher, CRIS Academic Support and Information Analyst, Lulu Kane CRIS Administrator. The views expressed are those of the authors and not necessarily those of the NHS, the NIHR or the Department of Health.
Financial Support
This research project was supported by the UK Clinical Record Interactive Search (UK-CRIS) system using data and systems of the NIHR Oxford Health Biomedical Research Center (BRC-1215-20005). J.G. is also supported by a Senior Investigator award (NF-SI-0514-10142). R.P. has received support from a Medical Research Council (MRC) Health Data Research UK Fellowship (MR/S003118/1) and a Starter Grant for Clinical Lecturers (SGL015/1020) supported by the Academy of Medical Sciences, The Wellcome Trust, MRC, British Heart Foundation, Arthritis Research UK, the Royal College of Physicians and Diabetes UK.
Conflicts of Interest
The authors declare no conflicts of interest.
Authorship Contributions.
K.M. devised the study aims and methods, conducted the analysis and wrote this document. Members of the CRIS network (including T.S.) assisted with obtaining and extracting the data. Members from the South London and Maudsley Biomedical Research Center (including R.P. and M.B.) performed the natural language processing. J.G. and K.E.A.S. provided advice at all stages of the project. All authors provided critical review and final approval of the manuscript.
Data Availability Statement
The data that support the findings of this study are available from NIHR Biomedical Research Center. Restrictions apply to the availability of these data, which were used under license for this study. Data are available from the authors with the permission of NIHR Biomedical Research Center.
Supplementary Materials
To view supplementary material for this article, please visit http://dx.doi.org/10.1192/j.eurpsy.2020.39.
Comments
No Comments have been published for this article.