Introduction
Cystic fibrosis (CF) is the most common inherited, life-limiting condition in Caucasians. In 2021, more than 10,900 people were living with CF in the UK (1). Current estimates indicate that the median predicted survival for a child born with CF is 53.3 years (1). Whilst CF is a multisystem disorder, the upper and lower airways and digestive system are most commonly affected (Reference Davies, Alton and Bush2). Amongst other problems, people with CF are susceptible to bacterial lung infections and reduced lung function, which adversely impact on survival and health-related quality of life (HRQoL) (Reference Emerson, Rosenfeld and McNamara3;Reference Mohindru, Turner and Sach4). People with CF require ongoing preventative medications which are intended to preserve lung function and reduce the incidence of pulmonary exacerbations (Reference Döring, Flume and Heijerman5). Most adults with CF self-manage their disease through a regimen of complex and time-consuming treatments that include inhaled antibiotic and mucolytic therapies delivered through a nebulizer (1).
As with many chronic diseases, adherence to inhaled therapies in people with CF is low. Based on medication possession ratio (MPR) data in 3,287 people with CF aged ≥6 years in the USA, adherence to preventive nebulized CF treatments has been estimated at 48 percent (Reference Quittner, Zhang and Marynchenko6). UK data using chipped nebulizers brought to the clinic to be downloaded suggest a lower median adherence rate of 36 percent (Reference Daniels, Goodacre and Sutton7). More recent analyses which take account of patients who do not readily bring nebulizers to the clinic suggest adherence levels closer to 30 percent (Reference Hoo, Curley and Walters8). Research has shown that poor adherence is a predictor of exacerbations which require rescue therapy (intravenous [IV] antibiotic therapies) (Reference Quittner, Zhang and Marynchenko6;Reference Eakin, Bilderback and Boyle9;Reference Briesacher, Quittner and Saiman10). Alongside negative impacts on patient health, poor adherence to preventative therapies has further economic implications for health services due to the wastage of prescribed medicines and increased requirements for inpatient rescue IV antibiotics. In 2012, the total UK spend for CF was estimated at GBP 100 million (EUR 104 million, based on 2021 Purchasing Power Parities for the European Union and the UK), of which GBP 30 million (EUR 31.17 million) was spent on preventative inhaled antibiotics and mucolytics. Despite high expenditures on preventative therapies, CF patients received 93,455 days of treatment with IV antibiotics in the hospital at an estimated cost of GBP 27 million (EUR 28.05 million) (pers. commun.: Dr Paul McManus, Lead Pharmacist for Specialised Services, South Yorkshire and Bassetlaw, NHS England; Dr Stephanie McNeil, Chief Statistician, CF Registry). Improving adherence to preventative therapies may allow some of these costs to be avoided.
The wider ACtiF research programme was initiated in 2015 with the overall aim of developing and evaluating a novel intervention to support adherence to treatment in people with CF (Reference Wildman, O’Cathain and Hind11). As part of this research programme, we undertook a randomized controlled trial (RCT) to assess the effectiveness of a novel digital platform and behavior change intervention versus usual care in adults with CF who were taking inhaled antibiotic and/or mucolytic therapies (Reference Wildman, O’Cathain and Maguire12). The primary outcome was the adjusted incidence rate ratio (IRR) of pulmonary exacerbations meeting the modified Fuchs’ criteria over a 12-month follow-up period (Reference Ratjen, Durham and Navratil13). Secondary outcomes included between-group changes in adherence, forced expiratory volume in the first second percent predicted (FEV1% pred.), and body mass index (BMI). The intervention evaluated within the trial – the CFHealthHub (CFHH) adherence intervention – comprised a web platform and app which displayed graphs and tables of objectively measured nebulizer adherence and included modules of behavior change techniques designed to increase motivation for adherence, to address the capability and opportunity barriers and to build habits for treatment-taking and an intervention manual including procedures and worksheets for delivery by a health professional. Participants in the intervention group received six face-to-face sessions over the first 12 weeks, with phased reviews delivered either face-to-face or by telephone every 12 weeks thereafter, or every 6 weeks thereafter for participants with objectively measured adherence of less than 25 percent. Participants with high adherence (>80 percent) during the baseline period of the study received two sessions with phased reviews every 12 weeks thereafter. Additional blocks of sessions were offered when the participant requested further support, the participant’s adherence was reduced by 20 percent over a 4-week period, or when the participant received IV antibiotics for an exacerbation. Participants were given eTrack data-logging controllers for their eFlow nebulizers which sent time- and date-stamped data to a 2net Hub (Capsule Technologies, San Diego, CA) for accurate recording of inhalation and adherence calculation. Further details of the intervention, as described using the Template for Intervention Description and Replication (TIDieR) checklist, are reported in Wildman et al. (Reference Wildman, O’Cathain and Maguire12). Control arm participants used eTrack data-logging controllers for adherence data collection, but did not have access to CFHH, behavioral change tools or content. The trial found that the intervention was associated with small but non-statistically significant improvements in exacerbations and lung function, whilst objectively measured adherence to inhaled medication sustained over 12 months was higher for the intervention group, and patients had lower perceived CF treatment burden, increased patient activation and increased BMI. Further details of the trial and its results can be found in Wildman et al. (Reference Wildman, O’Cathain and Maguire12).
This paper presents a model-based cost-effectiveness analysis which assesses whether the CFHH adherence intervention represents good value for money for the National Health Service (NHS) in the UK.
Methods
Economic Analysis Scope
We developed a health economic model to assess the cost-effectiveness of the CFHH adherence intervention versus usual care (no adherence intervention) for adults with diagnosed CF who are taking inhaled mucolytics and/or antibiotics. The economic analysis was undertaken from the perspective of the UK NHS and Personal Social Services (PSS) over a lifetime horizon. Cost-effectiveness was expressed in terms of the incremental cost per quality-adjusted life-year (QALY) gained. Health outcomes and costs were discounted at a rate of 3.5 percent per annum. Costs were valued at 2021/22 prices.
Model Structure and Assumptions
We developed a cohort-level state transition model based on the previous analyses used to inform the National Institute for Health and Care Excellence (NICE) appraisal of inhaled dry powder antibiotics for Pseudomonas aeruginosa lung infections and a pre-trial analysis of the CFHH adherence intervention (Reference Tappenden, Harnan and Uttley14;Reference Tappenden, Sadler and Wildman15). The model includes five health states: (1) FEV1 ≥ 70% pred.; (Reference Davies, Alton and Bush2) FEV1 40–69% pred.; (Reference Emerson, Rosenfeld and McNamara3) FEV1 < 40% pred.; (Reference Mohindru, Turner and Sach4) post-transplant; and; (Reference Döring, Flume and Heijerman5) death (Figure 1). During each model cycle, patients may remain in their current health state, transition to an improved or worsened health state or die. A small proportion of patients in the worst lung function state (FEV1 < 40% pred.) may undergo lung (or heart and lung) transplantation and do not receive subsequent nebulized treatment. HRQoL is defined according to FEV1% pred. stratum and transplant history, with a disutility applied to account for time spent receiving IV antibiotics at home or in hospital. The model uses a 12-month cycle length over 70 cycles; half-cycle correction is applied to account for the timing of events. Total QALYs are calculated as the sojourn time in each state weighted by state-specific utility scores, minus any IV-related QALY losses. The model includes costs associated with: (1) the CFHH adherence intervention; (Reference Davies, Alton and Bush2) health state resource use; (Reference Emerson, Rosenfeld and McNamara3) IV treatments (including inpatient stays, IV drugs, and consumables); (Reference Mohindru, Turner and Sach4) nebulizer devices; (Reference Döring, Flume and Heijerman5) nebulized therapies, and (Reference Quittner, Zhang and Marynchenko6) transplantation.
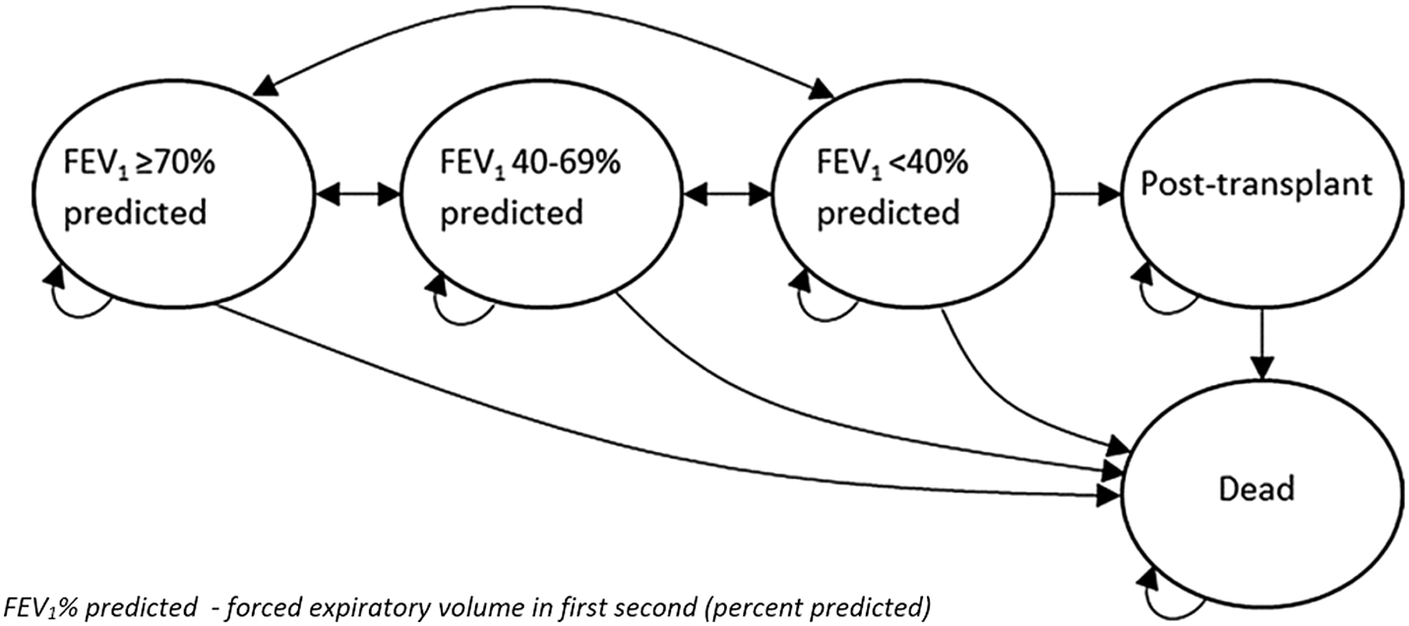
Figure 1. Model structure.
Note: Reproduced with permission from Wildman et al. (Reference Wildman, O’Cathain and Hind11). Published by NIHR Journals Library. This is an Open Access article distribution in accordance with the terms of the Creative Commons Attribution (CC BY 4.0) license, which permits others to distribute, remix, adapt and build upon this work, for commercial use, provided the original work is properly cited. See https://creativecommons.org/licenses/by/4.0/.
The model employs the following assumptions:
-
• At model entry, patients are assumed to be 30 years old (Reference Wildman, O’Cathain and Maguire12).
-
• Patients in each FEV1% pred. stratum can progress/regress to any other FEV1% pred. stratum during any model cycle.
-
• Patients who undergo transplantation cannot transition to other alive states.
-
• Mortality risk, exacerbation frequency, and HRQoL are conditional on the patient’s health state. Additional health losses are applied to patients experiencing exacerbations.
-
• The impact of the CFHH adherence intervention is assumed to apply through two mechanisms: (i) risk ratios (RRs) of switching FEV1% pred. strata in a given cycle; (ii) an IRR for the number of IV days incurred.
-
○ Treatment effects and costs related to the intervention are assumed to apply only in the years in which the intervention is given. The base case analysis assumes a treatment effect duration of 10 years; alternative durations are assessed in scenario analyses.
-
○ The intervention does not directly impact on the probability of undergoing transplantation or death.
-
-
• The costs of inhaled therapies are independent of adherence to those therapies (i.e., a patient who is more adherent does not receive more prescribed therapy).
Evidence Used to Inform the Model’s Parameters
The model parameters were informed by the CFHH trial (Reference Wildman, O’Cathain and Maguire12;Reference Wildman16), the CF Registry (17;18), other routine data (19), routine costing sources (20–22), published literature (Reference Anyanwu, McGuire and Rogers23–Reference Keogh, Szczesniak and Taylor-Robinson25), and expert opinion. The transition probabilities applied in the model are shown in Table 1; all other model parameters are presented in Table 2.
Table 1. Annual transition probabilities estimated from CF Registry dataset (excluding mortality adjustments)

Abbreviations: FEV1%, forced expiratory volume in the first second (percent predicted); Tx, transplant.
Table 2. Other model parameters

a Calculated cost reflects estimates of resource use for individual components (sampled from gamma distributions) combined with unit costs for those resource items (sampled from normal distributions).
Abbreviations: FEV1%, forced expiratory volume in first second (percent predicted); IRR, incidence rate ratio; IV, intravenous; MVN, multivariate normal; RR, risk ratio; Tx, transplant.
Baseline Characteristics
Initial patient characteristics (age, sex, and baseline FEV1% pred. strata) were based on the CFHH trial (Reference Wildman16).
Transition Probabilities, Usual Care
The probabilities of transitioning between the model health states under usual care were informed by analyses of a bespoke registry data set provided by the CF Trust (18). The data set contained patient-level records for all patients included in the CF Registry aged ≥16 years who had been prescribed nebulized mucolytics and/or antibiotics at any timepoint (data years 2006–2015). The data set included 44,464 records across 7,144 patients. We fitted a series of homogenous continuous time multistate models to the available data using the msm package in R (Reference Jackson26). As this method assumes that event hazards are constant over time, fitting a single model to the whole data set would have failed to reflect the increasing risk of death for older patients. Instead, we fitted separate models to subsets of the data relating to patients in the following age categories: 30–34; 35–39; 40–44; 45–49, and 50+ years. Annual transition probabilities for each age category were derived from the instantaneous transition intensities using the msm pmatrix function (Table 1). Within the economic model, constraints were applied to ensure that: (i) the risk of death for any CF patient is at least as high as that for the age- and sex-matched general population, based on English life tables (19), and (ii) the risk of death in patients aged 60 years or older is at least as high as that estimated by flexible parametric survival models reported by Keogh et al. (Reference Keogh, Szczesniak and Taylor-Robinson25). These constraints were applied to prevent the underestimation of mortality risk due to the limited number of older patients in the CF Registry data set.
Frequency of IV Antibiotic Days, Usual Care
The number of IV days per year is conditional on FEV1% pred. stratum under usual care was estimated from the CF Registry data set (18). Separate estimates were obtained according to setting (home or hospital). The data indicate that the mean number of days per year spent receiving IV treatments is higher in patients in the worse lung function strata (FEV1 > 70% pred. = 8.88 days; FEV140–69% pred. = 23.42 days; FEV1 < 40% pred. = 47.13 days).
Treatment Effects: Risk Ratios of Transitioning Between States and Relative Rate Ratio for IV Days
FEV1% pred. states were modeled using a cumulative link logit model with a nominal treatment group effect, baseline FEV1% pred. state and adjustment for the previous year’s IV days, fitted to data from the CFHH trial (Reference Wildman16). The logit model was used to generate predicted probabilities for each follow-up FEV1% pred. stratum, given baseline state and treatment allocation, from which RRs for between-state transitions by treatment group were derived. A bootstrap approach was used to estimate the standard errors (SEs) of the ratios on a log-normal scale.
IV day counts in the 12-month period following consent into the RCT were modeled using zero-inflated negative binomial regression with a treatment group factor, adjustment for baseline FEV1 status and the previous year’s IV days, and an offset for follow-up time. The treatment coefficient was exponentiated to give the IRR and the SE was estimated using the delta method. The model indicated a non-significant benefit in favor of the intervention (IRR = 0.92; 95 percent confidence interval [CI] 0.80, 1.06).
Follow-up in the trial was short, with a primary outcome window of 12 months. Whilst it is anticipated that the CFHH adherence intervention would be delivered on an ongoing basis, there is uncertainty surrounding the duration over which treatment effects on FEV1 and exacerbations persist. The base case analysis assumes that the intervention is given for 10 years; at this point, treatment effects are assumed to be lost. Given the absence of longer-term evidence, this is essentially an arbitrary assumption that is tested in sensitivity analyses.
Health-Related Quality of Life
Health utility values for the FEV1% pred. states were estimated using a de novo function developed to map from absolute FEV1% pred. scores to the 3-Level Euroqol 5-Dimensions (EQ-5D-3L) using data collected during clinic visits in the CFHH trial (Reference Wildman, O’Cathain and Hind11). The data used to estimate the mapping model included repeated observations on 607 patients. Observations were not included in the analysis if EQ-5D-5L or FEV1% pred. were missing or not measured in the same day/visit (2,308 excluded observations; 1,317 of those correspond to FEV1% pred. readings conducted as part of routine care, not the trial, and thus lack a contemporaneous EQ-5D-5L value). The final number of observations used was 2,386 (out of 4,694). The 5-level EQ-5D responses were mapped to the EQ-5D-3L using the function reported by van Hout et al. (Reference van Hout, Janssen and Feng27). An Adjusted Limited Dependent Variable Mixture Model (Reference Hernández Alava and Wailoo28;Reference Hernández Alava, Wailoo and Ara29) (ALDVMM) was used to estimate the relationship between FEV1% pred. and the mapped EQ-5D-3L values. Robust clustered SEs were used to account for repeated observations. Models using two to four components were estimated; based on goodness-of-fit statistics, a three-component model was selected for the base case analysis. Further details on model selection are provided in Wildman et al. (Reference Wildman, O’Cathain and Hind11). The final selected ALDVMM indicated lower mean utility for lower FEV1% pred. states (FEV1 > 70% pred. = 0.83; FEV1 40–69% pred. = 0.79; FEV1 < 40% pred. = 0.71).
Very few patients in the CFHH trial underwent transplantation; hence, the utility value for this state was instead taken from published literature (Reference Anyanwu, McGuire and Rogers23). QALY losses associated with receiving IV antibiotics were estimated non-parametrically using the CFHH trial data. Within the trial, the EQ-5D-5L was administered following the incidence of exacerbations requiring IV antibiotics (shortly after initiating IV antibiotics, and 2- and 4-weeks subsequently). The disutility associated with requiring IV antibiotics was estimated as the difference between the initial and 4-week exacerbation-related assessments. All health state utility values were adjusted for increasing age (Reference Ara and Brazier24). A sensitivity analysis was also conducted using published EQ-5D-3L estimates of health state utility and exacerbation-related disutility values (Reference Bradley, Blume and Balp30).
Resource Costs
Cost of Delivering the CFHH Adherence Intervention (Excluding Nebulizers)
The cost of the CFHH adherence intervention includes an initial cost associated with staff training and set-up; ongoing annual costs include data transfer, monitoring, maintenance, and hosting of the data platform; treatment fidelity support and delivery of the intervention by interventionists. The analysis assumes that 30 interventionists can support 5,900 adult CF patients receiving nebulized therapies. The per-patient cost of delivering the intervention was estimated to be GBP 777.18 (EUR 807.47) in the first year, and GBP 560.81 (EUR 582.67) in each subsequent year; based on resource use and costs taken from Jones and Burns (Reference Jones and Burns21); salary scales, and unpublished cost estimates from nebulizer manufacturers, local pharmacists and the ACtiF Project Management Group (PMG). Further details of the derivation of costs are provided in the Supplementary Material.
In line with the trial, patients in the intervention group were assumed to use the eTrack device (PARI GmbH), which tracks the number of treatment doses taken. Patients receiving usual care were assumed to use the regular eFlow device, which does not track device usage. Annuitized costs of the devices were estimated from confidential price information provided by PARI, assuming a device lifetime of 5 years and a discount rate of 3.5 percent.
Nebulized Drugs
Annual costs of nebulized antibiotics and mucolytics were based on CF Registry data (17), expert opinion, and unit costs from the British National Formulary (BNF) (22). Assuming that treatment is prescribed according to recommended guidelines (independent of patient adherence), nebulized drugs were estimated to cost GBP 11,632 (EUR 12,085) per patient per year. The costs of other CF treatments were assumed to be unaffected by the intervention and were thus excluded from the analysis.
Cost of Health State Resource Use, Exacerbations, and Transplantation
Health state resource use was estimated using a standardized form developed for use in the CFHH trial (Reference Wildman16). This included resource use associated with clinic visits, including GPs, hospital-based clinicians, nurses, dieticians, psychologists, physiotherapists, occupational therapists, radiographers, and social workers, as well as Accident and Emergency (A&E) and other visits, and other hospitalizations requiring IV treatment unrelated to exacerbations. Unit costs were taken from NHS Reference Costs and Jones and Burns. (20;Reference Jones and Burns21). Estimated annual costs were similar across the three FEV1% pred. strata (FEV1 > 70% pred. = GBP 3,368 [EUR 3,499]; FEV140–69% pred. = GBP 3,774 [EUR 3,921], FEV1 < 40% pred. = GBP 3,320 [EUR 3,449]).
Exacerbations treated in the hospital were assumed to include the costs of inpatient stays and IV drugs, whilst those treated at home included IV drugs, consumables, and home delivery. As CF care in England is funded through a national specialized commissioning tariff, unit costs for CF-related exacerbations do not exist. Instead, the cost per inpatient IV day was based on the cost of bronchiectasis, which requires treatment that is similar to CF (20). The daily costs of IV drugs were estimated to be GBP 27.82 (EUR 28.90) when delivered in the hospital and GBP 165.36 (EUR 171.81) when delivered at home (20;22). The mean cost of transplantation was estimated to be GBP 88,823.49 (EUR 92,285.87) (20); this is applied once to patients who enter the transplant health state.
Model Evaluation Methods
Central estimates of cost-effectiveness were based on the mean of 2,000 Monte Carlo samples. Parameter uncertainty was evaluated using deterministic sensitivity analysis (DSA) and probabilistic sensitivity analysis (PSA). DSAs were used to identify key drivers of cost-effectiveness, including exploring alternative assumptions regarding: the impact of increased adherence on costs of prescribed nebulized therapies; the magnitude of relative treatment effects on transition probabilities and IV days; utilities; costs and survival, and the impact of alternative discount rates. PSA was used to estimate the probability that the intervention is cost-effective across a range of willingness-to-pay (WTP) thresholds.
Model Verification and Validation
Several activities were undertaken to ensure the internal validity and credibility of the model. These included: consideration of key items contained within published economic evaluation/modeling checklists (Reference Weinstein, O’Brien and Hornberger31;Reference Drummond, Sculpher and Claxton32), discussion of the model structure amongst members of the PMG; double-programming the deterministic model; checking parameter values against their sources; using clinical input to determine alternative plausible assumptions, and comparing model-predicted health state occupancy and survival against CF Registry data (see Supplementary Material).
Results
Table 3 presents the central estimates of cost-effectiveness of the CFHH adherence intervention versus usual care. Compared with usual care, the CFHH adherence intervention is expected to generate small health improvements and cost-savings; hence, the intervention dominates usual care. The magnitude of health gains and cost-savings is greater for those scenarios in which the intervention is given over a longer time frame. Assuming that the intervention is delivered only for 1 year and that all treatment effects are lost at this point, the CFHH adherence intervention is expected to generate 0.02 additional QALYs and cost savings of GBP 71 (EUR 74) per patient compared with usual care. Assuming that the CFHH adherence intervention is delivered for 10 years (the base case), the intervention is expected to generate 0.17 additional QALYs and cost-savings of GBP 1,600 (EUR 1,662) per patient. Whilst the magnitude of these benefits and cost-savings is influenced by the duration over which effects and costs are assumed to apply, the general conclusion that the intervention dominates usual care is consistent across all scenarios considered, although these impacts remain small regardless of the time horizon. Assuming a WTP threshold of GBP 20,000 (EUR 20,780) per QALY gained, the probability that the intervention generates more net benefit than usual care is at least 0.79, irrespective of the duration over which the intervention is assumed to be delivered (see Figure S7 of the Supplementary Material).
Table 3. Cost-effectiveness results over different treatment effect durations, CFHH adherence intervention versus usual care, probabilistic model

a Undiscounted.
Abbreviations: ICER, incremental cost-effectiveness ratio; Inc., incremental; LYG, life year gained; QALY, quality-adjusted life year.
The DSAs indicate that the ICER for the CFHH adherence intervention is below GBP 20,000 (EUR 20,780) per QALY gained across most scenarios (Table 4). The analyses highlight that most of the health gain associated with the CFHH adherence intervention is attributable to impacts on the probability of switching health state, rather than reductions in IV days. Importantly, the scenario analyses indicate that if the treatment effects on transition probabilities are removed from the model, the ICER for the CFHH adherence intervention is in excess of GBP 90,000 (EUR 93,508) per QALY gained. Given the non-significant difference in change in FEV1% pred. observed in the trial, this means that the results of the model should be interpreted with some caution. A further area of uncertainty relates to the relationship between the increase in adherence resulting from the CFHH adherence intervention and its impact on nebulized drug treatment costs – when increased adherence is assumed to lead to a proportionate increase in the costs of prescribed nebulized therapies, the ICER is increased.
Table 4. DSA results over different durations for treatment effects and costs

Abbreviations: DSA, deterministic sensitivity analysis; IV, intravenous; QALY, quality-adjusted life year; TP, transition probability.
Discussion
Summary of Headline Cost-Effectiveness Results
To our knowledge, this is the first full economic analysis of an intervention to improve adherence to preventative therapies in CF based on evidence from an RCT. The economic model suggests that the CFHH adherence intervention is expected to generate additional health gains and cost savings compared with usual care. The analyses highlight that the magnitude of benefits and cost-savings is dependent on the duration over which the intervention is delivered. However, it is important to acknowledge that the CFHH trial did not demonstrate statistically significant benefits in terms of reduction in exacerbations (adjusted ratio for events, intervention versus control 0.96; 95 percent CI: 0.83, 1.12; p = .64) or improvements in FEV1% pred. (adjusted mean difference, intervention versus control 1.4; 95 percent CI: −.2, 3.0) (Reference Wildman, O’Cathain and Maguire12). These lower than expected treatment effect estimates may reflect the short study duration failing to fully capture the effect of the intervention on IV days, the fact that around 30 percent of trial patients already had high adherence (>75 percent) shortly after the baseline visit, the potential dilution of treatment effects due to control arm subjects having knowledge that their adherence behavior was being monitored, or simply that the intervention did not work as well as anticipated. Nevertheless, the model reflects the decision uncertainty around the available evidence and this suggests that the incremental net benefit of the intervention is expected to remain positive under the majority of plausible scenarios.
Strengths and Limitations
The model presented in this paper has several strengths. The underlying conceptual model is consistent with previous CF models which have focussed principally on lung function and pulmonary exacerbations (Reference Mohindru, Turner and Sach33). A minority of previous CF models (Reference Whiting, Al and Burgers34;35) have used a patient-level approach based on mortality risk equations, which necessitates the inclusion of other aspects of the disease including changes in BMI and chronic infections to predict survival. However, these models assume that within-patient changes in these factors are independent of one another, when in reality, they are likely to be highly correlated. We took the view that the assumption of independence between patient characteristics is unlikely to be reasonable; instead, we adopted a more conventional cohort-based modeling approach. The evidence used to estimate the clinical outcomes in the model is likely to be broadly generalizable to the wider adult CF population receiving inhaled therapy in the UK. The RCT used to estimate relative treatment effects included 607 patients from 19 CF centers (Reference Wildman, O’Cathain and Maguire12) and the CF Registry data set used to inform transition rates and exacerbation risks included data on more than 7,000 people with CF in the UK who had received inhaled therapy (10 data-years) (18). The disease natural history process was modeled using a multistate approach applied to patient-level data from the CF Registry which jointly estimates all transition rates under a competing risks framework. This baseline model may be valuable for future model-based economic analyses of CF interventions targeted at people taking nebulized therapy. In order to avoid bias resulting from imbalances in patient characteristics at baseline, treatment effects associated with the CFHH adherence intervention were estimated using an approach which accounts for stratification factors included in the trial. In addition, the de novo function to map from FEV1 to the EQ-5D-3L may be used in other economic analyses of CF interventions. Finally, we conducted extensive sensitivity analyses to assess the robustness of the conclusions.
The economic analysis is subject to several limitations which are driven largely by uncertainties in the available evidence. First, the short duration of the CFHH trial leads to uncertainty around the long-term impact of the CFHH adherence intervention on objectively measured and clinically relevant outcomes such as FEV1% and exacerbation rates. It is possible that given longer study follow-up, the treatment effects could be maintained, increased, or reduced. It is also noteworthy that more patients in the CFHH intervention group of the trial transitioned from FEV1 > 70% pred. to FEV1 40–60% pred. compared with the BSC group, and this is reflected in the modeled transition probabilities; this is likely to be due to randomness in the data caused by small patient numbers rather than a deleterious effect of the intervention. Second, it was necessary to fit separate models to the CF Registry data (18) by age band to better reflect the relationship between advancing age and the increasing hazard of death. Owing to limited data for older patients, it was necessary to constrain the survival probabilities using estimates from another published survival model (Reference Keogh, Szczesniak and Taylor-Robinson25). Whilst this is a limitation of the multistate approach, this constraint is applied only at the tail of the survival curve, many years after delivery of the CFHH adherence intervention and its benefits are assumed to have ceased. Third, the CFHH trial measured adherence using nebulizer devices. However, data were not collected on the associated impact of the intervention on medication prescribing levels – that is – whether increases in adherence also led to increases in drug prescriptions which would, in turn, lead to higher costs. The scenario analyses show that if greater adherence also increases drug prescriptions, the ICER becomes less favorable. A recently published analysis of MPR and objectively measured adherence in 275 patients using CFHH indicates that adherence improvements of the magnitude seen in the RCT would not increase system costs. This analysis also highlights how using objective adherence data to guide medicine deliveries has the potential to allow CFHH to create substantial savings (Reference Bevan, Hoo and Totton36).
Conclusions
The economic analysis suggests that the CFHH adherence intervention generates small health gains and cost-savings within a broad “all-comers” population of adult patients with CF who were taking inhaled antibiotic and/or mucolytic therapies. The relative effectiveness of the CFHH intervention observed in the trial was smaller than initially expected and the results are particularly sensitive to the relative treatment effect estimates applied to transition probabilities; hence, our conclusions regarding the cost-effectiveness of the intervention should be considered as uncertain. Future research should consider whether tailored support interventions may be of particular benefit for hard-to-reach CF populations with poor adherence. Ideally, such studies should include sufficient follow-up to fully demonstrate the impact of interventions on both adherence and objective measures of lung health.
Supplementary material
To view supplementary material for this article, please visit https://doi.org/10.1017/S0266462322003373.
Acknowledgments
We thank the CF Registry for providing access to data and the ACtiF Programme Management Group for providing advice throughout the study.
Funding statement
This project was funded by the National Institute for Health Research (NIHR) Programme Grants for Applied Research (project number RP-PG1212-20015). The views expressed are those of the authors and not necessarily those of the NHS, the NIHR, or the Department of Health and Social Care.
Conflicts of interest
The authors declare none.