No CrossRef data available.
Article contents
Deep Learning–Based Workflow for Analyzing Helium Bubbles in Transmission Electron Microscopy Images
Published online by Cambridge University Press: 30 July 2021
Abstract
An abstract is not available for this content so a preview has been provided. As you have access to this content, a full PDF is available via the ‘Save PDF’ action button.
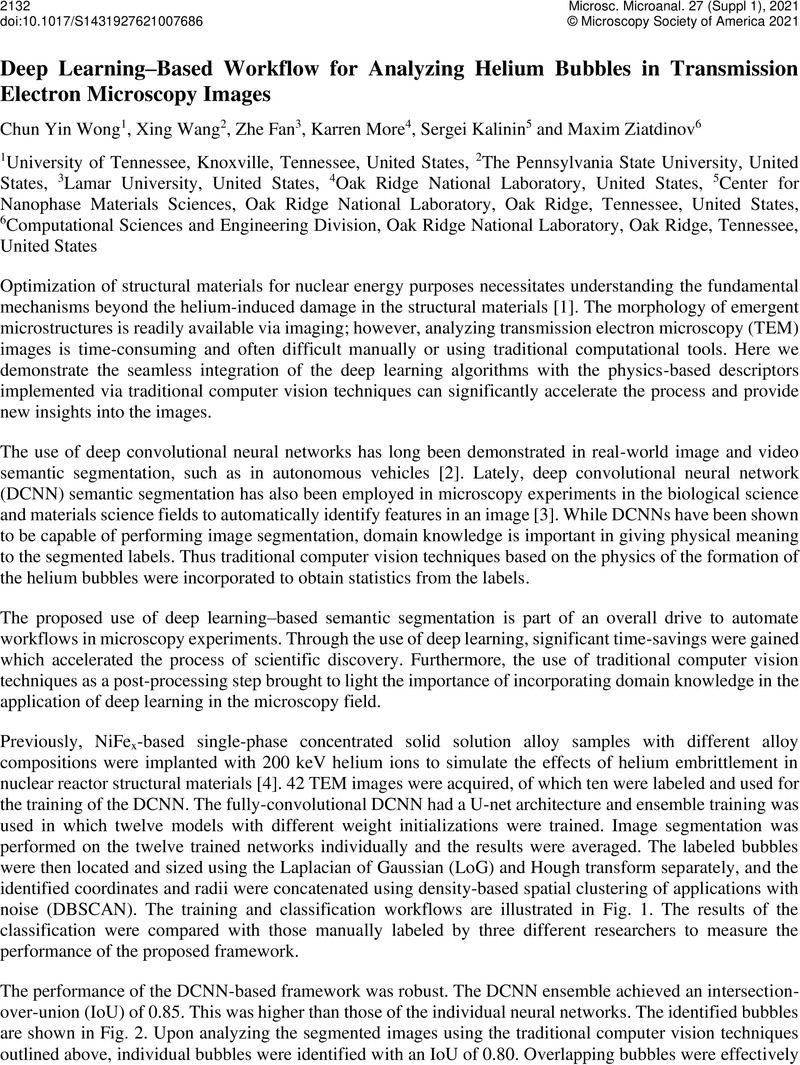
- Type
- Evaluation of Materials for Nuclear Applications
- Information
- Copyright
- Copyright © The Author(s), 2021. Published by Cambridge University Press on behalf of the Microscopy Society of America
References
Morishita, K., Sugano, R., and Wirth, B. D., “MD and KMC modeling of the growth and shrinkage mechanisms of helium–vacancy clusters in Fe,” Journal of Nuclear Materials, vol. 323, no. 2, pp. 243–250, Dec. 2003, doi: 10.1016/j.jnucmat.2003.08.019.CrossRefGoogle Scholar
Cordts, M. et al. , “The Cityscapes Dataset for Semantic Urban Scene Understanding,” in 2016 IEEE Conference on Computer Vision and Pattern Recognition (CVPR), Las Vegas, NV, USA, Jun. 2016, pp. 3213–3223, doi: 10.1109/CVPR.2016.350.Google Scholar
Ronneberger, O., Fischer, P., and Brox, T., “U-Net: Convolutional Networks for Biomedical Image Segmentation,” in Medical Image Computing and Computer-Assisted Intervention – MICCAI 2015, Cham, 2015, pp. 234–241, doi: 10.1007/978-3-319-24574-4_28.CrossRefGoogle Scholar
Wang, X. et al. , “Effects of Fe concentration on helium bubble formation in NiFex single-phase concentrated solid solution alloys,” Materialia, vol. 5, p. 100183, Mar. 2019, doi: 10.1016/j.mtla.2018.100183.CrossRefGoogle Scholar