Introduction
Major depressive disorder (MDD) is a common and debilitating psychiatric disorder. It is among the leading causes of disability worldwide, with a lifetime prevalence of >16% (Kessler et al., Reference Kessler, Angermeyer, Anthony, De Graaf, Demyttenaere, Gasquet and Ustun2007). The pathophysiology of major depression remains elusive despite intensive efforts made to identify the neurobiological mechanisms. Over the last decades, structural magnetic resonance imaging (MRI) has been widely used in investigating morphological brain differences in MDD. The two largest meta-analyses based on cohorts worldwide in the ENIGMA (Enhancing NeuroImaging Genetics through Meta-Analysis) MDD Working Group (Schmaal et al., Reference Schmaal, Pozzi, Tiffany, van Velzen, Veer, Opel and Veltman2020) have reported specific abnormalities, such as thinner orbitofrontal cortex and smaller hippocampal volumes (Schmaal et al., Reference Schmaal, Veltman, van Erp, Samann, Frodl, Jahanshad and Hibar2016, Reference Schmaal, Hibar, Samann, Hall, Baune, Jahanshad and Veltman2017). Recent evidence also suggests abnormal grey matter (GM) volume and cortical thickness in various brain regions (Ancelin et al., Reference Ancelin, Carriere, Artero, Maller, Meslin, Ritchie and Chaudieu2019; Binnewies et al., Reference Binnewies, Nawijn, van Tol, van der Wee, Veltman and Penninx2021; Kandilarova, Stoyanov, Sirakov, Maes, & Specht, Reference Kandilarova, Stoyanov, Sirakov, Maes and Specht2019; Li et al., Reference Li, Zhao, Chen, Long, Dai, Huang and Gong2020). To date, structural abnormalities in MDD have not been reported consistently across studies. Moreover, most previous studies were performed with either voxel- or surface-based morphometry (Enneking, Leehr, Dannlowski, & Redlich, Reference Enneking, Leehr, Dannlowski and Redlich2020; Kocsis et al., Reference Kocsis, Holczer, Kazinczi, Boross, Horvath, Nemeth and Must2021; Li et al., Reference Li, Zhao, Chen, Long, Dai, Huang and Gong2020; Serra-Blasco et al., Reference Serra-Blasco, Radua, Soriano-Mas, Gomez-Benlloch, Porta-Casteras, Carulla-Roig and Cardoner2021), both of which were univariate methods that fail to consider spatial information (covariation) across voxels/vertices.
MDD has been investigated as a potentially brain network-based disorder (Gong & He, Reference Gong and He2015; Li et al., Reference Li, Friston, Mody, Wang, Lu and Hu2018; Menon, Reference Menon2011). Abnormalities in the structural covariation were reported in previous studies (Han et al., Reference Han, Cui, Wang, Chen, Li, Li and Chen2020; Wu et al., Reference Wu, Sun, Wang, Yu, Li, Peng and Wang2017) that calculated the correlation between GM volume within predefined regions of interest. However, this approach did not fully capture patterns of structural covariation. Recently, the source-based morphometry (SBM) has provided a novel approach to examine whole-brain structural covariance networks. It is a multivariate, data-driven approach that applies independent component analysis (ICA) to segmented GM images and extracts clusters of GM voxels covarying across participants as structural networks (Xu, Groth, Pearlson, Schretlen, & Calhoun, Reference Xu, Groth, Pearlson, Schretlen and Calhoun2009). SBM could identify covarying networks across distinct voxels, rather than focusing on each voxel separately. For brain imaging, SBM provides two major advantages: (1) it does not need to predefine regions of interest and (2) it separates artifacts and real brain change with high precision (Gupta, Turner, & Calhoun, Reference Gupta, Turner and Calhoun2019). Prior studies suggested that structural covariance patterns of brain regions are highly related with functional networks, demonstrating significant spatial overlaps (Spreng & Turner, Reference Spreng and Turner2013; Zielinski, Gennatas, Zhou, & Seeley, Reference Zielinski, Gennatas, Zhou and Seeley2010). Recently, researchers highlighted that structural networks were enriched with brain local molecular and cellular metadata, and that it is connected with more nuanced representations of functional networks and properties (Suarez, Markello, Betzel, & Misic, Reference Suarez, Markello, Betzel and Misic2020). Using SBM, the structural networks have been widely identified in cognitive and affective aspects, such as intelligence (Yoon et al., Reference Yoon, Shin, Lee, Hur, Cho, Sohn and Kwon2017) and suicidal behavior (Harenski, Harenski, Calhoun, & Kiehl, Reference Harenski, Harenski, Calhoun and Kiehl2020), as well as psychiatric disorders including autism (Pappaianni et al., Reference Pappaianni, Siugzdaite, Vettori, Venuti, Job and Grecucci2018), bipolar disorder (Singh, Arya, Agarwal, Shree, & Kumar, Reference Singh, Arya, Agarwal, Shree and Kumar2022), schizophrenia (Gupta et al., Reference Gupta, Calhoun, Rachakonda, Chen, Patel, Liu and Turner2015; Xu et al., Reference Xu, Groth, Pearlson, Schretlen and Calhoun2009), and MDD (Depping et al., Reference Depping, Wolf, Vasic, Sambataro, Thomann and Wolf2016; Watanabe et al., Reference Watanabe, Kakeda, Katsuki, Ueda, Ikenouchi, Yoshimura and Korogi2020; Yang et al., Reference Yang, Kumar, Nickerson, Du, Wang, Chen and Ma2021). These findings showcased advantages of SBM in understanding brain structural covariations in both healthy and psychiatric populations.
Although previous studies have investigated the structural covariance networks in MDD (Depping et al., Reference Depping, Wolf, Vasic, Sambataro, Thomann and Wolf2016; Kakeda et al., Reference Kakeda, Watanabe, Nguyen, Katsuki, Sugimoto, Igata and Korogi2020; Nguyen et al., Reference Nguyen, Kakeda, Watanabe, Katsuki, Sugimoto, Igata and Yoshimura2020; Okamoto et al., Reference Okamoto, Watanabe, Ngyuyen, Ikenouchi, Kishi, Iwata and Yoshimura2020; Watanabe et al., Reference Watanabe, Kakeda, Katsuki, Ueda, Ikenouchi, Yoshimura and Korogi2020; Wolf et al., Reference Wolf, Nolte, Hirjak, Hofer, Seidl, Depping and Thomann2016; Yang et al., Reference Yang, Kumar, Nickerson, Du, Wang, Chen and Ma2021), the exact pattern of abnormalities in structural covariance networks remains unclear. While an earlier study found decreased volume in the fronto-striatal network, cingulate, and lateral prefrontal regions (Depping et al., Reference Depping, Wolf, Vasic, Sambataro, Thomann and Wolf2016), extensive abnormalities in the salience network, medial temporal lobe network, default mode network, and central executive network have also been reported (Li et al., Reference Li, Seidlitz, Suckling, Fan, Ji, Meng and Liao2021; Watanabe et al., Reference Watanabe, Kakeda, Katsuki, Ueda, Ikenouchi, Yoshimura and Korogi2020). Such inconsistency might be due to the clinical heterogeneity in MDD patients and/or small sample size. Several studies were conducted with around 20 MDD patients (Depping et al., Reference Depping, Wolf, Vasic, Sambataro, Thomann and Wolf2016; Okamoto et al., Reference Okamoto, Watanabe, Ngyuyen, Ikenouchi, Kishi, Iwata and Yoshimura2020; Wolf et al., Reference Wolf, Nolte, Hirjak, Hofer, Seidl, Depping and Thomann2016), and the largest study to date examined only 145 MDD patients (Yang et al., Reference Yang, Kumar, Nickerson, Du, Wang, Chen and Ma2021). Small sample size limited the statistical power to detect subtle differences in brain structural abnormalities. Furthermore, previous studies were conducted with first-episode and drug-naïve (FEDN) MDD patients (Kakeda et al., Reference Kakeda, Watanabe, Nguyen, Katsuki, Sugimoto, Igata and Korogi2020; Nguyen et al., Reference Nguyen, Kakeda, Watanabe, Katsuki, Sugimoto, Igata and Yoshimura2020; Watanabe et al., Reference Watanabe, Kakeda, Katsuki, Ueda, Ikenouchi, Yoshimura and Korogi2020) or those with recurrent episodes (Depping et al., Reference Depping, Wolf, Vasic, Sambataro, Thomann and Wolf2016). However, the differences in structural networks between first-episode and recurrent patients remain poorly examined, given that inconsistent patterns of structural abnormalities have been reported in the two ENIGMA studies (Schmaal et al., Reference Schmaal, Veltman, van Erp, Samann, Frodl, Jahanshad and Hibar2016, Reference Schmaal, Hibar, Samann, Hall, Baune, Jahanshad and Veltman2017). In addition, the relationship between whole-brain structural networks and clinical symptoms in MDD has not been well established. To our knowledge, only one study has investigated this issue and reported a negative correlation between the ‘cingulate network’ and the Hamilton Depression Rating Scale (HAMD) total score (Depping et al., Reference Depping, Wolf, Vasic, Sambataro, Thomann and Wolf2016). Taken together, these findings highlighted the necessity to explore whole-brain structural covariance networks in MDD with a focus on the relationship between recurrence status and clinical symptoms based on larger well-powered samples.
In this study, we aimed to investigate brain structural abnormalities in regional GM volume and structural covariance networks associated with MDD within a large, multi-site sample drawn from the REST-meta-MDD Project in China (Yan et al., Reference Yan, Chen, Li, Castellanos, Bai, Bo and Zang2019). Voxel-based morphometry (VBM) was applied to acquire regional GM volumes and a novel SBM approach was carried out to obtain structural covariance networks based on GM images. We first compared regional GM volume and structural covariance networks between 1082 MDD patients and 990 healthy control (HCs) and then examined the effects of clinical characteristics (i.e. single v. recurrent episodes, symptom severity) on potential brain abnormalities in MDD patients.
Methods
Participants
A total of 1082 patients with MDD and 990 HCs from 20 sites of the REST-meta-MDD consortium were recruited for this study (Yan et al., Reference Yan, Chen, Li, Castellanos, Bai, Bo and Zang2019). All study sites obtained approval from their local institutional review boards and ethics committees. All participants provided written informed consent before participation. As reported previously, participants were identified as ‘patients’ if he/she had a past or current MDD episode according to the DSM-5 or ICD-10 (Yan et al., Reference Yan, Chen, Li, Castellanos, Bai, Bo and Zang2019). All participants in the HC group did not have a prior or current episode of any psychiatric disorder based on ICD-10 or DSM-5 at the time of investigation. We excluded 308 participants for the following reasons: (1) the information on gender, age, or education was unavailable; (2) poor imaging quality or bad spatial normalization (via visual inspection); (3) younger than 18 years old; (4) from a site with a sample size of patients or controls smaller than 10; and (5) sample replication (site S4 was duplicated from site S14). Severity of depression and anxiety in patients was assessed using the 17-item HAMD and the Hamilton Anxiety Rating Scale (HAMA) separately in 19 and 10 sites. Finally, we examined 874 patients from 17 sites with information on duration of illness, 1006 patients from 19 sites with HAMD scores, and 637 patients from 10 sites with HAMA scores. According to the episode and medical information from 637 patients of six sites, 430 patients were first-episode and drug-naïve (FEDN MDD group) and the rest 207 had experienced more than one episode of MDD (i.e. the recurrent group). See Table 1 for detailed information of all patients, HCs, FEDN, and recurrent MDD groups. The demographic and clinical information for participants in each site was summarized in online Supplementary Table S1.
Table 1. Sample characteristics of all depressive patients and healthy controls, and subgroup of patients

MDD, major depressive disorder; FEDN, first-episode and drug-naïve; HAMD, Hamilton Depression Rating Scale; HAMA, Hamilton Anxiety Scale.
Group differences were compared using two-sample t tests or χ2 test (gender only).
Data acquisition
MRI scans were acquired at each local site. Data acquisition parameters for T1-weighted structural images including the scanner, time repetition, and voxel size were replicated as in a prior study (Yan et al., Reference Yan, Chen, Li, Castellanos, Bai, Bo and Zang2019).
Voxel-based morphometry processing
Structural MRI data for all participants were preprocessed at each site, using the same DPARSF protocol (Yan & Zang, Reference Yan and Zang2010). In brief, a fully automatic technique for the computational analysis of differences in regional brain volume throughout the brain was conducted using the SPM methods (Statistical Parametric Mapping; Institute of Neurology, London, UK). The T1 images in native space were segmented into GM and white matter images and then modulated and spatially normalized using the Diffeomorphic Anatomical Registration Through Exponential Lie Algebra (DARTEL) toolbox (Ashburner, Reference Ashburner2007). To preserve the GM volume within each voxel, the processed images were modulated using the Jacobean determinants derived from the spatial normalization by DARTEL. The shared modulated GM images were then smoothed using an 8 mm full-width at half-maximum Gaussian kernel.
Source-based morphometry processing
With the preprocessed GM images, we carried out the SBM processing using the GIFT toolbox (https://trendscenter.org/software). The number of independent components (ICs, i.e. GM structural networks) was 17 (Kakeda et al., Reference Kakeda, Watanabe, Nguyen, Katsuki, Sugimoto, Igata and Korogi2020). We performed principal component analysis using a neural network algorithm (Infomax), which minimized mutual information of the network outputs in order to identify naturally grouping and maximally independent sources (Bell & Sejnowski, Reference Bell and Sejnowski1995). This process was repeated 20 times in ICASSO (http://research.ics.aalto.fi/ica/icasso/) to ensure the consistency and reliability of the resulting components. Of the 17 ICs, four were judged as artifacts based on the criteria defined by Xu et al. (Reference Xu, Groth, Pearlson, Schretlen and Calhoun2009). Recent studies suggested that cerebellar circuits not only consisted of motor-related networks, but also the networks related to cognition, emotion, and depression (D'Mello, Gabrieli, & Nee, Reference D'Mello, Gabrieli and Nee2020; Pierce & Peron, Reference Pierce and Peron2020; Sokolov, Miall, & Ivry, Reference Sokolov, Miall and Ivry2017). Thus, two ICs that mainly included cerebellar networks were also subject to subsequent analyses. As a result, a total number of 13 independent components were examined (see Supplementary Fig. S1).
In the calculation for ICs, all preprocessed images were arrayed into a 2D matrix, with each row representing a participant, and each column indicating a voxel. This matrix was then decomposed into two matrices by the ICA. The first matrix (mixing matrix, size = 2072 × 13) consisted of one participant per row and an IC per column. It involved ‘loading coefficients’ demonstrating how each structural component contributed to the GM characteristics of 2072 subjects. The loading coefficients were then transformed into z-scores before analyses. The second matrix (source matrix), which specified the relationship between the ICs and the voxels, was used for the visualization of components. To produce brain maps, the source matrix was reshaped back into a three-dimensional brain image and scaled to unit standard deviations (Z maps).
Statistical analyses
Voxel-based morphometry analysis
The VBM analyses were performed using the SPM8. Whole-brain GM differences between the MDD group and HCs were assessed using the two-sample t test. Age, gender, education, and the scanning site were included as covariates of no interest. This analysis yielded statistical parametric maps based on a voxel-level threshold of p < 0.001. The cluster-level family wise error (FWE) correction was applied for multiple comparisons (p < 0.05).
Source-based morphometry analysis
SBM analyses were performed using the SBM statistical tool and Matlab (2019b). The loading coefficients were compared between the MDD group and HCs, using two-sample t tests in SBM. Age, gender, education, and sites were included as covariates. Results were corrected with the Bonferroni method at p < 0.05 (i.e. threshold was p < 0.05/13, 13 means the number of ICs).
In addition, a validation analysis with 30 ICs was conducted as suggested in previous studies (Castro et al., Reference Castro, Hjelm, Plis, Dinh, Turner and Calhoun2016; Harenski et al., Reference Harenski, Harenski, Calhoun and Kiehl2020). Nine components contained sharp edges near the boundary of the brain or appeared primarily in regions that do not contain GM. Thus, we repeated above analyses for the rest 21 ICs.
Influence of recurrence status
To examine the influence of recurrence status on GM differences, we compared regional GM volume and structural covariance networks (loading coefficients) between FEDN MDD and HC participants, recurrent MDD and HC participants, and FEDN MDD and recurrent MDD participants. Two-sample t tests were performed with age, gender, education, and sites included as covariates. Bonferroni correction at p < 0.05 was applied to VBM and SBM results separately.
Association with clinical characteristics
Finally, to explore the association between clinical characteristics and structural abnormalities in MDD patients, we performed partial correlation analyses for symptom severity (HAMD, HAMA) and illness duration. Age, gender, education, and sites were included as covariates. Results were corrected with the Bonferroni correction method at p < 0.05 (i.e. p < 0.05/n, n represents the number of significant brain regions or networks) for VBM and SBM separately.
Results
Demographic results
Demographic information including age, gender, and education for MDD patients (n = 1082) and HCs (n = 990) were shown in Table 1 and online Supplementary Table S1. The two groups matched on both gender (χ2 = 1.48, p = 0.223) and age (t = −1.72, p = 0.085). The HC group received significantly more education as compared to MDD group (MDD group = 11.63 ± 3.71, HC group = 13.25 ± 3.73; t = 1.11, p < 0.001). On average, MDD patients had a 38-month course of disease and a severe level of symptoms (HAMD = 20.75 ± 7.48).
The FEDN and recurrent MDD groups matched on gender, age, and education (Table 1). The recurrent MDD group had significantly longer illness duration as compared to the FEDN MDD group (FEDN MDD group = 21.60 ± 37.69, recurrent MDD group = 94.67 ± 87.04; t = −14.78, p < 0.001). However, as compared to the recurrent MDD group, FEDN MDD patients scored higher in HAMD (FEDN MDD group = 21.44 ± 5.87, recurrent MDD group = 19.10 ± 7.85; t = 4.20, p < 0.001) and HAMA (FEDN MDD group = 21.52 ± 8.75, recurrent MDD group = 17.57 ± 8.65; t = 4.39, p < 0.001).
Voxel-based morphometry
Group differences in grey matter regional volumes
As compared to HCs, MDD patients showed reduced GM volume in bilateral superior temporal cortices (left, MNI x/y/z = −46.5/10.5/−9, cluster size = 293, t = −6.90; right, MNI x/y/z = 49.5/13.5/−4.5, cluster size = 141, t = −7.02), dorsal anterior cingulate cortex (MNI x/y/z = 0/9/24, cluster size = 435, t = −6.25), right middle cingulate cortex (MNI x/y/z = 13.5/−21/36, cluster size = 169, t = −6.28), right inferior frontal cortex (MNI x/y/z = 42/13.5/21, cluster size = 134, t = −6.85), and precuneus (MNI x/y/z = 21/−61.5/42, cluster size = 100, t = −5.90). Brain regions and locations of these effects were shown in Fig. 1 and Table 2. No increases of GM volumes were found in MDD patients.

Fig. 1. Decreased grey matter volume in patients with major depressive disorder compared to healthy controls. Results were shown with a voxel-level threshold at p < 0.001 and a cluster-level threshold at p < 0.05 (FWE corrected). Sagittal and axial slices were shown with the ICBM52 MNI brain template.
Table 2. Grey matter volume differences between 1082 patients with major depressive disorder and 990 healthy controls

These results were corrected with a voxel-level threshold of p < 0.001 and a cluster-level threshold of p < 0.05 (FWE corrected).
Influence of recurrence status on grey matter regional volumes
As compared to HCs (n = 990), neither FEDN (n = 430) nor recurrent MDD (n = 207) groups showed any significant difference in GM regional volume (FWE correction). When comparing FEDN MDD patients with recurrent MDD patients, no significant difference was observed either. Nonetheless, at an uncorrected level of p < 0.05, recurrent and FEDN patients showed similar GM atrophy (see online Supplementary Fig. S2).
Association of grey matter regional volumes with symptom severity and illness duration
In the MDD patients, partial correlation analyses revealed that increased depression severity (HAMD, n = 1066) was negatively correlated with GM volume in the right inferior frontal gyrus (r = −0.10, p bonferroni < 0.05) and precuneus (r = −0.12, p bonferroni < 0.05). There was no correlation between regional GM volumes and anxiety severity (HAMA, n = 637), or illness duration (n = 874).
Source-based morphometry
Group differences in grey matter networks
Significant between-group difference was observed in the ‘prefrontal network’ of the ICs (Fig. 2, Table 3). Regions of the prefrontal network included the middle, superior, inferior frontal gyri, and precentral gyrus (Table 4). The average z-score for this component in MDD patients (−0.08 ± 0.98) was significantly lower than that of HCs (0.08 ± 1.02). In the validation analyses based on 30 ICs, the difference in prefrontal network was also significantly different between MDD patients (−0.074 ± 0.969) and HCs (0.031 ± 1.013). In addition, the location of prefrontal network in this comparison, which included the middle, superior, and medial frontal cortices (online Supplementary Fig. S3), was similar to that emerged from the main analyses.
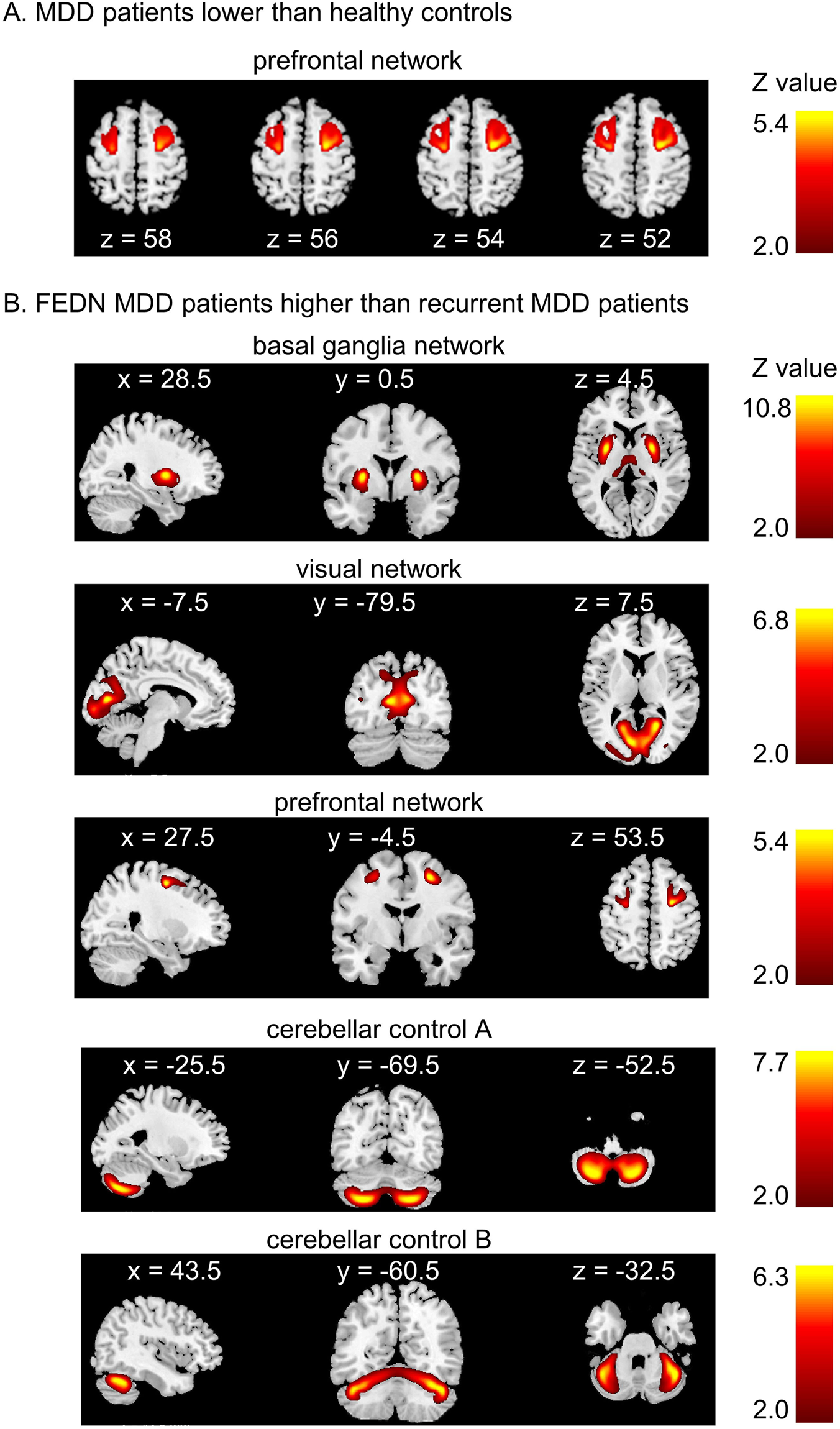
Fig. 2. Abnormal structural covariance networks in MDD patients v. healthy controls, and FEDN MDD patients v. recurrent patients. (a) The loading coefficients of the MDD patients in the prefrontal network were significantly lower than those of the healthy participants. (b) Compared to FEDN MDD patients, recurrent MDD patients showed lower loading coefficients in these five structural covariance networks.
Table 3. Differences in structural covariance networks between all MDD patients v. healthy controls, and FEDN patients v. recurrent patients

FEDN, first-episode and drug-naïve; MDD, major depressive disorder; HC, healthy controls.
The z-scores [mean (standard deviation)] of structural covariance networks were shown for each group. The p values were corrected using Bonferroni correction at p < 0.05.
Table 4. Location of structural covariance networks for which significant differences were observed in comparisons between MDD patients v. healthy controls, FEDN MDD patients v. healthy controls, recurrent MDD patients v. healthy controls, and FEDN patients v. recurrent MDD patients.

The volume of voxels in each area is provided in cubic centimeters (cc).
a Significant differences between recurrent MDD patients v. healthy controls.
b Significant differences between FEDN MDD patients v. recurrent MDD patients.
c Significant differences between FEDN MDD patients v. healthy controls.
Influence of recurrence status on grey matter networks
As compared to HCs (n = 990), the FEDN MDD group (n = 430) showed higher mean z-scores in the cerebellar A network (FEDN MDD = 0.21 ± 0.66, HCs = 0.01 ± 1.03; Fig. 2; Table 3) and lower mean z-scores in the superior temporal network (FEDN MDD = −0.20 ± 0.96, HCs = 0.04 ± 1.03). Locations of these structural covariance networks were shown in Table 4.
In addition, as compared to HCs (n = 990), the recurrent MDD group (n = 207) showed decreased z-scores in two cerebellar networks (network A, recurrent MDD: −0.22 ± 0.99, HCs: 0.01 ± 1.03; network B, recurrent MDD: −0.21 ± 1.05, HCs: 0.01 ± 1.02; Table 3), basal ganglia network (recurrent MDD: −0.88 ± 1.17; HCs: 0.003 ± 1.00), temporal network (recurrent MDD: −0.33 ± 0.95; HCs: 0.04 ± 1.03), prefrontal network (recurrent MDD: −0.17 ± 1.10; HCs: 0.08 ± 1.02), and visual network (recurrent MDD: −0.27 ± 0.94; HCs: −0.014 ± 1.00), and increased z-scores in parietal network (recurrent MDD: 0.29 ± 0.95; HCs: 0.04 ± 1.02). See Fig. 2 and Table 4 for the locations of these structural covariance networks.
The recurrent MDD group had significantly lower z-scores than the FEDN MDD group in five networks (see Table 3) including the basal ganglia network (FEDN: −0.07 ± 0.75; recurrent: −0.88 ± 1.17), visual network (FEDN: 0.08 ± 0.98; recurrent: −0.27 ± 0.94), and two cerebellar networks (cerebellar network A: 0.21 ± 0.66 and −0.22 ± 0.99; cerebellar network B: 0.14 ± 0.93 and −0.21 ± 1.05). In the prefrontal network, the z-scores in recurrent MDD patients (FEDN: 0.08 ± 0.99; recurrent: −0.17 ± 1.10, p bonferroni = 0.06) were modestly lower than those in FEDN MDD patients. Validation analyses showed similar results (online Supplementary Fig. S3).
Association of grey matter source volumes with symptom severity and illness duration
Partial correlation analyses showed that in the MDD group, increased depression severity (HAMD, n = 1066) was negatively correlated with z scores in the prefrontal network (r = −0.08, p bonferroni < 0.05). There was no correlation between the prefrontal network and either anxiety severity or illness duration. Given that several structural networks were different between the FEDN and recurrent MDD patients, we also investigated potential correlations in the FEDN MDD group and in the recurrent MDD patients, respectively. Results showed no correlation between GM networks and either symptom severity or illness duration in FEDN and recurrent MDD patients.
Discussion
To our best knowledge, this is the largest study to investigate structural covariance networks in MDD combining both VBM and SBM approaches in searching for brain structural biomarkers for MDD. VBM analyses found reduced GM volume in superior temporal cortices, cingulate cortices, inferior frontal cortex, and precuneus in MDD patients as compared to HCs. SBM analyses showed lower z-scores in the prefrontal network in MDD patients as compared to HCs. In addition, we identified extensive differences in structural covariance networks between FEDN and recurrent MDD patients. Furthermore, correlations with depression severity were also observed for specific brain regions and the prefrontal network. Our identification of abnormalities in the GM volume and structural covariance networks heightened the understanding of structural mechanisms in patients with MDD and the damages of recurrence on depressed brain structure.
The VBM findings showed decreased regional GM volume in the bilateral superior temporal cortices, dorsal anterior cingulate cortex, right middle cingulate cortex, right inferior frontal cortex, and precuneus in MDD patients. These findings are consistent with previous structural research on GM volume, cortical thickness, and surface area in depression (Kandilarova et al., Reference Kandilarova, Stoyanov, Sirakov, Maes and Specht2019; Schmaal et al., Reference Schmaal, Pozzi, Tiffany, van Velzen, Veer, Opel and Veltman2020; Serra-Blasco et al., Reference Serra-Blasco, Radua, Soriano-Mas, Gomez-Benlloch, Porta-Casteras, Carulla-Roig and Cardoner2021; Shen et al., Reference Shen, MacSweeney, Chan, Barbu, Adams, Lawrie and Whalley2021). Prior studies suggested that superior temporal regions responsive to sad stimuli, and its activation were lower in MDD patients (Fitzgerald, Laird, Maller, & Daskalakis, Reference Fitzgerald, Laird, Maller and Daskalakis2008). Dorsal anterior cingulate cortex was mainly associated with cognitive processes and evaluation of reward values (Bush et al., Reference Bush, Vogt, Holmes, Dale, Greve, Jenike and Rosen2002; Jahn, Nee, Alexander, & Brown, Reference Jahn, Nee, Alexander and Brown2016). Damage to the right inferior frontal gyrus impairs the performance on behavioral inhibition (Aron, Robbins, & Poldrack, Reference Aron, Robbins and Poldrack2014; Rolls et al., Reference Rolls, Cheng, Du, Wei, Qiu, Dai and Feng2020). Precuneus is a core region of the default mode network, and abnormalities in this region have been associated with low self-esteem and high rumination in depression (Cheng et al., Reference Cheng, Rolls, Qiu, Yang, Ruan, Wei and Feng2018). Our results also showed that severity of depression symptoms was associated with regional GM volume in the right inferior frontal gyrus and precuneus, which further suggest that these structural abnormalities were associated with various domains of depression.
Although VBM showed structural abnormalities related to MDD, it failed to detect abnormal GM clusters in the comparisons between the FEDN group and the recurrent MDD group. Uncorrected brain maps demonstrated that FEDN and recurrent patients had a similar trend and distribution of GM atrophy. These results suggested that FEDN and recurrent patients had similar abnormalities in local brain regions. To distinguish the differences related to episodes, a network perspective would be needed.
The SBM analyses identified 13 structural covariance networks in total and significant differences were found in seven of them, especially the prefrontal network (Table 3). The prefrontal network effect was significant in MDD group v. HCs, recurrent MDD v. HCs and recurrent MDD v. FEDN MDD group in this large, multi-site sample. This network encompassed regions mainly in the prefrontal cortex (Table 4), which has been associated with many processes such as cognitive control (Miller, Reference Miller2000) and emotional regulation (Dixon, Thiruchselvam, Todd, & Christoff, Reference Dixon, Thiruchselvam, Todd and Christoff2017). A previous study reported abnormal prefrontal networks in FEDN patients as compared to HCs (Kakeda et al., Reference Kakeda, Watanabe, Nguyen, Katsuki, Sugimoto, Igata and Korogi2020). In addition, the prefrontal network was negatively associated with levels of severity of depression symptoms. Therefore, our findings suggest that depression was associated with low structural covarying in prefrontal network. The prefrontal regions might not work together cohesively in depressive patients and thus they might encounter problems in emotion regulation and attention to negative stimuli (Disner, Beevers, Haigh, & Beck, Reference Disner, Beevers, Haigh and Beck2011; Wang et al., Reference Wang, LaBar, Smoski, Rosenthal, Dolcos, Lynch and McCarthy2008; Zhou et al., Reference Zhou, Chen, Shen, Li, Chen, Zhu and Yan2020).
Other structural covariance networks identified in FEDN MDD v. HCs or recurrent MDD v. HCs included basal ganglia network, temporal network, parietal network, visual network, and two cerebellar control networks. The basal ganglia network has been implicated in processing rewards (Haber, Reference Haber2008; Schultz, Tremblay, & Hollerman, Reference Schultz, Tremblay and Hollerman2000), and has been a critical neural marker for antidepressant and neuromodulating intervention in MDD and other psychiatric disorders (Bewernick et al., Reference Bewernick, Hurlemann, Matusch, Kayser, Grubert, Hadrysiewicz and Schlaepfer2010; Schneier et al., Reference Schneier, Slifstein, Whitton, Pizzagalli, Reinen, McGrath and Abi-Dargham2018). The temporal network has been known to engage in emotional memory (Murty, Ritchey, Adcock, & LaBar, Reference Murty, Ritchey, Adcock and LaBar2010); the parietal network is thought to play a role in attention control (Rohr et al., Reference Rohr, Vinette, Parsons, Cho, Dimond, Benischek and Bray2017); the visual network has been associated with visual processing biases in MDD (Desseilles et al., Reference Desseilles, Balteau, Sterpenich, Dang-Vu, Darsaud, Vandewalle and Schwartz2009). Both structural and functional abnormalities in these networks have been constantly observed in patients with MDD (Gong & He, Reference Gong and He2015; Wang et al., Reference Wang, Wang, Qu, Zhou, Li, Deng and Xie2016). By contrast, the cerebellar control does not have a well-established role in current literature of depression, although abnormal volume and function in the cerebellum have been reported in MDD (Bogoian, King, Turner, Semmel, & Dotson, Reference Bogoian, King, Turner, Semmel and Dotson2020; Kaiser, Andrews-Hanna, Wager, & Pizzagalli, Reference Kaiser, Andrews-Hanna, Wager and Pizzagalli2015). Structural abnormalities may contribute to decreases in brain functioning levels in affected regions (Paquola, Bennett, & Lagopoulos, Reference Paquola, Bennett and Lagopoulos2018; Suarez et al., Reference Suarez, Markello, Betzel and Misic2020). The abnormalities in structural covariance networks supported the idea that MDD might be a brain network-based disorder. A recent review study supported the more reliable biomarkers of psychiatric disorders with a network viewpoint (Sullivan, Olsen, & Widge, Reference Sullivan, Olsen and Widge2021). Scangos et al. (Reference Scangos, Khambhati, Daly, Makhoul, Sugrue, Zamanian and Chang2021) implemented the networks perspective with deep based stimulation, with focal stimulation identifying individual symptom-specific biomarker and a treatment region stimulating for symptoms improvement. As a result, network mapping and related biomarker stimulation reduced depressive symptoms and improved depression status sustainably. Taken together, brain structural networks might be valuable as potential intervention and treatment targets, especially for those that have been associated with cognition and emotion processes in depression.
It is not surprising that there is no direct overlap between the results of VBM and SBM. As explained by Xu et al. (Reference Xu, Groth, Pearlson, Schretlen and Calhoun2009), SBM differs from VBM in utilizing the interrelationship among voxels, spatially filtering artificial sources, capturing covariation of specific sources and minimizing the number of comparisons. Previous studies comparing VBM with SBM demonstrated that SBM might be more sensitive in detecting structural abnormalities (Harenski et al., Reference Harenski, Harenski, Calhoun and Kiehl2020; Pappaianni et al., Reference Pappaianni, Siugzdaite, Vettori, Venuti, Job and Grecucci2018; Xu et al., Reference Xu, Groth, Pearlson, Schretlen and Calhoun2009), while opposite findings were also reported (Kunst et al., Reference Kunst, Marecek, Klobusiakova, Balazova, Anderkova, Nemcova-Elfmarkova and Rektorova2019). When compared to surface-based morphometry (e.g. cortical thickness), results were also inconsistent (Kunst et al., Reference Kunst, Marecek, Klobusiakova, Balazova, Anderkova, Nemcova-Elfmarkova and Rektorova2019; Pappaianni et al., Reference Pappaianni, Siugzdaite, Vettori, Venuti, Job and Grecucci2018). In this study, the SBM method revealed effects that were not detected by VBM in basal ganglia, occipital lobe, and the cerebellar in the comparisons between FEDN and recurrent MDD patients, indicating that SBM might be more sensitive than VBM to morphometry abnormalities. Our results are consistent with Xu et al. (Reference Xu, Groth, Pearlson, Schretlen and Calhoun2009), as SBM could incorporate additional information about the grouping of the regions within several distinct, anatomically consistent sources. Future studies could apply SBM to identify the biomarkers of structural covariance networks in MDD and other neurobehavioral disorders.
Another interesting finding was that recurrent MDD patients, as compared to the FEDN patients, showed significantly greater abnormalities in the prefrontal network, basal ganglia network, visual network, and two cerebellar control networks. In addition, the difference in prefrontal network observed in the comparison between MDD group and HCs was driven by recurrent MDD participants. These results corresponded to brain structural and functional differences between first-episode and recurrent patients that were reported in previous literature (Schmaal et al., Reference Schmaal, Veltman, van Erp, Samann, Frodl, Jahanshad and Hibar2016, Reference Schmaal, Hibar, Samann, Hall, Baune, Jahanshad and Veltman2017; Yan et al., Reference Yan, Chen, Li, Castellanos, Bai, Bo and Zang2019). Previous studies suggested that MDD had a high rate of recurrence, with approximately 50–60% of patients suffering recurrence after an initial episode (Burcusa & Iacono, Reference Burcusa and Iacono2007; Nobbelin, Bogren, Mattisson, & Bradvik, Reference Nobbelin, Bogren, Mattisson and Bradvik2018). Our identification of abnormal structural covariance networks in recurrent MDD is a significant contribution to understanding recurrent MDD. These results suggest that treatment of recurrent MDD patients may need to focus more on brain networks.
The cross-sectional design of the present study prevents it from determining whether the abnormalities observed existed before or after MDD. Future longitudinal studies are needed to investigate the developmental trajectory of regional volumes and structural covariance networks, and to associate potential differences to symptom profiles and treatment responses. The heterogeneity in MDD patients who were recruited across 20 sites is also expected to be considerable. Whole-group analyses were limited by varied recurrence status, medication status, illness duration, and symptoms severity, which were tested for in the analyses. As we found, delineating differences in first-episode/recurrent and other associations would have revealed more neurobiologically characteristics and clinically meaningful findings. Finally, as previous studies showed, patterns of brain abnormalities and symptom profiles in adults and adolescents with MDD might be different (Rice et al., Reference Rice, Riglin, Lomax, Souter, Potter, Smith and Thapar2019; Schmaal et al., Reference Schmaal, Hibar, Samann, Hall, Baune, Jahanshad and Veltman2017). The data collected in this study do not allow a reliable investigation of brain morphometry in adolescents, because of the sample size and heterogeneity of sample sources, with 47 adolescent MDD patients from seven sites and 19 normal adolescents from two of those sites. Differences in structural covariance networks between adolescent MDD patients, adult MDD patients, and HCs require further investigation.
Conclusion
Our findings suggest that MDD patients exhibit abnormalities in both selected brain regions and certain structural covariance networks. VBM showed more scattered brain regions as compared to SBM when comparing between the whole MDD group and HCs, but SBM found more robust and widespread abnormalities in the recurrent MDD group as compared to FEDN MDD. Analysis of clinical characteristics suggests that diverse patient populations might present significant confounders in neuroimaging findings in major depression. Taken together, the VBM and SBM are expected to reveal different profiles of structural abnormalities related to MDD. Future longitudinal studies are needed to examine brain changes in regions/networks highlighted in the present study, and to establish links between differences in symptom profiles and treatment responses in MDD.
Supplementary material
The supplementary material for this article can be found at https://doi.org/10.1017/S0033291722000320
Acknowledgements
The REST-meta-MDD consortium was initiated and established by Dr Chaogan Yan, who came from the Institute of Psychology, Chinese Academy of Sciences. This consortium aimed to address limited statistical power and analytic heterogeneity of major depressive disorder. For this purpose, 25 research groups from 17 hospitals in China formed the REST-meta-MDD consortium and agreed to share final resting-state fMRI indices and the structural data of patients with depression and matched normal controls. Detailed information can be found in http://rfmri.org/REST-meta-MDD. Researchers in the REST-meta-MDD consortium include Chao-Gan Yan, Xiao Chen, Le Li, Francisco Xavier Castellanos, Tong-Jian Bai, Qi-Jing Bo, Jun Cao, Guan-Mao Chen, Ning-Xuan Chen, Wei Chen, Chang Cheng, Yu-Qi Cheng, Xi-Long Cui, Jia Duan, Yi-Ru Fang, Qi-Yong Gong, Wen-Bin Guo, Zheng-Hua Hou, Lan Hu, Li Kuang, Feng Li, Kai-Ming Li, Tao Li, Yan-Song Liu, Zhe-Ning Liu, Yi-Cheng Long, Qing-Hua Luo, Hua-Qing Meng, Dai-Hui Peng, Hai-Tang Qiu, Jiang Qiu, Yue-Di Shen, Yu-Shu Shi, Chuan-Yue Wang, Fei Wang, Kai Wang, Li Wang, Xiang Wang, Ying Wang, Xiao-Ping Wu, Xin-Ran Wu, Chun-Ming Xie, Guang-Rong Xie, Hai-Yan Xie, Peng Xie, Xiu-Feng Xu, Hong Yang, Jian Yang, Jia-Shu Yao, Shu-Qiao Yao, Ying-Ying Yin, Yong-Gui Yuan, Ai-Xia Zhang, Hong Zhang, Ke-Rang Zhang, Lei Zhang, Zhi-Jun Zhang, Ru-Bai Zhou, Yi-Ting Zhou, Jun-Juan Zhu, Chao-Jie Zou, Tian-Mei Si, Xi-Nian Zuo, Jing-Ping Zhao, and Yu-Feng Zang. We gratefully acknowledge all of them and all participants.
Financial support
This research was supported by the National Natural Science Foundation of China (32000760) to KW, China Postdoctoral Science Foundation Funded Project to KW (2019M662433), Postdoctoral Innovation Project (239735) in Shandong Province to KW, National Social Science Foundation of China (21AYY014) to YW, Fundamental Research Funds for the Provincial Universities of Zhejiang to YW, and China Scholarship Council to XZ.
Conflict of interest
None.