No CrossRef data available.
Article contents
Neural Networks for Dose Reduced Reconstruction Image Denoising in Neutron Tomography
Published online by Cambridge University Press: 22 July 2022
Abstract
An abstract is not available for this content so a preview has been provided. As you have access to this content, a full PDF is available via the ‘Save PDF’ action button.
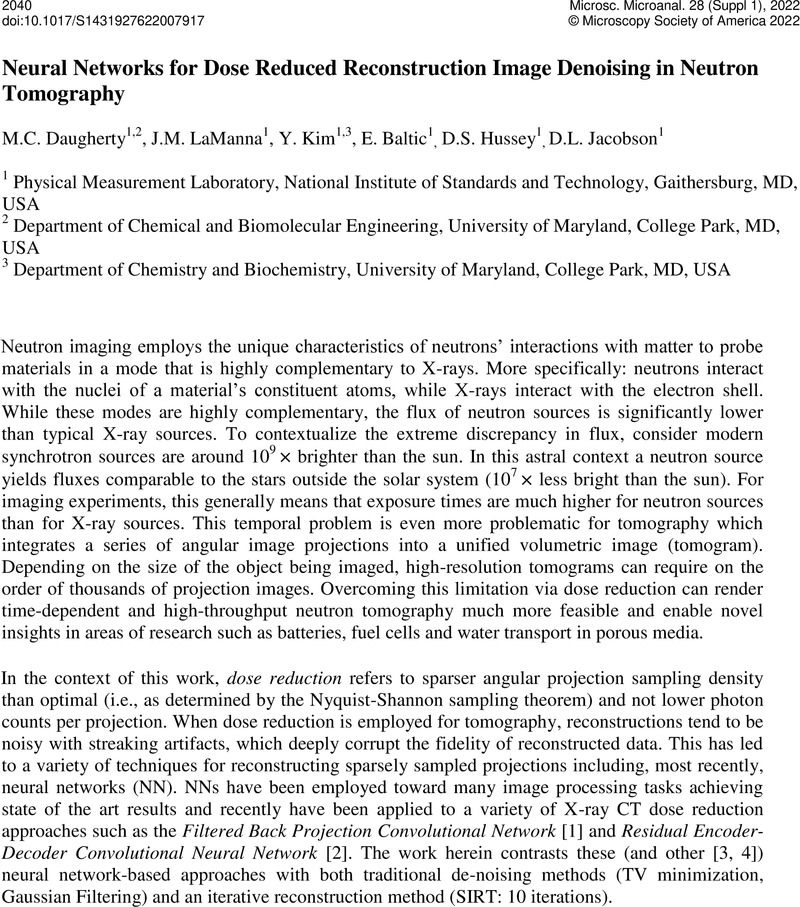
- Type
- Correlative Microscopy and High-Throughput Characterization for Accelerated Development of Materials in Extreme Environments
- Information
- Copyright
- Copyright © Microscopy Society of America 2022
References
Jin, K. H. et al. “Deep Convolutional Neural Network for Inverse Problems in Imaging”. IEEE Transactions on Image Processing 26.9 (2017), pp. 4509–4522. arXiv: 1611.03679.CrossRefGoogle Scholar
Chen, H. et al. “Low-Dose CT with a residual encoder-decoder convolutional neural network”. IEEE Transactions on Medical Imaging 36.12 (2017), pp. 2524–2535.CrossRefGoogle ScholarPubMed
Ledig, C. et al. Photo-realistic single image super-resolution using a generative adversarial network. 2017. arXiv: 1609.04802.Google Scholar
Lee, D. et al. “High quality imaging from sparsely sampled computed tomography data with deep learning and wavelet transform in various domains”. Medical Physics 46.1 (2019), pp. 104–115.CrossRefGoogle ScholarPubMed
Vo, N. T. et al. “Superior techniques for eliminating ring artifacts in X-ray micro-tomography”. Optics Express 26.22 (2018), p. 28396.CrossRefGoogle ScholarPubMed
Acknowledgements: Research was sponsored by the DEVCOM Army Research Laboratory (ARL) and was funded under Cooperative Agreement (CA) Number W911NF-20-2-0284. The views and conclusions contained in this document are those of the authors and should not be interpreted as representing the official policies, either expressed or implied, of the DEVCOM Army Research Laboratory or the U.S. Government. The U.S. Government is authorized to reproduce and distribute reprints for Government purposes -notwithstanding any copyright notation hereon.Google Scholar