No CrossRef data available.
Article contents
Predicting local plasmon resonances and geometries using autoencoder networks in complex nanoparticle assemblies
Published online by Cambridge University Press: 30 July 2021
Abstract
An abstract is not available for this content so a preview has been provided. As you have access to this content, a full PDF is available via the ‘Save PDF’ action button.
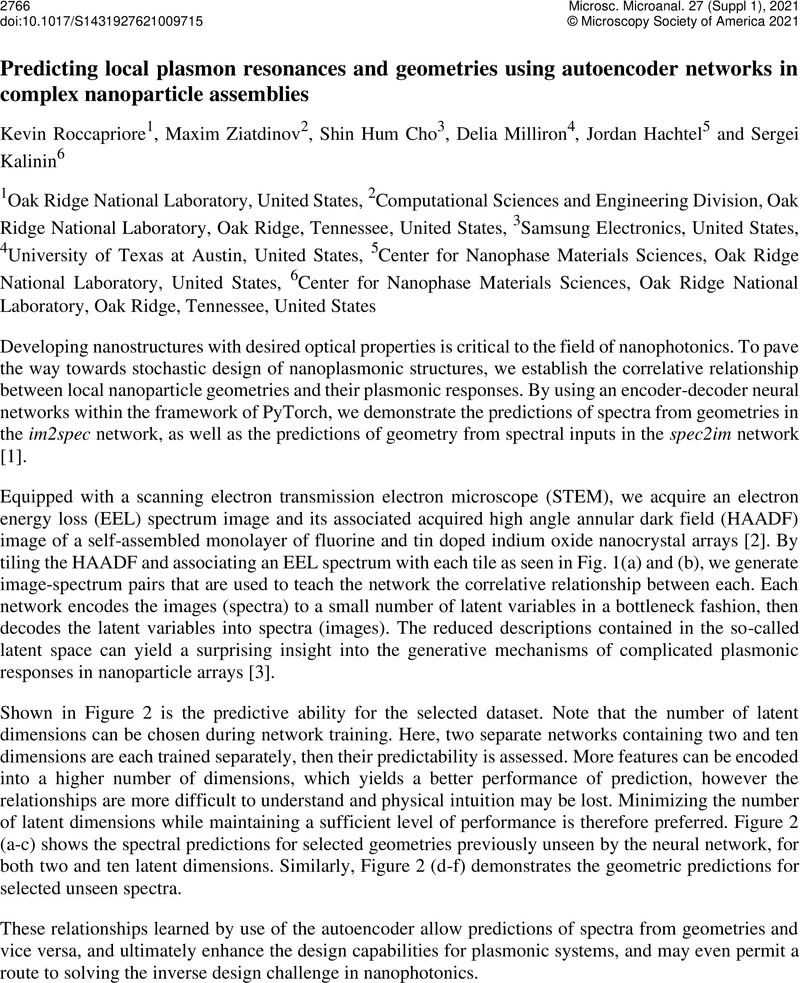
- Type
- Full System and Workflow Automation for Enabling Big Data and Machine Learning in Electron Microscopy
- Information
- Copyright
- Copyright © The Author(s), 2021. Published by Cambridge University Press on behalf of the Microscopy Society of America
References
This effort (ML and STEM) is based upon work supported by the U.S. Department of Energy (DOE), Office of Science, Basic Energy Sciences (BES), Materials Sciences and Engineering Division (K.M.R., S.V.K.) and was performed and partially supported (J.A.H., M.Z.) at the Oak Ridge National Laboratory's Center for Nanophase Materials Sciences (CNMS), a U.S. Department of Energy, Office of Science User Facility. S.H.C acknowledges (NSF, CHE-19052631609656, CBET-1704634, NASCENT, an NSF ERC EEC-1160494, and CDCM, an NSF MRSEC DMR-1720595), the Welch Foundation (F-1848), and the Fulbright Program (IIE-15151071).Google Scholar