An accumulating body of work has documented brain structure differences in individuals suffering from mental health conditions including psychosis, depression, and anxiety (Bas-Hoogendam et al., Reference Bas-Hoogendam, van Steenbergen, Tissier, Houwing-Duistermaat, Westenberg and van der Wee2018; Castagna et al., Reference Castagna, Roye, Calamia, Owens-French, Davis and Greening2018; Fraguas, Díaz-Caneja, Pina-Camacho, Janssen, & Arango, Reference Fraguas, Díaz-Caneja, Pina-Camacho, Janssen and Arango2016; Jeon, Mishra, Ouyang, Chen, & Huang, Reference Jeon, Mishra, Ouyang, Chen and Huang2015; Schmaal et al., Reference Schmaal, Hibar, Sämann, Hall, Baune, Jahanshad and Ikram2017). Much of this work has centered on adult populations (Canu et al., Reference Canu, Kostić, Agosta, Munjiza, Ferraro, Pesic and Filippi2015; Maglanoc et al., Reference Maglanoc, Kaufmann, Jonassen, Hilland, Beck, Landrø and Westlye2020; Schmaal et al., Reference Schmaal, Hibar, Sämann, Hall, Baune, Jahanshad and Ikram2017; Shepherd, Laurens, Matheson, Carr, & Green, Reference Shepherd, Laurens, Matheson, Carr and Green2012). Further, existing research has emphasized individuals meeting clinical criteria for different disorders (Canu et al., Reference Canu, Kostić, Agosta, Munjiza, Ferraro, Pesic and Filippi2015; Chiapponi et al., Reference Chiapponi, Piras, Fagioli, Piras, Caltagirone and Spalletta2013; Freitas-Ferrari et al., Reference Freitas-Ferrari, Hallak, Trzesniak, Santos Filho, Machado-de-Sousa, Chagas and Crippa2010; Frick et al., Reference Frick, Howner, Fischer, Eskildsen, Kristiansson and Furmark2013; Strawn et al., Reference Strawn, Wegman, Dominick, Swartz, Wehry, Patino and DelBello2014). More recently, dimensional conceptualizations of psychopathology have heralded an era of insights on mechanisms underlying mental illness vulnerability, offering insights on both transdiagnostic and specific factors (Krueger & Markon, Reference Krueger and Markon2011). Examining neural features during late childhood, while the brain is in the crux of significant gray matter reorganization and various forms of serious psychopathology are first emerging, could allow for a necessary perspective on overlapping and unique pathogenesis (Cicchetti & Rogosch, Reference Cicchetti and Rogosch1996; Gogtay et al., Reference Gogtay, Giedd, Lusk, Hayashi, Greenstein, Vaituzis and Toga2004; Lyall et al., Reference Lyall, Shi, Geng, Woolson, Li, Wang and Gilmore2015). Further, adopting a longitudinal perspective could add further nuance to understanding developmental processes during this period. As such, the present investigation aimed to (1) establish whether brain structure relates to psychosis, depressive, and anxiety symptomatology in late childhood, and (2) determine whether structural neural features could predict change trajectories over a 2-year period of psychosis, depressive, and anxiety symptomatology. Taken together, these efforts hold promise for informing early prevention and intervention efforts for mental illness and identifying vulnerability markers.
Depressive and anxiety disorders occur commonly in a significant proportion of adolescents, with rates estimated at 9.9% for anxiety disorders and 9.5% for depressive disorders in adolescents by age 16 (Costello, Mustillo, Erkanli, Keeler, & Angold, Reference Costello, Mustillo, Erkanli, Keeler and Angold2003). Psychotic-like experiences (PLEs), including unusual beliefs, suspiciousness, and perceptual abnormalities, can occur as early as childhood and are observed in 13–15% of children, while formal psychotic disorder onset typically occurs during late adolescence and early adulthood (Laurens et al., Reference Laurens, Hodgins, Maughan, Murray, Rutter and Taylor2007). After clinically impairing illness onset, confounds related to factors such as medication use and functional decline make it difficult to distill factors driving illness onset (Zhao et al., Reference Zhao, Chen, Zhang, Xiao, Shah, Zhu and Jia2017). As such, assessment prior to adolescence of associated symptoms or experiences on spectrums of symptomatology provides a promising alternative for identifying factors relating to disorder etiology prior to the typical age of onset for these disorders.
Dimensional models conceptualize mental disorders as delineating continuous underlying susceptibilities to experience psychopathology, assessing symptomatology on a spectrum of severity ranging from normative to more severe (Krueger & Markon, Reference Krueger and Markon2011). Identifying symptomatology dimensionally could aid in predicting vulnerable youth at greater risk of later developing more severe symptomatology during adolescence and young adulthood. For example, subthreshold depressive and anxiety symptoms have been shown to predict later disorder onset through adolescence (Costello et al., Reference Costello, Mustillo, Erkanli, Keeler and Angold2003). Evidence suggests vulnerability for developing depressive and psychotic disorders can emerge during childhood (Keyes, Gary, O'Malley, Hamilton, & Schulenberg, Reference Keyes, Gary, O'Malley, Hamilton and Schulenberg2019). PLEs have also been related to pathogenic factors observed in psychotic disorders (Kelleher & Cannon, Reference Kelleher and Cannon2011; Papanastasiou et al., Reference Papanastasiou, Mouchlianitis, Joyce, McGuire, Boussebaa, Banaschewski and Desrivières2020), and experiencing PLEs earlier in life has been linked to later risk for psychotic disorder onset (Kline et al., Reference Kline, Thompson, Bussell, Pitts, Reeves and Schiffman2014).
While the literature has harnessed structural magnetic resonance imaging (MRI) methodology to distinguish individuals with different DSM-5 diagnoses, these efforts have largely centered on adolescents and adults with an existing diagnosis (Carnevali et al., Reference Carnevali, Mancini, Koenig, Makovac, Watson, Meeten and Ottaviani2019; Forbes et al., Reference Forbes, Stefler, Velakoulis, Stuckey, Trudel, Eyre and Kisely2019; Frodl et al., Reference Frodl, Janowitz, Schmaal, Tozzi, Dobrowolny, Stein and Jahanshad2017; Molent et al., Reference Molent, Maggioni, Cecchetto, Garzitto, Piccin, Bonivento and Perna2018; Vieira et al., Reference Vieira, Gong, Pinaya, Scarpazza, Tognin, Crespo-Facorro and Scheepers2020). The existing literature on mental health vulnerability earlier in development, during childhood, has been less frequent, while having yielded critical insights. Childhood and pre-adolescence studies examining mental health vulnerability across different dimensions of symptomatology in the same sample, while adopting a longitudinal perspective, are lacking. The Adolescent Brain and Cognitive Development (ABCD) study provides an excellent opportunity for expanding our understanding, as the largest study to date on adolescent development (Casey et al., Reference Casey, Cannonier, Conley, Cohen, Barch, Heitzeg and Garavan2018). Existing investigations have begun to explore these questions, assessing high risk for depression and relations to gray matter volume (Pagliaccio, Alqueza, Marsh, & Auerbach, Reference Pagliaccio, Alqueza, Marsh and Auerbach2020), as well as gray matter volume with regards to dimensions of psychopathology in children (Durham et al., Reference Durham, Jeong, Moore, Dupont, Cardenas-Iniguez, Cui and Kaczkurkin2021). These studies have not examined different dimensions of psychopathology in the same sample while teasing out relations to cortical thickness and surface area more specifically; the perspective is crucial given distinct underlying processes and developmental trajectories that are not captured by volumetric measures alone (Tamnes et al., Reference Tamnes, Herting, Goddings, Meuwese, Blakemore, Dahl and Crone2017). Similarly, due to high comorbidity among mental disorders, understanding the dimensional presentation of different disorders within the same sample is critical (Kotov et al., Reference Kotov, Krueger, Watson, Cicero, Conway, DeYoung and Latzman2021). The approach could aid in distinguishing overlapping and distinct etiological and vulnerability factors (Cicchetti & Rogosch, Reference Cicchetti and Rogosch1996). Finally, symptom trajectories over time are lacking, rendering missing a nuanced developmental perspective of emerging vulnerability.
Childhood and pre-adolescence mark a dynamic period for neural development – by 9 years old, the brain has already reached 96% of its maximum size (Jeon et al., Reference Jeon, Mishra, Ouyang, Chen and Huang2015; Lyall et al., Reference Lyall, Shi, Geng, Woolson, Li, Wang and Gilmore2015; Tamnes et al., Reference Tamnes, Herting, Goddings, Meuwese, Blakemore, Dahl and Crone2017; Wierenga, Langen, Oranje, & Durston, Reference Wierenga, Langen, Oranje and Durston2014). As such, widespread grey matter volume decreases begin around puberty (Norbom et al., Reference Norbom, Ferschmann, Parker, Agartz, Andreassen, Paus and Tamnes2021). The developmental timing of volume, thickness, and surface area varies by cortical region, with cortical thickness and surface area developing independently of one another (Wierenga et al., Reference Wierenga, Langen, Oranje and Durston2014). For example, both cortical thickness and surface area show normative decreases throughout late childhood and adolescence, with cortical thinning happening at a much greater degree compared to surface area (Garcia, Kroenke, & Bayly, Reference Garcia, Kroenke and Bayly2018; Jeon et al., Reference Jeon, Mishra, Ouyang, Chen and Huang2015; Tamnes et al., Reference Tamnes, Herting, Goddings, Meuwese, Blakemore, Dahl and Crone2017; Wierenga et al., Reference Wierenga, Langen, Oranje and Durston2014). While surface area could reflect processes related to cortical folding and gyrification (Garcia et al., Reference Garcia, Kroenke and Bayly2018), cortical thickness could index developmental processes including synaptic pruning, cell shrinkage, apoptosis, and dendritic arborization (Jeon et al., Reference Jeon, Mishra, Ouyang, Chen and Huang2015; Tamnes et al., Reference Tamnes, Herting, Goddings, Meuwese, Blakemore, Dahl and Crone2017).
With total grey matter volume decreasing and white matter myelination increasing, cortical thinning and pruning process are particularly marked during adolescence (Tamnes et al., Reference Tamnes, Herting, Goddings, Meuwese, Blakemore, Dahl and Crone2017). As such, while existing studies have largely focused on gray matter volume metrics, measures related to gray matter cortical thickness and surface area could provide a unique understanding on developmental processes and underlying biological mechanisms (Jeon et al., Reference Jeon, Mishra, Ouyang, Chen and Huang2015; Lyall et al., Reference Lyall, Shi, Geng, Woolson, Li, Wang and Gilmore2015; Tamnes et al., Reference Tamnes, Herting, Goddings, Meuwese, Blakemore, Dahl and Crone2017; Wierenga et al., Reference Wierenga, Langen, Oranje and Durston2014). While other metrics such as white matter and network cohesion are at earlier stages of development during late childhood, gray matter features are in the crux of foundational developmental processes (Jeon et al., Reference Jeon, Mishra, Ouyang, Chen and Huang2015; Wierenga et al., Reference Wierenga, Langen, Oranje and Durston2014). As such, these metrics could provide insights into emerging vulnerability in the years prior to adolescence and young adulthood, when other contextual, interpersonal, and neurodevelopmental vulnerabilities typically emerge to drive formal illness onset.
The present exploratory study addressed questions related to mental health vulnerability for psychosis, depression, anxiety, and gray matter structure in childhood. The first aim was to relate PLEs, depressive, and anxiety symptoms to cortical thickness and area regions, and subcortical region volume. The second aim was to determine which cortical and subcortical regions would predict symptom trajectories over time. Taken together, the present investigation provides an opportunity for clarifying factors related to trajectories of early vulnerability for mental illness.
Method
Participants
The ABCD dataset release 3.0 includes children aged 9–11 years old across 22 centers in the USA (Barch et al., Reference Barch, Albaugh, Avenevoli, Chang, Clark, Glantz and Yurgelun-Todd2018). All centers obtained the parents' informed consent as well as the children's assent. Research procedures followed ethical guidelines laid out by respective Institutional Review Boards. The current sample used baseline data for participants who had available data for PLEs, depressive and anxiety symptoms. A total of 8645 had structural MRI data collected at baseline passing quality control procedures, along with available baseline symptoms and demographics data (see online Supplementary Material). Participants were interviewed at baseline, 1-year follow-up, and 2-year follow-up. Of the baseline sample, a subsample of 5632 had available symptoms data for the three timepoints. Trajectory analyses were conducted in this subgroup. As the ABCD study did not collect information on clinician-rated diagnoses, these were not included.
Measures
Structural MRI
Participants completed a high-resolution T1-weighted structural MRI scan (1 mm isotropic voxels) using scanners from GE Healthcare (Waukesha, Wisconsin), Philips Healthcare (Andover, Massachusetts), or Siemens Healthcare (Erlangen, Germany) (Casey et al., Reference Casey, Cannonier, Conley, Cohen, Barch, Heitzeg and Garavan2018). Structural MRI data were processed using FreeSurfer version 5.3.0 (http://surfer.nmr.mgh.harvard.edu/) (Fischl, Sereno, Tootell, & Dale, Reference Fischl, Sereno, Tootell and Dale1999) according to standard processing pipelines (Casey et al., Reference Casey, Cannonier, Conley, Cohen, Barch, Heitzeg and Garavan2018). Processing included removal of non-brain tissue, segmentation of gray and white matter structures (Fischl et al., Reference Fischl, Salat, Busa, Albert, Dieterich, Haselgrove and Klaveness2002) and cortical parcellation. All scan sessions underwent radiological review whereby scans with incidental findings were identified. Quality control for the structural images comprised visual inspection of T1 images and Free-Surfer outputs for quality (Hagler et al., Reference Hagler, Hatton, Cornejo, Makowski, Fair, Dick and Harms2019). Subjects whose scans failed inspection (due to severe artifacts or irregularities) were excluded. The Desikan-Killiany Atlas was used for cortical parcellation (see online Supplementary Material) (Hagler et al., Reference Hagler, Hatton, Cornejo, Makowski, Fair, Dick and Harms2019). For subcortical parcellation, the Aseg atlas was used (Fischl et al., Reference Fischl, Salat, Busa, Albert, Dieterich, Haselgrove and Klaveness2002).
PLEs
The Prodromal Questionnaire-Brief Child version was administered to youth to assess PLEs (Karcher et al., Reference Karcher, Barch, Avenevoli, Savill, Huber, Simon and Loewy2018). The 21-item self-report questionnaire was previously validated in the ABCD study sample. Participants were asked about specific PLEs that could be endorsed with a yes/no response. In addition, youth indicated whether there was distress related to endorsed symptoms on a Likert scale ranging from 1 to 5. PLE scores accounting for distress were calculated consistent with prior research, whereby total number of endorsed symptoms were weighted by the level of distress. A score of 0 indicated no endorsement, 1 indicated endorsement without distress, and 2–6 indicated endorsement with incremental distress levels (Karcher et al., Reference Karcher, Barch, Avenevoli, Savill, Huber, Simon and Loewy2018).
Depressive and anxiety symptoms
The Child Behavior Checklist (CBCL) was administered to parents of participants (Barch et al., Reference Barch, Albaugh, Avenevoli, Chang, Clark, Glantz and Yurgelun-Todd2018). The CBCL contains 113 question cores on a three-point Likert scale (with 0 representing ‘not true’, 1 representing ‘somewhat/sometimes’, and 2 indicating ‘very true/often’). DSM-5 oriented empirically based subscales were derived for anxiety and depressive symptoms (Achenbach, Dumenci, & Rescorla, Reference Achenbach, Dumenci and Rescorla2003). For the anxiety scale, scores ranged from 0 to 18, with items related to experiencing being dependent, not wanting to sleep alone, not leaving the home, having fears, being upset by separation, being nervous, having nightmares, fearing daycare/school, panicking, and worrying. For the depressive scale, scores ranged from 0 to 19, with items related to experiencing apathy, crying, not eating well, trouble sleeping, looking unhappy, overeating, being overtired, having little interest, sleeping little, being underactive, and being sad. Raw scores were used to extract slopes for change in symptoms over time. The CBCL has been successfully used for assessing anxiety (Wadsworth, Hudziak, Heath, & Achenbach, Reference Wadsworth, Hudziak, Heath and Achenbach2001) and depression (Wadsworth et al., Reference Wadsworth, Hudziak, Heath and Achenbach2001) in youth.
Data analysis
To account for clustering, demographic and socio-economic selection bias, the current study opted for three-level (site, family, individual) multi-level specifications (along with controlling for relevant demographic and socio-economic features including race, sex, and family income), rather than employing multilevel-weighting methods, as current evidence does not suggest that multilevel weighting methods improve the accuracy or precision of the model fit (Heeringa & Berglund, Reference Heeringa and Berglund2020). Results were visualized using R packages ggseg version 1.6.3, and ggseg3d version 1.6.3 (Mowinckel & Vidal-Piñeiro, Reference Mowinckel and Vidal-Piñeiro2020). The car package, version 3.0.11 was used to calculate variance inflation factor (VIF) to further assess for multicollinearity (Fox, Reference Fox J2019).
Statistical modeling of symptoms over time. Mixed-effect models were run using the nlme version 3.1.148 (Pinheiro et al., Reference Pinheiro, Bates, DebRoy, Sarkar, Heisterkamp, Van Willigen and Maintainer2021) package in R version 4.0.2 using R studio version 1.2.1335 (Team, 2018). Individual change paths over the three timepoints were calculated for PLEs, depressive and anxiety symptoms. Residual, individual change slope terms were extracted. Adjusted residual slopes were used as the outcome variable. Imputation was done using the MICE package version 3.13.0 in R to account for missing longitudinal data (Zhang, Reference Zhang2016). Results were mostly consistent with or without imputation; see online Supplementary Material for analyses without imputation.
Cortical area/thickness as predictors of baseline symptoms and symptom trajectory. Desikan-Killiany Atlas cortical regions (68 total, 34 regions with left and right hemisphere) were together used as predictors in a single mixed-effect model accounting for age, sex, and medication use (including antipsychotics, sedatives, serotonin selective reuptake inhibitors, stimulants, and mood stabilizers) as fixed effects, and family and site as random effects, with baseline PLE/depressive/anxiety symptoms and change trajectories for PLE/depressive/anxiety symptoms as outcome variables. Analyses were run such that one model included all the Desikan-Killiany-delineated regions as predictors. The single model controlled for all other brain regions, and different models conducted for area, thickness, and symptoms concerned distinct research questions. Raw symptoms data were log transformed. Prior to analyses, variables were converted to standardized units (z scores). Standardized brain metrics did not correlate highly with each other (r ranging from <0.001 to 0.6); as such, collinearity due to brain metric predictors was not a concern in the models. VIF ranged from 1.014 to 5.199, and remained <5 in 99.99% of cases (see online Supplementary Material).
Analyses were run for cortical thickness and surface area separately, given their earlier mentioned distinct developmental trajectories and underlying neural structural indicators. For analyses, regions were averaged across hemispheres. As an exploratory aim, sensitivity analyses explored whether bilateral region findings were present in only one or more hemispheres (see online Supplementary Material).
Subcortical volumes as predictors of baseline symptoms and symptom trajectory. Previous studies have already examined volume in baseline/non-longitudinal data (Durham et al., Reference Durham, Jeong, Moore, Dupont, Cardenas-Iniguez, Cui and Kaczkurkin2021). Given that thickness and area measures were not available for subcortical regions, volumetric measures were used for subcortical regions only. Aseg Atlas subcortical regions (7 total) were together used as predictors in a single mixed-effect model accounting for age, sex, family income, race, and medication use as fixed effects, and family and scanner serial number as random effects, with baseline PLE/depressive/anxiety symptoms as outcome variables (Tables 1–3).
Table 1. Demographic characteristics
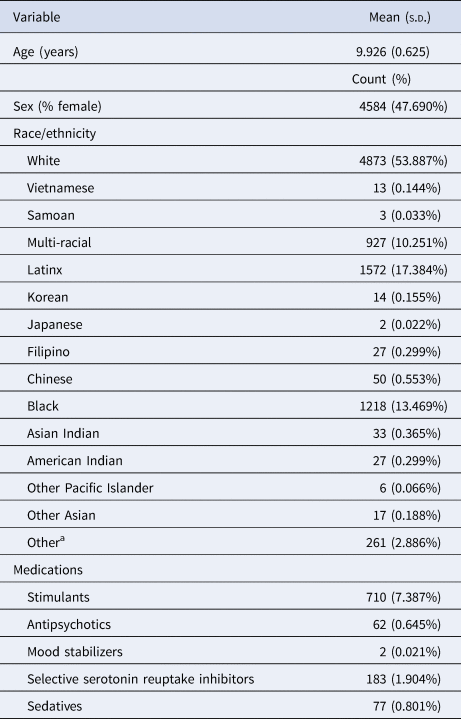
a Did not know, refused to answer, missing data.
Table 2. Descriptive statistics for baseline symptoms and symptom trajectories
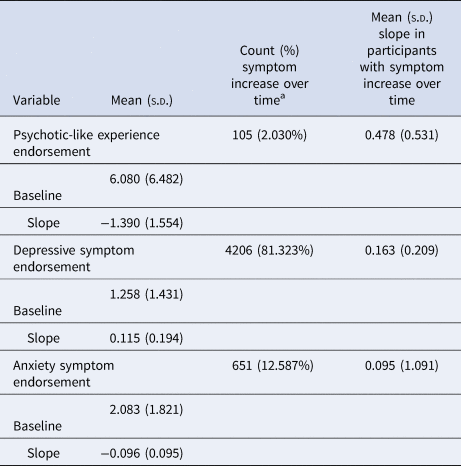
a Participants with a non-zero positive slope.
Table 3. Summary of regions that showed associations with baseline symptoms or symptom trajectories, while accounting for other cortical and subcortical regions

a Analysis timepoint.
b Direction of the association, ↑↓ indicates that as thickness/area/volume goes down, symptoms increase, while ↑↑ indicates that as thickness goes up, symptoms go up.
Results
A series of independent theories were tested in the present study: whether dimensions of symptoms (anxiety, depression, PLEs) would relate to baseline symptomatology, and whether baseline neutral structure would predict symptom trajectories over time (Armstrong, Reference Armstrong2014; Cabin & Mitchell, Reference Cabin and Mitchell2000; Fiedler, Kutzner, & Krueger, Reference Fiedler, Kutzner and Krueger2012). There are three distinct theoretically related tests (for anxiety, depression, and PLEs), which were separately predicted for baseline symptomatology as well as symptom trajectories. In addition to correcting for all cortical and subcortical regions within the same model, further correction for comparisons was conducted for each gray matter metric tested (cortical thickness, surface area, and subcortical volume) using Bonferroni thresholds for three tests of one similar hypothesis, resulting in a threshold of 0.016 (see Table 4).
Table 4. Summary of associations that passed Bonferroni correction
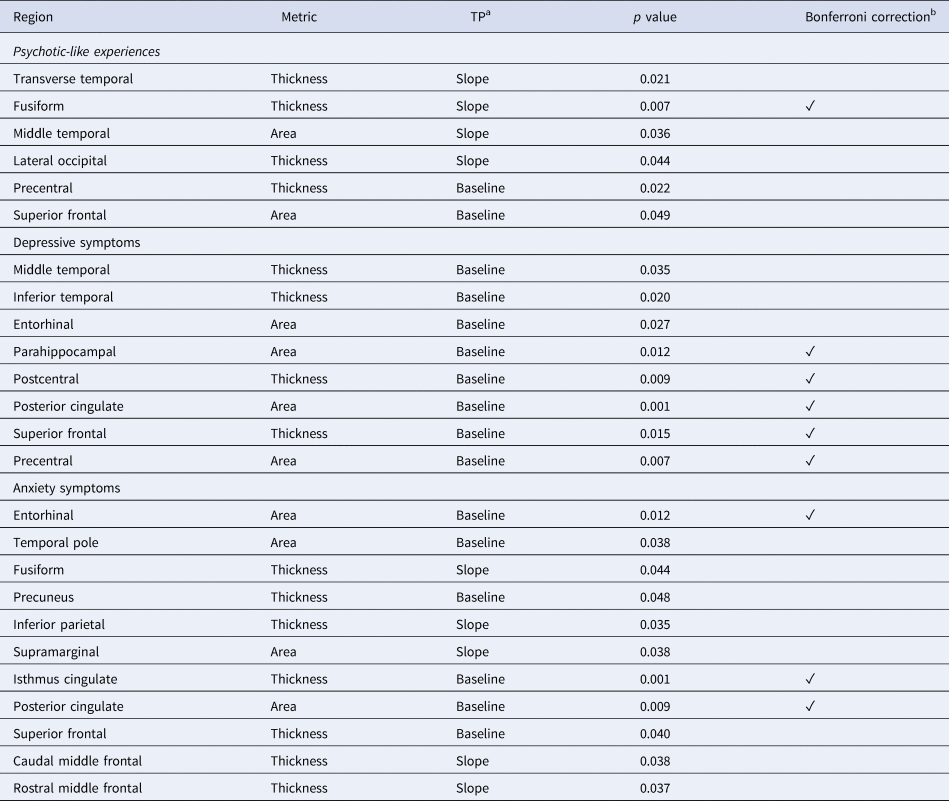
a Analysis timepoint.
b Threshold set at 0.0167, ✓ indicates the association passed Bonferroni correction.
The baseline sample and symptom trajectory subsample had differences in endorsement of baseline PLEs [those without follow-up data had higher baseline PLE endorsement, t(8643) = −2.552, p = 0.011, Cohen's d = 0.06], as well as demographic differences in age [those without follow-up data were younger, t(8295.680) = 33.599, p < 0.001, Cohen's d = 0.71]. These variables were controlled for in trajectory analyses.
PLEs and gray matter morphometry
Lower thickness/area in prefrontal regions including pre-central thickness (β = −0.046, 95% CI −0.086 to 0.007, t = −2.294, p = 0.022) and superior frontal area (β = −0.048, 95% CI −0.096 to −0.0001, t = −1.966, p = 0.049) related to increased baseline PLEs. With regards to symptom trajectories, lower fusiform (β = −0.0289, 95% CI −0.0499 to −0.008, t = −2.696, p = 0.007) and greater transverse temporal thickness (β = 0.0191, 95% CI 0.002–0.035, t = 2.318, p = 0.021) predicted increases in PLEs over time (Fig. 1). Notably, greater lateral occipital (β = 0.024, 95% CI 0.001–0.0480, t = 2.015, p = 0.044) thickness predicted increases in PLEs over time, which was not the case for depressive or anxiety symptoms. Lower middle temporal area (β = −0.029, 95% CI −0.057 to −0.002, t = −2.102, p = 0.036) also predicted increased PLEs over time (Fig. 2).
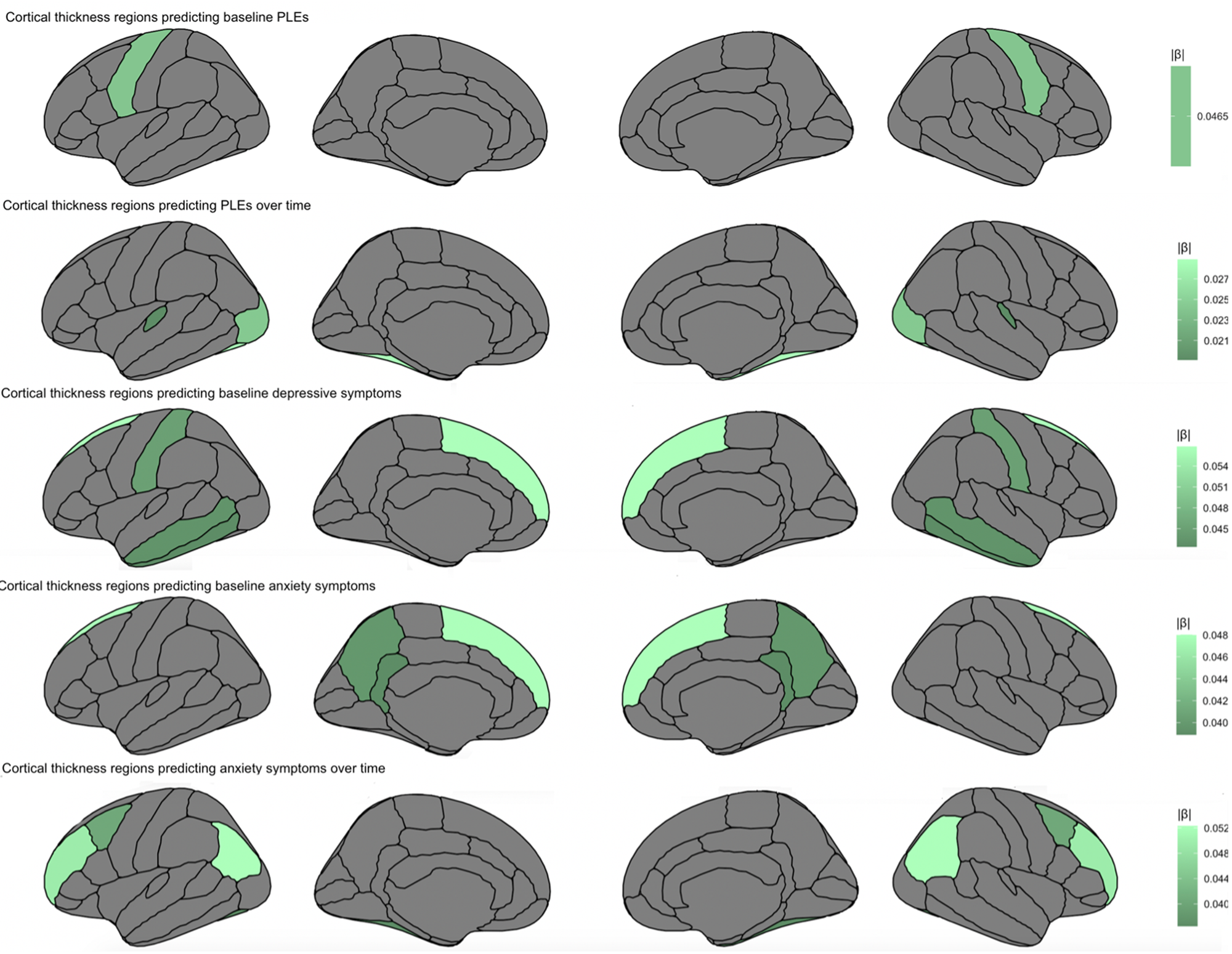
Fig. 1. Cortical thickness regions predicting baseline symptoms and symptom trajectories over a 2-year period, accounting for other cortical regions, age, sex, medication use, family income, race, family, and site.
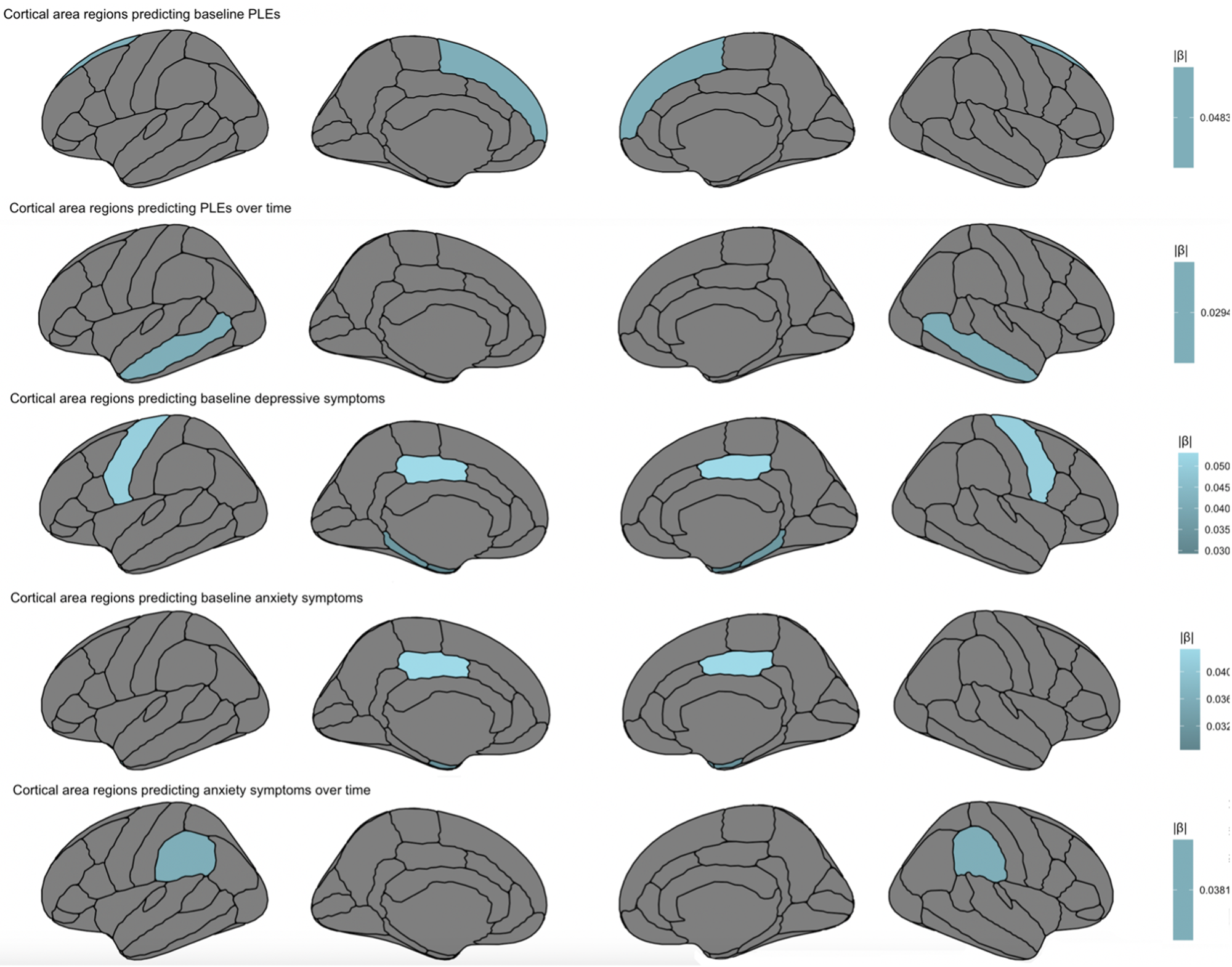
Fig. 2. Cortical area regions predicting baseline symptoms and symptom trajectories over a 2-year period, accounting for other cortical regions, age, sex, medication use, family income, race, family, and site.
Depressive symptoms and gray matter morphometry
In temporal regions, lower inferior temporal thickness (β = −0.042, 95% CI −0.078 to 0.007, t = −2.233, p = 0.020) and higher middle temporal thickness (β = 0.043, 95% CI −0.003 to 0.084, t = 2.106, p = 0.035) predicted higher baseline depressive symptoms. In turn, higher entorhinal area (β = −0.029, 95% CI 0.003–0.055, t = −2.217, p = 0.027) and lower parahippocampal area (β = −0.035, 95% CI −0.062 to −0.008, t = −2.525, p = 0.012) predicted greater baseline depressive symptoms. Within parietal regions, lower postcentral thickness (β = −0.045, t = −2.609, p = 0.009) predicted greater baseline depressive symptoms. Similar to PLEs and anxiety symptoms, lower superior frontal thickness (β = −0.057, 95% CI −0.103 to −0.011, t = −2.426, p = 0.015) predicted greater baseline depressive symptoms. Also within frontal lobe, lower precentral area (β = −0.050, 95% CI −0.087 to −0.014, t = −2.693, p = 0.007) predicted increased baseline depressive symptoms. Finally, for limbic regions, greater posterior cingulate area (β = 0.053, 95% CI 0.021–0.086, t = 3.208, p = 0.001) predicted greater baseline depressive symptoms (Fig. 3).
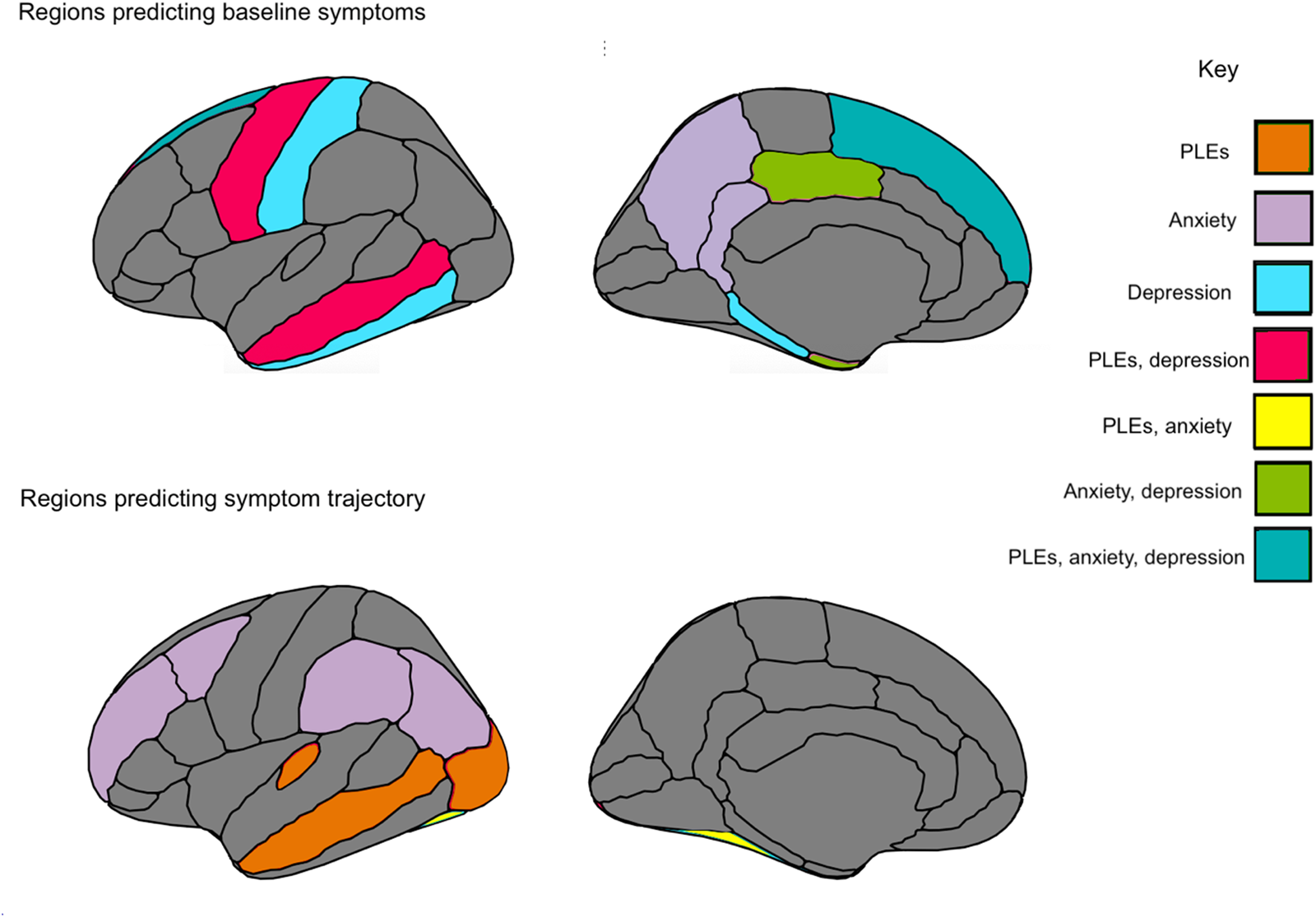
Fig. 3. Summary figure of regions for cortical thickness and surface area that related to symptomatology at baseline assessment, and across a 2-year period.
Anxiety symptoms and gray matter morphometry
Several parietal regions related uniquely to anxiety symptoms, including greater precuneus thickness (β = 0.039, 95% CI 0.003–0.079, t = 1.977, p = 0.048) predicting higher baseline anxiety symptoms, and greater supramarginal area (β = 0.038, 95% CI 0.002–0.074, t = 2.082, p = 0.038) predicting increased anxiety symptoms over time, with lower inferior parietal thickness (β = −0.052, 95% CI −0.101 to −0.004, t = −2.113, p = 0.035) predicting increased anxiety symptoms over time. Within temporal lobe, similar to PLEs, lower fusiform thickness (β = −0.037, 95% CI −0.073 to −0.002, t = −2.019, p = 0.044) predicted increased anxiety symptoms over time. Increased entorhinal area (β = 0.031, 95% CI 0.005–0.057, t = 2.333, p = 0.012) predicted increased anxiety symptoms at baseline, as was observed with depressive symptoms. Finally, lower temporal pole area (β = −0.028, 95% CI −0.055 to −0.001, t = −2.069, p = 0.038) uniquely predicted greater anxiety symptoms at baseline (Fig. 4).
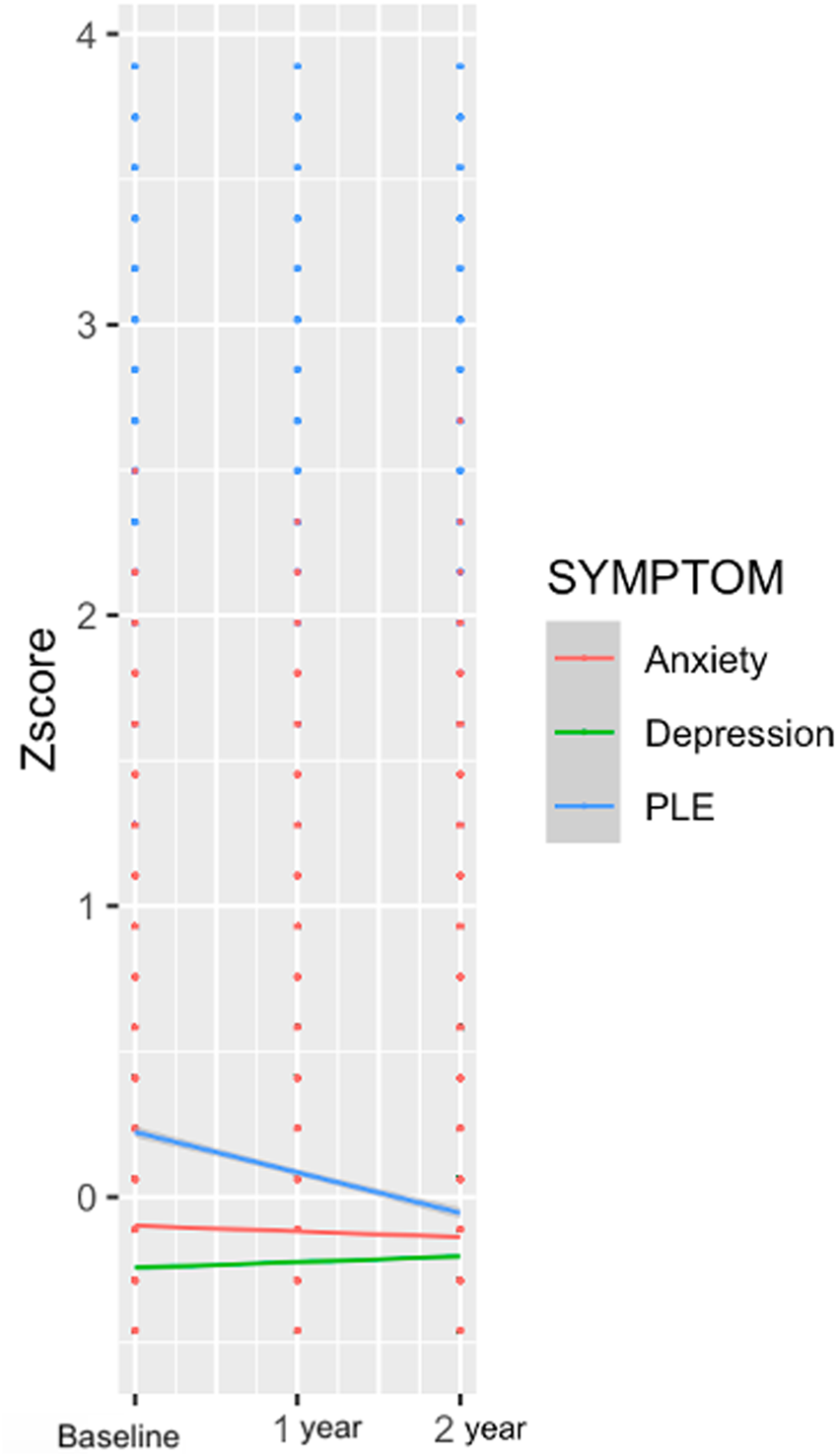
Fig. 4. Symptom trajectories over a 2-year period for PLEs, depressive symptoms, and anxiety symptoms.
Within limbic lobe, higher isthmus cingulate (β = 0.039, 95% CI 0.015–0.062, t = 3.254, p = 0.001) thickness predicted greater baseline anxiety symptoms, as did greater posterior cingulate (β = 0.043, 95% CI 0.012–0.076, t = 2.607, p = 0.009) area. Within prefrontal regions, consistent with depressive symptoms and PLEs, lower superior frontal thickness (β = −0.048, 95% CI −0.094 to −0.002, t = −2.040, p = 0.040) predicted greater baseline anxiety symptoms. Unique to anxiety symptoms, greater caudal middle frontal thickness (β = −0.040, 95% CI 0.0001–0.076, t = 2.075, p = 0.038) and lower rostral middle frontal thickness (β = −0.050, 95% CI −0.095 to −0.002, t = −2.094, p = 0.037) predicted increased anxiety symptoms over time (Table 3).
Discussion
Thickness and area in temporal regions related to symptomatology across PLEs, depressive and anxiety symptoms, offering putative childhood transdiagnostic predictors or neural vulnerability factors (Figure 3). There was also evidence of specificity. PLE symptom trajectory was predicted by lateral occipital thickness, as well as by transverse temporal thickness. With regards to depressive symptoms, symptoms were uniquely predicted by regions implicated in memory recall, including parahippocampal and interior temporal thickness, while both depressive and anxiety symptoms were predicted by limbic cingulate regions implicated in affective and reward processing. Finally, with regards to anxiety symptoms, symptom trajectories were uniquely predicted by parietal regions involved in memory, perception, and multisensory integration; regions included precuneus and inferior parietal thickness, and supramarginal area. The study builds on the broader literature (Ducharme et al., Reference Ducharme, Albaugh, Hudziak, Botteron, Nguyen, Truong and Byars2014; Durham et al., Reference Durham, Jeong, Moore, Dupont, Cardenas-Iniguez, Cui and Kaczkurkin2021; Merz, He, & Noble, Reference Merz, He and Noble2018; Newman et al., Reference Newman, Thompson, Bartsch, Hagler, Chen, Brown and Libiger2016; Norbom et al., Reference Norbom, Ferschmann, Parker, Agartz, Andreassen, Paus and Tamnes2021), contributing additional evidence that vulnerability to psychopathology is observable along with relations to neural structure as early as childhood. Results have the potential toward identifying emergent vulnerability, offering targets for early prevention and intervention.
The current study assessed symptomatology over time and relations to baseline gray matter cortical thickness/surface area and subcortical volume. The whole-brain approach of accounting for all cortical and subcortical regions in the same models allowed for assessing regional effects over and above other cortical and subcortical regions. Notably, superior frontal thickness and area related to PLE, depression, and anxiety symptoms at baseline. In addition, temporal regions predicted symptom trajectories over time, with middle temporal thickness and area relating to PLEs and depressive symptoms, and fusiform thickness relating to PLE and anxiety symptom trajectories across time (Table 2). Perhaps these regions could serve as transdiagnostic vulnerability markers for psychopathology as early as childhood (Beard & Björgvinsson, Reference Beard and Björgvinsson2013). Results could represent common vulnerabilities for emerging psychopathology across a 2-year period preceding the tumultuous adolescence period, where further developmental, social, and biological factors further compound vulnerability for mental illness (Cohen, Andrews, Davis, & Rudolph, Reference Cohen, Andrews, Davis and Rudolph2018; Del Re et al., Reference Del Re, Stone, Bouix, Seitz, Zeng, Guliano and Lyall2021).
PLEs
Consistent with predictions, and with a recent investigation on adolescents at clinical high risk for psychosis, PLEs related broadly to thickness across frontal, temporal, and occipital regions, which was not the case with surface area (Del Re et al., Reference Del Re, Stone, Bouix, Seitz, Zeng, Guliano and Lyall2021). Associations being more prevalent with thickness could reflect vulnerability in related processes, including synaptic pruning, apoptosis, cell shrinkage, and dendritic arborization (Lyall et al., Reference Lyall, Shi, Geng, Woolson, Li, Wang and Gilmore2015). Specifically, lateral occipital thickness predicted PLE trajectory (which was not the case with anxiety or depressive symptoms), widely consistent with research on youth at clinical high risk for psychosis (Sugranyes et al., Reference Sugranyes, de la Serna, Ilzarbe, Pariente, Borras, Romero and Bernardo2020; Ziermans et al., Reference Ziermans, Schothorst, Schnack, Koolschijn, Kahn, van Engeland and Durston2012), adults with PLEs (Fonville et al., Reference Fonville, Drakesmith, Zammit, Lewis, Jones and David2019) and early-onset psychosis (Fraguas et al., Reference Fraguas, Díaz-Caneja, Pina-Camacho, Janssen and Arango2016). PLEs were predicted by regions implicated in language and sensory processing, including transverse temporal, fusiform, medial, and superior temporal regions (Cone, Burman, Bitan, Bolger, & Booth, Reference Cone, Burman, Bitan, Bolger and Booth2008). Of note, precentral thickness predicted change in PLEs over time, with underlying functions relating to motor control, gestures, and decision-making, all critically and etiologically relevant to psychosis pathogenesis (Choudhury et al., Reference Choudhury, Steines, Nagels, Riedl, Kircher and Straube2021; Walther, Mittal, Stegmayer, & Bohlhalter, Reference Walther, Mittal, Stegmayer and Bohlhalter2020). The precentral region also predicted depressive symptomatology at baseline, supporting emerging research of motor dysfunction as a transdiagnostic vulnerability marker for both depressive and psychotic disorders (Damme et al., Reference Damme, Park, Vargas, Walther, Shankman and Mittal2021).
Depressive symptomatology
Within the limbic lobe, the posterior cingulate area predicted depressive symptoms, which was also the case with anxiety symptoms. Findings extend observations in the broader adult and adolescent literature involving limbic regions, with underlying functions related to affective processing, in depressive symptomatology (Bora, Fornito, Pantelis, & Yücel, Reference Bora, Fornito, Pantelis and Yücel2012; Hubachek et al., Reference Hubachek, Botdorf, Riggins, Leong, Klein and Dougherty2021; Jaworska et al., Reference Jaworska, Yücel, Courtright, MacMaster, Sembo and MacQueen2016; Wehry et al., Reference Wehry, McNamara, Adler, Eliassen, Croarkin, Cerullo and Strawn2015). Superior frontal thickness related to increased baseline depressive symptoms, consistent with investigations with diagnosed adolescents and adults (Suh et al., Reference Suh, Schneider, Minuzzi, MacQueen, Strother, Kennedy and Frey2019; Wise et al., Reference Wise, Radua, Via, Cardoner, Abe, Adams and Périco2017), as well as cross-sectional and longitudinal studies including children and adolescents (Bos, Peters, van de Kamp, Crone, & Tamnes, Reference Bos, Peters, van de Kamp, Crone and Tamnes2018; Wehry et al., Reference Wehry, McNamara, Adler, Eliassen, Croarkin, Cerullo and Strawn2015). With regards to depressive symptoms, there was evidence of specificity with regards to associations within parahippocampal and inferior temporal regions that were not observed for PLEs or anxiety symptoms. Middle temporal regions involved in memory processing and encoding predicted depressive symptoms, including parahippocampal and entorhinal area, with primary projections to the hippocampus, along with middle temporal thickness, consistent with theories of depression and endophenotypes related to memory and recall (Goldstein & Klein, Reference Goldstein and Klein2014), and with previous neuroimaging findings (Bora et al., Reference Bora, Fornito, Pantelis and Yücel2012; Hubachek, Reference Hubachek2021; Jenkins et al., Reference Jenkins, Chiang, Vause, Hoffer, Alpert, Parrish and Wang2020).
Anxiety symptomatology
Anxiety symptoms related to a number of regions relating to memory, multisensory integration, and perception (Newman et al., Reference Newman, Thompson, Bartsch, Hagler, Chen, Brown and Libiger2016). Notably, multiple parietal regions predicted symptom trajectory, which was not the case for depressive symptoms or PLEs. Higher supramarginal area and lower inferior parietal thickness predicted increased anxiety symptom endorsement over time. Results are consistent with GAD adult studies, extending these findings to sub-clinical symptomatology in children (Makovac et al., Reference Makovac, Meeten, Watson, Garfinkel, Critchley and Ottaviani2016; Molent et al., Reference Molent, Maggioni, Cecchetto, Garzitto, Piccin, Bonivento and Perna2018). Greater precuneus thickness also predicted higher baseline anxiety, consistent with a study in adults with SAD (Syal et al., Reference Syal, Hattingh, Fouché, Spottiswoode, Carey, Lochner and Stein2012), a study of volume in adolescents diagnosed with GAD (Strawn et al., Reference Strawn, Wegman, Dominick, Swartz, Wehry, Patino and DelBello2014), and others of gyrification and volume in adults with GAD (Molent et al., Reference Molent, Maggioni, Cecchetto, Garzitto, Piccin, Bonivento and Perna2018).
Within th temporal lobe, entorhinal and temporal pole area predicted baseline anxiety symptoms, while lower fusiform thickness predicted greater anxiety symptom endorsement over time; results are consistent with a broader literature (Couvy-Duchesne et al., Reference Couvy-Duchesne, Strike, de Zubicaray, McMahon, Thompson, Hickie and Wright2018), including adults diagnosed with GAD (Molent et al., Reference Molent, Maggioni, Cecchetto, Garzitto, Piccin, Bonivento and Perna2018) and patients with SAD (Syal et al., Reference Syal, Hattingh, Fouché, Spottiswoode, Carey, Lochner and Stein2012). Finally, within the limbic lobe, cingulate regions are related to anxiety symptoms, with isthmus cingulate thickness uniquely relating to anxiety (and not depressive symptoms or PLEs). Greater isthmus cingulate thickness predicted higher anxiety symptoms at baseline, as was found in a study with adults diagnosed with GAD (Carnevali et al., Reference Carnevali, Mancini, Koenig, Makovac, Watson, Meeten and Ottaviani2019). Additionally, higher posterior cingulate thickness predicted higher anxiety symptoms, consistent with a study on adolescents diagnosed with GAD (Strawn et al., Reference Strawn, Wehry, Chu, Adler, Eliassen, Cerullo and DelBello2013).
Future directions and limitations
Study findings have to bear for conceptualizations of multifinality, finding some evidence of processes contributing to divergence in outcomes given common origins (transdiagnostic vulnerability markers) (Cicchetti & Rogosch, Reference Cicchetti and Rogosch1996). A growing literature has found evidence for a ‘common’ vulnerability emerging during childhood, theorizing that going into adolescence, structural neurodevelopmental changes and environmental factors ultimately determine distinct trajectories with regards to psychopathology (Cohen et al., Reference Cohen, Andrews, Davis and Rudolph2018). Others have additionally found that even among those that exhibit a common vulnerability, the unique components of anxiety and depression grow independently over time (Conway, Zinbarg, Mineka, & Craske, Reference Conway, Zinbarg, Mineka and Craske2017). Current results are a starting point with regards to conceptualizations of features predicting symptom trajectories in the critical years prior to typical disorder onset during adolescence and early adulthood. Future studies delineating orthogonal and general psychopathology factors and relations to neural structure longitudinally would provide further insight into common v. unique neural vulnerability. Similarly, results lend support to HiTOP and dimensional models of psychopathology, affirming the utility of assessing symptomatology on a spectrum (Kotov et al., Reference Kotov, Krueger, Watson, Cicero, Conway, DeYoung and Latzman2021; Krueger & Markon, Reference Krueger and Markon2011). The identification of neural markers of vulnerability is also in line with RDoC aims of identifying unique and transdiagnostic markers of vulnerability at multiple levels of analysis, including parent and youth-reported symptomatology and brain metrics (Casey, Oliveri, & Insel, Reference Casey, Oliveri and Insel2014).
Notably, anxiety and depressive symptoms did not relate to specific subcortical structures (over and above other subcortical regions), countering a wide literature finding relations with subcortical regions implicated in affect regulation and threat sensitivity (Bos et al., Reference Bos, Peters, van de Kamp, Crone and Tamnes2018; Kaczkurkin et al., Reference Kaczkurkin, Park, Sotiras, Moore, Calkins, Cieslak and Cui2019; Kribakaran, Danese, Bromis, Kempton, & Gee, Reference Kribakaran, Danese, Bromis, Kempton and Gee2020; Taylor et al., Reference Taylor, Eastman, Frenzel, Embury, Wang, Stephen and Wilson2020). Future studies incorporating more timepoints, a greater variety of symptomatology, and more diverse samples will be helpful to clarify future understanding. With regards to PLEs, relations between symptoms and prefrontal regions were localized to the precentral region, which is partially inconsistent with specificity theories of prefrontal dysfunction in schizophrenia, which posit a broader dysfunction across the frontal lobe (Callicott et al., Reference Callicott, Mattay, Verchinski, Marenco, Egan and Weinberger2003; Muller, Reference Muller1985). Future studies examining the emergence of dysfunction relative to stages of neurodevelopment and symptom severity will further inform these questions.
Study observations represent a promising first step in identifying key regions that could have etiological relevance to certain symptom dimensions. The present investigation did not collect information on clinician-rated diagnostic categories, and this is a critical future direction. In addition, studies enriched for a greater proportion of individuals diagnosed with mental disorders would provide a greater understanding of more severe symptom and brain structure manifestation (especially given that restricted range in the current study could have impacted the magnitude of effects (Figure 4)). Future studies expanding beyond this study's age range across the life span would much strengthen the understanding of neurodevelopmental differences and contributors to vulnerability across the lifetime. Of note, especially given the small effect sizes observed, it will be critical to establish whether effects are clinically meaningful by exploring whether the altered structure in these regions maps on to other functional and social dysfunction related to symptomatology. Further, the current study was exploratory in its aim of determining gray matter morphometry features that could relate to domains of symptomatology. Future investigations could build on results with more specific hypotheses based on specific neural regions, allowing for more targeted correction criteria.
Supplementary material
The supplementary material for this article can be found at https://doi.org/10.1017/S0033291721005304.
Acknowledgements
Data used in the preparation of this article were obtained from the Adolescent Brain Cognitive Development (ABCD) Study (https://abcdstudy.org), held in the NIMH Data Archive (NDA). This is a multisite, longitudinal study designed to recruit more than 10 000 children age 9–10 and follow them over 10 years into early adulthood. The ABCD Study is supported by the National Institutes of Health and additional federal partners under award numbers U01DA041022, U01DA041028, U01DA041048, U01DA041089, U01DA041106, U01DA041117, U01DA041120, U01DA041134, U01DA041148, U01DA041156, U01DA041174, U24DA041123, U24DA041147, U01DA041093, and U01DA041025. A full list of supporters is available at https://abcdstudy.org/federal-partners.html. A listing of participating sites and a complete listing of the study investigators can be found at https://abcdstudy.org/Consortium_Members.pdf. ABCD consortium investigators designed and implemented the study and/or provided data but did not necessarily participate in the analysis or writing of this report. This manuscript reflects the views of the authors and may not reflect the opinions or views of the NIH or ABCD consortium investigators. The ABCD data repository grows and changes over time. The ABCD data used in this report came from [NIMH Data Archive Digital Object Identifier (DOI) 10.15154/1506121]. DOIs can be found at https://nda.nih.gov/general-query.html?q=query=studies%20~and~%20orderBy=id%20~and~%20orderDirection=Ascending.
Financial support
The ABCD Study is supported by the National Institutes of Health and additional federal partners under award numbers U01DA041022, U01DA041028, U01DA041048, U01DA041089, U01DA041106, U01DA041117, U01DA041120, U01DA041134, U01DA041148, U01DA041156, U01DA041174, U24DA041123, U24DA041147, U01DA041093, and U01DA041025. The research reported in this manuscript was also supported by the National Institute of Mental Health of the National Institutes of Health under Award Number F31MH119776 (T.V.). The content is solely the responsibility of the authors and does not necessarily represent the official views of the National Institutes of Health.
Conflict of interest
None.
Ethical standards
The authors assert that all procedures contributing to this work comply with the ethical standards of the relevant national and institutional committees on human experimentation and with the Helsinki Declaration of 1975, as revised in 2008. The authors assert that all procedures contributing to this work comply with the ethical standards of the relevant national and institutional guides on the care and use of laboratory animals.