No CrossRef data available.
Article contents
Materials and process discovery by correlated STEM imaging and spectroscopy with electrical testing
Published online by Cambridge University Press: 30 July 2021
Abstract
An abstract is not available for this content so a preview has been provided. As you have access to this content, a full PDF is available via the ‘Save PDF’ action button.
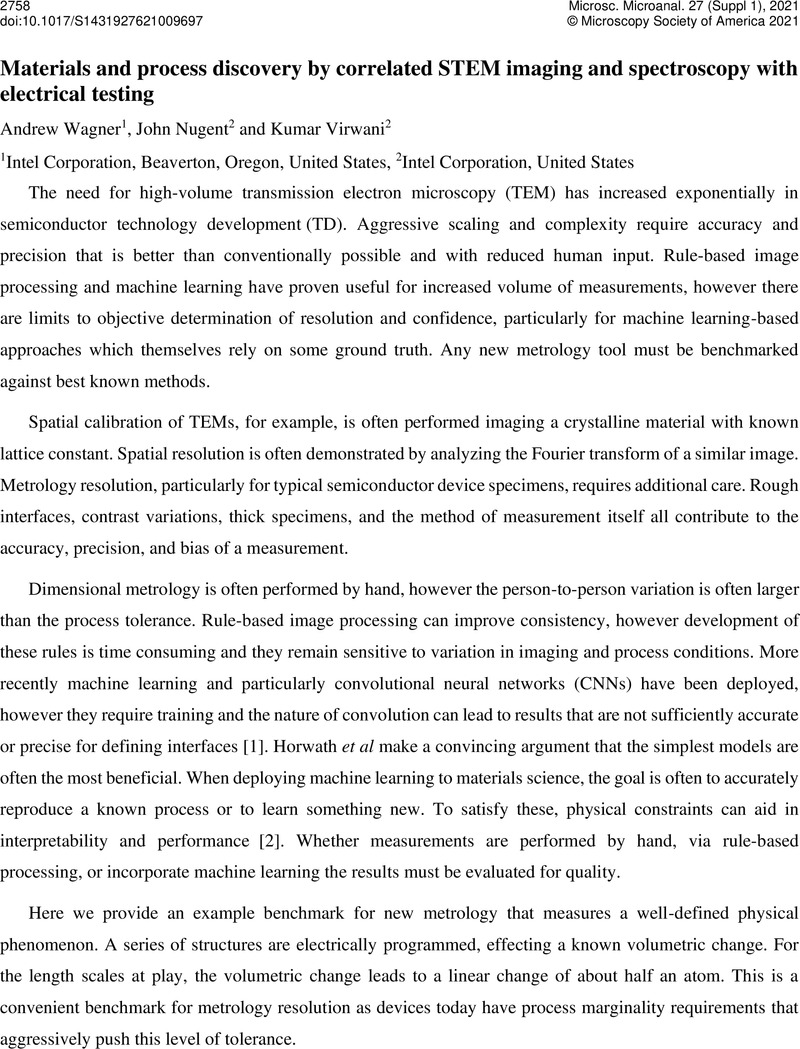
- Type
- Full System and Workflow Automation for Enabling Big Data and Machine Learning in Electron Microscopy
- Information
- Copyright
- Copyright © The Author(s), 2021. Published by Cambridge University Press on behalf of the Microscopy Society of America
References
Horwath, J., Zakharov, D., Megret, R. and Stach, E., "Understanding important features of deep learning models for segmentation of high-resolution transmission electron microscopy images," npj Comput Mater, pp. 6, 108, 2020.Google Scholar
Vasudevan, R., Ziatdinov, M., Vlcek, L. and Kalinin, S., "Off-the-shelf deep learning is not enough, and requires parsimony, Bayesianity, and causality," npj Comput Mater, pp. 7, 16, 2021.Google Scholar