Behavioral Models of Aging
In this section, we review models considering what cognitive abilities may be impaired with age, based largely on behavioral data. Each of these models primarily focuses on one ability, suggesting that losses in this ability can account for more widespread changes to memory or other cognitive abilities with age.
Sensory Deficit Hypothesis
The sensory deficit hypothesis proposes that cognitive changes associated with aging may be attributed to changes in sensation (i.e., deficits in vision and hearing). Specifically, processing of visual and auditory information is based on abilities that deteriorate with age (e.g., Stine, Wingfield, & Poon, Reference Stine, Wingfield, Poon, Poon, Rubin and Wilson1989; Chapter 10). Numerous studies have found that the correlation between sensory function and cognitive performance is stronger in old age than during earlier periods of adulthood (Anstey, Lord, & Williams, Reference Anstey, Lord and Williams1997; Anstey, Luszcz, & Sanchez, Reference Anstey, Luszcz and Sanchez2001; Anstey, Stankov, & Lord, Reference Anstey, Stankov and Lord1993; Baltes & Lindenberger, Reference Baltes and Lindenberger1997; Lindenberger and Baltes, Reference Lindenberger and Baltes1994; Lindenberger & Ghisletta, Reference Lindenberger and Ghisletta2009). For example, Baltes and Lindenberger (Reference Baltes and Lindenberger1997) found that the average proportion of individual differences in perceptual speed, reasoning, knowledge, fluency, and, importantly, memory were strongly related to visual and auditory acuity in old age (age range 70–103). Baltes and Lindenberger argued that the strong relationship between sensory and cognitive domains might be the result of a third common factor, or ensemble of factors. This common cause explanation suggests that the association between sensory and cognitive function occurs because “both sets of measures are an expression of the physiological architecture of the aging brain” (Baltes & Lindenberger, Reference Baltes and Lindenberger1997, p. 13).
An alternative interpretation is that sensory and perceptual tasks place an additional cognitive load on older adults. The result would be a reduction in the available cognitive resources. This is known as the cognitive permeation hypothesis (Li et al., Reference Li, Lindenberger, Freund and Baltes2001; Lindenberger & Ghisletta, Reference Lindenberger and Ghisletta2009; Lindenberger, Marsiske, & Baltes, Reference Lindenberger, Marsiske and Baltes2000; Rabbitt, Reference Rabbitt1968; Rabbitt, Reference Rabbitt1991). There are very few studies that have empirically evaluated the notion that sensory decline results in reduced processing resources. However, research does suggest an aging-induced permeation of sensorimotor functioning (the combined functions of sensory and motor activities) with cognition (Lindenberger et al., Reference Lindenberger, Marsiske and Baltes2000).
Although the theory originated with a focus on deficits in sensory systems underlying cognitive deficits with aging, the common cause model has transformed somewhat over time. Associations between sensory and cognitive functions may not be as strong as were initially reported, based on cross-sectional data (Anstey, Hofer, & Luszcz, Reference Anstey, Hofer and Luszcz2003; Lindenberger & Ghisletta, Reference Lindenberger and Ghisletta2009). However, the appreciation that a small number of factors account for cognitive changes with age (see the section “Domain-General versus Domain-Specific Age-Related Changes” about domain-general effects) and that there is a loss of specialization in sensorimotor systems (see the section “Dedifferentiation”) is in line with the idea of a “common cause” of aging.
Speed of Processing
The speed of processing model (cf. Salthouse, Reference Salthouse1991b, Reference Salthouse1996) argues that cognitive operations are limited by general processing constraints and variations in the efficiency or effectiveness of completion of specific processes. In other words, the theory assumes that the ability to process information from the environment becomes less efficient as we age. Therefore, with increasing age, capacity and mental energy, or cognitive resources, required to perform information-processing routines becomes more limited. It is assumed that general limitations frequently impose constraints on many types of processing (e.g., encoding) and hence, they have consequences for the performance of a large variety of cognitive tasks. A reduction with increased age in the speed with which many cognitive operations can be executed is hypothesized to be a major contributor to the adult age differences in many measures of cognition (e.g., digit symbol substitution; visual matching; measures of working and long-term memory) (Salthouse, Reference Salthouse1979, Reference Salthouse1985a, Reference Salthouse1985b, Reference Salthouse1991a, Reference Salthouse1996; Salthouse, Babcock, & Shaw, Reference Salthouse, Babcock and Shaw1991; Salthouse et al., Reference Salthouse, Legg, Palmon and Mitchell1990; Salthouse & Mitchell, Reference Salthouse and Mitchell1990).
According to this model, there are two factors that contribute to overall processing speed effects on cognitive processes: limited time and simultaneity (Salthouse, Reference Salthouse1996). In the context of the limited time mechanism older adults execute relevant cognitive operations too slowly for successful completion. This limited time mechanism suggests that the processing of information occurs in discrete steps, and response latencies represent the sum of the duration of the individual steps. According to the processing speed theory, as we age, we may spend more time executing early operations associated with a particular cognitive task, restricting the time available for later processing.
The key assumption in the simultaneity mechanism is that a slower speed of processing results in less information available for simultaneous processing. The concept of working memory is another way of referring to the amount of simultaneously active information, and there are many reports of age-related declines in measures of working memory (Gazzaley et al., Reference Gazzaley, Sheridan, Cooney and D’Esposito2007; Hasher & Zacks, Reference Hasher and Zacks1988; Park & Payer, Reference Park, Payer, Bialystok and Craik2006). When the rate of executing operations is slow, the relevant information is less likely to be useful because it may be impoverished by the time preceding operations are completed (Salthouse, Reference Salthouse1996).
Inhibitory Deficit Hypothesis
A third theoretical model for cognitive aging is the inhibitory deficit hypothesis. In addition to processing speed, research suggests that older adults may manifest inhibitory deficits in working memory. Hasher and Zacks (Reference Hasher and Zacks1988) proposed the inhibitory deficit framework, which suggests that an efficient (fast and accurate) mental life requires the ability to limit activation to information most relevant to one’s goals. Three functions of inhibition were proposed: controlling access to attention’s focus, deleting irrelevant information from attention and working memory, and suppressing or restraining strong but inappropriate responses. The inhibitory deficit hypothesis has generally been supported by findings from a variety of experimental paradigms, including negative priming (Kane et al., Reference Kane, Hasher, Stoltzfus, Zacks and Connelly1994; Tipper, Reference Tipper1991), text processing (Hamm & Hasher, Reference Hamm and Hasher1992), and speech production (Arbuckle & Gold, Reference Arbuckle and Gold1993).
Hasher and Zacks (Reference Hasher and Zacks1988) and Hasher and Campbell in this handbook (Chapter 8) presented compelling evidence demonstrating that older adults were more likely to maintain disconfirmed antecedent information that they heard earlier than younger adults, and that this irrelevant information affected subsequent cognitive performance. Additional inhibitory deficit evidence comes from working memory capacity experiments with older adults. For example, in a standard working memory capacity experiment, participants are presented with lists in increasing order of length, from shortest to longest (e.g., Daneman & Carpenter, Reference Daneman and Carpenter1980) or in a random order (e.g., Engle, Cantor, & Carullo, Reference Engle, Cantor and Carullo1992), setting the stage for recall of the longest lists to be vulnerable to disruption from any nonsuppressed materials from earlier lists. When the longest sets are given first to younger and older adults, age differences in span are reduced and can even be eliminated (see Lustig, May, & Hasher, Reference Lustig, May and Hasher2001; May, Hasher, & Kane, Reference May, Hasher and Kane1999; Rowe, Hasher, & Turcotte, Reference Rowe, Hasher and Turcotte2008). According to Lustig, Hasher, and Zacks (Reference Lustig, Hasher, Zacks, Gorfein and MacLeod2007) the typical age differences observed on working memory span tasks seem to be the product of a reduced ability to delete or suppress no longer relevant materials, rather than of age differences in processing resources (see also Bunting, Reference Bunting2006; Friedman & Miyake, Reference Friedman and Miyake2004; Hedden & Park, Reference Hedden and Park2003).
Recollection Deficits
So far, the discussed theoretical models propose age-related changes in general processing that cut across a number of cognitive domains, and generally impact cognitive functioning. Each theoretical model has garnered much support, yet some age-related changes in cognition may not be yoked to a single core deficit that affects all aspects of cognition. In this section, we discuss specific changes in episodic memory that are associated with normal aging.
Episodic memories are those that not only include the “what,” but also the “when” and “where” of our daily lives. They are memories that once initially learned, or encoded, are dropped from consciousness, and consolidated into long-term memory. Remembering occurs when the memory returns to consciousness. Episodic memory can be thought to include two types of information: (1) memory for items previously encountered, and (2) memory for the context in which those items were encountered. Further, if we adopt a dual-process model of episodic memory retrieval we assume that retrieval of episodic events (including items and/or contexts) can be based on processes of familiarity and recollection (see also Chapter 11). Conscious recollection of the context and other details associated with prior experience can be an important influence in attributing information to its specific prior experience. However, frequently retrieved events are attributed to prior experience, not because of a conscious recollective experience, but rather, because those events accompany some feeling of familiarity (Jacoby, Kelley, & Dywan, Reference Jacoby, Kelley and Dywan1989b; Light, Reference Light, Naveh-Benjamin and Ohta2012; Reinitz et al., Reference Reinitz, Séguin, Peria and Loftus2012; Rhodes, Castel, & Jacoby, Reference Rhodes, Castel and Jacoby2008; Wong, Cramer, & Gallo, Reference Wong, Cramer and Gallo2012). Familiarity and recollection can be thought of as two independent mechanisms that influence remembering. Whereas recollection is a cognitively effortful conscious process, familiarity has been described as a faster, more automatic basis for responding (Atkinson & Juola, Reference Atkinson, Juola, Krantz, Atkinson, Luce and Suppes1974; Hintzman & Curran, Reference Hintzman and Curran1994; Jacoby, Yonelinas, & Jennings, Reference Jacoby, Yonelinas, Jennings, Cohen and Schooler1997; McElree, Dolan, & Jacoby, Reference McElree, Dolan and Jacoby1999; Yonelinas & Jacoby, Reference Yonelinas and Jacoby1996a, Reference Yonelinas and Jacoby1996b, Reference Yonelinas and Jacoby2012).
In the context of this dual-process model, recollection seems to experience age-related declines, whereas familiarity remains relatively constant across the life span. For example, in one study Jacoby (Reference Jacoby1999) demonstrated that older adults were more likely to use familiarity to make memory decisions, as opposed to conscious recollective processes. In these experiments, younger (e. g., college undergraduates) and older participants (aged 60–94) were presented with a list of words to read. Words were presented one, two, or three times. Following this, participants were presented with a list of words aurally and told these words were to be remembered for an upcoming memory test. There was no overlap between read and heard words. For the test, participants were instructed to indicate that a word had previously been presented if the word was presented in the second heard list. Older adults were more likely to falsely claim repeatedly presented read words were heard as compared to younger adults. In fact, younger adults were more likely to correctly reject read words as the number of repetitions increased. When younger adults were forced to respond under a very short deadline that prevented the recollective process, they demonstrated the same familiarity-dependent pattern of errors as older adults. Jacoby argued that younger adults were more likely to engage in conscious recollective processes as compared to older adults.
Jacoby (Reference Jacoby1991) argued that conscious recollective processes at retrieval could be employed only in situations in which it was facilitated by prior processing. For example, in situations of divided attention at encoding, individuals may not have the capability of engaging in recollective processes at retrieval. One might argue that older adults would then likely be at a disadvantage if domain-general theoretical models of cognitive aging are considered in the context of this domain-specific proposal. That is, older adults may engage in less effortful or shallower processes at encoding, because of limits in processing speed and/or because of problems inhibiting irrelevant thoughts. However, even when older and younger adults were equated on original learning, they were less likely to engage in conscious recollective experiences as compared to younger adults (Jacoby et al., Reference Jacoby, Bishara, Hessels and Toth2005; see Daselaar et al., Reference Daselaar, Fleck, Dobbins, Madden and Cabeza2006, for a parallel finding in the neuroimaging literature revealing that age impairs recollection-related hippocampal recruitment, while showing heightened familiarity-related recruitment in cortical medial temporal lobe [MTL] regions).
Binding Deficits
Research suggests that one reason older adults may demonstrate a deficit in recollection is because they have not sufficiently bound the elements of an event into an integrated episode, or memory. Episodic retrieval can involve access to individual items or details of a memory, the retrieval of the context in which the item(s) were presented, or a combination of both. Memory errors may occur because such individual pieces of a memory are not sufficiently integrated at encoding (Dodson, Holland, & Shimamura, Reference Dodson, Holland and Shimamura1998; Gruppuso, Lindsay, & Kelley, Reference Gruppuso, Lindsay and Kelley1997; Jacoby et al., Reference Jacoby, Kelley, Brown and Jasechko1989a; Kelley & Sahakyan, Reference Kelley and Sahakyan2003). Memory errors can also occur when source information, or contextual details, is similar across episodes (Hashtroudi, Johnson, & Chrosniak, Reference Hashtroudi, Johnson and Chrosniak1989; Lindsay, Johnson, & Kwon, Reference Lindsay, Johnson and Kwon1991; Mitchell & Zaragoza, Reference Mitchell and Zaragoza2001; Thomas & Bulevich, Reference Thomas and Bulevich2006; Thomas, Bulevich, & Loftus, Reference Thomas, Bulevich and Loftus2003), such that retrieval results in the incorrect source or details being retrieved alongside an item which was not originally paired together at encoding.
There is considerable evidence to support the conclusion that older, compared to younger, adults demonstrate more severe source-monitoring deficits (Chalfonte & Johnson, Reference Chalfonte and Johnson1996; Glisky, Rubin, & Davidson, Reference Glisky, Rubin and Davidson2001; Mitchell et al., Reference Mitchell, Johnson, Raye, Mather and D’Esposito2000; Naveh-Benjamin, Reference Naveh-Benjamin2000; Naveh-Benjamin, Brav, & Levy, Reference Naveh-Benjamin, Brav and Levy2007; O’Hanlon, Wilcox, & Kemper, Reference O’Hanlon, Wilcox and Kemper2001; Thomas & Bulevich, Reference Thomas and Bulevich2006). For example, researchers have demonstrated that older adults are less likely to remember various contextual features, such as color or print style of materials (Kausler & Puckett, Reference Kausler and Puckett1981b; Light et al., Reference Light, LaVoie, Valencia-Laver, Albertson Owens and Mead1992; McIntyre & Craik, Reference McIntyre and Craik1987), the gender of a speaker (Ferguson, Hashtroudi, & Johnson, Reference Ferguson, Hashtroudi and Johnson1992; Kausler & Puckett, Reference Kausler and Puckett1981a), the location of targets (Light & Zelinski, Reference Light and Zelinski1983; Park, Puglisi, & Sovacool, Reference Park, Puglisi and Sovacool1983; Pezdek, Reference Pezdek1983), the context in which a word was previously presented (Hashtroudi et al., Reference Hashtroudi, Johnson and Chrosniak1989; Mitchell, Hunt, & Schmitt, Reference Mitchell, Hunt and Schmitt1986), or whether a test item came from a videotape or from photographs (Schacter et al., Reference Schacter, Koutstaal, Johnson, Gross and Angell1997).
This age-related deficit in associative memory has also been shown to be greater than the age-related deficit demonstrated for item memory (for review see Kaszniak & Newman, Reference Kaszniak, Newman, Honn Qualis and Abeles2000; Old & Naveh-Benjamin, Reference Old and Naveh-Benjamin2008; Spencer & Raz, Reference Spencer and Raz1995). Typically, in these studies researchers present older and younger participants with item-item or item-context pairs at study. At test, item memory and associative memory are assessed using a recognition test including both intact and recombined pairs (targets and lures, respectively). Recombined pairs consist of items originally presented during the initial study, but not part of the same trial. Using this paradigm, researchers have demonstrated that older adults store individual items similarly to younger adults, as measured by performance on an item memory recognition test; however, they demonstrated a deficit in memory for associative information, with memory errors arising in association with the endorsement of lures as targets. This had led researchers to suggest that the binding deficit demonstrated by an associative recognition test may be due to the engagement of simple, less effortful retrieval processes, specifically that of recollection opposed to familiarity processes.
Deficits in Self-Initiated Processes
A broad examination of findings used to support both domain-specific and domain-general theories of cognitive aging suggests that older adults may present deficits in both encoding and retrieval. Take, for example, the speed of processing account. According to this proposal, age-related deficits in memory arise because relevant cognitive operations are executed too slowly to be successfully completed. Shallow processing or limitations in encoding also may be linked to domain-specific accounts for age-related deficits in memory. First, older adults have difficulties initiating effective encoding “strategies” that could promote memory for the associative details of an experience. Craik and Byrd (Reference Craik, Byrd, Craik and Trehub1982) suggested that older adults may be deficient in the ability to engage in what they called “self-initiated processing.” According to this view, these deficits reduce the kinds of self-initiated activities that are required for efficient task completion at encoding. Thus, older adults may have more difficulty in generating elaborate and distinctive memory traces (encoding deficit). In addition, if older adults do not devote significant cognitive resources to search at retrieval, even an elaborate memory trace will fail to be retrieved. If effective processes are not engaged at either encoding or retrieval, memory accuracy will likely be impaired. More recently, DeCaro and Thomas (Reference DeCaro and Thomas2019) demonstrated that the deficits in self-initiated processing or strategic regulation of learning and remembering may be task dependent. Older participants were as successful in the restudy decisions as younger adults when the decision to restudy was made immediately after a retrieval attempt as opposed to when the decision was delayed. Oft-found age-related differences in restudy decisions have been within the context of blocked designs where the restudy choice block follows an evaluation and retrieval attempt block. These findings are important because they suggest that age-related differences in self-initiated processes or in strategic regulation should be considered in the context of how experiments have been designed to address these issues.
Important to the discussion of strategy use in aging, neuroimaging studies show that providing older adults with a memory strategy can improve performance through the modulation of neural processing during both encoding (Berry et al., Reference Berry, Zanto and Clapp2010; Kirchhoff et al., Reference Kirchhoff, Anderson, Smith, Barch and Jacoby2012; Nyberg et al., Reference Nyberg, Sandblom and Jones2003) and retrieval (Belleville et al., Reference Belleville, Clément and Mellah2011; Hampstead et al., Reference Hampstead, Sathian and Phillips2012; Kirchhoff et al., Reference Kirchhoff, Anderson, Smith, Barch and Jacoby2012). For example, Kirchhoff and colleagues (Reference Kirchhoff, Anderson, Smith, Barch and Jacoby2012) found that providing older adults with a semantic encoding strategy lead to both improved memory and increased recollection-related activity in the bilateral hippocampus and bilateral middle and inferior prefrontal cortex during retrieval. Further, activity within the prefrontal and left lateral cortex was also associated with self-initiated semantic strategy use during encoding. Results suggest that some of the age-related neural deficits observed in memory tasks (see Chapter 11) may be due to older adults’ failure to engage the same strategies employed by their younger counterparts.
Brain-Based Models of Aging
One of the notable advances in research on cognitive aging over the past couple of decades has been efforts to characterize and interpret age differences in neural activation. While conventional thinking might assume that age-related decline in cognitive functioning would simply be associated with decreases in neural recruitment associated with a given cognitive task, the story is much more complicated. Decades of research has shown that neural activity associated with cognitive aging is characterized by decreases as well as increases in activation. Early work in this area focused largely around the idea of neurocognitive decline, dedifferentiation, and compensation. Several models have been proposed to account for the different patterns of activation that are typically observed across aging studies. Within these models researchers have included several factors, including (but not limited to) the link between neural activation and behavior, task difficulty, and individual differences as characterized by such theoretical concepts as reserve and maintenance. Below we highlight a few of these models, while focusing on their usefulness with respect to interpretation of data.
Compensation
Early work in neurocognitive aging characterized age differences in activation with respect to two fairly consistent patterns of age-related differences in brain activity, HAROLD and PASA. The HAROLD, or Hemispheric Asymmetry in OLDer adults, pattern was originally described by Cabeza and colleagues (Reference Cabeza, Grady and Nyberg1997) to account for the fact that older adults tended to exhibit greater bilaterality in their neural recruitment compared to what was found in younger adults. Cabeza and colleagues further suggested that, as this pattern typically was associated with successful cognitive outcomes in older adults (e.g., subsequent memory success, accurate perception, accuracy in working memory), such bilateral recruitment was related to the concept of compensation. A similar interpretation was associated with the observation of the PASA (posterior to anterior shift in aging) pattern (Dennis & Cabeza, Reference Dennis, Cabeza, Craik and Salthouse2008). This pattern was first observed by Grady and colleagues (Reference Grady, Maisog and Horwitz1994), who suggested that older adults compensate for deficits in visual processing with higher-order processing undertaken by the prefrontal cortices (PFC). Since then studies spanning perception, attention, working memory, and episodic memory have also reported age-related increases in more anterior brain regions (mainly PFC) coupled with decreases in posterior regions (mainly occipital cortex), when compared to the distribution of activity seen in younger adults.
While most subsequent studies supported a compensation account to these patterns (for a review see Dennis & Cabeza, Reference Dennis, Cabeza, Craik and Salthouse2008), it is critical to understand that vital to such an interpretation of age-related differences in neural activity is the relationship between the increased activation and cognitive performance. This fundamental aspect of understanding age differences in neural activation was outlined by Cabeza (Reference Cabeza2002) and furthered by Reuter-Lorenz and Cappell (Reference Reuter-Lorenz and Cappell2008) with their compensation-related utilization of neural circuits hypothesis (CRUNCH). Specifically, CRUNCH outlines situations in which age-related overactivation is compensatory and integrates in the concept of strategies differences underlying age-related differences in patterns of neural activity. CRUNCH asserts that a compensation hypothesis requires that performance be matched across age groups or that overactivation in older adults is correlated with higher performance (for an expanded view regarding compensation see Cabeza and Dennis, Reference Cabeza, Dennis, Stuss and Knight2012). With regard to HAROLD, the compensatory account is supported by studies that showed positive correlations between bilateral activation and cognitive performance (e.g., working memory; Reuter-Lorenz et al., Reference Reuter-Lorenz, Jonides and Smith2000) as well as those showing bilateral recruitment only in older adults whose task-related performance is matched to young adults, rather than those showing behavioral deficits (Cabeza, Reference Cabeza2002; Rosen et al., Reference Rosen, Prull and O’Hara2002). Similarly, PASA has been associated with equivalent cognitive performance across age groups (e.g., perception; Grady et al., Reference Grady, Maisog and Horwitz1994) as well as positive correlation with cognitive performance (e.g., perception and episodic memory; Davis et al., Reference Davis, Dennis, Daselaar, Fleck and Cabeza2008).
Fundamental to the notion of compensation is that such overaction occurs in response to issues of task difficulty. Also fundamental to compensation is the idea that overaction is undertaken due to a fundamental need of the network/system in older adults to work harder at a task, compared to younger adults, in order to accomplish task-related objectives (e.g., cognitive processes). Of course, these concepts are related and not unique to aging. The need for compensation has been suggested to be related to both the structural and functional integrity of neural networks in aging as well as the need to meet demands of task difficulty (see Reuter-Lorenz & Cappell, Reference Reuter-Lorenz and Cappell2008, for a discussion). More recently the relationship between cognitive architecture and resources and neural compensation in aging was reviewed by several cognitive aging researchers with the intent of further defining the concept of compensation (Cabeza et al., Reference Cabeza, Albert and Belleville2018). They further endorse the view that compensation be used to describe increased neural recruitment that is linked to cognitive performance measures and upregulated in response to increases in cognitive demand. Ideally, this cognition-enhancing recruitment will support performance, allowing older adults to meet task demands. However, more often such increases in neural activation may fall short and be insufficient to overcome limitations of the aging brain (see a discussion of STAC in the section “ Scaffolding Theory of Aging and Cognition”). This relationship has been described by an inverted U function with respect to task demands and overall neural recruitment (see Cappell, Gmeindl, & Reuter-Lorenz, Reference Cappell, Gmeindl and Reuter-Lorenz2010; Reuter-Lorenz and Cappell, Reference Reuter-Lorenz and Cappell2008).
Dedifferentiation
An alternative explanation to overactivation in older adults is that of dedifferentiation, whereby more widespread activation is considered to reflect an age-related impairment in the recruitment of specialized neural mechanisms (Li & Lindenberger, Reference Li, Lindenberger, Nilsson and Markowitsch1999). Dedifferentiation thus has links to the common cause model discussed in the section “Sensory Deficit Hypothesis” and that of age-related increases in correlations across tasks (e.g., Lindenberger and Baltes, Reference Lindenberger and Baltes1994). With respect to the relationship between compensation and dedifferentiation at the neural level, the latter is often associated with the lack of behavioral markers to account for the increased activation as well as findings that the same brain regions are active across different tasks.
One of the earliest demonstrations of age-related changes in line with dedifferentiation is that visual regions responsible for processing specific classes of stimuli in younger adults respond less selectively in older adults (Park et al., Reference Park, Polk and Park2004). For example, in younger adults, certain neural regions responded highly selectively to viewing faces or places, but those same regions in older adults responded to multiple classes of stimuli (e.g., faces and places). These fMRI data were reanalyzed with multivoxel pattern analysis (MVPA), a technique that allows for comparison of the pattern of neural activity across space rather than simply comparing the magnitude of activation in a spatially contiguous region as done with univariate approaches. These results revealed that older adults’ less selective neural activity emerged not only in ventral visual regions but also in the early visual cortex, suggesting widespread dedifferentiation in the neural response with age (Carp et al., Reference Carp, Park, Polk and Park2011). Dedifferentiation appears to result from both a loss of tuning with age, such that neurons respond less selectively in older adults (e.g., a neuron responds to both faces and scenes), and greater attenuation in neural responses with age, such that the neural response to the preferred stimulus is weakened (e.g., a face-selective neuron has a reduced response to faces) (Park et al., Reference Park, Carp and Kennedy2012).
Although dedifferentiation has been replicated numerous times in visual regions (e.g., Bowman, Chamberlain, & Dennis, Reference Bowman, Chamberlain and Dennis2019; Burianová et al., Reference Burianová, Lee, Grady and Moscovitch2013; Park et al., Reference Park, Carp and Kennedy2012), dedifferentiation has also been observed across memory-related regions (e.g., striatum/MTL; Dennis & Cabeza, Reference Dennis and Cabeza2011) and the motor system (Carp et al., Reference Carp, Park, Polk and Park2011). One important outcome of dedifferentiation is that the less selective neural regions may be able to respond more broadly to support age-related compensation (e.g., Dennis & Cabeza, Reference Dennis and Cabeza2011). Dedifferentiation can also contribute to findings of domain-general cognitive changes with age; as regions respond in more homogeneous ways across multiple tasks, this generality of response can contribute to a factor extending across specific domains (see discussion of domain-general vs. domain-specific changes with age in the section “Domain-General versus Domain-Specific Age-Related Changes”).
Failure to Suppress the Default Mode Network
Aging appears to impact the default mode network differently than other networks of the brain. This cortical midline network, consisting of medial prefrontal and parietal cortical regions, tends to be deactivated during tasks that demand external attention (e.g., judging the properties of a word) but activated during internal reflection (e.g., thinking about oneself; mind-wandering). In the first study of the effects of aging on this network (Lustig et al., Reference Lustig, Snyder and Bhakta2003), participants completed a semantic classification task in which they made making living/nonliving judgments about the concepts represented by words. Whereas younger adults deactivated this network, or activated it less than baseline, older adults did not do so to the same extent. Moreover, older adults with Alzheimer’s disease activated some regions of the default network when they should be deactivated. These patterns are shown in Figure 1.1. Most cognitive processes require external attention, making these changes in ability to suppress the default mode network during such tasks potentially a widespread challenge with aging. Subsequent research extended the finding of reduced deactivation of the default mode with age, coupled with increased engagement of the task-positive network (i.e., those regions activated for externally focused, cognitively demanding tasks), across a number of different tasks (Grady et al., Reference Grady, Protzner and Kovacevic2010). In addition, age differences in suppression of the default mode might be most apparent for cognitively demanding tasks but not emerge at low levels of task demand (Persson et al., Reference Persson, Lustig, Nelson and Reuter-Lorenz2007). This suggests that older adults may have difficulty modulating the default mode network in response to task demands.
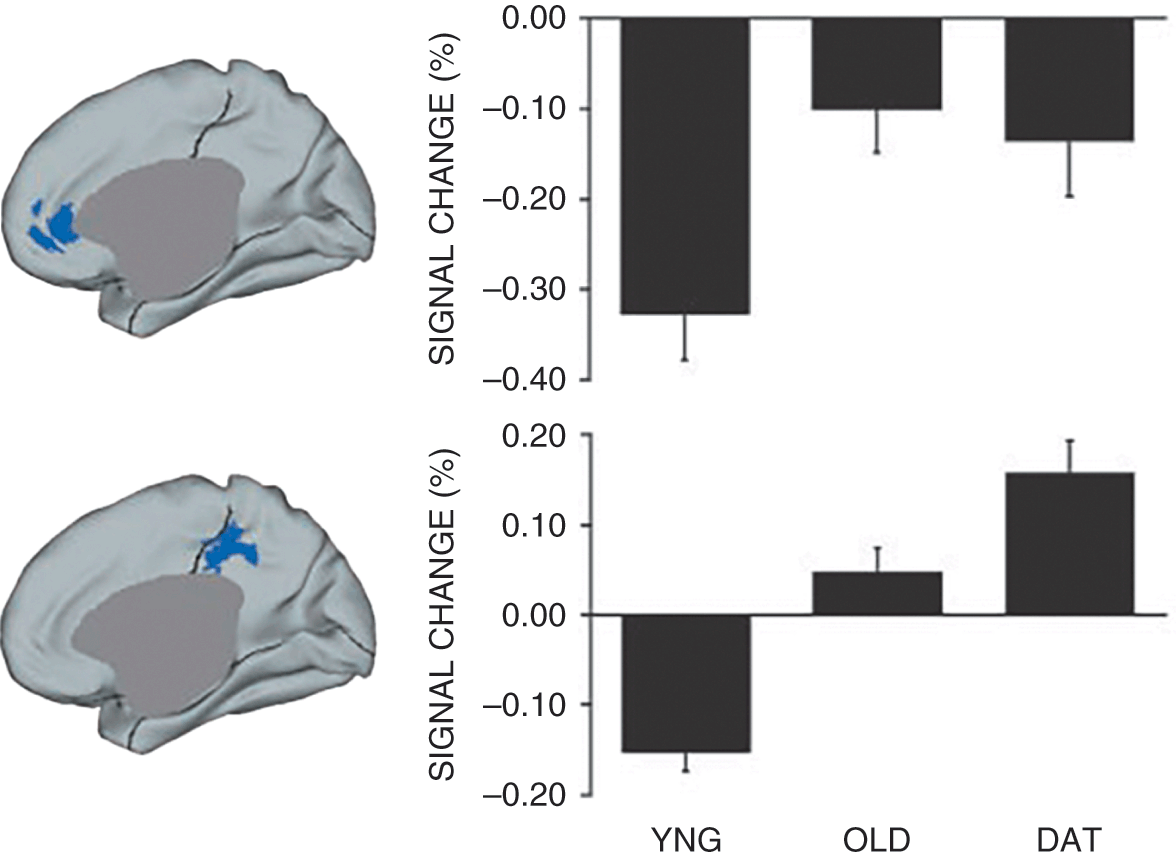
Figure 1.1 Functional deactivations: Change with age and dementia of the Alzheimer type.
The engagement of default mode regions in younger adults (YNG), older adults (OLD), and in older adults with dementia of the Alzheimer’s type (DAT) during word judgments, and an externally focused task. For both the medial prefrontal cortex (top row) and the medial parietal/posterior cingulate cortex (bottom row), older adults do not suppress the regions as much as younger adults. Older adults with Alzheimer’s disease show exaggerated failure to suppress in the posterior region. Figure adapted from Lustig et al. (Reference Lustig, Snyder and Bhakta2003).
One theory focuses on age-related changes to the default mode network, considered alongside changes in the executive network. The default-executive coupling hypothesis of aging (DECHA) (Spreng & Turner, Reference Spreng and Turnerin press; Turner & Spreng, Reference Turner and Spreng2015) proposes that aging impacts the coupling of the default mode network and the lateral prefrontal brain regions that contribute to cognitive control. The difficulty modulating prefrontal control regions may lead older adults to rely more on semantic processing with age. This shift reflects not only the impairment to frontal control processes, but also the relative preservation with age of semantic-based crystallized abilities (Horn & Cattell, Reference Horn and Cattell1967), such as knowledge of facts, schemas, and scripts. As functional networks can flexibly couple together, DECHA emphasizes the context dependency of this age-related change in the coordination of networks. Factors such as the availability of cognitive control resources and the goal relevance of task-congruent knowledge can determine whether the default and executive networks activate together for older adults.
Scaffolding Theory of Aging and Cognition
The scaffolding theory of aging and cognition (STAC; Park & Reuter-Lorenz, Reference Park and Reuter-Lorenz2009) adopts a broader approach to understanding cognitive aging. This conceptual model integrates the types of behavioral theories described in the section “Behavioral Models of Aging” that identify age differences in sensory processing, speed of processing, inhibition, recollective memory, memory binding, and self-initiated processes alongside the neural frameworks described in the section “Brain-Based Models of Aging” through models such as HAROLD, PASA, and CRUNCH. By combining these disparate findings, STAC incorporates factors associated with cognitive decline and those that affect compensation. The model highlights the ways in which the brain responds to challenges by functionally reorganizing and repairing itself in a continuous fashion to support cognition with age. The way in which these functional changes emerge could be seen as in line with the idea of environmental support (section “Self-Initiated Processes and Environmental Support”), as the brain generates a type of support for cognitive performance when original approaches fail.
The STAC model incorporates behavioral and neural findings from a rich history of research. As shown Figure 1.2, age-related changes in the brain such as cortical thinning (Chapter 3), white matter changes (Chapter 3), loss of dopamine (Chapter 5), failures to suppress the default mode during tasks that demand external attention (section “Failure to Suppress the Default Mode Network”), and reduced engagement of the medial temporal lobes (Chapter 11) present neural challenges that can lead to functional deterioration that would impact cognitive functioning. However, compensatory scaffolding through processes depicted in Figure 1.1 such as increased bilaterality (Cabeza, Reference Cabeza2002; Reuter-Lorenz et al., Reference Reuter-Lorenz, Jonides and Smith2000) or recruitment of frontal regions (Davis et al., Reference Davis, Dennis, Daselaar, Fleck and Cabeza2008; Grady et al., Reference Grady, McIntosh and Horwitz1995; Gutchess et al., Reference Gutchess, Welsh and Hedden2005) could alter the trajectory of aging, potentially mitigating or reducing the extent of age-related impairments. Through these examples, one can see that the prefrontal cortex is often a site for scaffolding. Despite sometimes pervasive age-related changes in this region of the brain (e.g., Chapter 11), this region is one of the most flexible, responsible for implementing strategies (Chapter 7) and responding to difficulty and conflict. STAC accounts for how cognitive performance can be relatively preserved despite the extent of neural changes that occur with age. Engaging compensatory scaffolding by recruiting new neural pathways or pathways originally used during earlier development or new learning is a normal response to the challenges associated with aging, as it makes up for neural circuitry that has become inefficient. In this way, the STAC framework differs somewhat from the concept of cognitive reserve (Chapter 2); the cognitive reserve framework emphasizes the response of the brain in late life when under challenge whereas STAC considers these processes to be a normal process occurring across the life span.
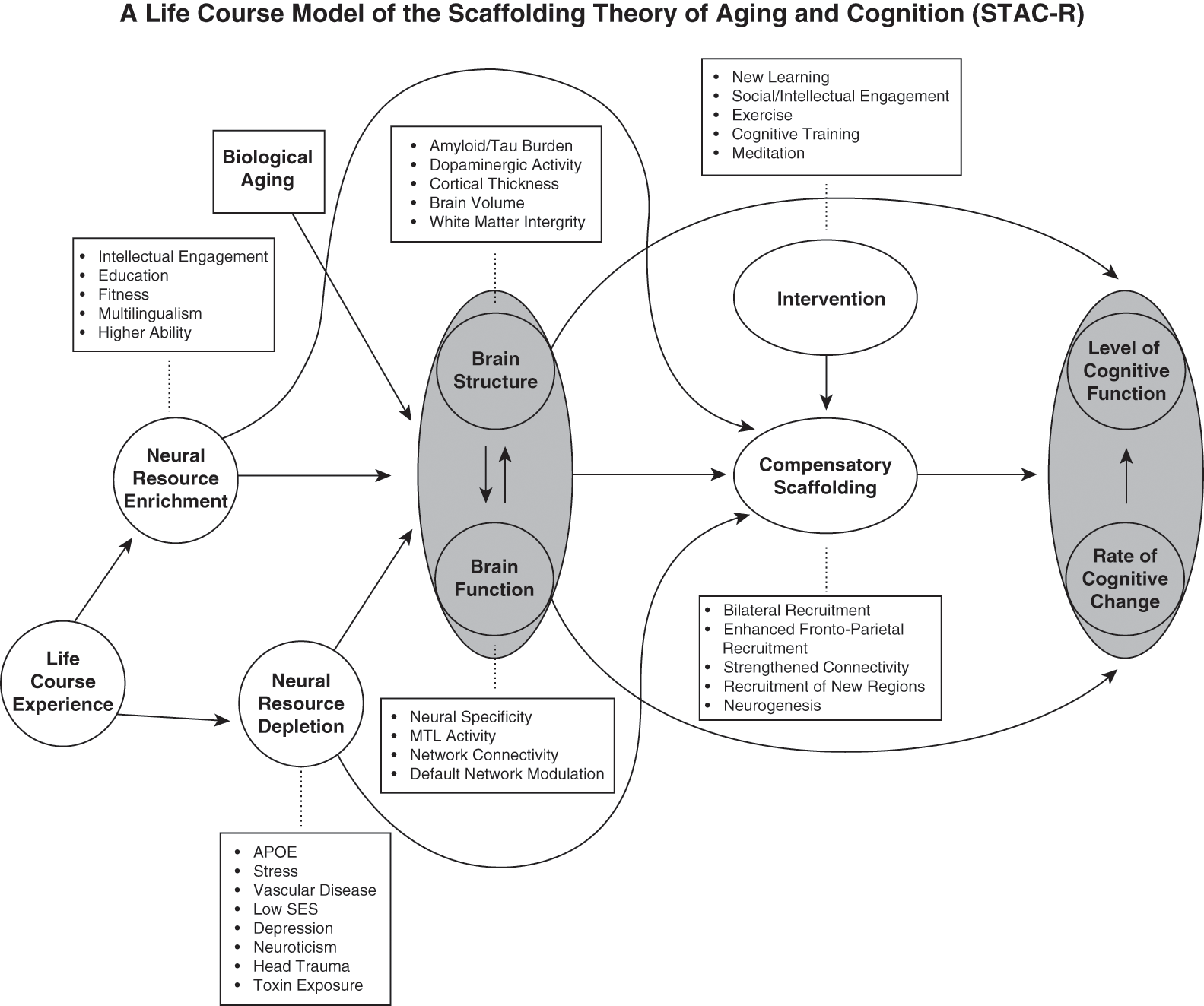
Figure 1.2 The scaffolding theory of aging and cognition (STAC) – revised.
Since original publication of the STAC model in 2009, strong evidence for life-span compensatory processes emerged in the literature, particularly from longitudinal studies. The revised STAC model (STAC-r; Reuter-Lorenz & Park, Reference Reuter-Lorenz and Park2014) introduces into the model even more factors that influence developmental trajectories, with a particular emphasis on the types of experiences that accumulate across the entire life span, from birth to death. The resulting framework provides a way to think about how the dynamic changes to the brain with age reflect the response to both challenges and compensatory processes. These life-span “neural resource enrichment” factors include those experiences that shape cognition in positive ways, such as education (Chapter 2), multilingualism (Chapter 26), and higher ability at early life stages (Chapter 24; Deary, Pattie, & Starr, Reference Deary, Pattie and Starr2013; Gow et al., Reference Gow, Johnson and Pattie2011). “Neural resource depletion” factors, including low socioeconomic status and personality traits such as neuroticism, exert long-term negative effects. Both neural resource enrichment and depletion factors can exert effects directly through brain structure or function as well as indirectly, through increasing capacity for scaffolding, as shown in Figure 1.2. The model further delineates potential interventions, such as new learning, training, and meditation, from other factors that may lead to individual differences as a result of genetic differences, early life experiences, or long-term exposure (see Figure 1.2).
Despite the potential for scaffolded networks to support cognition, there are some limitations. The development of skills and learning reflected in faster, more accurate performance is accompanied by neural networks that are pruned and honed to be more specialized. Performance relying on scaffolds will be less efficient than when relying on the original networks that are sculpted and specialized for that purpose. In addition, neurobiological changes with age will, over time, limit the flexibility that is available in networks. As aging progresses, the ability to plastically reorganize and recruit additional networks may not be able to keep up with the need for compensatory scaffolding, resulting in greater expression of age-related losses in cognition. Pathology, such as through Alzheimer’s disease, eventually accumulates to the point at which the scaffolds collapse and fail to support performance.
Individual difference factors impact the need for compensatory scaffolds as well as the availability of them. Health conditions (e.g., Chapter 32), advanced age, or genetic factors (e.g., such as possessing two copies of the APOE4 allele; see Chapter 30) can have adverse effects on compensatory scaffolds. Compensatory scaffolds also can be enhanced, as depicted in Figure 1.2, through factors such as engaged lifestyles, including new learning and experiences (Chapter 37), physical activity (Chapter 35), and cognitive training (Chapter 36).
STAC makes several predictions that could be tested. For one, scaffolding should reflect the degree of neural insults such that more neural challenges (e.g., reduced integrity of white matter; cortical thinning) would be associated with more use of scaffolding. However, this relationship may only occur up to the point at which scaffolds can no longer support additional cognitive performance. Scaffolding should also emerge systematically in regions that have a role in the task or are engaged by young adults at high levels of task difficulty, rather than by randomly recruiting regions. However, the site of scaffolding might be more variable with age than the site of the primary regions engaged by tasks, reflecting what regions are most healthy in an individual. Furthermore, although compensatory recruitment is beneficial to older adults, recruiting scaffolds in younger adults may predict poorer performance and the risk of aging at a faster rate. Finally, scaffolds are flexible, and can be altered through training, though this can be an effortful way to create scaffolds.
Although evidence has accumulated in support of some of these predictions, others are harder to test. For example, what degree of neural insult should elicit the creation of scaffolds? At what point can scaffolds no longer support cognition? How does one measure the effort needed to create scaffolds through training? These types of questions require a precision of measurement that is not currently available, including extensive measurement of change within an individual, particularly given the potential for variability across individuals. Reuter-Lorenz and Park (Reference Reuter-Lorenz and Park2014) also note the difficulty in identifying the mechanisms that support the benefits of training on creating new scaffolds, and the importance of identifying when certain types of interventions or life experiences would exert their strongest effect on late-life cognition (e.g., are the cognitive benefits of engaging in physical exercise the same across young, middle, and late life?). In addition, they suggest the importance of being able to predict the neural patterns that predict brain health, and how compensatory scaffolds would be anticipated to support performance in individuals exhibiting different markers of brain health.
Despite the impressive breadth of scope of STAC-r, future research is positioned to add even more socioemotional factors to consider. Although the model incorporates some factors such as depression and social engagement, there are many others, particularly socioemotional ones, for which we are only beginning to understand their effects on cognition and brain function (e.g., Gutchess, Reference Gutchess2014). For example, social networks (Chapter 19), future time perspective (Chapter 14), or other motivational factors (Chapter 18) have been suggested to impact cognitive performance, and emotion regulation may play a large role in well-being and the ability to process information in emotionally challenging settings (Chapter 16). How do these factors influence the recruitment and deployment of compensatory scaffolds? Is the need for scaffolds the same for strictly cognitive tasks (e.g., memory) as well as those tasks completed in a socioemotional contexts (e.g., collaborative cognition, Chapter 15; emotion recognition, Chapter 20), or concerning information relevant to goals? Does being under stereotype threat (Chapter 22) impact one’s ability to rely on compensatory scaffolds? Integrating a socioemotional perspective may further enrich the understanding of the life course processes that support and stifle the development and use of compensatory scaffolds.
Ongoing Debates and Future Directions
Domain-General versus Domain-Specific Age-Related Changes
The extent to which different abilities and cognitive processes reflect the effects of aging on separate underlying processes or a smaller number of interrelated processes continues to be debated. This question raises, for example, whether impairments in memory result from effects of aging on a distinct memory system, or whether other general factors, such as reduced speed of processing or impaired inhibition, also account for disruptions to memory. In neural terms, do the effects of aging lead to widespread changes that extend across brain regions or does aging differentially affect distinct systems (e.g., the frontoparietal attention network; default mode network), such that systems can be affected by aging at different rates or to different degrees?
To further probe the extent to which effects of aging are domain-general versus domain-specific, one large-scale study investigated over 1,200 adults who completed twelve cognitive measures in a longitudinal study (Tucker-Drob, Reference Tucker-Drob2011). Results suggested that there was a large contribution from global factors, with 39 percent of individual differences (see Chapters 4, 30), on average, accounted for by this domain-general factor. This finding converged with earlier work (e.g., Salthouse, Reference Salthouse1994) that also suggested that there were only a few independent factors that accounted for age-related changes in cognition. Domain-specific abilities, such as episodic memory, speed of processing, and abstract reasoning, also contributed, with estimates of 33 percent on average. Specific tasks accounted for the final proportion. These different levels of factors, from global, to domain-specific, to task-specific, are depicted in Figure 1.3, along with the factor loadings. A more recent meta-analysis (Tucker-Drob, Brandmaier, & Lindenberger, Reference Tucker-Drob, Brandmaier and Lindenberger2019) further supports the idea that domain-general factors play a large role in cognitive aging. Moreover, results suggest that the role may be even larger than indicated in previous work, accounting for potentially 60 percent of individual differences. Domain-general effects emerged not only for older adults but across the adult life span (Tucker-Drob, Reference Tucker-Drob2011), though the magnitude of the effects does tend to be larger for older than younger or middle-aged adults (Tucker-Drob et al., Reference Tucker-Drob, Brandmaier and Lindenberger2019).
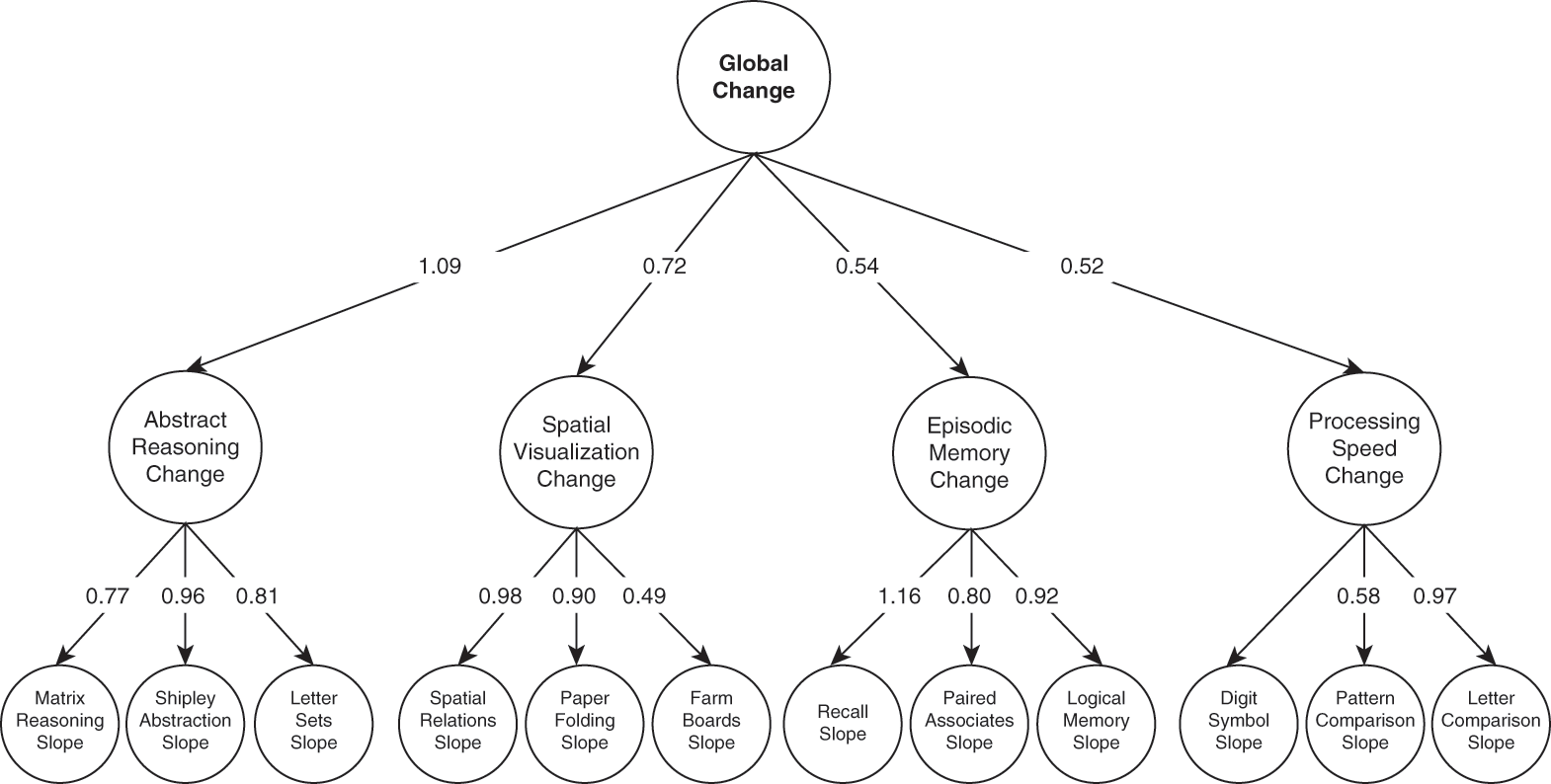
Figure 1.3 Global and domain-specific changes in cognition throughout adulthood.
Depiction of the factor solution that best accounts for changes in individuals’ performance on cognitive tasks over time. The top level displays the global factor, with domain-specific ones in the middle, and task-specific ones in the bottom row.
Despite the strong evidence for a global factor that contributes greatly to cognitive aging, there is yet to be strong support for what that factor is. As discussed by Kiely and Anstey (Reference Kiely, Anstey and Pachana2015), despite suggestions for various mechanisms, including dopaminergic function (see Chapter 5), inflammation, or “brain aging,” few of these mechanisms have been directly measured in these studies. Thus, stronger tests are needed, particularly including measures of putative mechanisms as well as longitudinal data from life-span samples.
Limitations of Models and Methodological Advances
One challenge for all models of cognitive aging is the difficulty in establishing the underlying mechanisms that are impacted by aging. For example, speed of processing has been suggested to reflect the integrity of the myelination of the neurons, as myelin contributes to the speed of neural transmission (Chevalier et al., Reference Chevalier, Kurth and Doucette2015; Salthouse, Reference Salthouse2000). Yet isolating even this mechanism is difficult. For one, multiple abilities seem to be inherently linked, in line with domain-general models of aging (see the section “Domain-General versus Domain-Specific Age-Related Changes”). Older adults who have more deterioration of myelin likely have other pronounced changes to the brain (e.g., cortical thinning). Does decline in one system lead to decline in another system, or do the systems independently exhibit age-related change? Given how much research proceeds in parallel, with different investigators focusing on different levels of analysis (e.g., changes to behavior; changes at the level of the neural system, such as failure to suppress the default mode network; changes to neurotransmitters; changes to the structure of a neuron, such as the number of synapses), it is difficult to gain an appreciation for the complex interactions across these mechanisms. Critically, it is not possible to manipulate the amount of myelin in an experimental way in humans; animal models are limited in the types of complex cognitive tasks they can accomplish.
Similarly, the largely descriptive nature of the models is a challenge. Particularly for the brain-based models of aging, it is difficult to determine the role of increased, or decreased, neural activity with age without knowing the trajectory of the responsiveness of the region or the associations with behavior over time. It could be the case that increases in neural activity initially reflect compensation and that the additional activity initially contributes to improved cognitive performance compared to had the region not been engaged. Or it could be the case that additional activation reflects an unsuccessful attempt at compensation that is not associated with enhanced behavioral performance (e.g., Cabeza and Dennis, Reference Cabeza, Dennis, Stuss and Knight2012). Moreover, the same level of neural activity could mean different things across different individuals, based on the initial level of cognitive ability, the amount of cognitive or brain reserve (see Chapter 2), and the point at which the individual is located along the trajectory of cognitive aging.
Cognitive neuroscience methods that allow some manipulation of neural activity in an experimental fashion are important for further understanding the effects of aging. Noninvasive methods such as repetitive transcranial magnetic stimulation (rTMS) or transcranial direct current stimulation (tDCS) can enhance or suppress neural activity in a region, allowing for an experimental test of whether a region contributes to task performance. An early rTMS study tested the role of bilateral recruitment in older adults, finding that inhibiting either hemisphere of the prefrontal cortex impaired older adults’ performance (Rossi et al., Reference Rossi, Miniussi and Pasqualetti2004). This finding was in contrast to the lateralized deficits in young adults; inhibiting only the hemisphere that was critical for younger adults impaired their performance. Thus far, the literature on neurostimulation is mixed. When benefits to task performance do emerge for older adults, they are generally modest. One meta-analysis, consisting largely of tDCS studies, found support for the benefits of neurostimulation for older adults, particularly when administered over multiple sessions (Hsu et al., Reference Hsu, Ku, Zanto and Gazzaley2015). More research is needed to better understand for what tasks and under which circumstances or methods of administration older adults can benefit from neurostimulation.
Despite the prevalence of fMRI research, the vast majority of work examining the neural basis for cognition in aging has focused on the use of univariate analyses to characterize age differences. Noted above, this metric has several limitations that preclude one’s ability to form widespread conclusions regarding the brain-behavior relationships in aging, absent such information as behavior, biological factors, cognitive history, etc. The recent emergence of multivariate methods including MVPA and representational similarity analyses offers promising avenues for future characterization of age-related neural function. For example, MVPA analyses have been used to show age-related reductions in the fidelity of memory representations in visual regions leading to memory impairments (Bowman et al., Reference Bowman, Chamberlain and Dennis2019; Koen, Hauck, & Rugg, Reference Koen, Hauck and Rugg2019). The benefit of these multivariate approaches to examining neural function lies in the fact that they enable a more mechanistic investigation of neural representations, neural fidelity, and processes that underlie age differences in cognition. Combined with approaches such as network analyses (e.g., Monge et al., Reference Monge, Stanley, Geib, Davis and Cabeza2018) they have the potential to elucidate not just age differences in how cognitive networks represent information, but the fidelity of whole-brain functional networks.
Conclusions
Despite the evidence for decline and losses with age, the growth in the use of neuroscience techniques that integrate brain and behavior has resulted in appreciation of additional processes that change with age. As a result of these methods, there also is increased appreciation of the flexibility with which the brain responds to the challenges posed by aging. Much work remains to be done to understand which types of experiences are protective and how these processes can be harnessed to extend the “health span” of the aging brain. The expanding research landscape, including longitudinal studies that incorporate neural measures, the wealth of interest in investigating interventions, and methodological advances, will result in additional rich insights into cognitive aging.